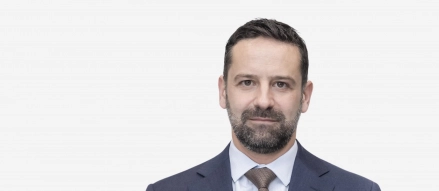
Benchmark study of approaches to estimate probability of default in the context of climate risk
Benchmark study of approaches to estimate probability of default in the context of climate risk
Recently, initiatives to tackle climate-related and environmental risks in the financial services industry have begun across the world. These initiatives followed the adoption of the United Nations Paris Agreement on climate change, the 2030 agenda for Sustainable Development and the European Green Deal.
Stress testing and scenario analysis are a common framework proposed by different regulatory and supervisory bodies across various countries to assess the impact of climate-related risks on the financial system. Countries like the United Kingdom and France, having started working on pilot climate stress test exercises, are leading by example. However, no consensus regarding the best methodology to use in this context has been reached as of today.
In this article, Mazars presents the results obtained by implementing two methodological approaches to estimate the sectoral probability of default (PD) parameters in the context of climate risk stress testing:
- The first methodology studied was developed as part of the UN Environmental Programme (UNEP) Finance Initiative when piloting the implementation of recommendations outlined by Task Force on Climate-related Financial Disclosures (TCFD)[1]; and
- the second corresponds to the approach followed by the Autorité de Contrôle Prudentiel et de Résolution (ACPR) in its 2020 climate risk stress test pilot exercise[2].
Common basis of the two approaches
Both approaches are built upon a well-established PD methodology, known as the Merton Framework, where the default dynamics are captured via macroeconomic and financial risk drivers. This allows one to project the expected default rate according to the anticipated movements of these risk drivers. The Merton model has been widely used in the industry to derive the IFRS 9 forward-looking and point in time PDs and perform stress testing.
With this foundation, the two approaches differ in the way the framework has been adapted for climate stress testing. In particular, the approaches differ in the mode of incorporation of climate risk factors into the PD calculations. The results obtained by Mazars show that these two distinct approaches can yield similar results.
Sectoral sensitivity approach
In this approach, climate variables and their respective sensitivity coefficients -estimated using a scorecard – are added into the sectoral PD Merton models. As previously mentioned, the method was developed by the UNEP, and we refer to it as the “sensitivity approach” throughout this article.
This approach has two clear advantages:
- it requires few additional inputs; and,
- one can leverage the work performed by the UNEP when determining the hierarchy of the sensitivities (i.e. which sectors have a high climate risk impact vs low).
However, a key drawback is the lack of guidance or references for the level of the sensitivities, so the determination of these inputs is based on expert judgement.
Sectoral approach
The French regulator, the ACPR, produced a set of sectoral Gross Value Added (GVA) forecasts and existing leveraged models from banks when building its climate risk stress testing framework. Climate PD forecasts were produced using the sectoral forecasts given by the ACPR along with either the bank’s pre-existing models or with newly developed models. It is worth noting that when the bank’s PD models did not include GDP as a default risk driver, new models were developed to add it. This variable was necessary to produce forecasts with the sectoral granularity required for the climate risk stress testing assessment. Similarly, the BoE produced a set of sectoral GVA forecasts for its 2021 Climate Biennial Exploratory Scenario (CBES), accounting for different economic sectors’ varying degrees of exposure to climate risks.
This approach has three advantages:
- the work performed by the ACPR can be used as a reference;
- generally, results can be generated with slight modifications to existing models; and
- the model does not introduce additional parameters.
On the other hand, the disadvantages include:
- a strong modelling assumption: “models are still pertinent with sectoral data”; and,
- the requirement of sectoral macro-economic forecasts.
Our study
Mazars implemented both methodologies in an attempt to understand whether the methodologies yield consistent results. In practice, our study consisted of the following steps:
Step 1. Calibrating a PD model to the historical default rate series of the global corporates segment, which, by design, included a GDP variable.
Step 2. Applying the PD model (obtained in step 1) to the ACPR sectoral forecasts to derive results from the sectoral approach.
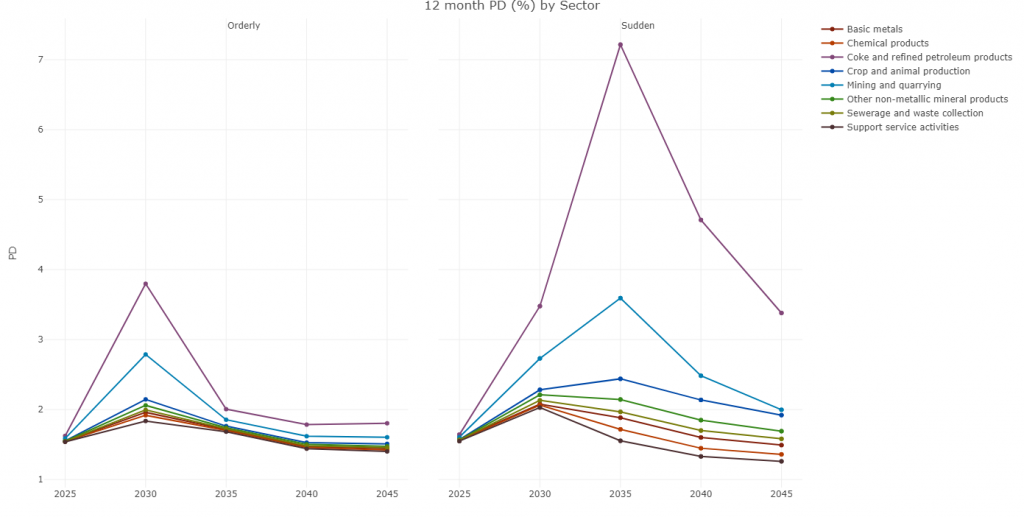
Figure 1. Forecast of 12-month PD by sector for two ACPR scenarios
Figure 1 illustrates the 12-month PD forecast at each reporting date for two ACPR scenarios: orderly and sudden. To provide some context, the ACPR orderly scenario reflects the French roadmap designed to fulfil the commitments made under the Paris Agreement. On the other hand, the sudden and delayed scenarios consist of a sharp increase in the carbon price, the latter starting only in 2030.
As expected, one can see that the risk parameter (i.e. PD) increases with the “severity” of the scenario. A similar fact can be highlighted for the segments: a higher PD is observed for those segments expected to have stronger negative impacts from climate change.
Step 3. Calibrating the sensitivities (in the sensitivity approach) of each sector to climate variables. The sensitivity for each sector was estimated such that the difference in 12-month PD at each calculation date between the results from the sensitivity approach and the sectoral approach is minimised.
Mazars selected the annual difference in carbon price as the climate variable. Other transformations of carbon price were analysed but won’t be considered in this article because the results obtained were consistent with those discussed here.
Figure 2 illustrates the 12-month PD by sector at three reporting dates (2035, 2040 and 2045) utilising the two alternative approaches.
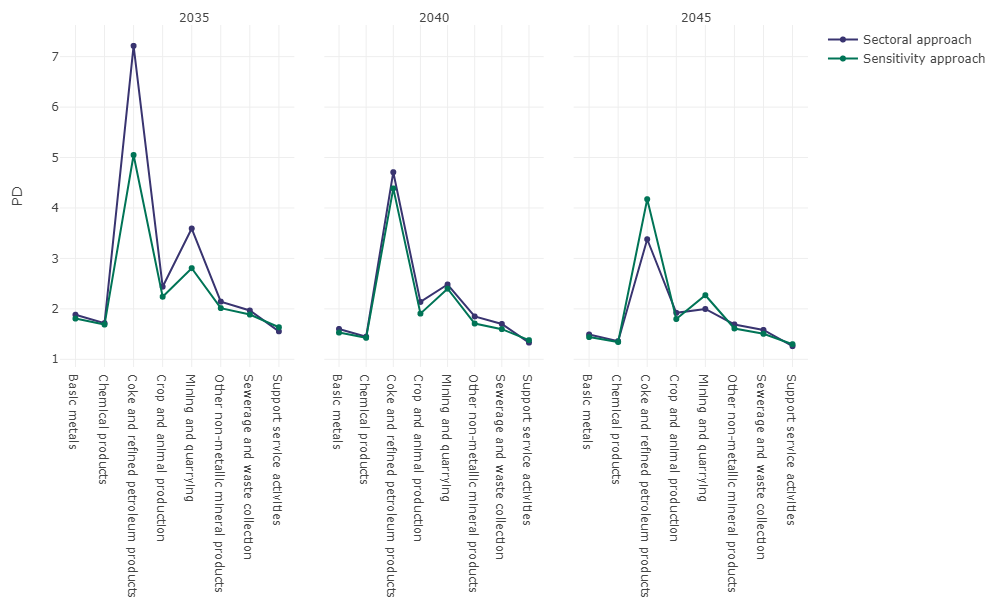
Figure 2. 12-month PD in 2035, 2040 and 2045 for the sudden scenario using the difference in the carbon price
The left graph in Figure 2 illustrates the 12-month PD by sector for the sudden scenario as at 2035 and shows that the two approaches yield consistent results for five out of the seven sectors analysed. There is, however, a significant difference when comparing the two most sensitive sectors: coke and refined petroleum and mining and quarrying.
On the other hand, for the 2040 reporting date, the results show convergence for all the sectors (refer to the graph in the centre in Figure 2). Small discrepancies were observed for the 2045 reporting date (refer to the right graph in Figure 2). These can be explained by differences in the information carried by the selected climate risk variables (GVA forecasts vs difference in carbon price).
In an attempt to reduce the discrepancies between the two approaches, an additional analysis was performed by implying a climate factor from the GVA forecast of the most affected sector (i.e. coke and refined petroleum products) relative to the GDP forecast. We will refer to this variable as the ACPR implied climate factor and, for each year, define it as follows:

