Perspectives | 30 October 2018
With a portfolio in excess of €1300 billion in 2017, euro savings are firmly established as the preferred life-insurance product for people in France, thanks to their threefold benefits of guaranteed capital, attractive returns and instant liquidity. However, recent financial and regulatory developments raise questions as to the legitimacy of this product in the savings landscape. This is because a background of persistently low rates spells the end of traditional euro funds: prompted by the regulator, insurers are setting aside profit-sharing reserve [1] and are substantially reducing the interest rates paid. In parallel, the Solvency II Directive drives the point home, since the associated capital requirements reduce the margin of manoeuvre for achieving better returns through taking riskier positions.
This state of affairs has set French insurers off in a race against the clock to redirect policy-holders towards unit-linked products. In this move towards the end of an all-euro world, various strategies have been introduced (commercial premiums, incentive policies, the differentiation of fees and so on) and are slowly starting to see results. But the impacts remain limited: despite unit-linked sales of 28% in 2017 compared with 20% in 2016, euro contracts still predominate. Insurers struggle to convince a public that is broadly unfamiliar with the language of unit-linked products, and remains nervous about giving up guaranteed capital. Clearly, the options of manager-guided or discretionary management are contributing to the democratisation of risk, so insurers are trying to promote these, while restructuring their distribution networks. However, these expensive options are only accessible to high net worth clients. So how can risk-awareness be increased among a broader spectrum, and the general public be brought on board at low cost?
A new distribution channel may be a game-changer: robo-advisors. These digital advisors are programmed to execute every step of the customer’s asset management experience, including registration, the analysis of the investor’s profile and objectives, advice on asset allocation, and portfolio monitoring and rebalancing. Launched around a decade ago in the United States with the company Betterment as a forerunner, robo-advisors emerged in France more unobtrusively between 2012 and 2016. Today, you can still count the main players on one hand: Yomoni, WeSave, Fundshop, Advize and Marie Quantier. A study conducted by Statista estimates that in 2017, these French robo-advisors handled only $214 million – just a drop in the savings ocean (€1 676 billion in 2017). But the interest shown by the main French savings institutions (such as BNP Paribas AM, Aviva France, and Crédit Mutuel Arkéa), which are mainly in the running-in or pilot phase of robo development, demonstrates the strategic challenge that this new distribution channel represents.
The very expression “robo-advisor” fosters the fantasy of an artificial intelligence capable of replacing the traditional asset investment advisor. Certainly, it represents a revolution in terms of the experience offered to savers: as on e-commerce sites, the pathway is entirely digitised and based on ergonomic interfaces. And it’s the opportunity offered by this cutting-edge distribution channel that has launched the race for robo-advisors. But is this technological innovation restricted to a digitised experience, or can we expect the emergence of powerful algorithms which surpass traditional management and provide a more personalised approach? And should we be worried that this dehumanization of the advisor role risks a breakdown in the insurer’s duty of advice? Certainly, a number of lessons can already be drawn from the practices of French players. Analysis of the customer journey suggests that there are three key stages in which robotisation makes, or could make, a difference: profiling clients, allocating portfolios and giving advice to savers.
In practice, clients are profiled using a score calculated on the basis of the answers they give to a questionnaire. If subjects tackled in this questionnaire are in line with French regulator’s (ACPR) recommendations in terms of the duty of advice to purchasers[2], the construction of a score and the attribution of risk profiles is carried out beforehand and based on business expertise. Machine learning could overcome this limit in the future: unsupervised learning techniques, for example, would make it possible to improve customer clustering; another approach could use predictive algorithms which, based on the optimisation of KPIs such as customer satisfaction or conversion rates, could be used to adjust profiles retrospectively.
However, there appear to be two obstacles to these prospects. First, recent developments in data protection curb the use of such ‘black box’ machine learning methods: under article 13 of the General Data Protection Regulation (GDPR), the data controller must be capable of explaining the origins of an automated decision. It may be possible to turn to a new generation of algorithms, such as LIME[3], which can make complex models easy to interpret and provide a de facto educational tool in the distribution chain. Secondly, the relevance of the profile is limited by the nature of the information provided in the questionnaires, which does not entirely represent the client’s expectations. How can we enrich this data with discriminative information? Increasing the complexity of the questionnaire would encumber the client journey, with the risk of discouraging a generation used to instant gratification.