where and
are the annual differences of each variable respectively.
The results obtained using the ACPR implied climate factor for 2035, 2040 and 2045 are shown in Figure 3.
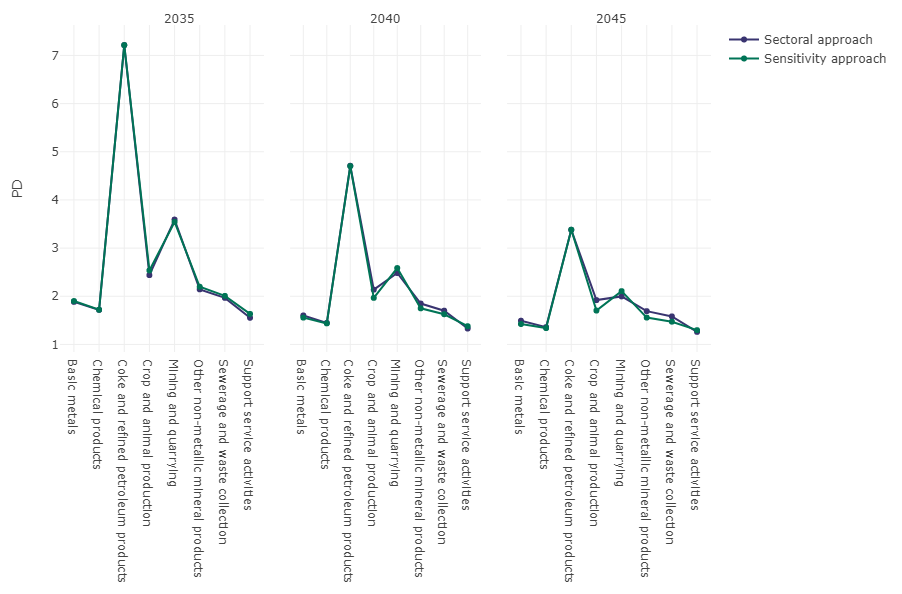
The sensitivity approach results fit the sectoral GVA approach results almost perfectly, particularly for the coke and refined petroleum products sector. The latter behaviour was expected as the ACPR implied climate factor was built using the GVA forecasts for this sector. We observed slight discrepancies for some sectors in the sudden and delayed scenarios and when looking at all reporting dates.
Step 4. Analysis of the results.
Table 1 summarises the results of the calibrations performed in step 3, along with the ratio of the sectoral GVA forecast from the ACPR divided by the GDP forecast (sector independent). These ratios are used to benchmark our results as they represent the ACPR expected climate impacts for each of the sectors.
Furthermore, the values in Table 1 have been colour-coded such that the sectors that are highly impacted by the climate risk are highlighted in red, and those that are less impacted are highlighted in green.
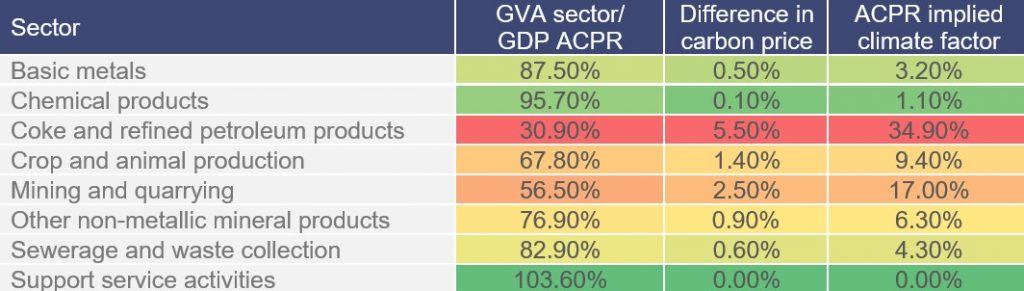
The results obtained when calibrating the sensitivities with both variables (difference in carbon price and the ACPR implied climate factor) are consistent. With both methods, the most impacted sector was coke and refined petroleum products, followed by mining and quarrying, and the least impacted sector was support service activities. The same conclusion is reached when comparing the sensitivities with the GVA/GDP ratios[3].
Finally, we compared our results with the scorecard ratings provided in the UNEP/TCFD study “Beyond the Horizon”[4]. Table 2 outlines the mapping applied and the associated UNEP/TCFD ratings by sector.
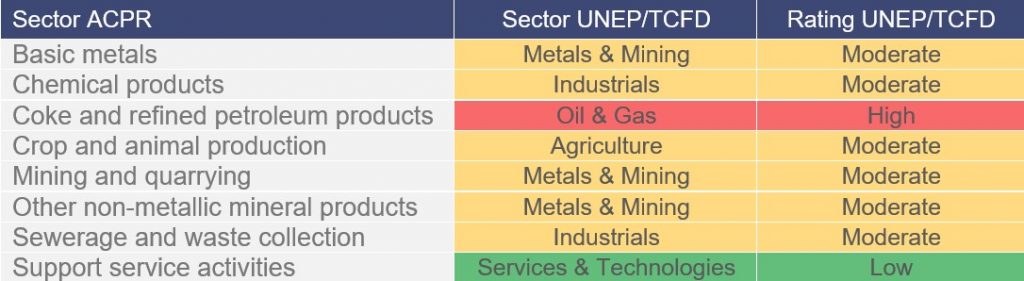
Overall, the results presented in Table 1 appear to be consistent with the ratings summarised in Table 2 except for the Metals & Mining sector. The UNEP rated this sector as “Moderate”, whereas the GDP ratios from ACPR indicate the segment is treated as “Highly Moderate”. Notice that there is a clear difference between the ratios of this sector (orange rows in Table 1) and the rest of the sectors rated as “Moderate” (yellow rows in Table 2). The discrepancy could be explained by differences in the segmentation approaches of the two sources.
Conclusion
Based on the results obtained, we conclude that both sensitivity and sectoral approaches can yield equivalent results, particularly when both methods use the same climate risk information to project PDs.
[1] Extending Our Horizons by J. Colas, I. Khaykin, A. Pyanet and J. Westheim, April 2018, available here.
[2] Analysis and synthesis no. 122: The main results of the 2020 climate pilot exercise, by ACPR, May 2021, available here.
[3] These ratios are estimated as the average ratio across scenarios for each sector for the 2050 forecast. For example, we divided the basic metals GVA 2050 forecast of the accelerated scenario by the GDP 2050 forecast for the accelerated scenario respectively, and then average them to consider the three scenarios.
[4] Beyond the horizon: New Tools and Frameworks for transition risk assessments from UNEP FI’s TCFD Banking Programme, by D. Carling and R. Fischer, September 2020, available here.
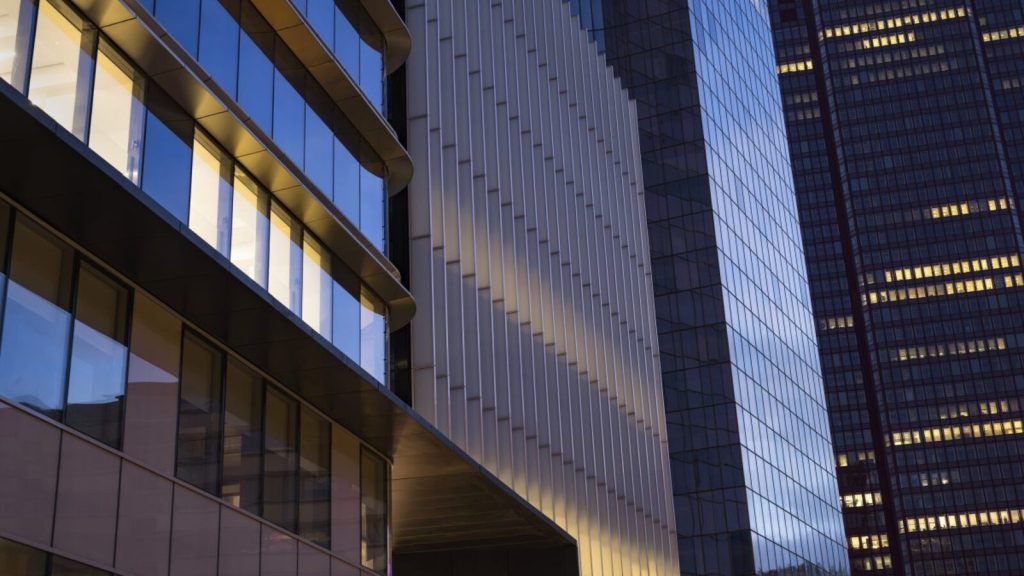
Financial services tax digest – June 2025
As the saying goes, “the only constant in life is change.” As we enter the third quarter of 2025, global economic uncertainty continues to escalate. President Trump’s pause on “reciprocal” tariffs is set to expire on 8 July 2025. Currently, the U.S. has only reached trade deals with the UK and China. However, negotiations with […]
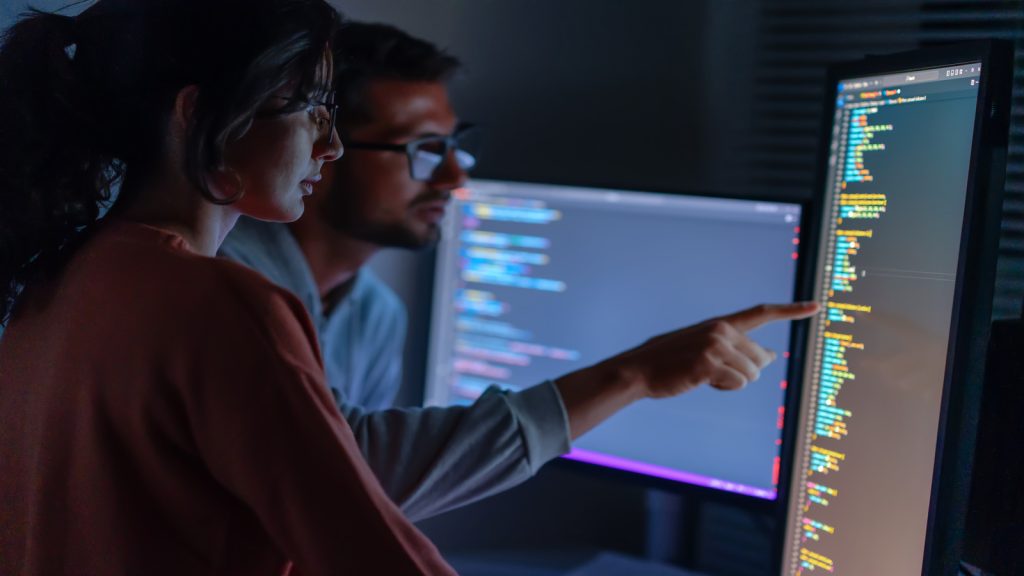
Artificial intelligence in the EU financial sector – balancing regulation and innovation
To harness the potential benefits of AI safely, financial institutions must ensure that they have appropriate guardrails in place to allow safe innovation. The EU’s AI Act will help shape how institutions govern artificial Intelligence across functions. Boards must focus on ensuring that AI risks are identified and addressed across the development, procurement and use […]
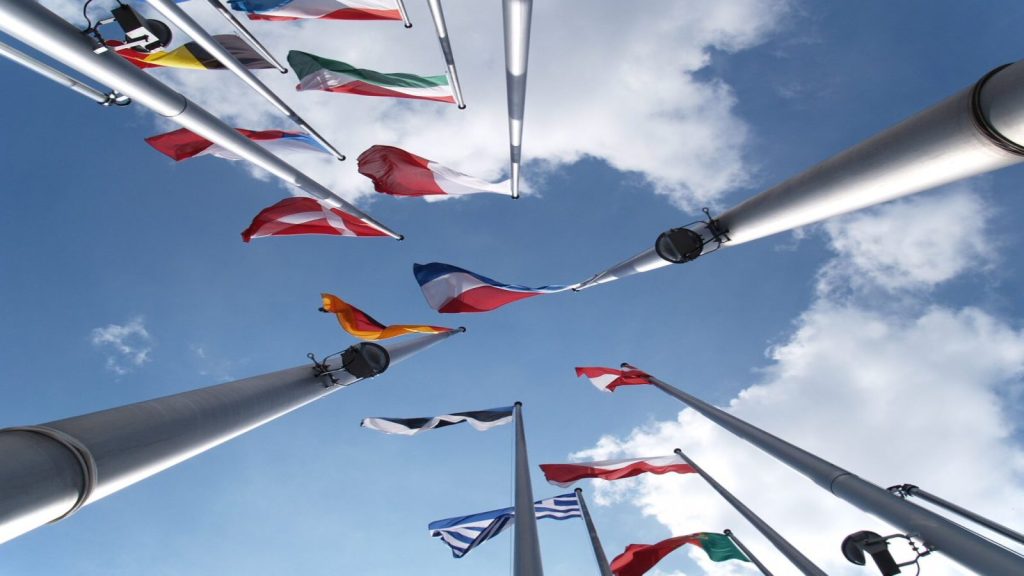
Sanctions compliance in Europe: navigating complexity with confidence
Sanctions compliance has rapidly evolved from a niche area of regulatory focus into a critical and high-risk concern for financial services firms. As geopolitical tensions rise and sanctions regimes become more expansive and ever changeable, firms operating across borders must navigate an increasingly fragmented and high-stakes landscape. In advance of the launch of Forvis Mazars’ […]
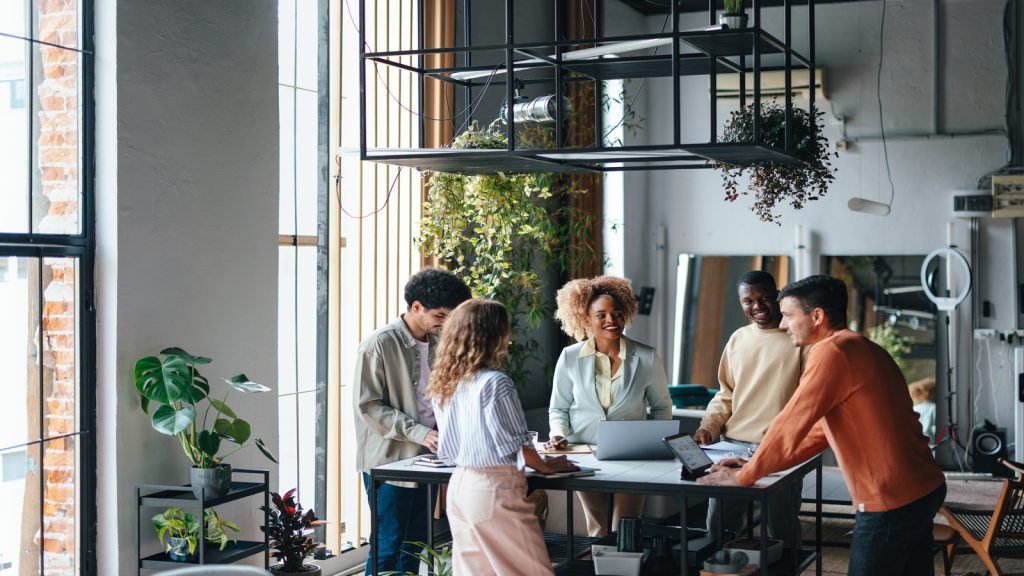
Managing ESG and climate risks: rising supervisory expectations for financial institutions in the UK and EU
As ESG and climate risks climb the supervisory agenda, financial institutions face rising expectations from both UK and EU regulators. This article compares the evolving approaches of the PRA and EBA—and what firms must do now to stay ahead. In the past six months, the Prudential Regulation Authority (PRA) and the European Banking Authority (EBA) […]
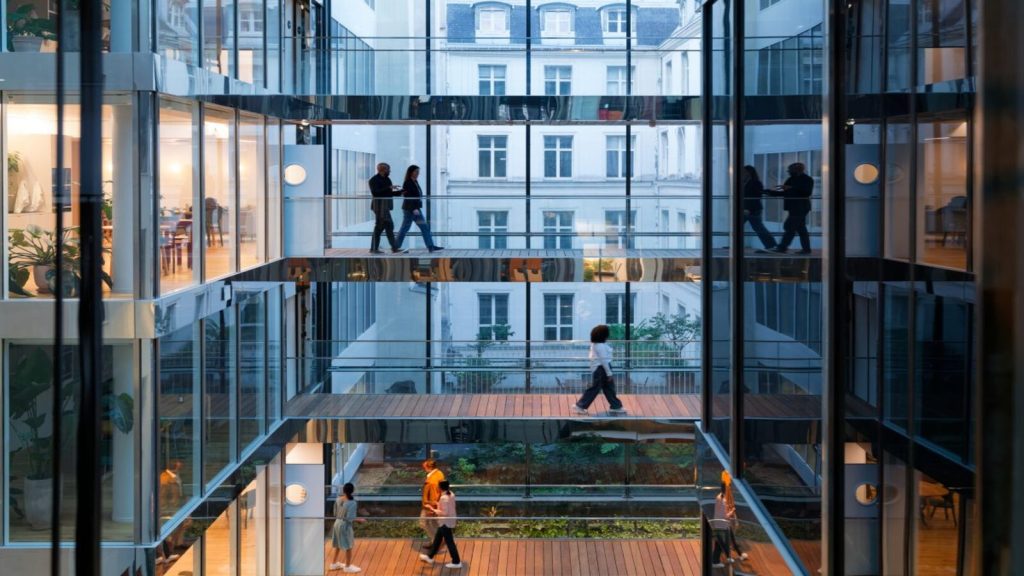
Bridging the capital markets integration gap: an operational framework for a supervisory efficiency test
Europe’s capital markets are increasingly integrated — but supervision remains fragmented. Without a more coherent supervisory model, regulatory arbitrage and inefficiencies will grow. A new approach is needed. The EU’s financial market supervision remains fragmented and misaligned with the depth of market integration achieved through initiatives such as the Capital Markets Union (CMU) and the […]
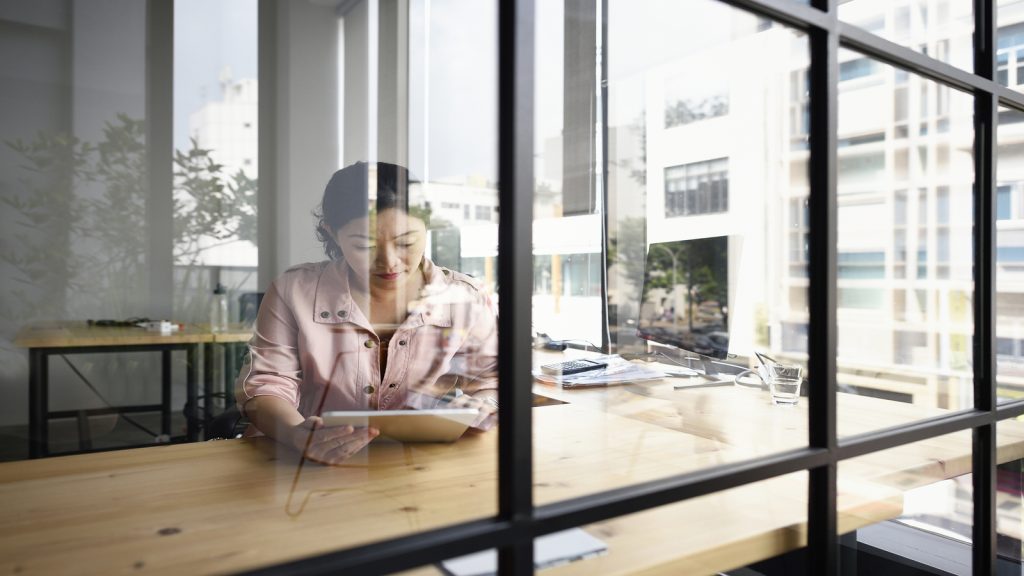
Measuring climate risk exposure with a flexible and readable metric: carbon beta
The reform of the Solvency II Directive, set to be enforced no later than January 30, 2027, introduces new sustainability-related risk requirements. These include (i) the creation of transition plans with quantifiable goals and processes to monitor and address financial risks from sustainability factors in the short, medium, and long term and (ii) an assessment […]
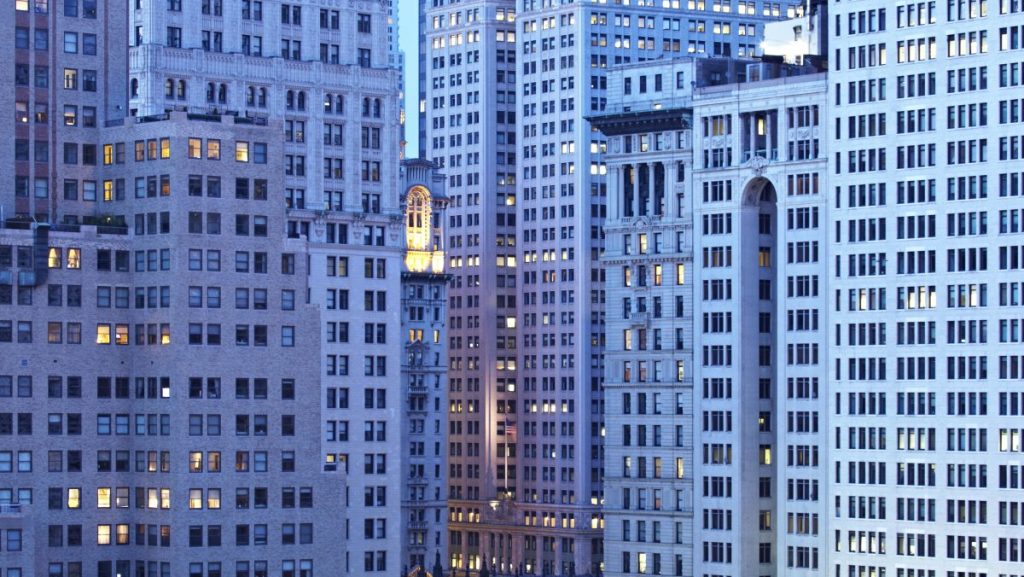
Beyond the baseline: addressing tail risk in Expected Credit Loss models
A follow-up to our previous analysis In our previous article – Navigating the new trade war: implications on Expected Credit losses – we explored the rise in provisioning needs triggered by both direct impacts (sector/geography-specific exposure to tariffs) and indirect impacts (macroeconomic degradation influencing model parameters). Through simulations and stress testing, we showed how seemingly […]
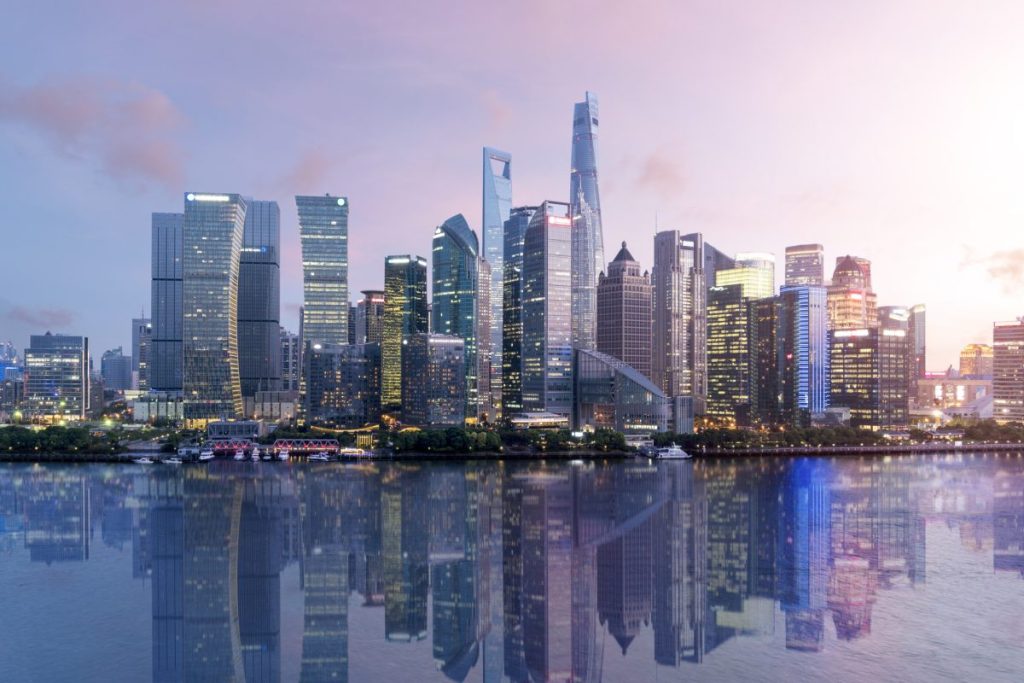
Financial services: navigating the new trade war
Political background Since early 2025, under President Donald Trump’s second term, the United States has escalated tariff actions on a wide array of imports, reigniting global trade tensions. A series of key announcements* has introduced significant volatility into financial markets: *This context is accurate as of 10 April 2025. However, given the extreme pace of […]
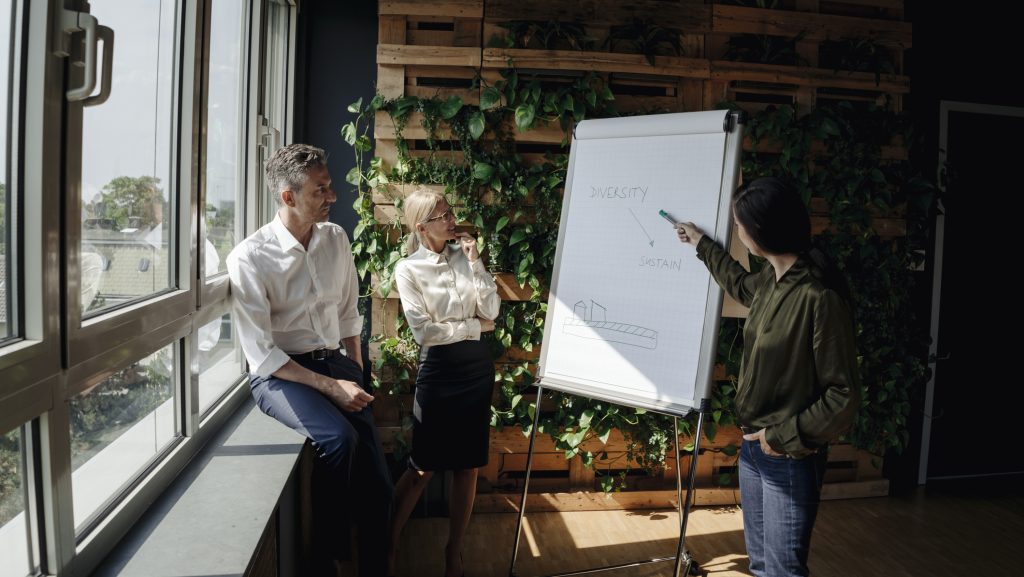
Putting people at the heart of sustainability: interview with Simon Rawson – Executive Director, The Taskforce on Inequality and Social-related Financial Disclosures
In an exclusive interview with Forvis Mazars, Simon Rawson, Executive Director, The Taskforce on Inequality and Social-related Financial Disclosures (TISFD) discusses the work, objectives and approach of the taskforce. Simon explains how the taskforce is about creating a knowledge base, evidence and recommendations on a social-related disclosure framework that could be used for businesses to […]
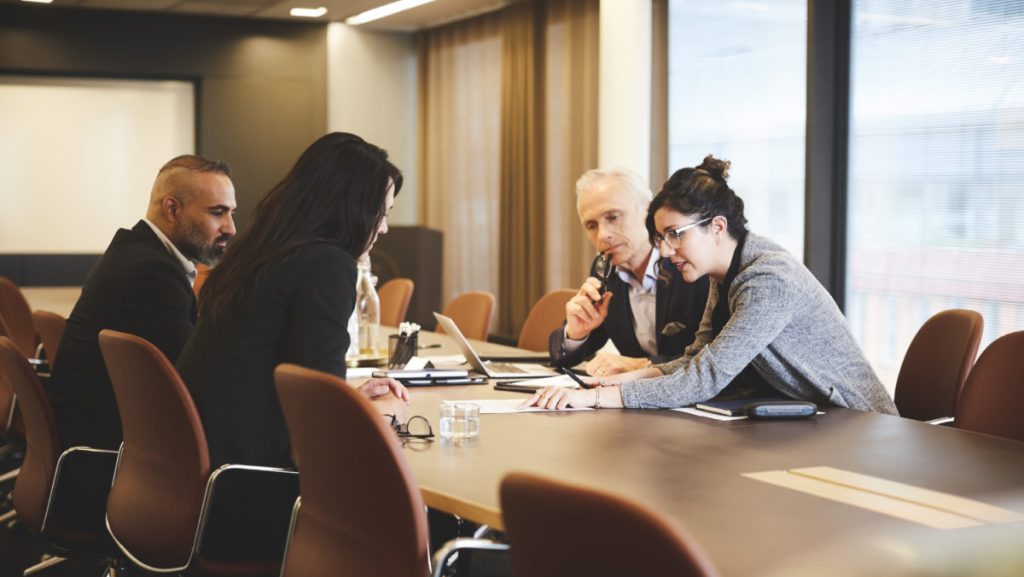
Financial services tax digest- April 2025
As Benjamin Franklin famously said, nothing is certain in this world except death and taxes. As we enter the second quarter of 2025, we find great uncertainty – and tax is at the heart of it. The prospect of an international tariff war looms. Although financial services businesses are not expected to be the primary […]
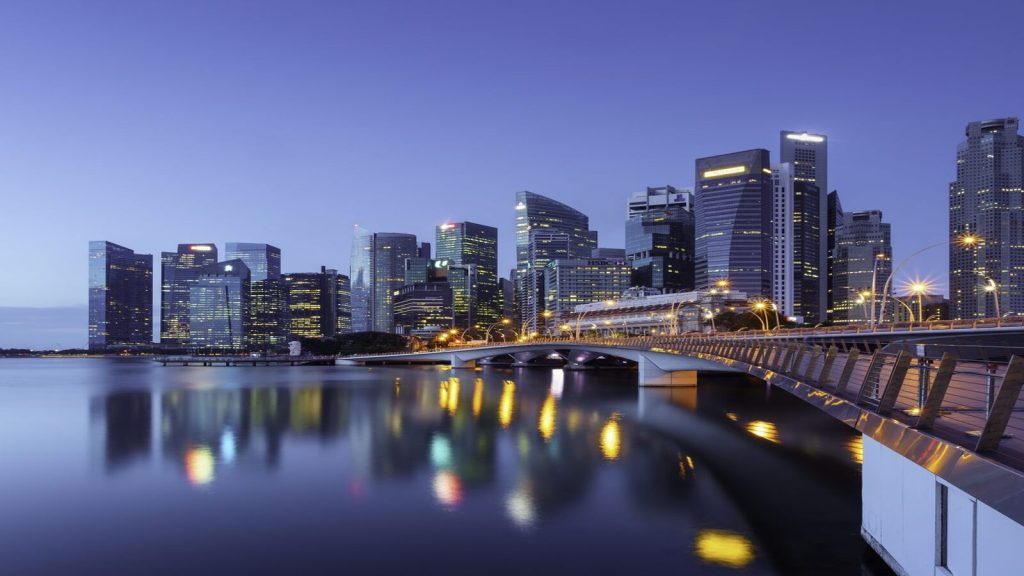
Financial services regulatory landscape in APAC: navigating the complexities and emerging trends
In 2025, the financial services landscape in the Asia-Pacific (APAC) region continues to experience rapid and structural change driven by complex and interconnected risks. These elements are fundamentally reshaping regulatory priorities across the region, prompting diverse responses from national authorities. The region’s vast diversity can lead to regulatory fragmentation, requiring financial institutions to navigate a […]
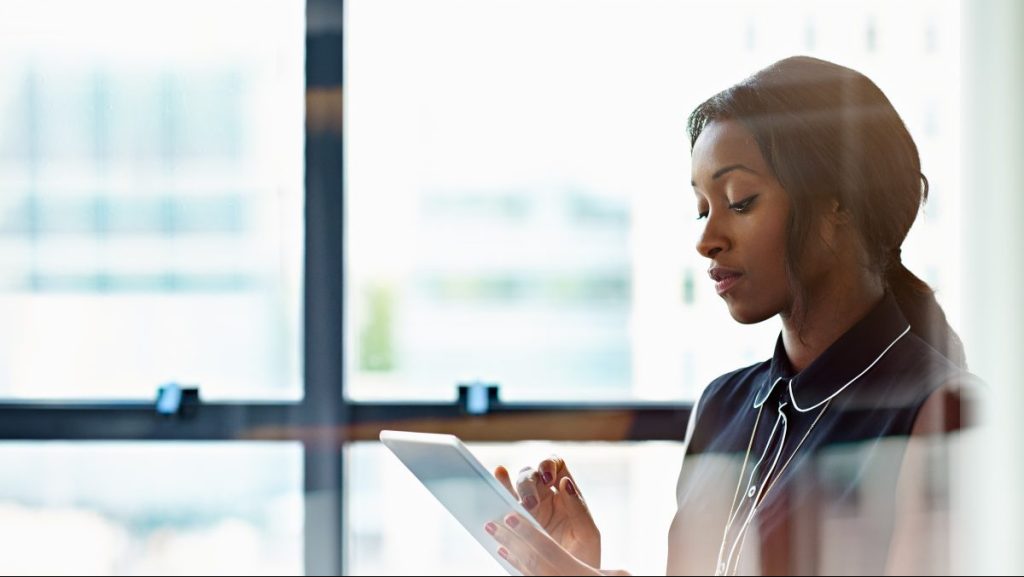
Understanding DORA compliance and regulatory expectations for financial institutions
The DORA (Digital Operational Resilience Act) regulation came into force across the financial sector on 17 January 2025. Unsurprisingly, only a small number of firms affected by DORA have declared themselves completely ready and compliant with all areas of the regulation. The European Supervisory Authorities (ESAs) have affirmed their position on the minimum requirements firms […]
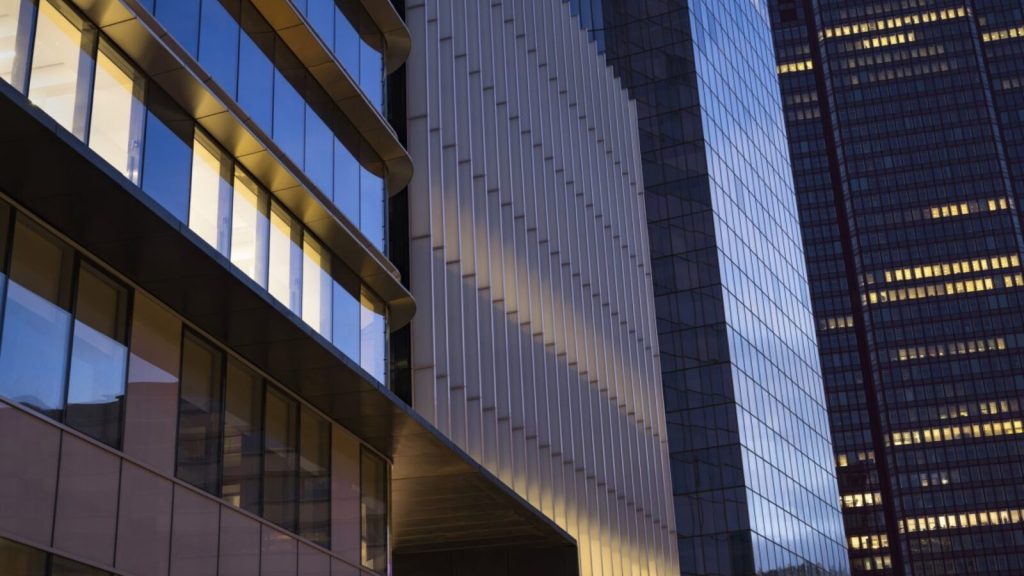
Deregulatory pressures to encourage competitiveness and growth: there is no such thing as a free lunch
This article was written in collaboration between our Forvis Mazars Economics Hub and Forvis Mazars Global Financial Services Regulatory Centre. In the aftermath of the 2008 Global Financial Crisis (GFC), stringent regulations were developed for the banking and insurance sector to prevent the need for future public bailouts during times of financial stress. Nearly two […]
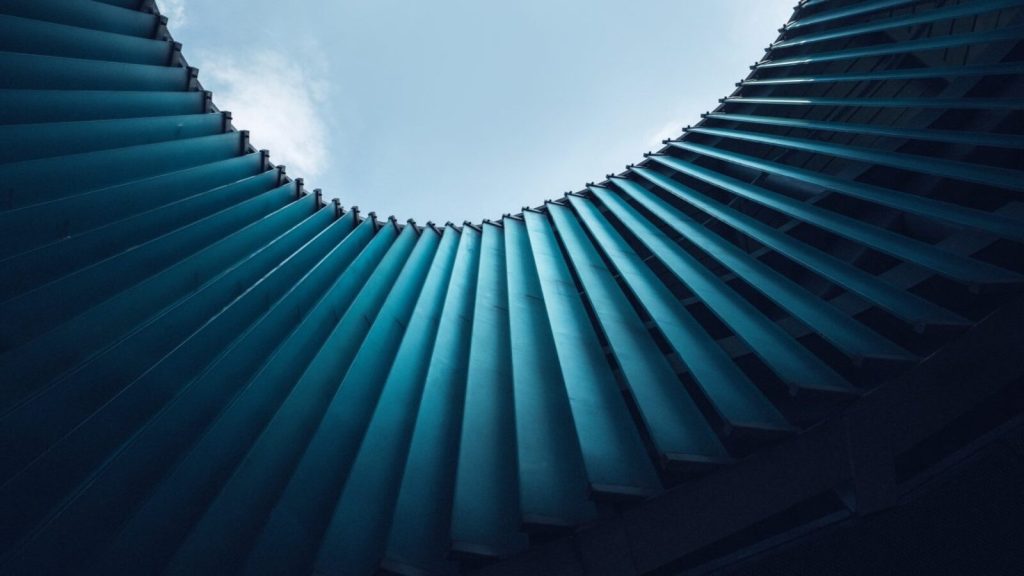
Single Resolution Board (SRB) 2025 work programme
On 2 December 2024, The Single Resolution Board (SRB) published its work programme for 2025. The Programme sets out the SRB’s main priorities for the next year, alongside the continued and important work on standard operations. An overarching priority is to streamline the resolution planning process and resolution plans to make them more efficient and […]
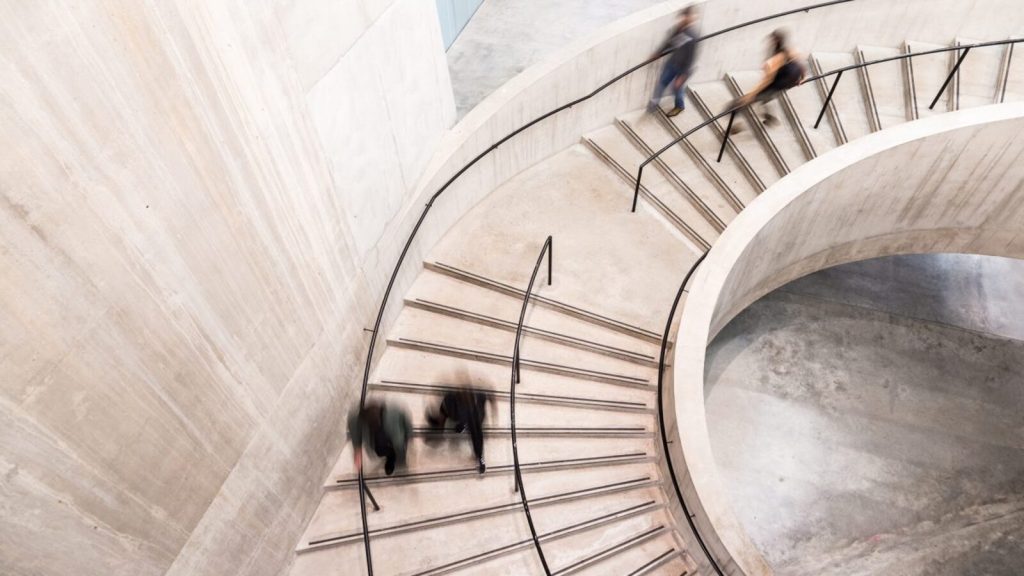
European Securities and Market Authority (ESMA) strategic priorities for 2025
On 1 October 2024, ESMA published its strategic priorities for 2025: ESMA’s annual work programme. The strategic priorities for this year build on the five-year ESMA strategy for 2023 to 2028, focusing on fulfilling ESMA’s mandates in legislation and expanding upon activities from 2023 and 2024. ESMA’s 2025 strategic priorities are outlined are three strategic […]
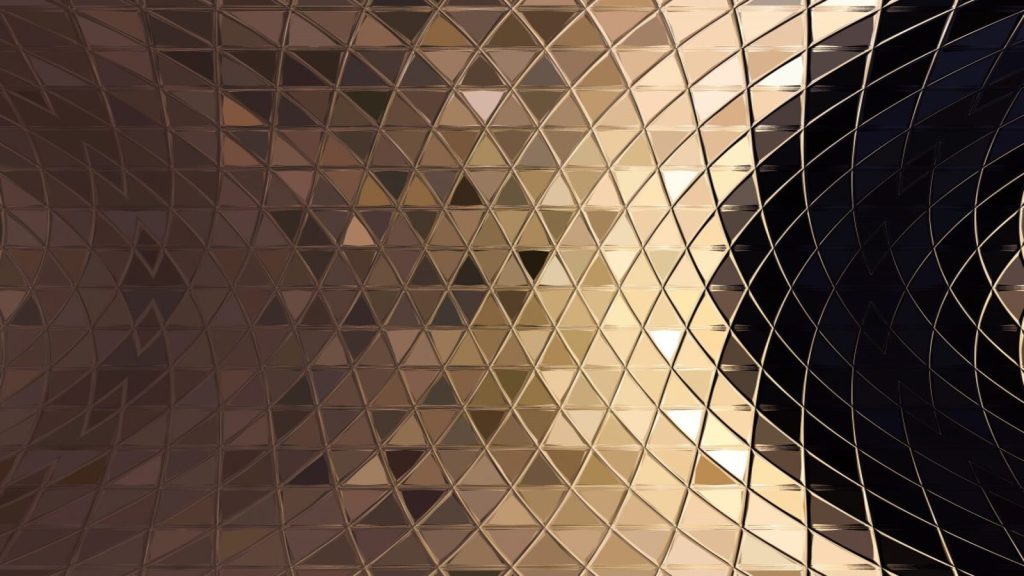
European Central Bank (ECB) supervisory priorities for 2025-2027
On 18 December 2024, The European Central Bank (ECB) published its supervisory priorities for 2025-2027, which reflect the Bank’s medium-term strategy. Forvis Mazars interviewed Patrick Montagner, member of the ECB Supervisory Board, to discuss the ECB’s supervisory priorities for 2025-2027 and the 2025 stress test. The conversation highlights how the ECB is adapting its supervisory […]
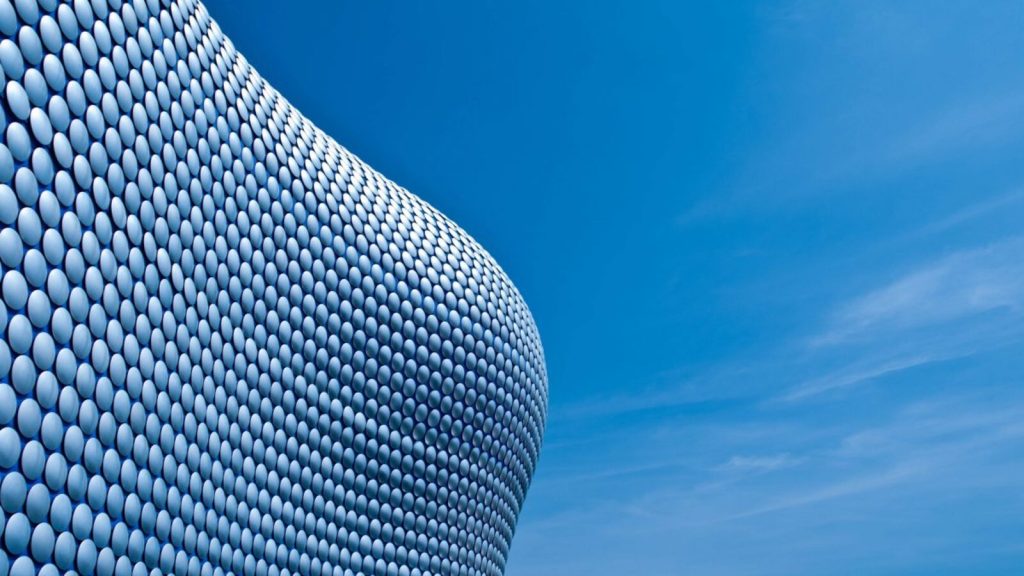
European Banking Authority (EBA) 2025 work programme
On 2 October 2024, the European Banking Authority (EBA) outlined its work programme for 2025, focusing on the continued implementation of the EU banking package (Capital Requirements Regulation (CRR) III / Capital Requirements Directive VI) and the enhancement of the Single Rulebook, which was also a priority in 2024. Additionally, the EBA will take on […]
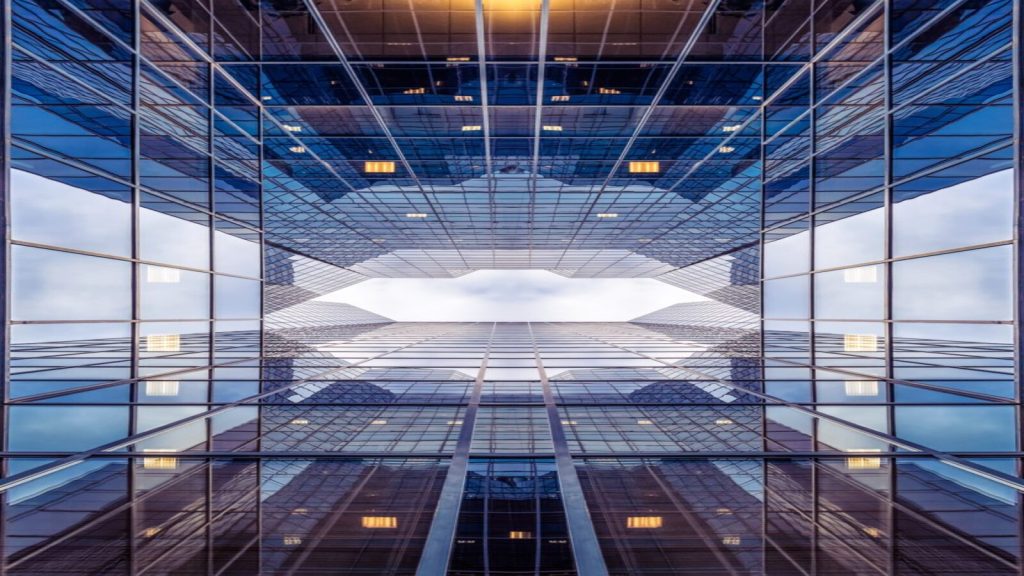
Basel Committee work programme and strategic priorities for 2025/2026
The Basel Committee work programme for 2025/2026 outlines the strategic priorities for its policy, supervisory and implementation activities for the two-year period. The key themes of the committee’s 2025-2026 work programme include: The work programme builds on its mandate to strengthen the regulation, supervision and practices of banks worldwide with the purpose of enhancing global financial […]
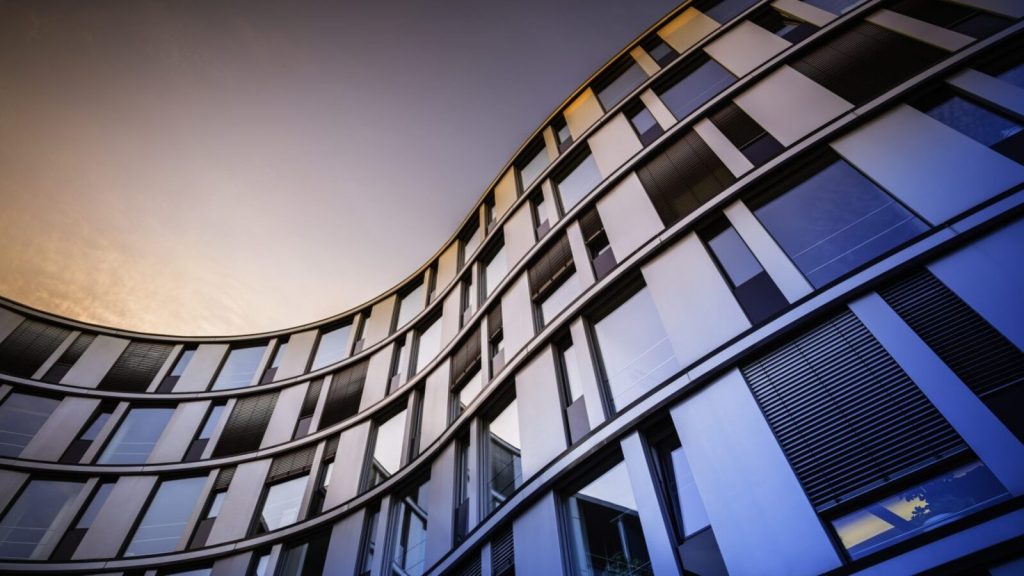
European Insurance and Occupational Pensions Authority (EIOPA) 2025 work programme
The European Insurance and Occupational Pensions Authority (EIOPA) published its 2025 work programme which is embedded in its revised single programme document 2025-2027. The year 2025 marks the start of this three-year plan. EIOPA’s mission is to protect the public interest by contributing to stability in the short, medium and long term. This mission is […]
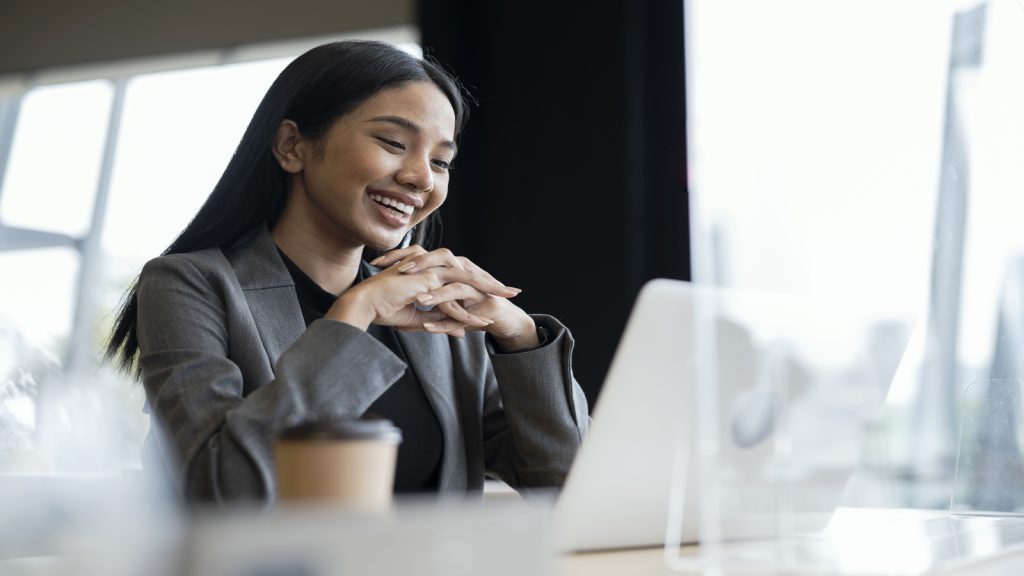
EU Cloud: can Europe manage to create an attractive alternative?
Sovereignty raises debate and there is a wide divergence in the way to deal with that topic. The current debate in Europe on the EU Cloud Services (EUCS) High+ criteria is an illustration of the diverse positions existing in that field. Addressing sovereignty as an isolated concept does not make sense. Sovereignty should be addressed […]
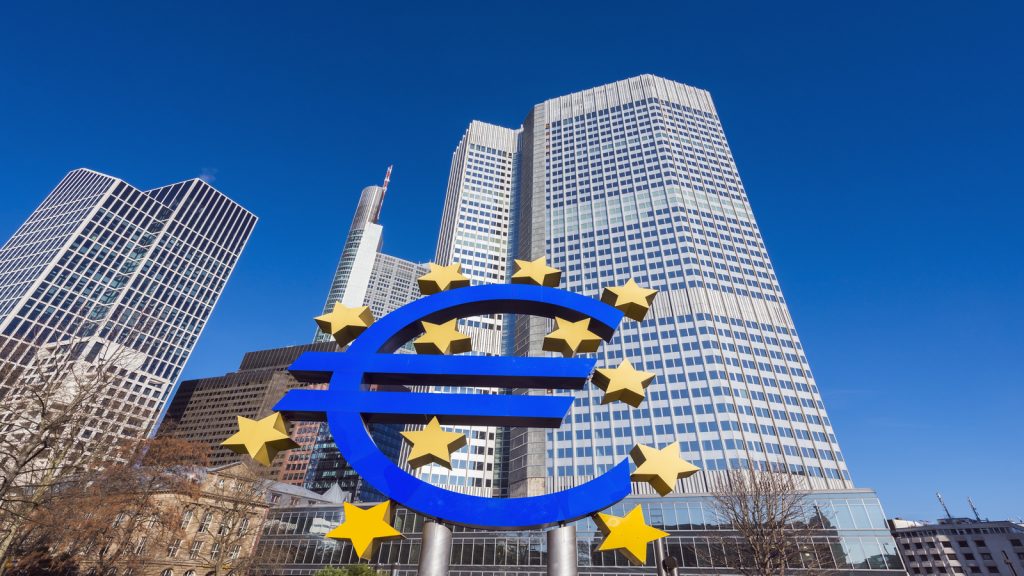
ECB interview: 2025-2027 ECB supervisory priorities and 2025 stress test
In this exclusive interview by Forvis Mazars, Patrick Montagner, member of the ECB Supervisory Board, discusses the ECB’s supervisory priorities for 2025-2027 and the 2025 stress test. The conversation highlights how the ECB is adapting its supervisory focus and practices to address the evolving risks faced by the banks it supervises, including geopolitical shocks, climate […]
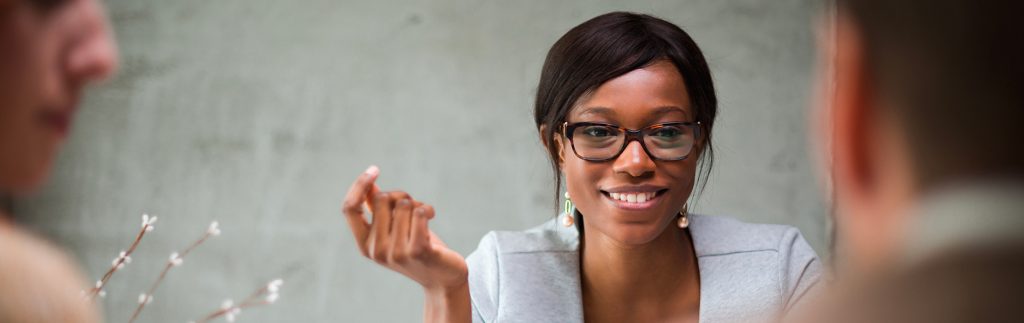
Financial services tax digest- January 2025
As we say goodbye to 2024 and look forward positively to 2025, at Forvis Mazars we know that financial institutions and funds will have to deliver in an evolving complex domestic and/or cross-border tax environment. Banks, insurers and asset managers all over the world may have to capture M&A opportunities and/or deal with some efficient […]
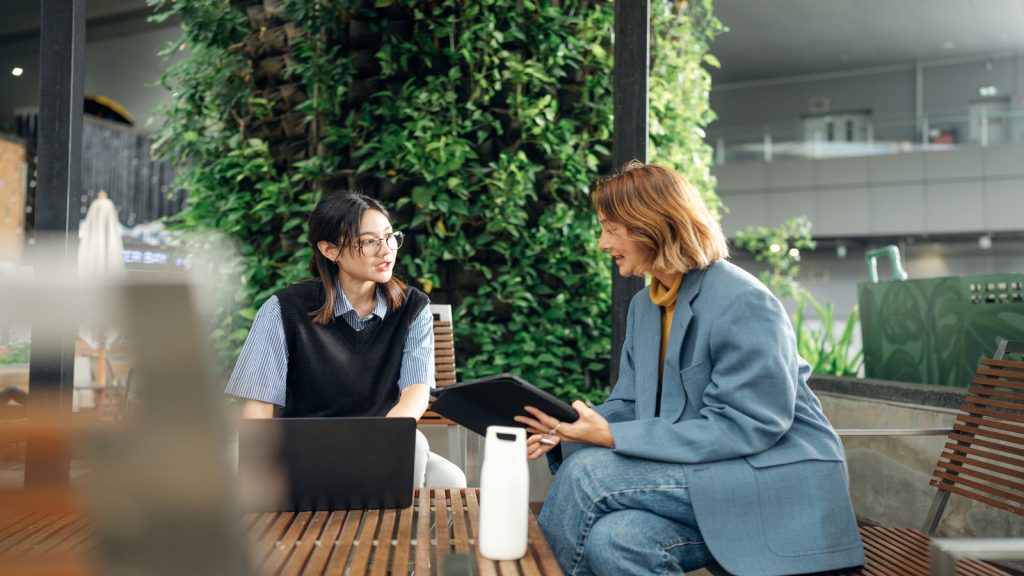
Latest NGFS climate risk scenarios: Implications for financial institutions
In November 2024, the Network for Greening the Financial System (NGFS) released the latest version (termed Phase V) of its climate risk scenarios. These introduce significant advancements that enhance financial institutions’ accuracy when undertaking climate-related financial risk assessments. Key advancements include the incorporation of a new damage function for physical risk assessment, the integration of […]
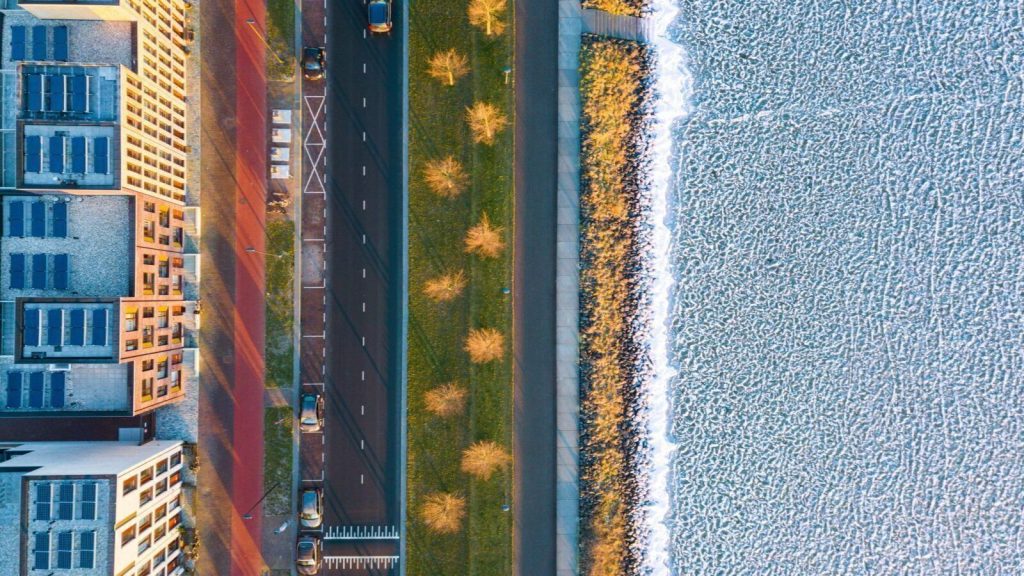
Assessing climate risk for the insurance industry: How reliable are climate scenarios?