However, if we look at the detection of fraudulent behaviour, the European Banking Authority offers a potential solution, recommending the use of information from external sources[4], for instance from social media. In the name of the duty to advise, the regulator might take inspiration from this and consider diversifying the sources of information… provided, of course, that the limits set by the GDPR are not exceeded. And bankinsurers, who are struggling to keep up with traditional insurance companies in the pursuit of unit-linked products, could make the most of the opportunity to cross-reference the data with their savers’ banking information.

Figure: Simplified representation of results of the predictive profiling algorithm
LIME is used to identify the weight of the variables in the black box decision process models. In this illustration, LIME tells the user that the machine learning model has classified an individual as belonging in risk profile no 3 mainly due to the answers to the investment questionnaire under the headings “financial_assets”, “investment_goals”, “investment_experience”, and “investment_horizon”. However, the age entered by the individual counts against his allocation to this risk profile: this information could constitute a warning note in the subscription process.
The architecture adopted by French robo-advisors consists of associating a model portfolio to each risk profile. How automated is this stage? First let’s note that robo-advisors have opted for passive management, prefering exchange-traded funds in order to limit management costs. The construction of model portfolios relies on traditional management methods, generally with periodic rebalancing, which still leaves scope for human intervention to adjust positions. It appears, therefore, to be too soon to claim that there is full robotisation in the French market, firstly because the portfolios proposed are not individualised and secondly because model portfolios are not designed on the basis of an internal decision taken by an artificial intelligence application. However, some functionalities may strengthen the process of robotisation. For example, Marie Quantier stands out from its competitors by offering investors tools to help customise their allocation. Across the Atlantic, some robo-advisors have automated the so-called tax loss harvesting strategy, which consists of realising latent unrealised losses and re-investing in correlated securities in order to reduce taxation while maintaining the same exposure. However, promising these prospects appear, individualised management by robot brokers doesn’t yet seem to be on the agenda.
The democratisation of saving and investing will first entail raising general awareness of financial risk. Now, for the general public, investment is something for insiders; and investment products are denounced for their lack of transparency. The most recent regulatory developments, with the European PRIIPS regulation and the MiFID (ii) Directive, have contributed to standardising and clarifying the information provided. At first sight, distributors could see this as an additional constraint on the distribution process. On the contrary: could they not take advantage of this constraint in the design of robo-advisors? For example, some key aspects defined in the PRIIPS, such as transparency on costs, the summary risk indicator (SRI) and the Performance (“r”) scenarios all help to deliver advice comparable to that provided by an asset manager. The production of these indicators may be automated, so that robo-advisors have a decision-making tool for investors, just like an advisor who steers savers in their allocation choices.
And why not re-think the entire robo-advisor architecture? To increase the personalisation of portfolio allocations, Musto and al. .[5]suggest the use of algorithms known as case-based recommender systems (CBRS). These algorithms adopt a method of resolution based on the use of previously solved problems. In this instance, a search of “similar” individuals who had received human advice would be conducted, and the optimal portfolio would be chosen from among the portfolios of this sub-group. To reduce the number of proposals to users, Musto and al. suggest maximising the weighting of the historical yield of the candidate portfolios, subject to a portfolio diversification constraint. This approach therefore allows for considerable customisation and diversity in the allocations suggested, exceeding that of the traditional robo-advisor architecture. Such an approach may appeal to users, since the client pathway recalls the customer experience offered by websites like Netflix or Amazon, where products are suggested on the basis of collaborative algorithms similar to the CBRS.