As the impacts of climate risks become more pronounced, regulators and the insurance industry are under increasing pressure to refine risk assessment frameworks. This article examines regulatory developments, a critical review of climate scenarios and limitations of models within the insurance sector, the implications of new regulatory developments, and the role of actuaries in establishing […]
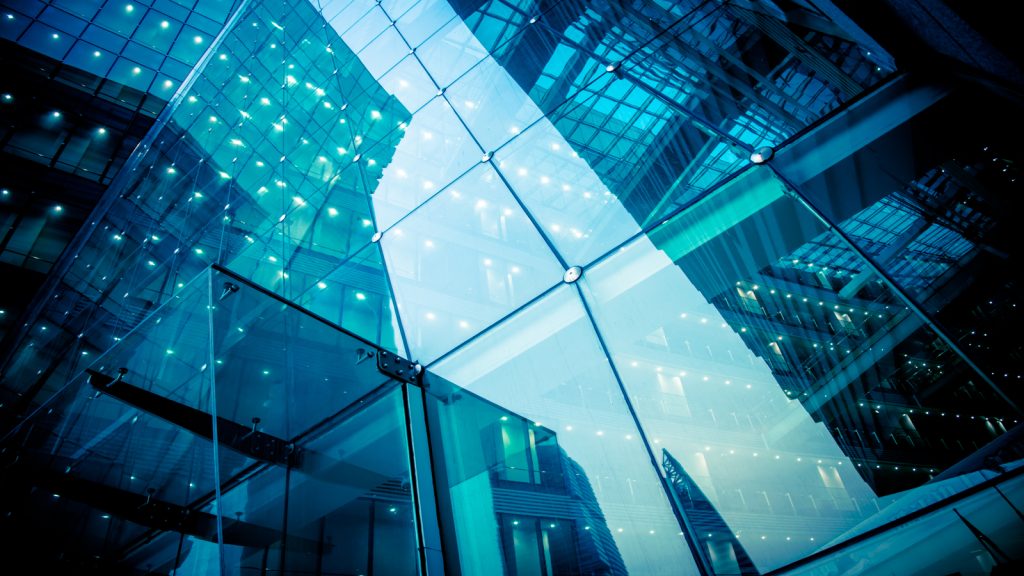
The rising importance of risk culture in banking supervision
In the wake of financial crises, the question ‘Where was the board?’ has become a rallying cry, reflecting the demand for greater accountability within banking institutions. Recent high-profile bank collapses underscore the necessity of robust internal governance frameworks that include a strong risk culture at their core. Supervisors worldwide continue to elevate risk culture as […]
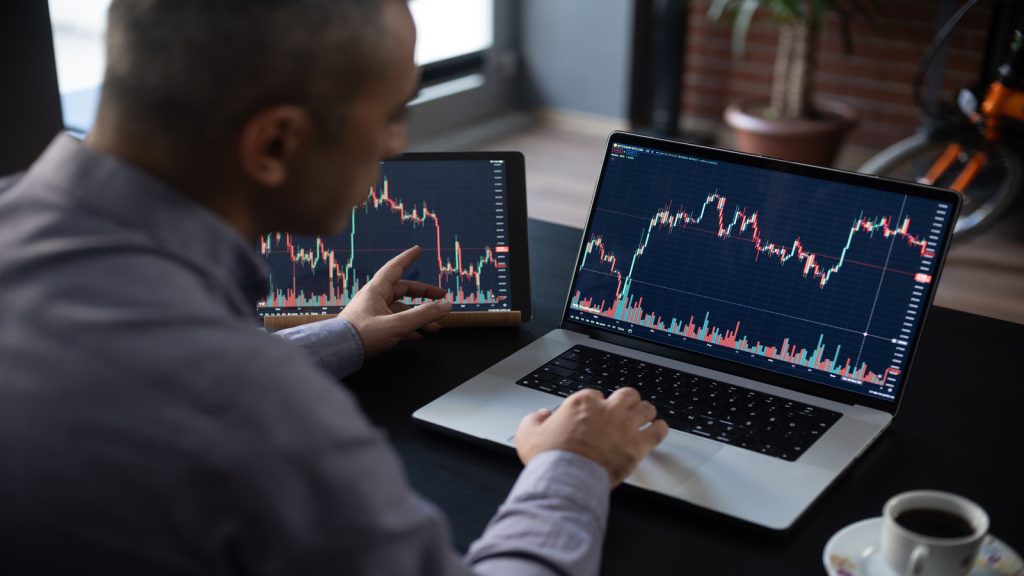
Top risks facing financial services firms in 2025: key highlights
As part of our annual series on “Top risks facing financial service (FS) firms”, we have identified and ranked the key risks for financial services business leaders in 2025. We discuss in the article below the top five areas that FS firms should prioritise in 2025. Our more detailed assessment can be found here, which contains […]
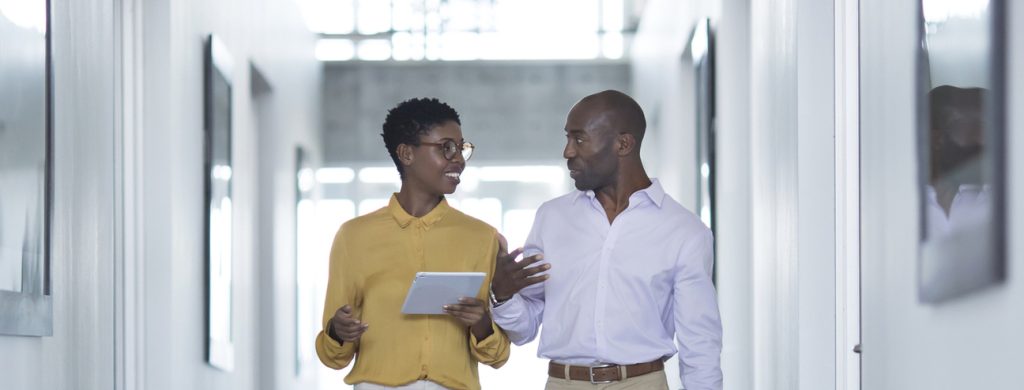
Reflections on the Africa Financial Summit: adapting to global shifts in risks and regulations
Forvis Mazars proudly participated in the Africa Financial Summit (AFIS) 2024, held from 9-10 December in Casablanca, Morocco, and sponsored the awards ceremony. Below are some reflections on the state of play and future of Africa’s financial sector. In recent years, the world has undergone dramatic changes, challenging the status quo in unprecedented ways. This […]
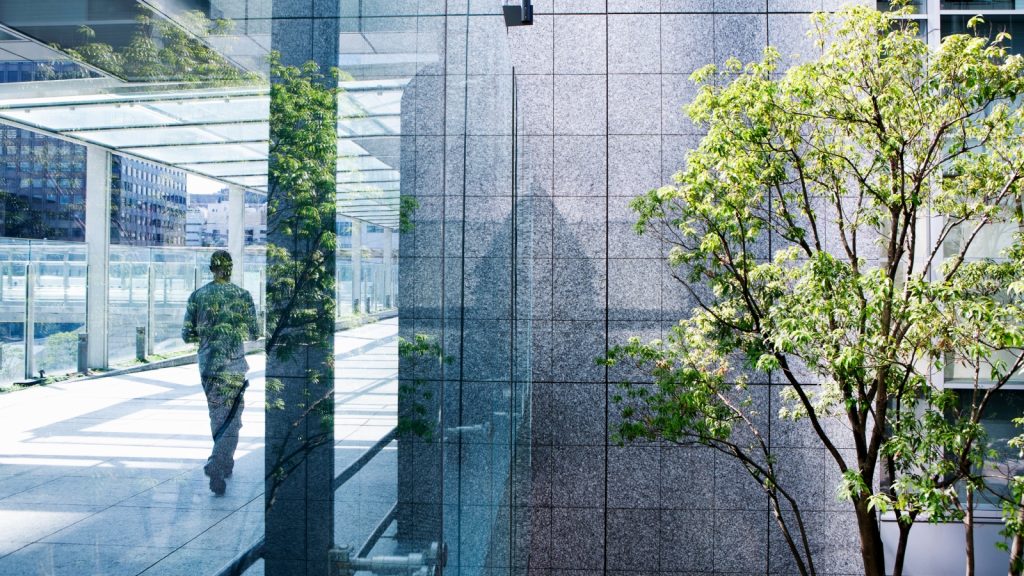
NGFS phase V climate risk scenarios: good progress however unaddressed limitations remain
In November 2024, the NGFS[1] (Network for Greening the Financial System) published phase V of its widely used climate scenarios updated with the most recent economic and climate data, policy commitments, and model versions. This phase V also introduces a new damage function for physical risk assessment. The new damage function incorporates various climate variables […]
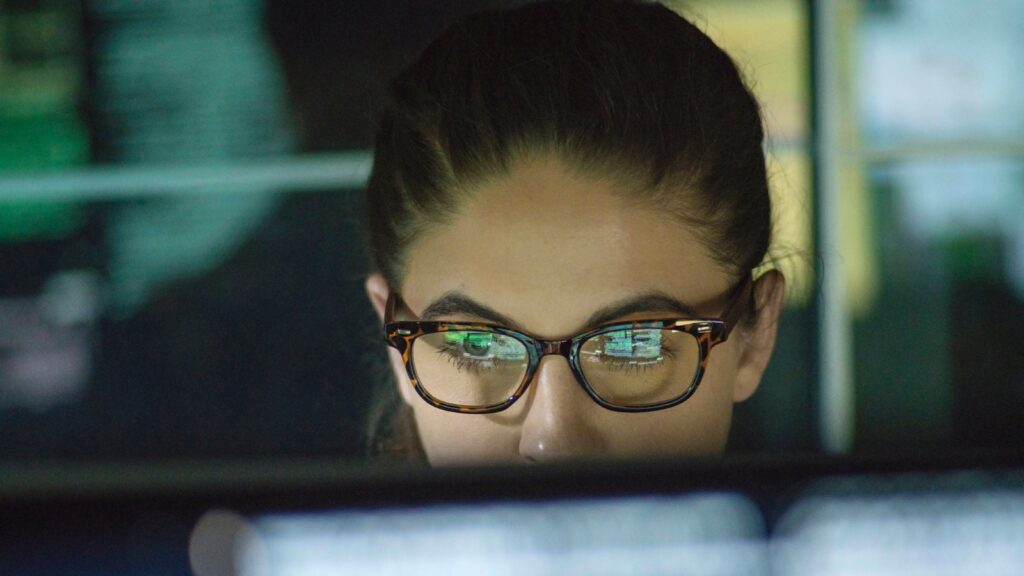
Industry Cloud Platforms: Unlocking innovation through tailored cloud solutions
Cloud computing has been with us for over 15 years, but we have barely scratched the surface of its potential.[1] Industry Cloud Platforms (ICPs) are cloud-based solutions targeting the specific needs of industry verticals that generic and traditional solutions do not adequately address. These solutions encompass cloud services, applications and data models tailored to meet […]
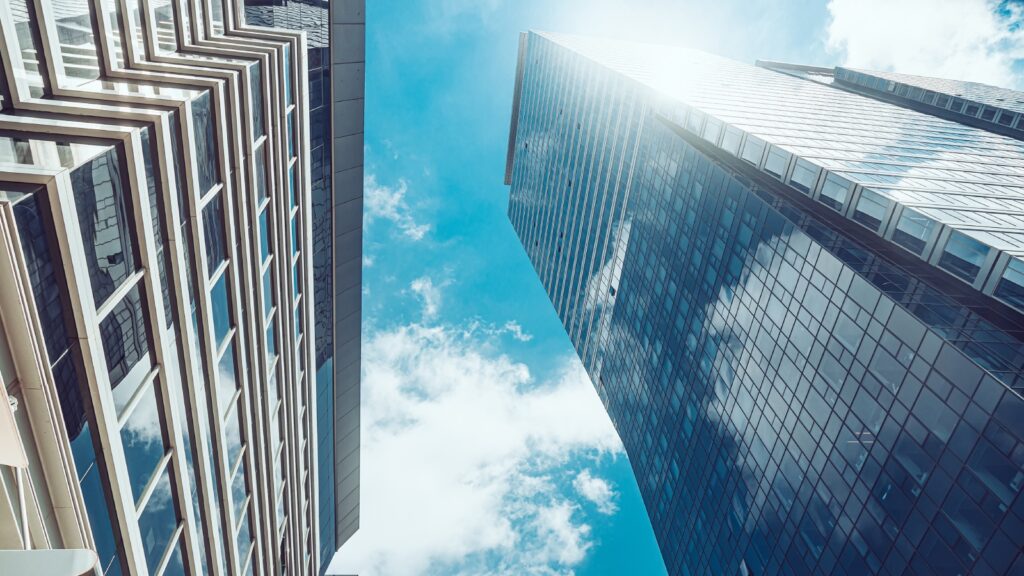
Financial reporting of European banks: towards the end of the “golden age” of post-model adjustments (PMAs) / overlays to banks’ expected credit losses (ECL)?
When the Covid-19 pandemic broke out in 2020, the banks had to make post-model adjustments[1] (or management overlays) to incorporate the impact of this unprecedented situation into the expected credit losses recognised by the banks. While the end of the pandemic should have put an end to these exceptional adjustments, the events that followed (war […]
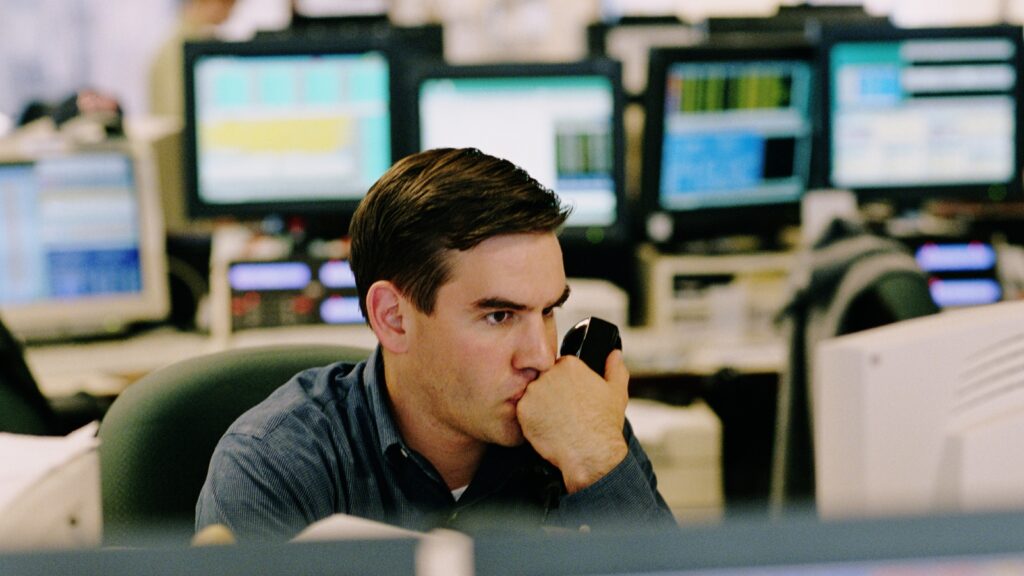
Financial reporting of European banks: an overview of expected credit losses indicators against a backdrop of continuing uncertainty in YE 2023
After several years of significant macroeconomic and geopolitical events such as the Covid-19 crisis, the war in Ukraine, the return of inflation and rising interest rates, the year 2023 seemed to mark a form of stabilisation in the international environment in the absence of any notable new event, despite the continuing uncertainties. How has the […]
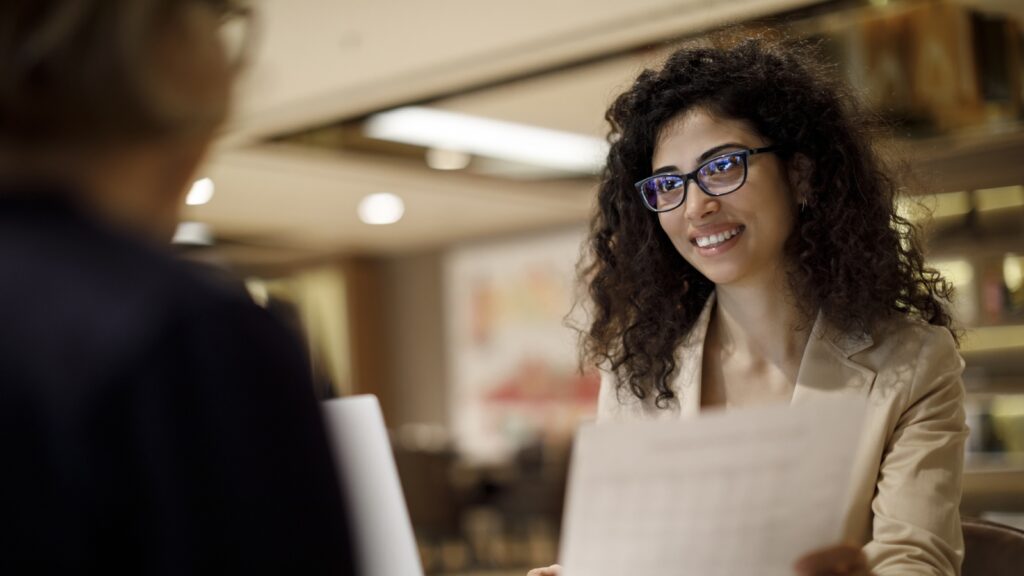
Understanding the impact and future of the DORA regulation
The European Supervisory Authorities (ESAs) published the final texts of the DORA (Digital Operational Resilience Act) regulation in July. These definitive texts will come into effect in January 2025, enhancing digital operational resilience and IT risk management for all financial entities. Key takeaways from the final DORA regulation texts In July, the European Supervisory Authorities […]
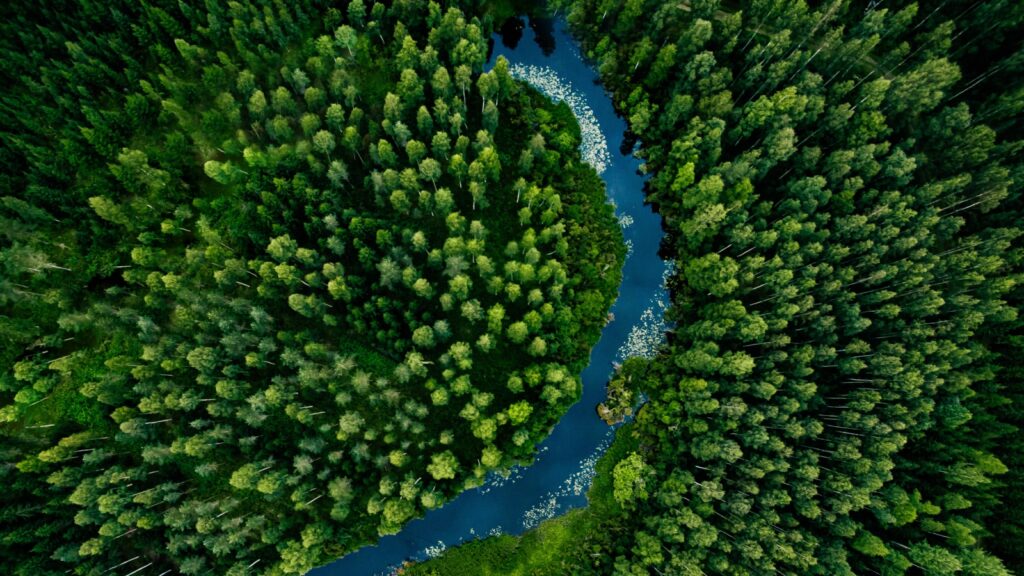
What to know: Global FS sustainability disclosure requirements
A day doesn’t seem to go by without there being another sustainability regulation announcement. This article is a whistle-stop tour around selected jurisdictions and provides an update on what has happened in the past few months, what is to come, and what these developments mean for firms. In summary, reporting on sustainability issues is growing […]
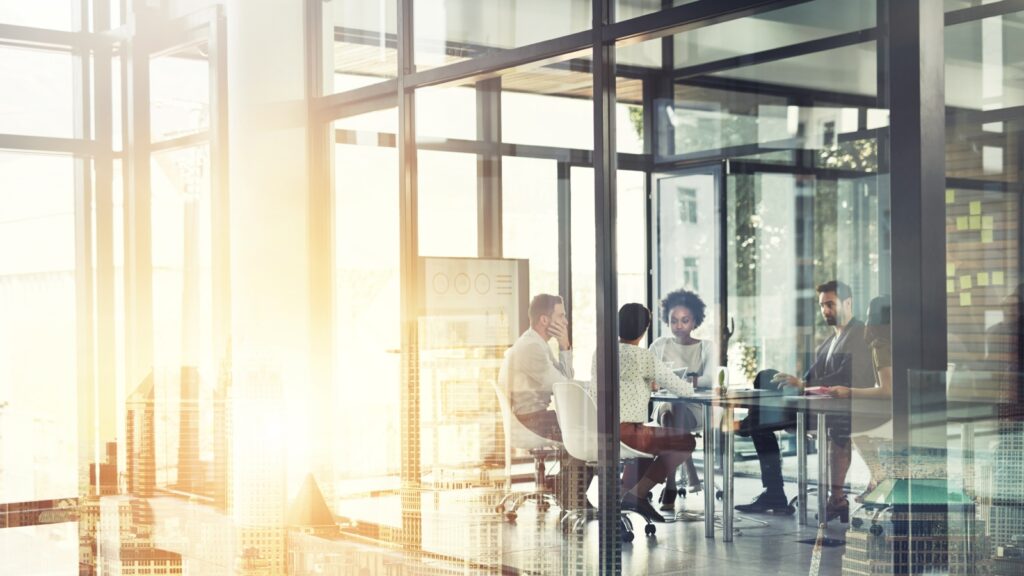
New international principles to strengthen third-party risk management by banks
In the ongoing digitalisation of the banking sector and the rapid growth of financial technology, banks increasingly rely on third-party service providers (TPSPs), including for some of their critical functions. This dependency introduces significant risks, as banks do not always have direct control over these external entities. These risks are further exacerbated by cyber threats, […]
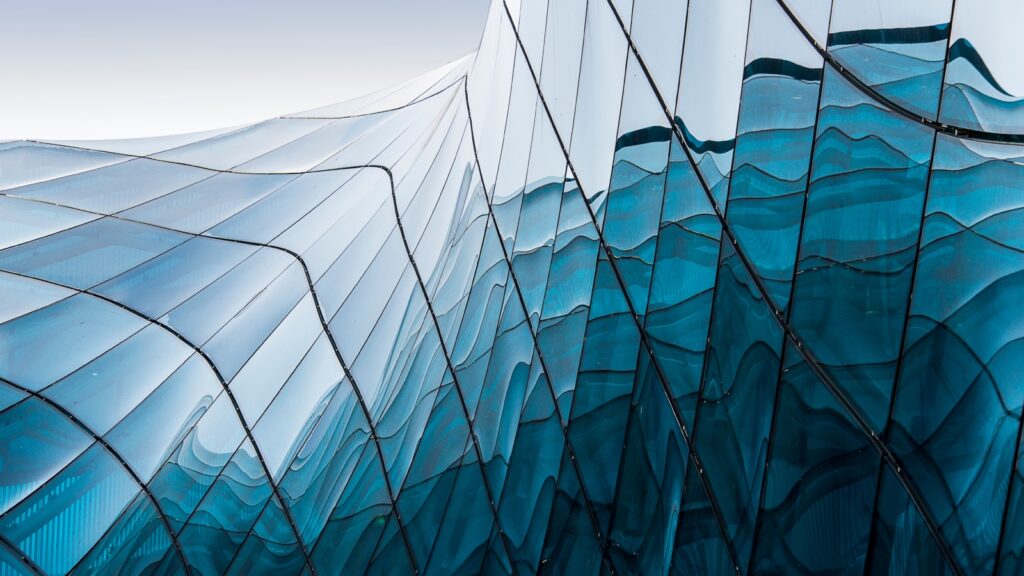
How can AI driven solutions aid AMLA in curbing money laundering?
Traditional methods of tackling financial crime are no longer effective in a world where bad actors use increasingly sophisticated techniques to exploit weaknesses in financial crime controls. With the establishment of a new European regulator, embedding the latest AI-enabled solutions to counter financial crime is of utmost importance. The formation of the Anti-Money Laundering and […]
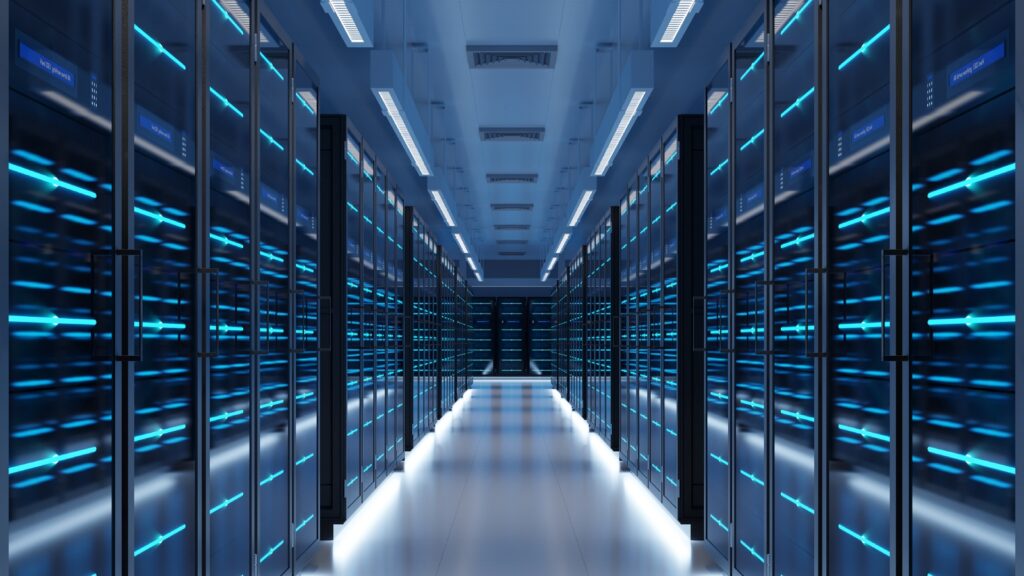
Adapting regulations to the fast-paced world of crypto assets
In today’s dynamic financial landscape, cryptocurrencies and their underlying technology have taken centre stage, attracting both enthusiasts and sceptics. The volatility and potential of digital assets prompt questions about their evolving positions in the traditional financial market. While the promise of decentralisation sparks innovation, ongoing debates on regulation, security, and stability keep the narrative of […]
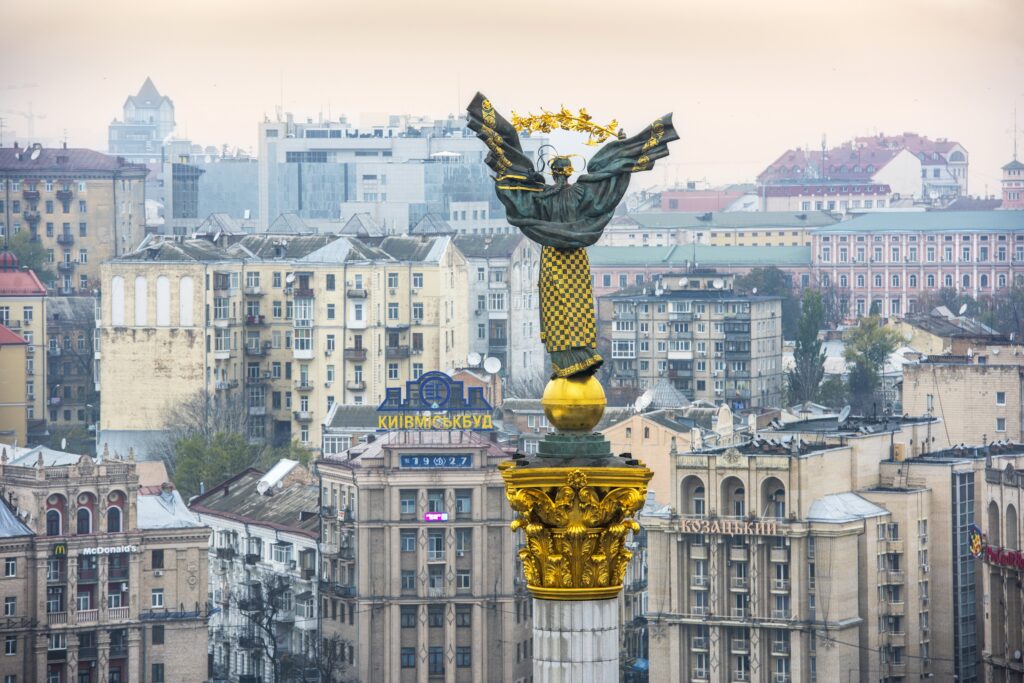
Ukraine’s reconstruction: challenges and opportunities
On 24 February 2024, Ukraine and the rest of the world marked the second anniversary of Russia’s invasion. Despite extensive media coverage of the war and its geopolitical and socio-economic ramifications on Europe and the rest of the world, there is an opportunity for investors to explore the potential in Ukraine. This article aims […]
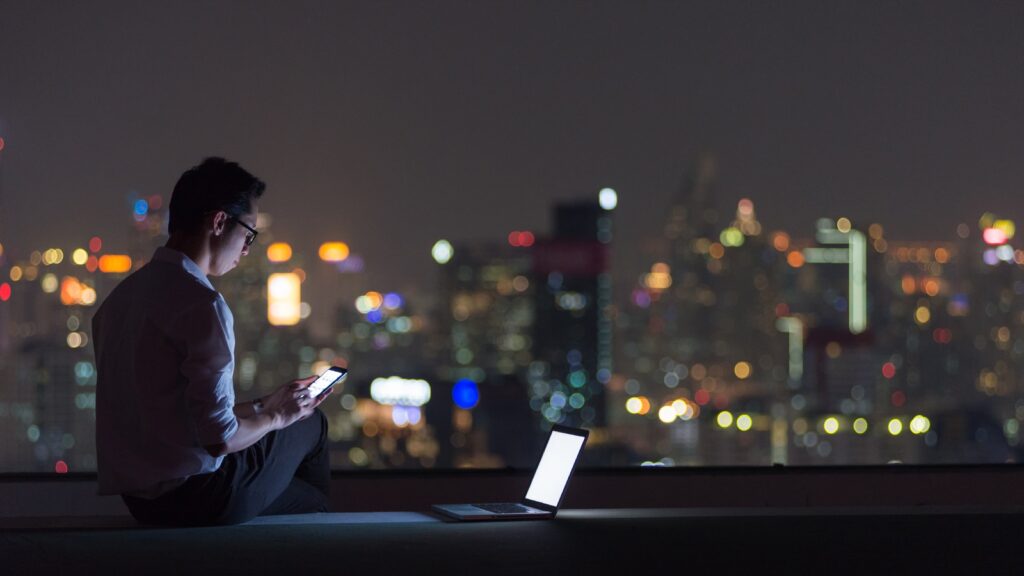
Identifying and managing the ethical risks of AI in financial services
The role of artificial intelligence (AI) in financial services continues to exercise C-suite minds. Not least, how to balance AI’s benefits to enhance the customer experience and improve back-office operations with the ethical challenges that AI presents. Sparking the debate is how financial organisations can employ AI tools such as machine learning (ML), large language […]
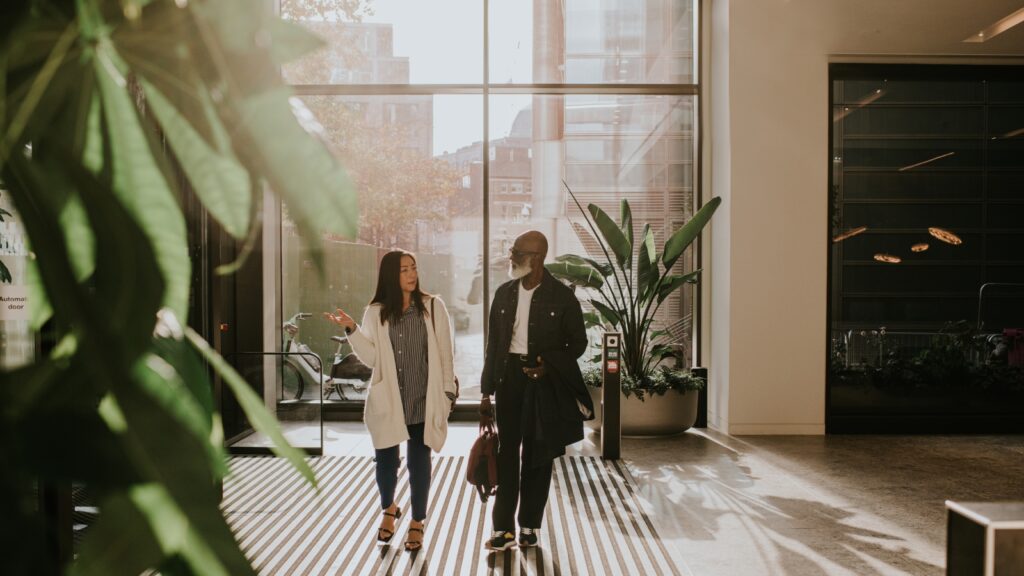
Using emerging technology to support ESG and sustainability reporting
The financial services sector is increasingly looking to technology to help tackle the rising levels of regulation they face. According to the latest Forvis Mazars C-Suite Barometer, the prominence of new technology as a global trend is on the rise in financial services, making it one of the most important issues now topping the C-Suite […]
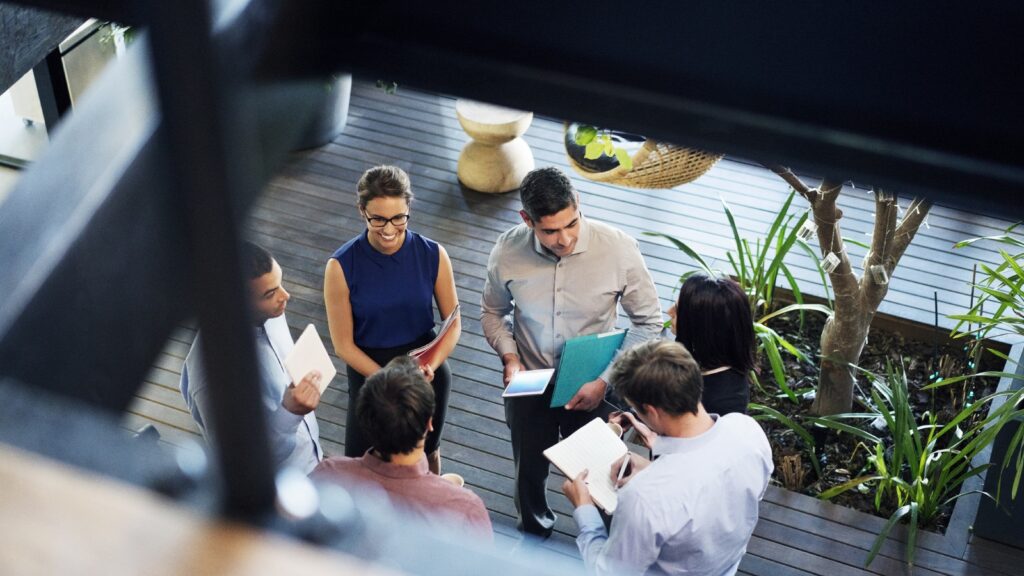
Developing a technology talent strategy
As financial services organisations increasingly focus on digital transformation, having the right expertise and skillsets is critical. However, it’s not simply a question of human capital. It requires developing a talent strategy that recognises the profound impact technology will have across the organisation. Technologies such as cloud, containers, artificial intelligence and machine learning, internet of […]
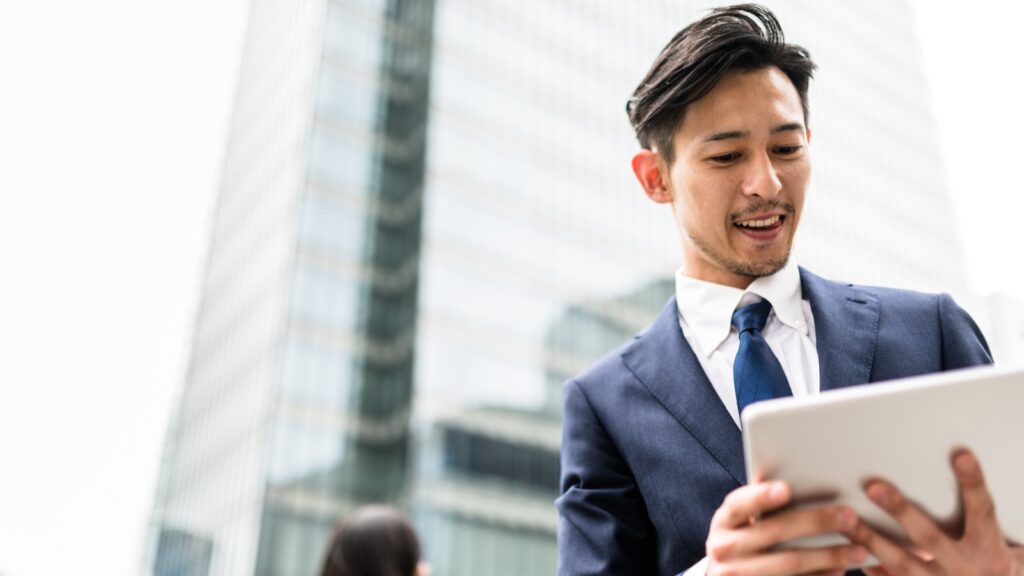
Mapping digital transformation in financial services
Digital transformation now tops the C-Suite agenda of financial services organisations. According to the latest Forvis Mazars C-Suite Barometer, one-third of respondents identified the need to transform company IT as a strategic priority ahead of issues such as sustainability initiatives. While the competitive landscape has been evolving for several years following the arrival of digital-first challenger […]
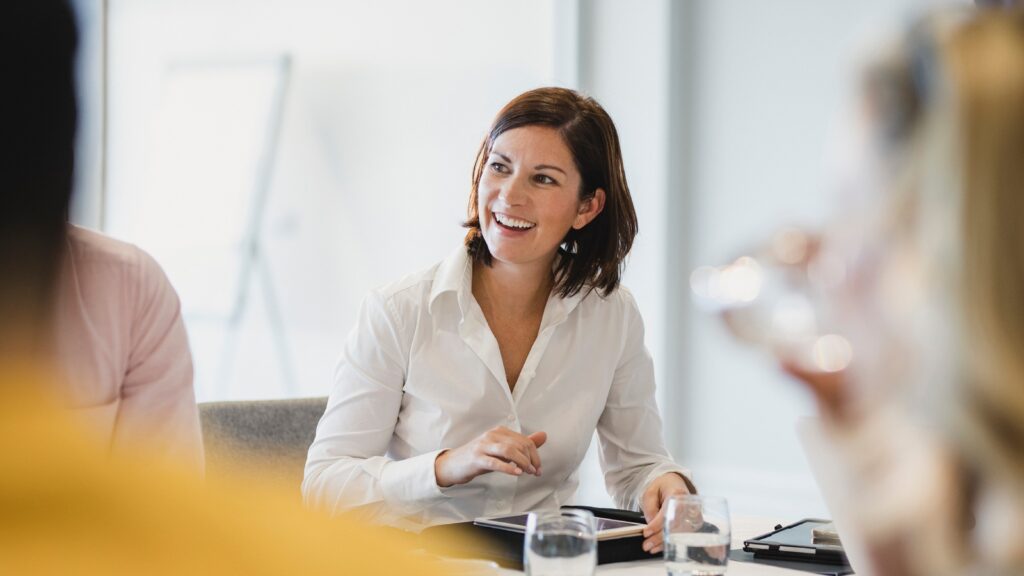
Managing confidence and optimism in financial services
Optimism in the financial services sector is riding high. According to the latest Forvis-Mazars C-Suite Barometer, 94% of financial services respondents say they’re growing, which is five points higher than the global average and up overall from 92% last year. At the same time, 97% of leaders in financial services predict growth in 2024, up […]
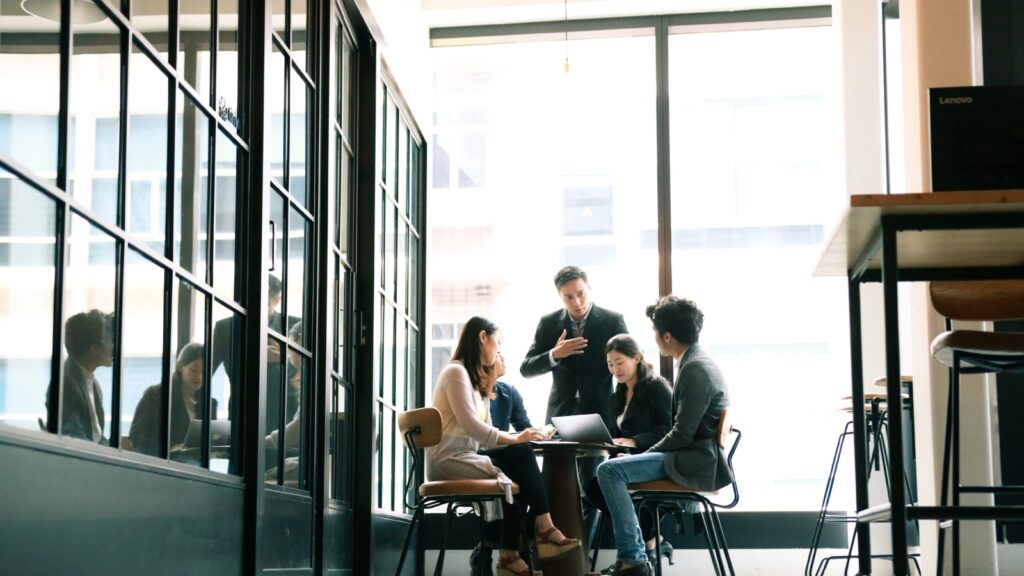
Challenges in governance – managing the ever increasing complexity
In the aftermath of the Global Financial Crisis, regulators and legislators around the world tried to make sense of its causes and then implement rules to stop it happening again. In the UK, the resulting regulation introduced by the Financial Conduct Authority, FCA, was the Senior Managers Certification Regime, which in its current state, carries […]
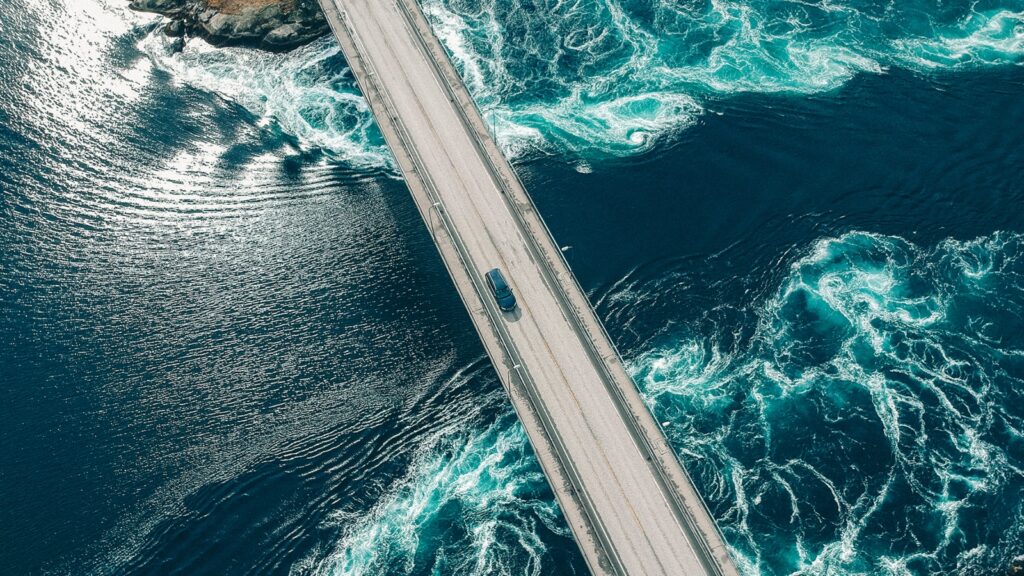
Navigating regulatory waters: a comprehensive look at recent developments in the financial sector
In the dynamic world of finance, regulatory updates play a pivotal role in shaping industry practices and safeguarding the interests of stakeholders. From strengthening anti-money laundering measures to fostering transparency in crypto-asset markets, recent months have witnessed a flurry of regulatory activity across various domains. In this comprehensive overview, we delve into the intricate details […]
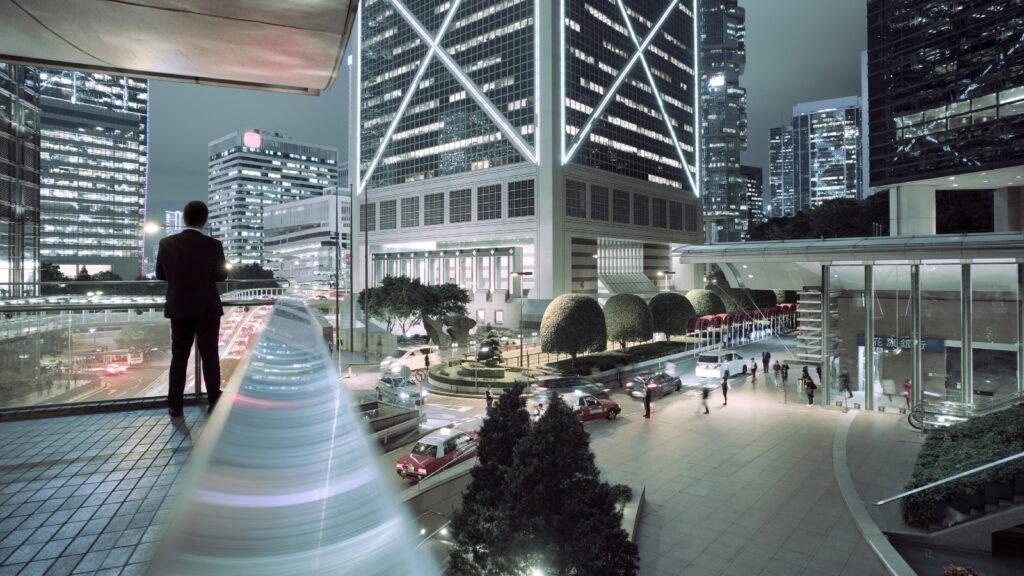
Operational resilience: where do we stand and what does this mean for cross-border banks?
Operational resilience is the ability of firms to prepare for, prevent, adapt and respond to, recover and learn from operational disruption. It is a complex and multi-faceted challenge for cross-border banks to prepare for and respond to. This is because potential causes of operational failure can enter a firm from several directions and their impacts […]
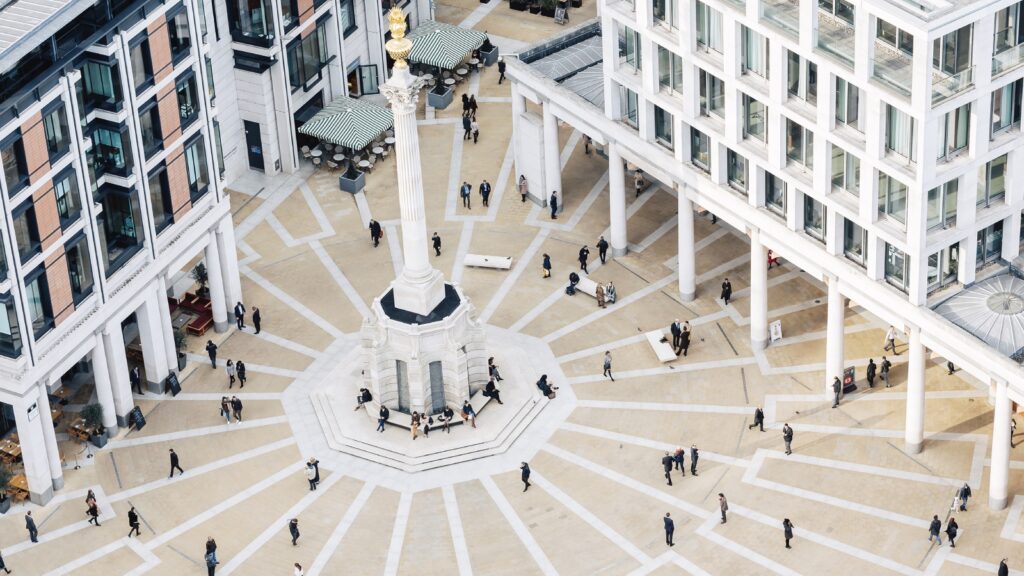
Basel 3 implementation: what is still at stake?