Whatever routes are taken by robo-advisors, they will be subject to approval by the regulator, whose opinion in this area should therefore be followed closely. In several notices it has expressed its interest in FinTechs and automated advice, recognising their value in the transition to unit-linked products. However, there are certain reservations on the risks which may arise, in particular in terms of the failure of black box tools. In 2016 Banque de France governor, François Villeroy de Galhau, stated: “The contributions of digitisation to financial services are undeniable […]. Nevertheless, they are accompanied by risks which must be managed. There must be a closer analysis of vulnerabilities, the regulations must be adapted, the security of transactions maintained, and prudential oversight must be both flexible and vigilant […]. These are the challenges that the authorities are preparing to meet.”[6]
If robo-advisors are to have a role in encouraging savers to embrace unit-linked products, the industry also has to play their part in meeting such challenges.
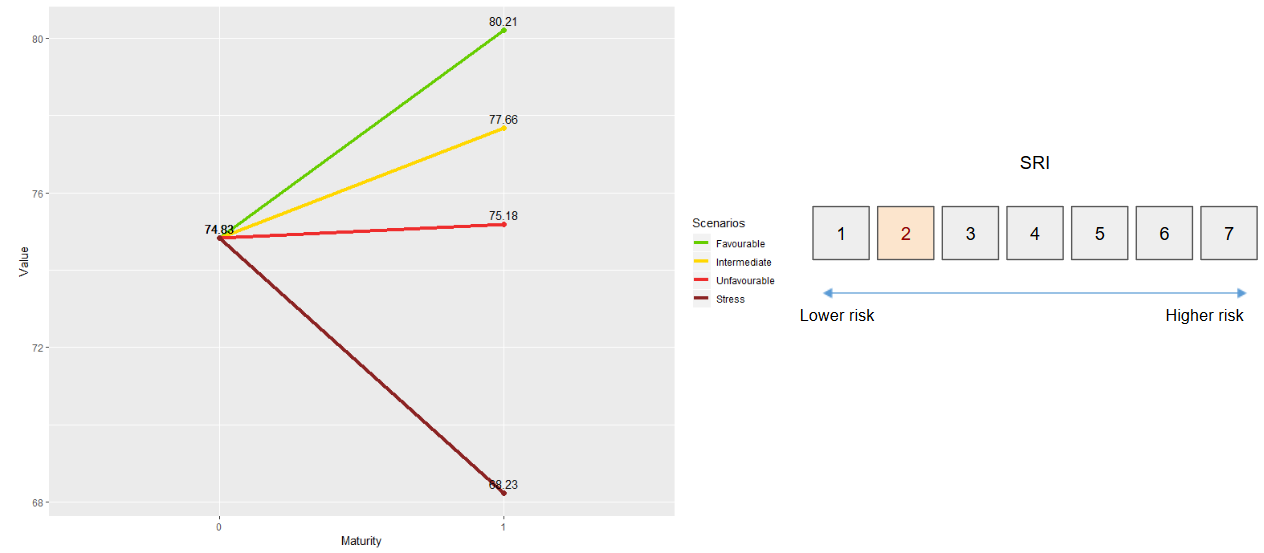
Figure: Portfolio performance scenarios and SRI
Savers may be guided in their portfolio selection process by indicators inspired by the PRIIPS Regulations. Performance scenarios (shown on the left) help to give substance to the concepts of risk and reward for the customer. The summary risk indicator (on the right) provides a comparison between the shortlisted funds.
Article written by Florian Montanier (Junior Actuary, Mazars France) and Axel Truy (Manager, Mazars France).
[1] Regulatory provisions allowing profits to be placed in a reserve for subsequent use during the eight years after its constitution. Insurers constitute this reserve in anticipation of adverse circumstances in future.
[2] Recommendation on the KYC information gathering in the context of the duty to provide life-insurance advice – 2013-R-01 of 8 January 2013 – ACPR
[3] Local Interpretable Model-Agnostic Explanations
[4] https://www.eba.europa.eu/documents/10180/1720738/Report+on+Innovative+uses+of+data+2017.pdf
[5] Musto C., Semeraro G., Lops P., Gemmis M. D. & Lekkas G. (2015), Personalized finance advisory through case-based recommender systems and diversification strategies, Decision Support Systems (Elsevier)
[6] Revue de Stabilité Financière 20, La Stabilité Financière à l’ère du numérique, 2016