An EU vs UK vs US perspective Sixteen years ago, the collapse of Lehman Brothers triggered the global financial crisis, which highlighted significant weaknesses in the global banking sector. The oversight of the sector was quite fragmented between jurisdictions, leading to inadequacies in regulation and supervision. In response, the Basel Committee, which sets global standards […]
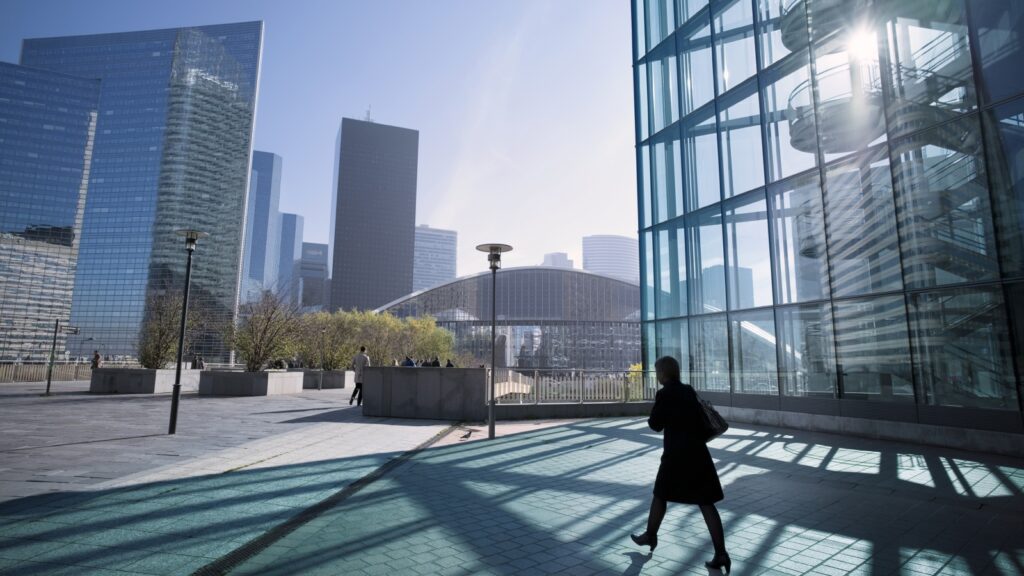
AMLA: what challenges may it face and what lessons can be learnt from other jurisdictions
Differing approaches to Anti-Money Laundering (AML) and Counter-Terrorist Financing (CTF) supervision across the EU have severely limited member countries’ ability to fight efficiently this growing threat. The creation of the Anti-Money Laundering and Countering the Financing of Terrorism Authority (AMLA) is a huge step in addressing this issue. AMLA will be charged with i) supervising […]
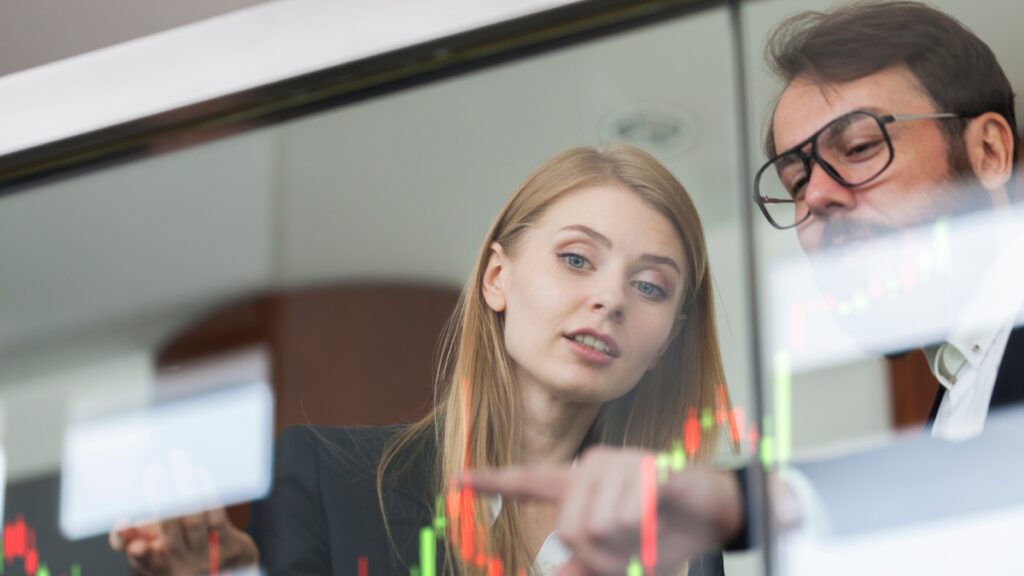
The pressure is on EU banks to rapidly improve their risk data capabilities
Following the 2007-08 global financial crisis, substantial deficiencies were identified in many banks’ risk data aggregation capabilities and risk reporting practices globally. This impacts banks’ ability to make timely risk decisions, creating risks not only for themselves but for the stability of the global financial systems. The Basel Committee responded by developing in 2013 the […]
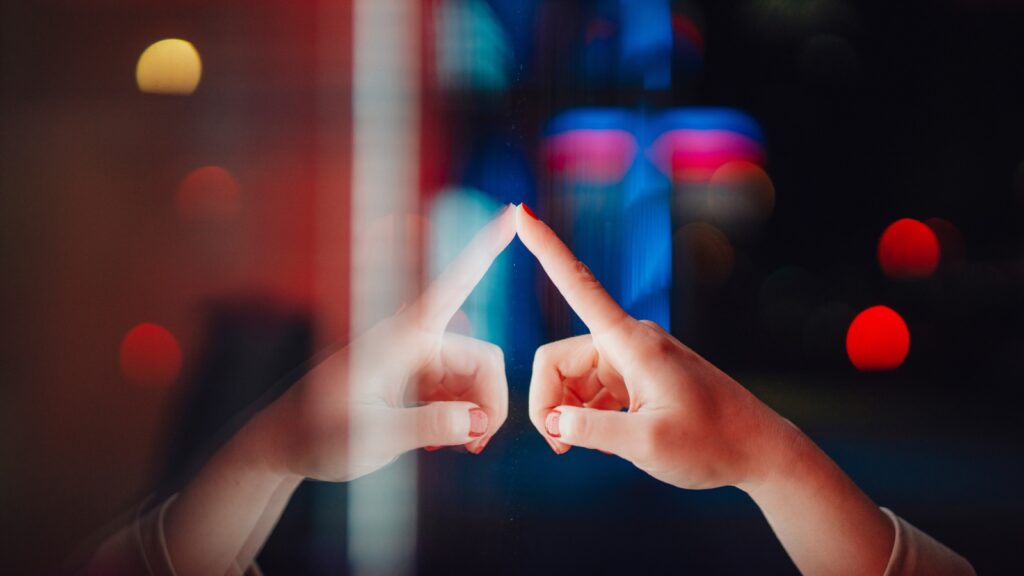
Maximise the value of the Cloud with FinOps: a guide to optimising Cloud costs
Financial institutions are faced with several challenges: strong economic pressure, regulatory changes, evolving customer expectations, punctuated by strong competition from fintechs and insurtechs; which rely natively on emerging technologies to offer best-in-class services. To meet these challenges and stand out from fintechs and insurtechs, financial services (FS) companies have invested massively in move-to-Cloud strategies, rapidly […]
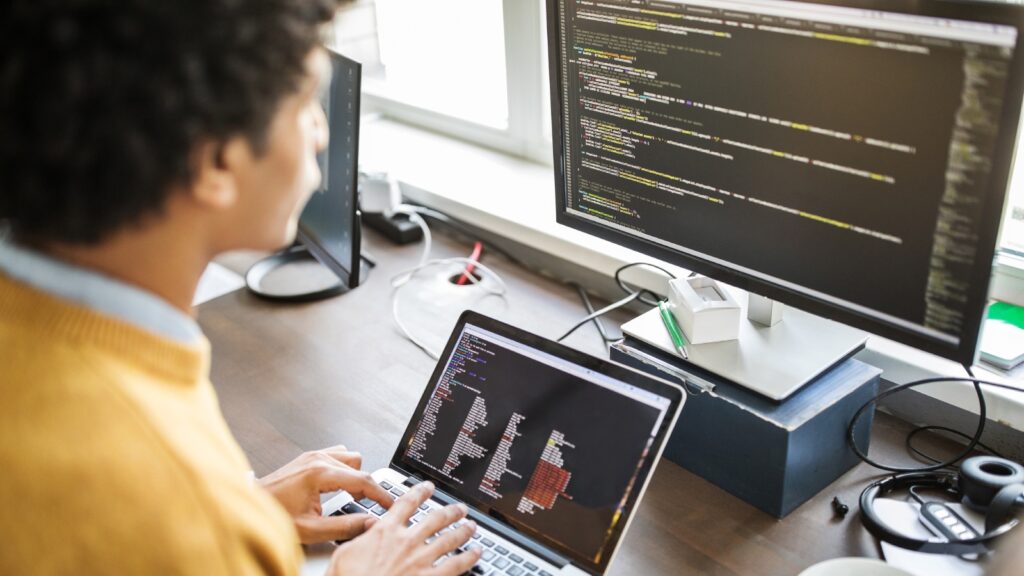
Reinforcement learning in financial companies: reconciling performance and ethics
Reinforcement Learning (RL) is a branch of Machine Learning (ML) that is still little known to the general public. In this approach, an agent repeats actions and, depending on the result of these actions, receives a reward or punishment in the form of a score. Focussed on maximising the score, the agent adapts the next […]
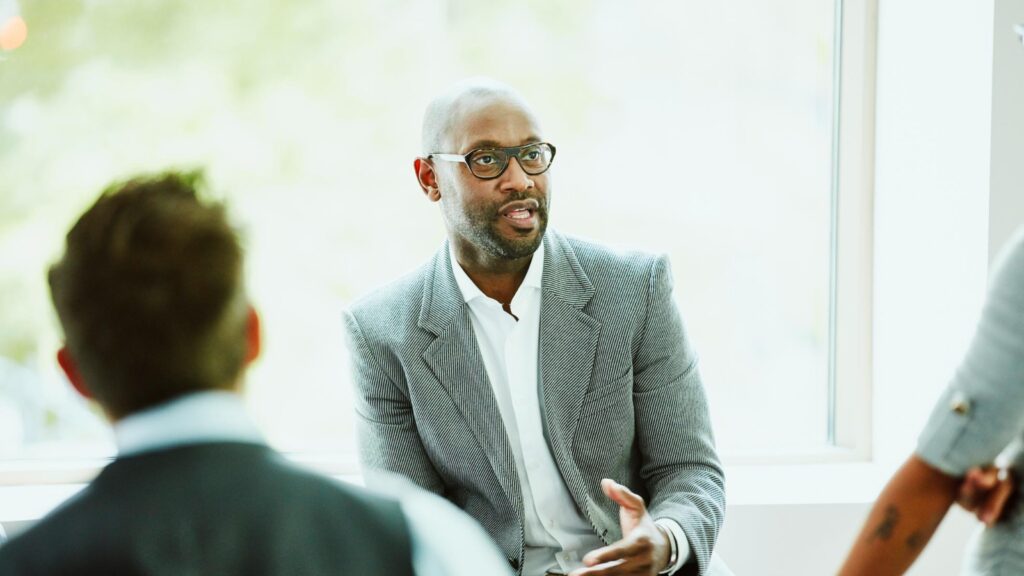
Bridging borders by combatting illicit financial flows and corruption for global financial integrity
Africa’s economic potential is hindered by the pervasive presence of illicit financial flows (IFFs), fraud, and corruption, which not only strip the continent of vital resources but also obstruct its path towards sustainable development. The staggering annual loss of $88.6 billion to IFFs, as reported by the United Nations Conference on Trade and Development (UNCTAD), […]
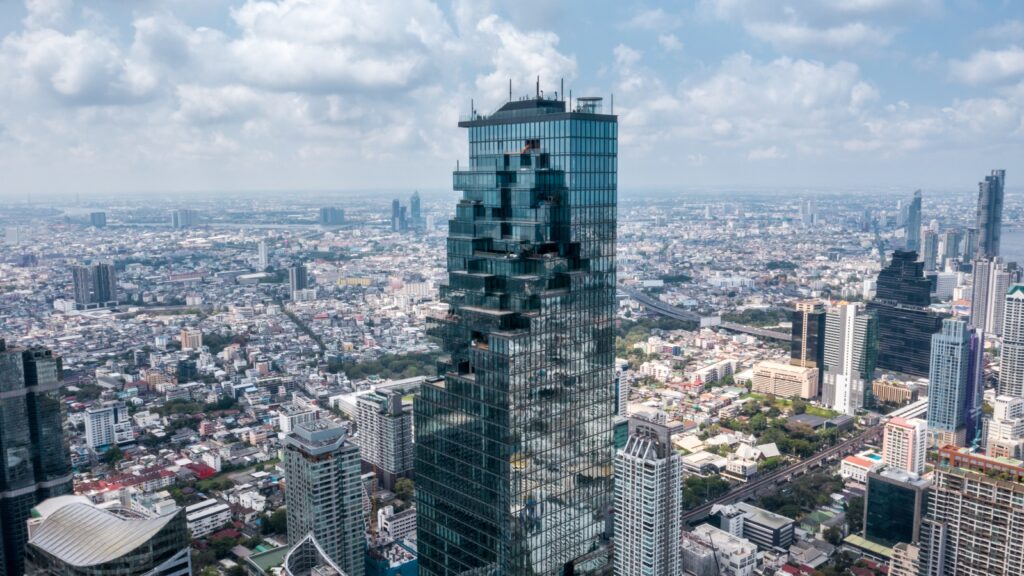
Strengthening global collaboration for asset confiscation and seizure: dynamics and innovations
In the ongoing battle against financial crimes spanning money laundering, terrorist financing, and the proliferation of weapons of mass destruction, collaborative efforts in asset confiscation and seizure stand as vital bulwarks. As we navigate the complexities of the financial landscape in 2024, recent advancements, particularly within the European Union (EU), underscore the critical importance of […]
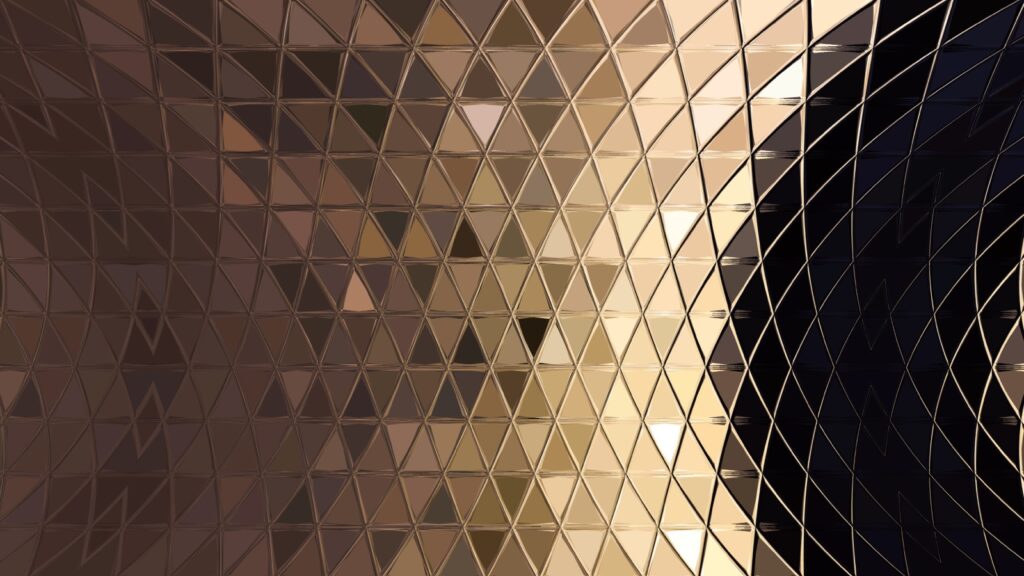
Our top risks for financial services firms in 2024
We have identified and ranked the key risks for financial services business leaders in 2024 based on market research, regulatory insights as well as our assessment of the current difficulties facing firms. We discuss in this article the key takeaways for you and your organisation. A more detailed assessment can be found here, which contains […]
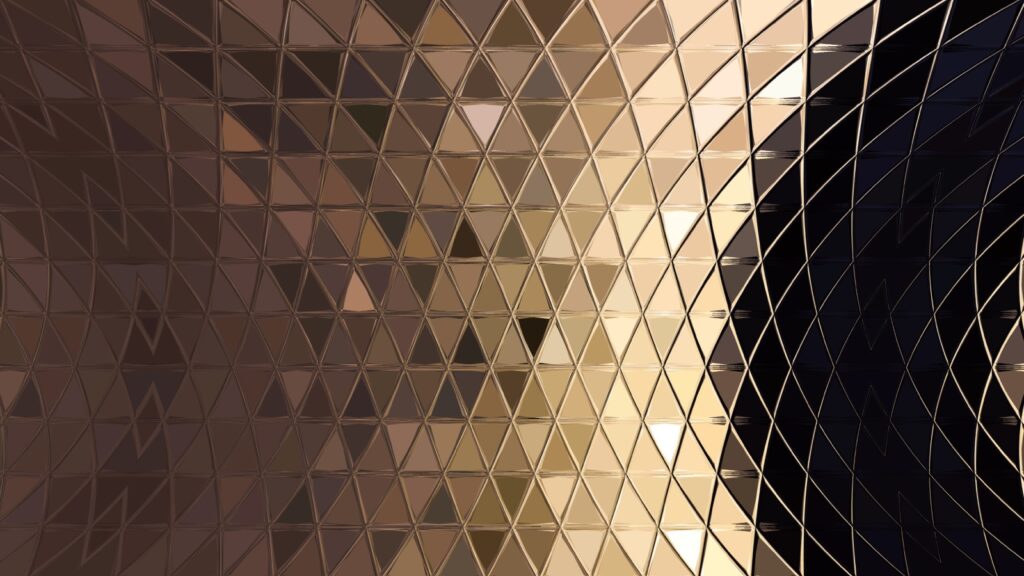
Our top risks for financial services firms in 2024 – complete analysis
We have identified and ranked the key risks for financial services business leaders in 2024 based on market research, regulatory insights as well as our assessment of the current difficulties facing firms. We also highlight the changes in risk rankings compared to last year, justified by global events and new regulations that have surfaced in […]
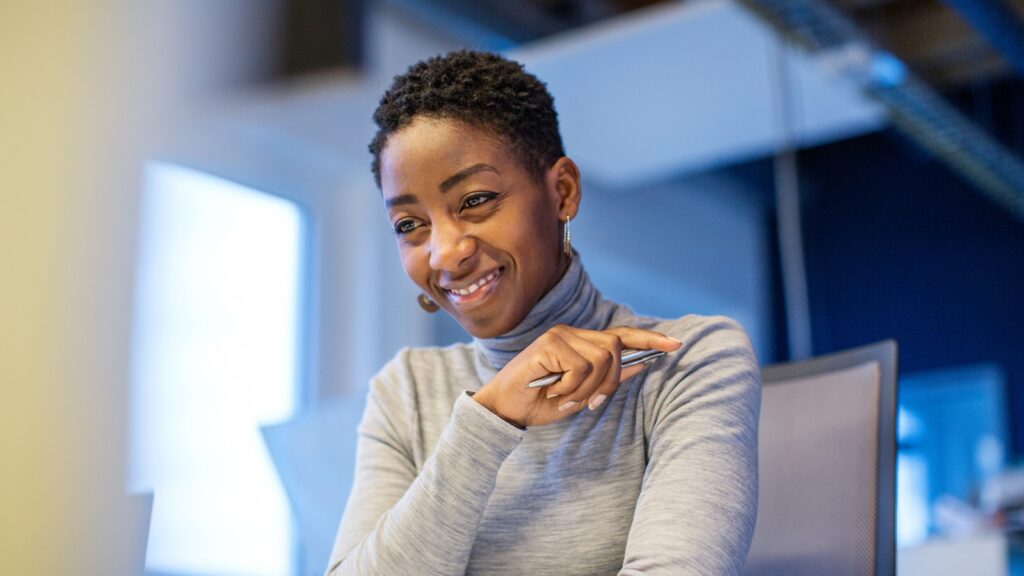
Unlocking the potential of ecosystems: a deep dive into open insurance and APIs
In the contemporary landscape of insurance, the business model of open insurance, characterised by the strategic opening of insurers’ resources to external collaborators, has been a focal point of discussion. Unlike the banking sector, where regulatory mandates such as the Payment Services Directive (PSD2) of 2018 have compelled the development of open banking practices, insurers […]
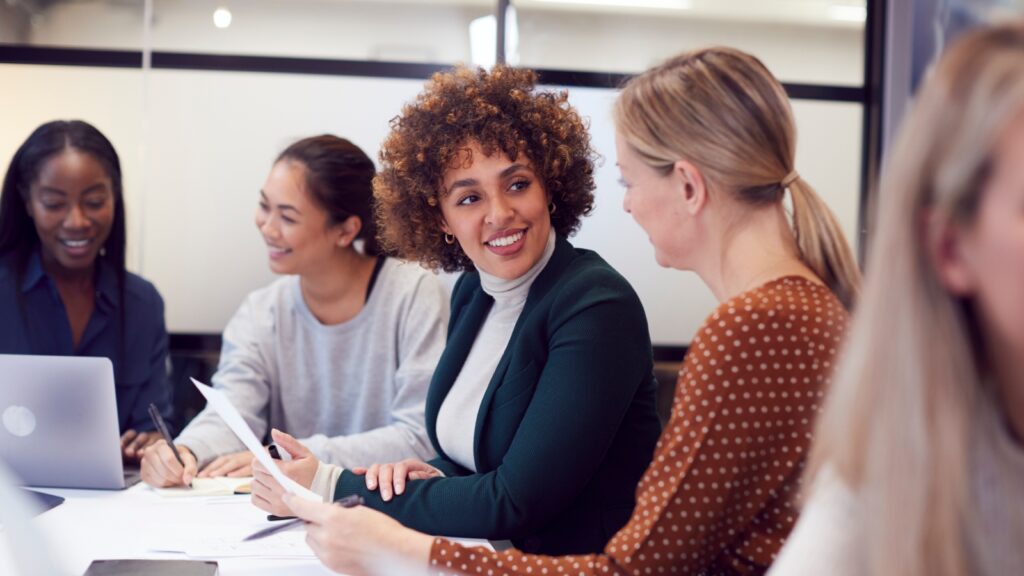
Being an Independent Non-Executive Director in different jurisdictions: lessons learned from Mazars in Ireland roundtable
Mazars recently hosted a Financial Services INED Roundtable Dining Event in Dublin[1]. Over 50 financial services independent non-executive directors (INEDs) attended, from banking, asset management, funds and insurance entities operating across the EU and UK. The roundtable focussed on the challenges facing INEDs in the current unpredictable macroeconomic environment. The new and emerging risks and […]
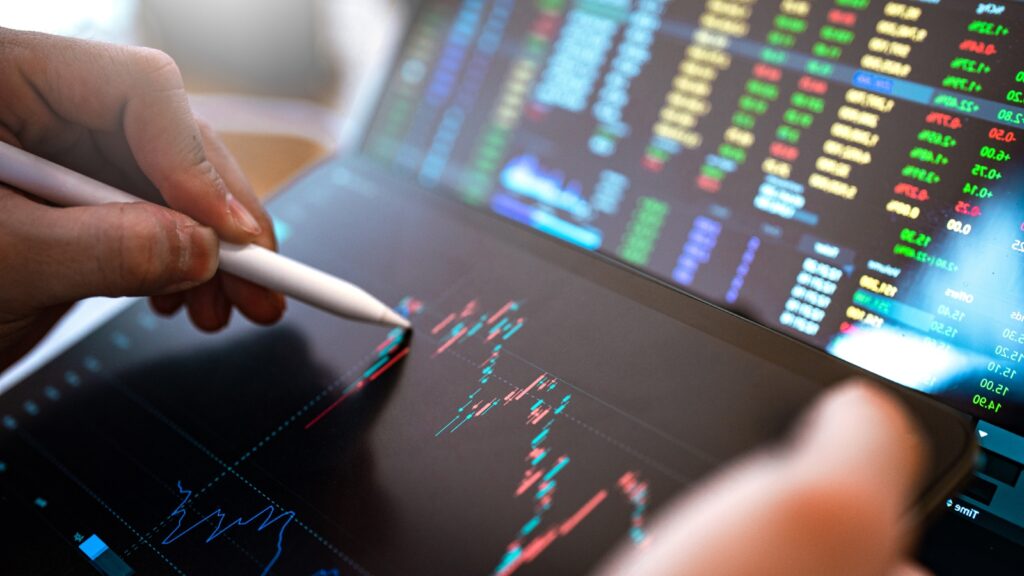
Navigating challenges in the retail digital euro landscape
Last October, the European Central Bank (ECB) initiated the preparation phase of the Digital Euro project, following two years of investigation and a proposal on the establishment of a digital euro published by the European Parliament (EP) in June 2023. In January, the ECB began seeking potential providers to develop a digital euro platform and infrastructure […]
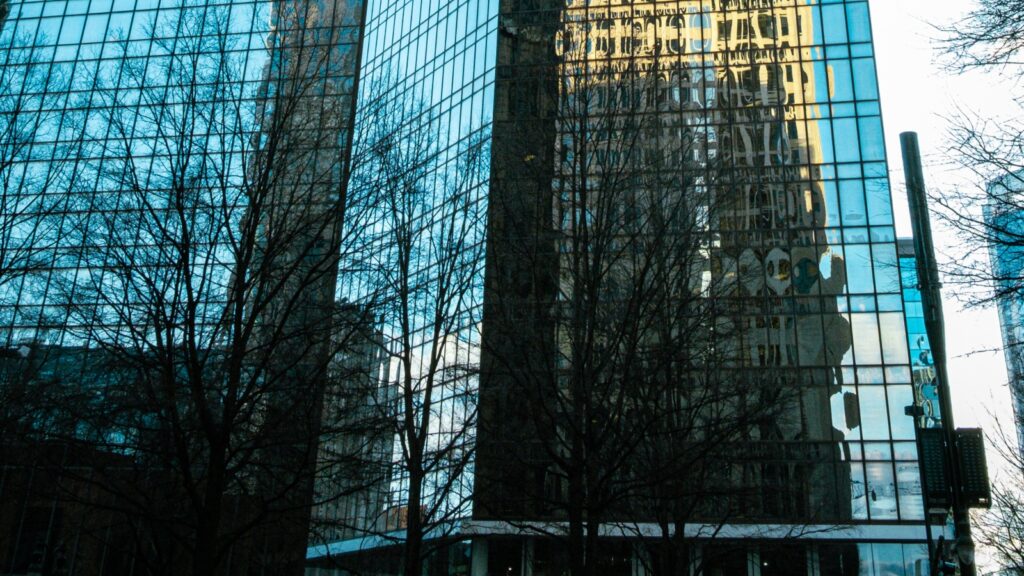
The European Central Bank’s priorities for 2024: where do we stand after the first quarter?
The European Central Bank (ECB) issued the SSM supervisory priorities for the 2024-2026 cycle on 19 December 2023. They sum up what institutions under the direct supervision of the ECB should expect in terms of areas of supervision, in 2024 notably, and allow firms to prepare themselves for forthcoming onsite inspections or thematic reviews. Please […]
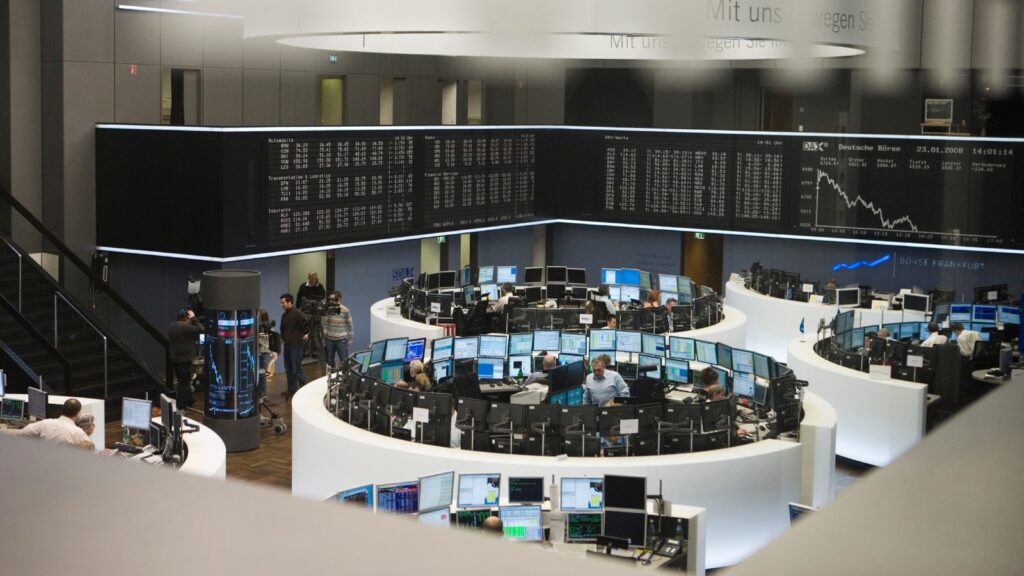
Unveiling the European Central Bank’s strategy: data, scenarios and models
In January 2024, the European Central Bank (ECB) published its Climate and Nature Plan for 2024-2025. This plan aims to: This plan underscores those financial risks stemming from climate change remain a key area of attention for the ECB. The ECB will continue working on several topics including stress testing, scenarios and climate-related data, and […]
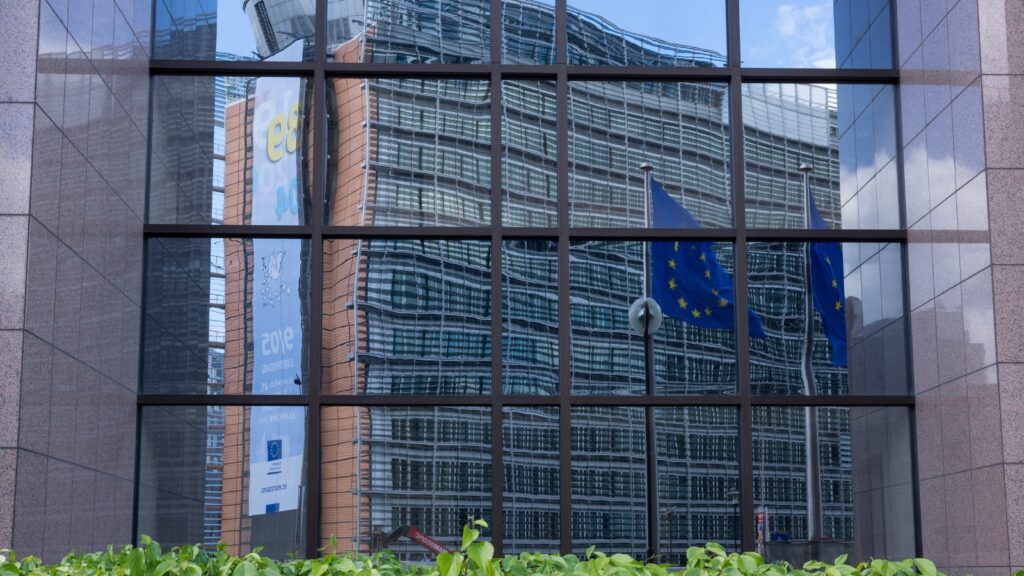
Drafting the future: unveiling the next chapter of DORA
DORA is a legislative proposal that aims to improve the digital operational resilience and ensure the performance and stability of the financial system of the member countries of the European Union in the face of the risks associated with ICT (Information and Communication Technology) in the financial sector (cyber-threats, cyber-attacks). The DORA requirements will apply […]
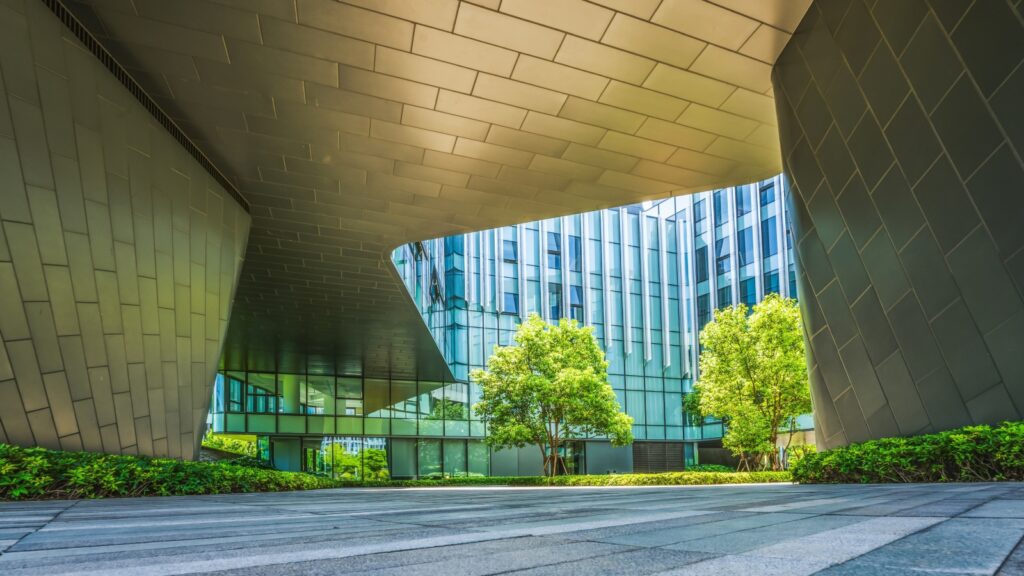
How banks and insurers have progressed in embedding sustainability into their businesses
In late 2023, and to coincide with COP 28, Mazars published its latest Sustainability practices survey on the progress banks and insurers have made in embedding sustainability into their businesses, our most comprehensive and information-rich report to date covering 404 executives in banks and insurance companies in 16 countries. Despite sustainability being in the limelight […]
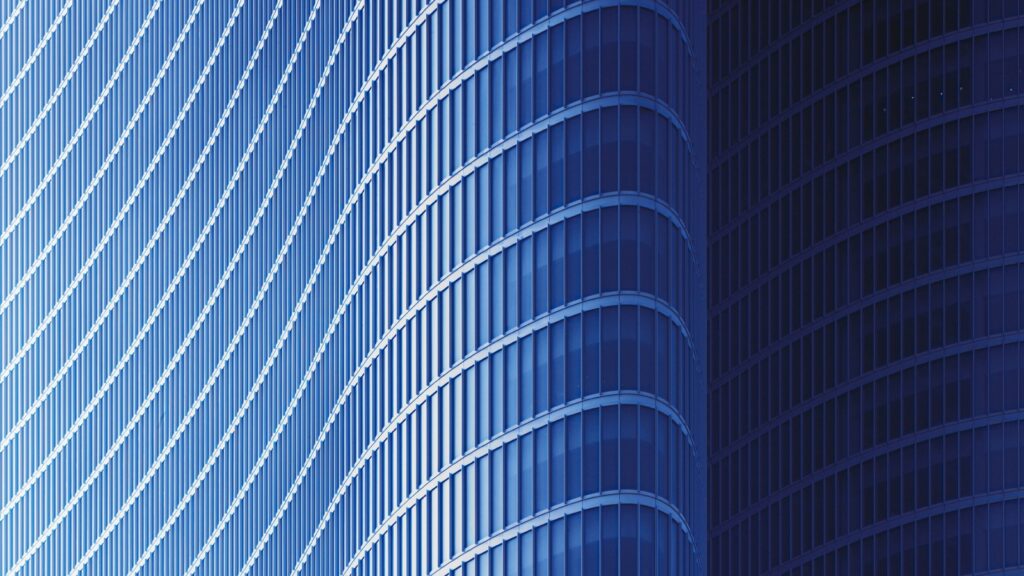
SRB annual conference 2024: entering a new phase of banks testing and operationalisation of resolution plans
The Single Resolution Board (SRB) convened its annual conference on 13 February 2024 with a theme highlighting the focus for the year: ‘The road ahead: risk, readiness and resilience’. While significant strides have been made with the EU banking resolution framework and tools, the SRB’s Chair Dominique Laboureix recalled this is not the end of […]
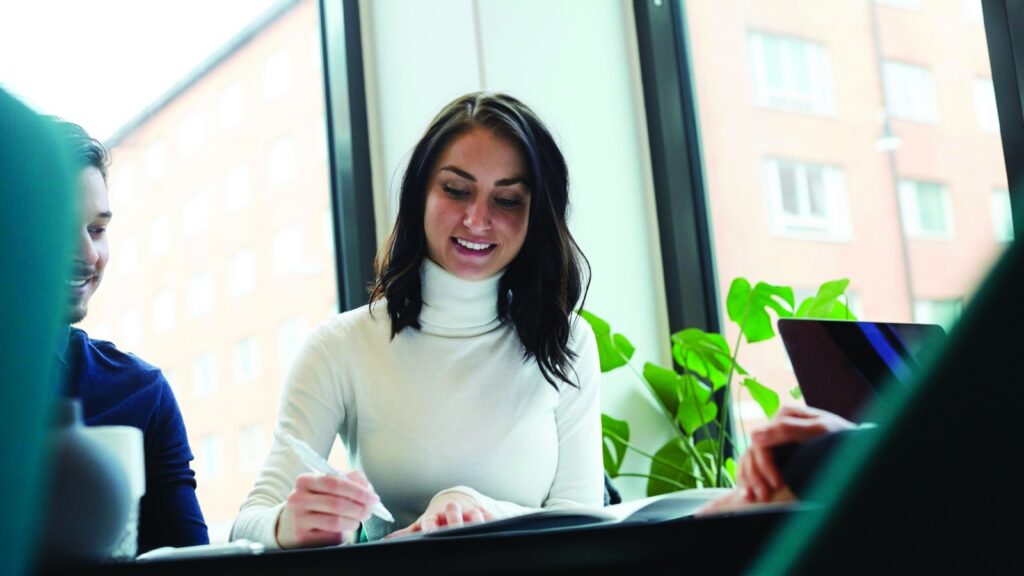
Assessing materiality and verification of sustainability disclosures
In environmental, social and governance (ESG) reporting, materiality is crucial for enhancing transparency and accountability in sustainability and climate-related disclosures. Importantly, it helps identify and report on matters that are deemed significant, emphasizing their relevance to stakeholders. Materiality comes in various forms. Financial materiality focuses on sustainability issues impacting financial performance, aligning with annual financial […]
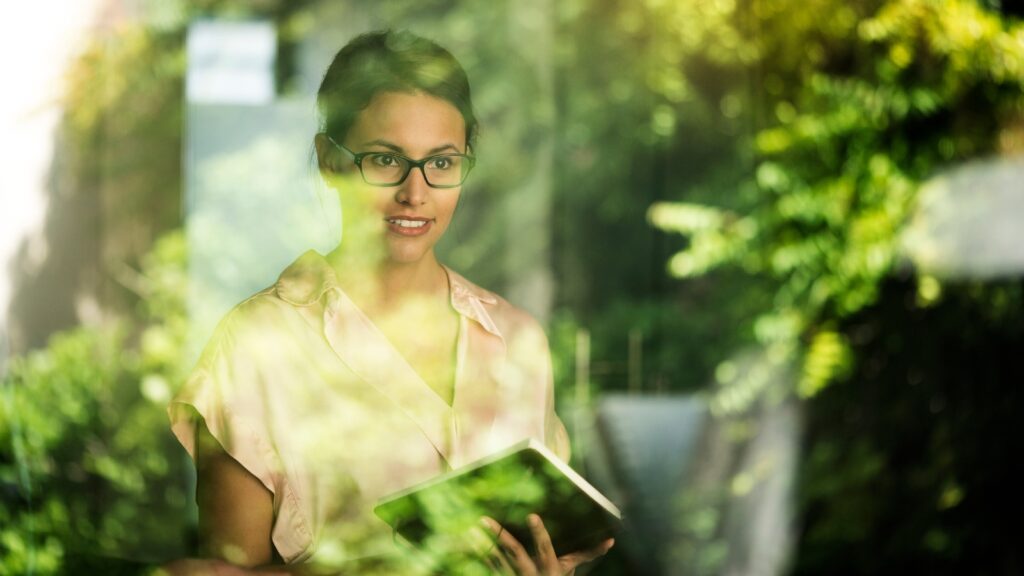
What’s driving financial firms’ sustainability strategies?
To adapt to the swiftly evolving regulatory landscape and meet stakeholders’ expectations, financial firms are increasingly formulating sustainability strategies to address environmental, social and governance (ESG) factors. Notably, emissions reduction and the pursuit of net-zero targets have become central elements of ESG strategies for many financial firms, according to the latest Mazars’ survey Sustainability practices stocktake: […]
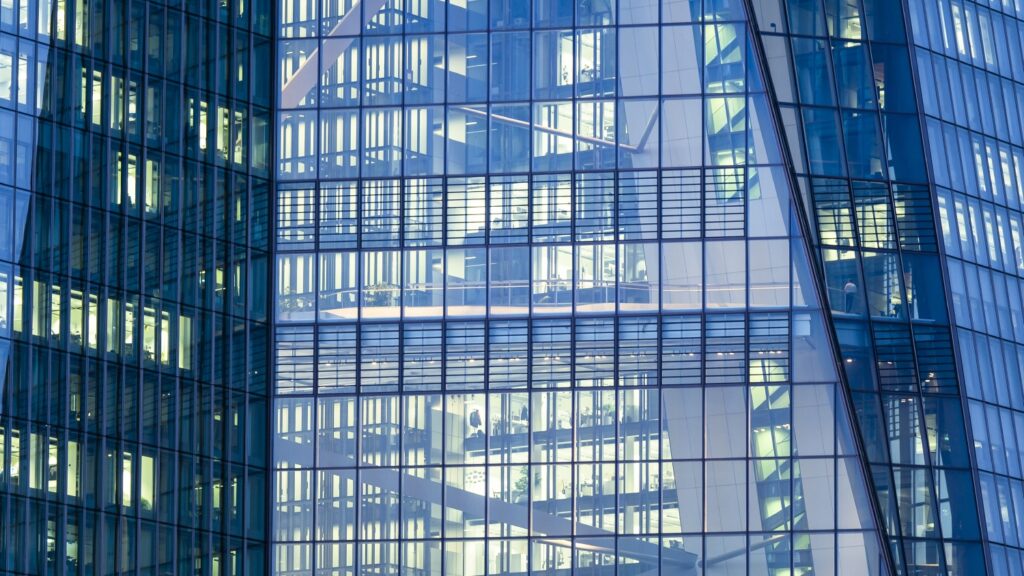
Market in crypto assets regulation: where we stand now
On 29 June 2023, the European Union’s (EU) Markets in Crypto Assets Regulation (MiCA) entered into force. It is being implemented in stages depending on the provisions, between June and December 2024. The regulation, which provides clearer rules for crypto-asset service providers and token issuers, is much needed for this fast-changing industry. The rapidly approaching […]
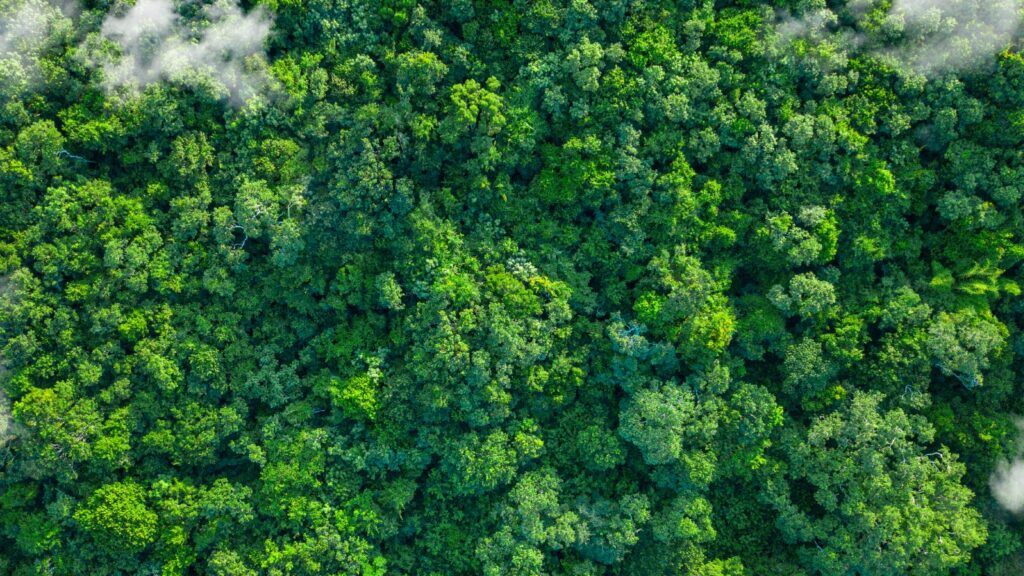
Mitigating the financial impacts of climate-related risks
The integration of environmental, social and governance (ESG) considerations into strategic planning is increasingly becoming a common practice among financial services firms. However, climate-related risks can also serve as drivers of financial risk for institutions. These risks can manifest through various transmission channels, translating climate and environmental (C&E) risks into more conventional categories such as […]
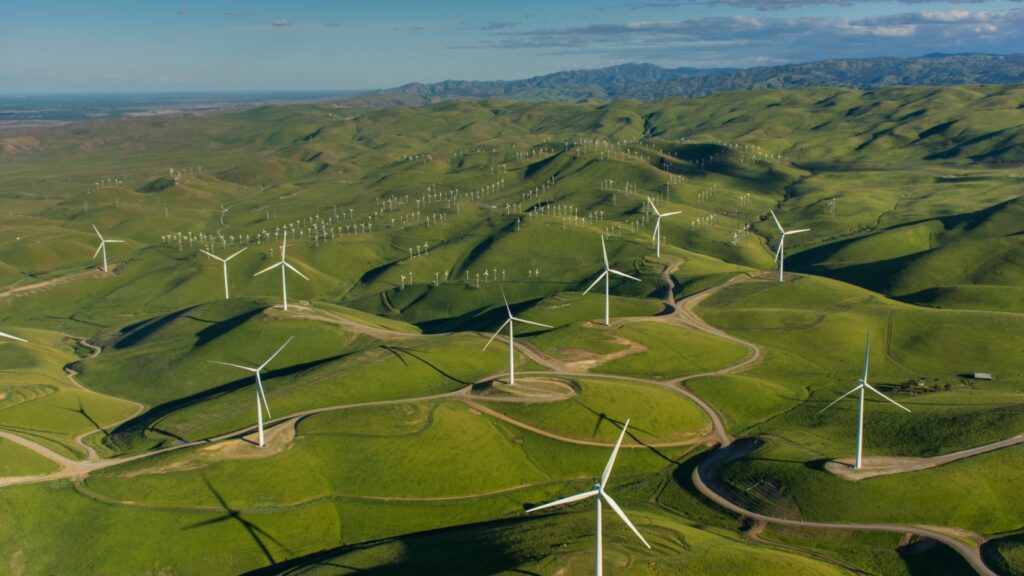
How are financial institutions reflecting C&E considerations in risk appetite statements?
There is growing pressure for banks and insurers to incorporate C&E factors in their risk management frameworks (RMF). As a practice, it gives the ability to set clear thresholds for the climate impacts banks and insurers are willing and able to absorb. By establishing these thresholds, firms can effectively monitor their exposure to C&E risks, […]
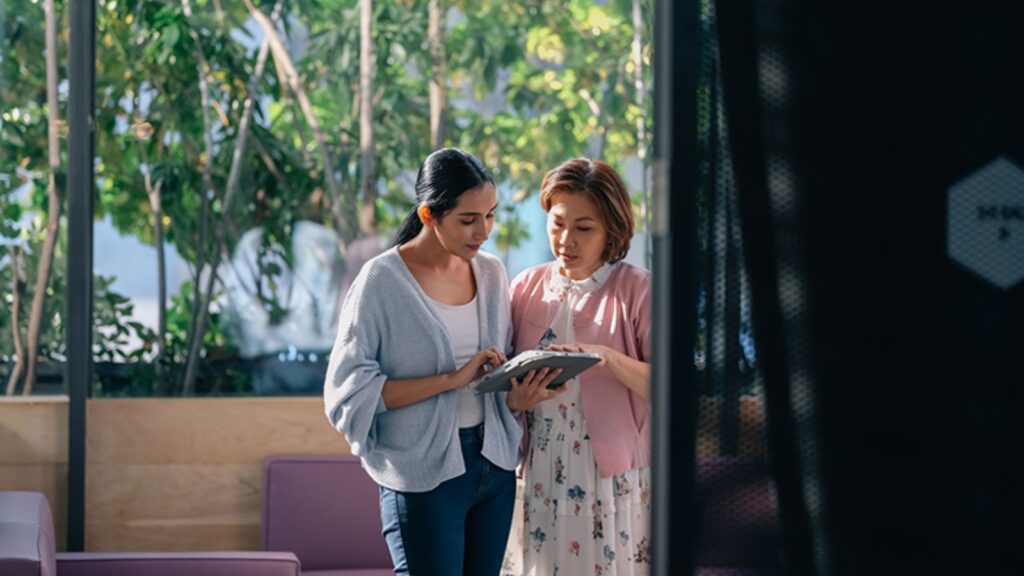
Adapting governance to spearhead sustainability more effectively
There are increasing regulatory expectations globally for financial institutions to disclose and demonstrate how sustainability-related responsibilities are allocated within the organisation. In this respect, the increasing global trend towards mandatory sustainability disclosure frameworks continues to underscore the significant role that the finance function is anticipated to assume in sustainability. It’s a trend reflected in Mazars’ […]
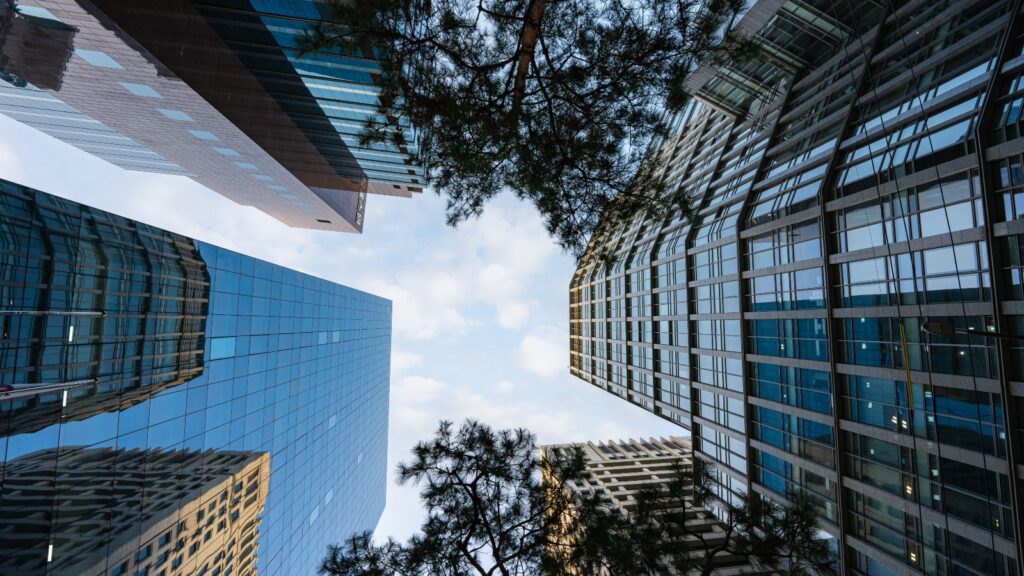
SSM supervisory priorities for 2024-2026: addressing identified vulnerabilities in banks
On 19 December 2023, the European Central Bank (ECB) published its updated Single Supervisory Mechanism (SSM) strategic supervisory priorities for the period 2024 to 2026. The priorities indicate what banks should expect in terms of supervisory activities in 2024. For 2024-2026, the ECB supervisory priorities are built upon three main pillars: These priorities were informed […]
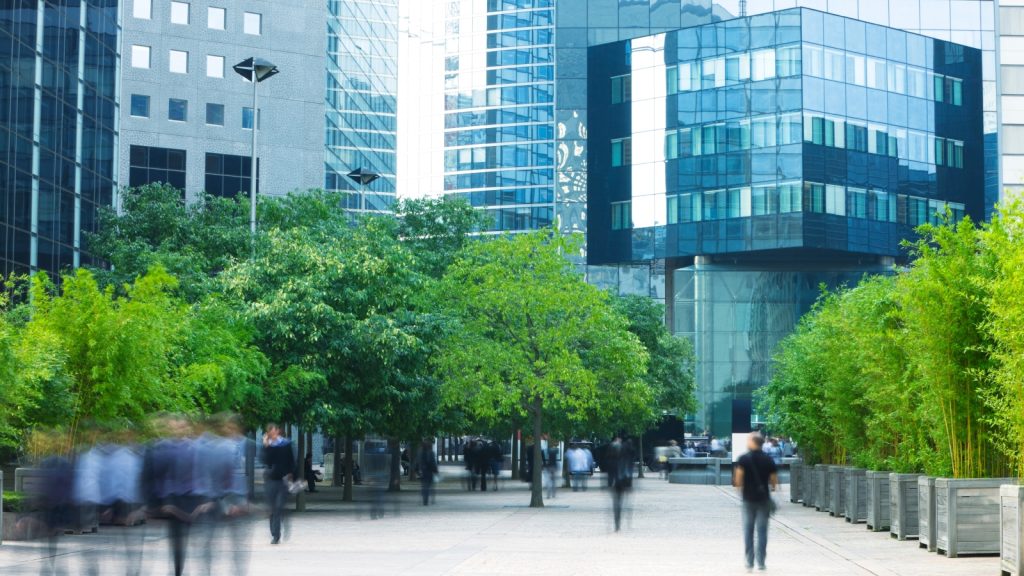
How financial institutions can move sustainability reporting to real-world application
The increased demand for sustainable finance shows that awareness of environmental, social and governance (ESG) issues is generally high among financial institutions. Indeed, for many of the larger players subject to the EU’s Non-Financial Reporting Directive (NFRD), there has been a requirement to include ESG information in annual reports for some time. Others have incorporated […]
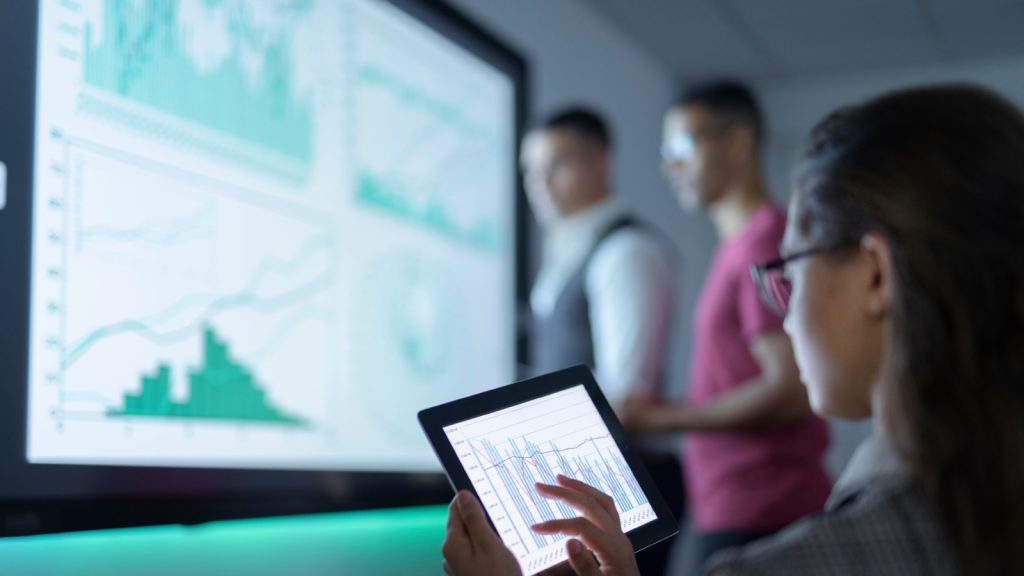
Managing tomorrow’s banking risks
While the banking sector has shown resilience over recent years, the economic environment and geopolitical situation remain tense. So, what does this mean for risks to the banking sector? More specifically, what is the impact on capital requirements for banks with the implementation of the Capital Requirements Regulation (CRR3) and the Capital Requirements Directive (CRD6), […]
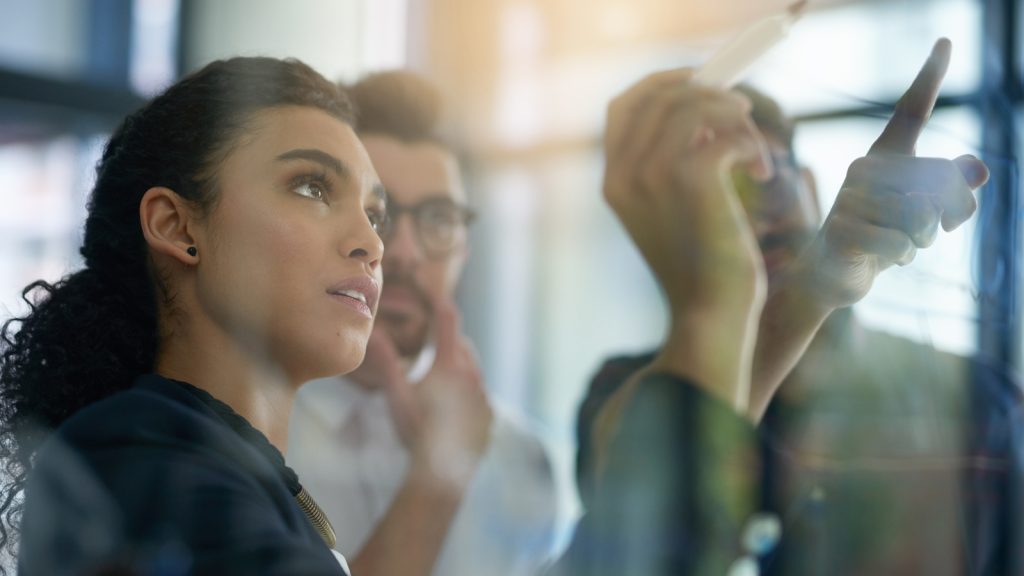
Lessons from the spring 2023 banking turmoil: five areas for banks to focus their attention
The Basel Committee on Banking Supervision (BCBS) and Financial Stability Board (FSB) published reports in October 2023 on the causes and lessons learnt from the Spring 2023 banking turmoil. The BCBS report provides an assessment of the causes of the banking turmoil, the regulatory and supervisory responses, and the initial lessons learnt. The FSB report […]
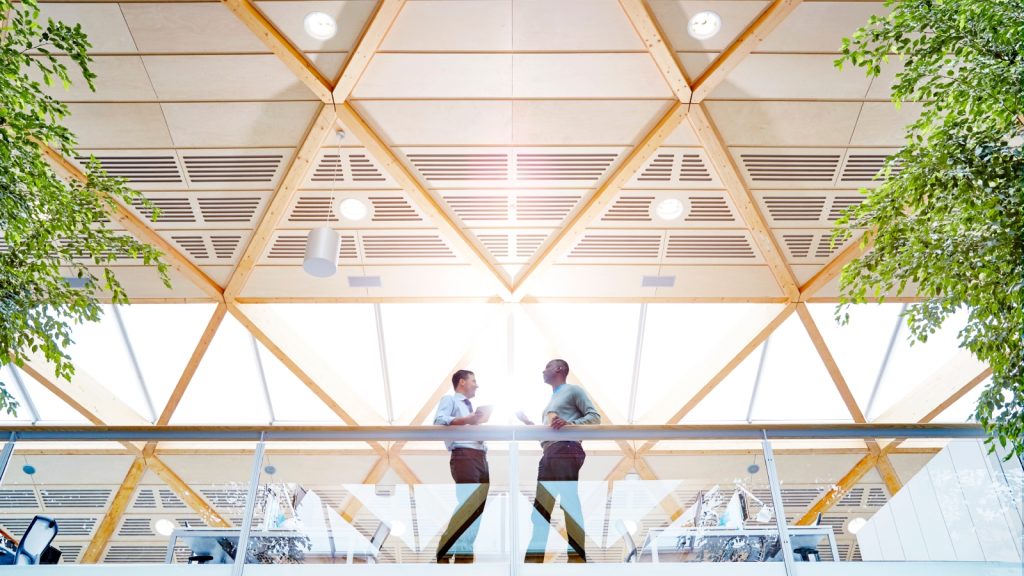
European green taxonomy eligibility ratios in the banking sector
The implementation of the European Union’s ‘Taxonomy’ regulation, which integrates two climate objectives, was carried out on 1 January 2022 through the ‘Climate’ Delegated Act released in April 2021. In line with this, the banking sector has been provided with corresponding regulations, allowing banks to measure the portion of their financing dedicated to sustainable economic […]
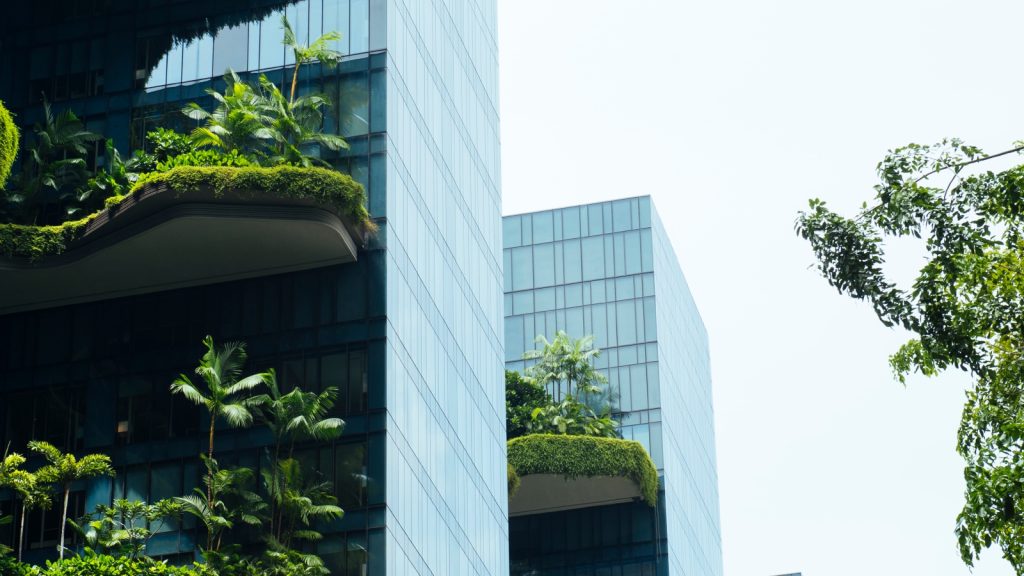
Transitioning to greener practices in the real estate sector
In 2022, the European Union implemented the green taxonomy for the second year, requiring companies to disclose indicators related to climate objectives. The green taxonomy aims to guide capital investment towards environmentally sustainable activities, making companies assess their alignment with the EU’s sustainable transition and enabling financial institutions to prioritise funding for projects contributing the […]
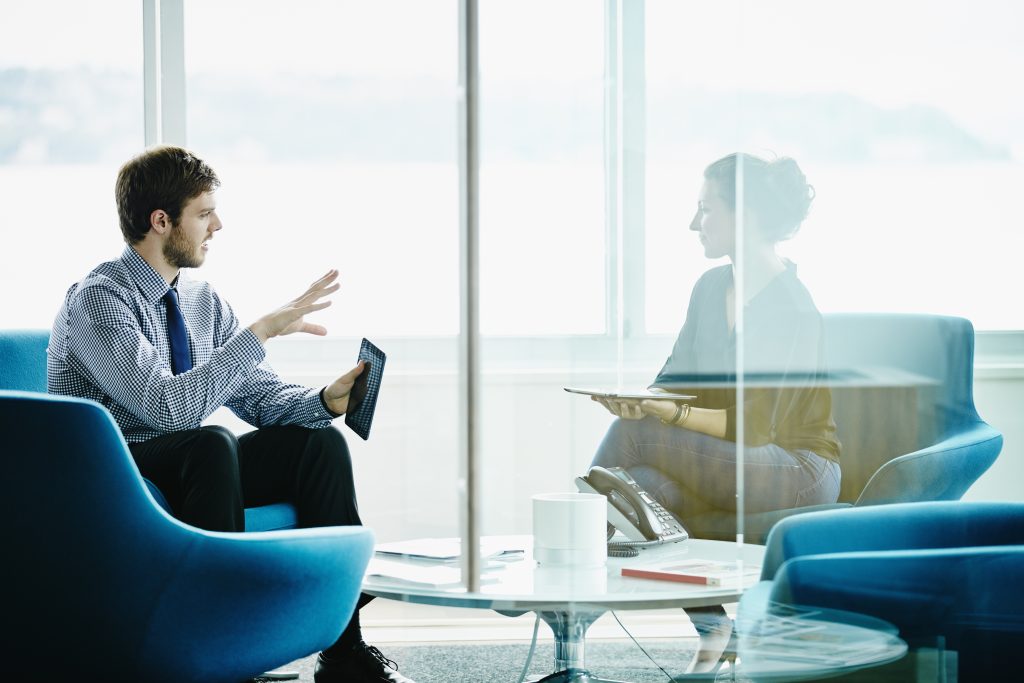
Eligibility ratios in the insurance sector: improved practices based on recommendations issued by regulators
2023 marks the second year in which insurance and reinsurance companies have published their eligibility ratios for the European Green Taxonomy. For the insurance sector, the objective is to measure the proportion of investments, as well as the proportion of gross premiums collected in non-life insurance, dedicated to financing economic activities in accordance with the […]
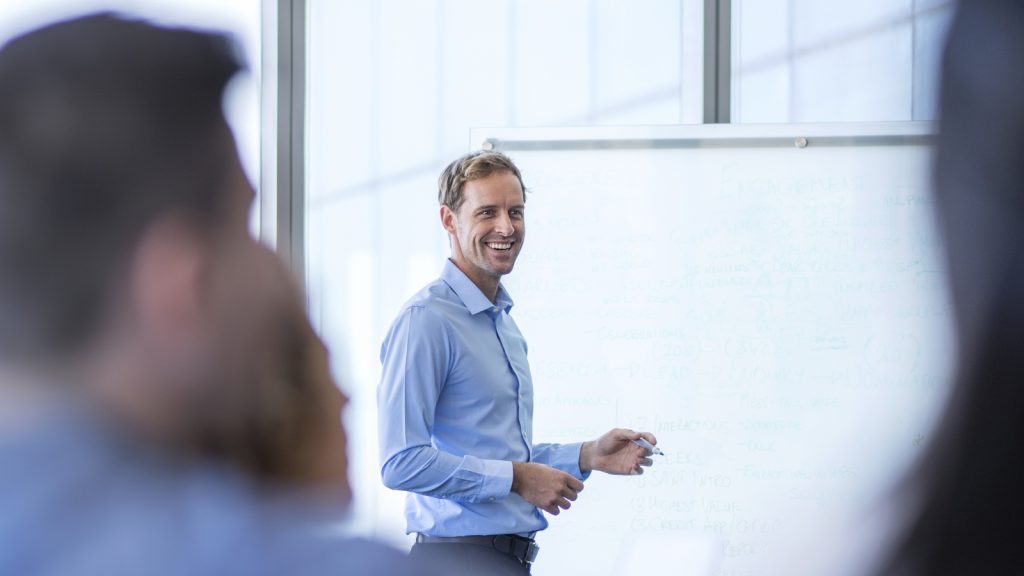
IIF annual membership meeting: building resilience amid turbulence and transformation
The IIF Annual Membership meeting is a setting for insights and perspectives from global financial regulators and senior financial sector executives on topical economic and regulatory issues. Being a forum with global coverage means that it is a valuable setting for picking up future economic and regulatory directions. A packed agenda under the theme of Building […]
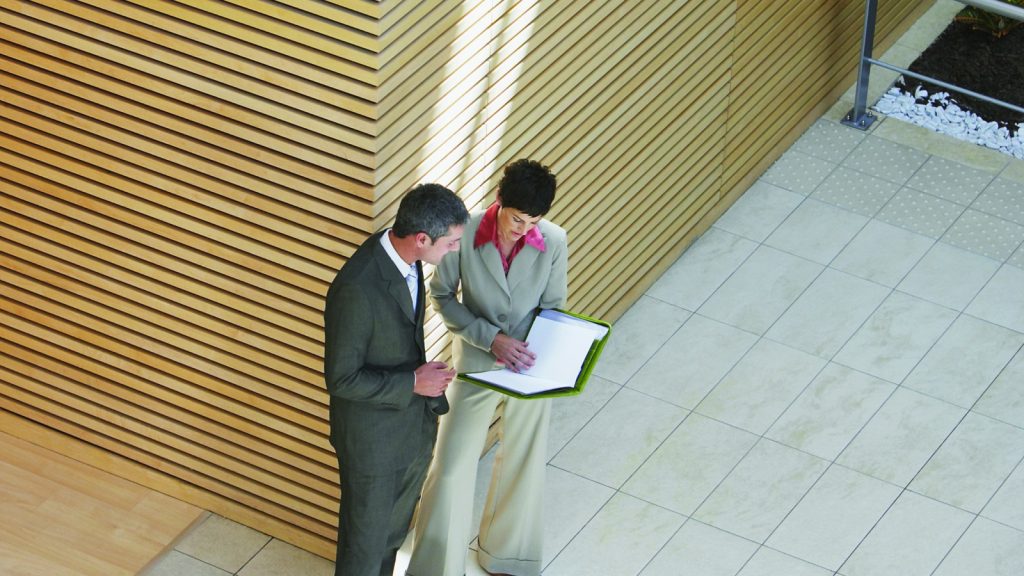
DORA: how to move from operational risk management to operational resilience?
DORA (the Digital Operational Resilience Act) is the key regulatory outlook for IT and Cyber risk between now and 2025. The European Supervisory Agencies have sought to strengthen the resilience of institutions by emphasising the need to evolve the approach to operational risk management, of which information and communication technology risks are a part. DORA […]
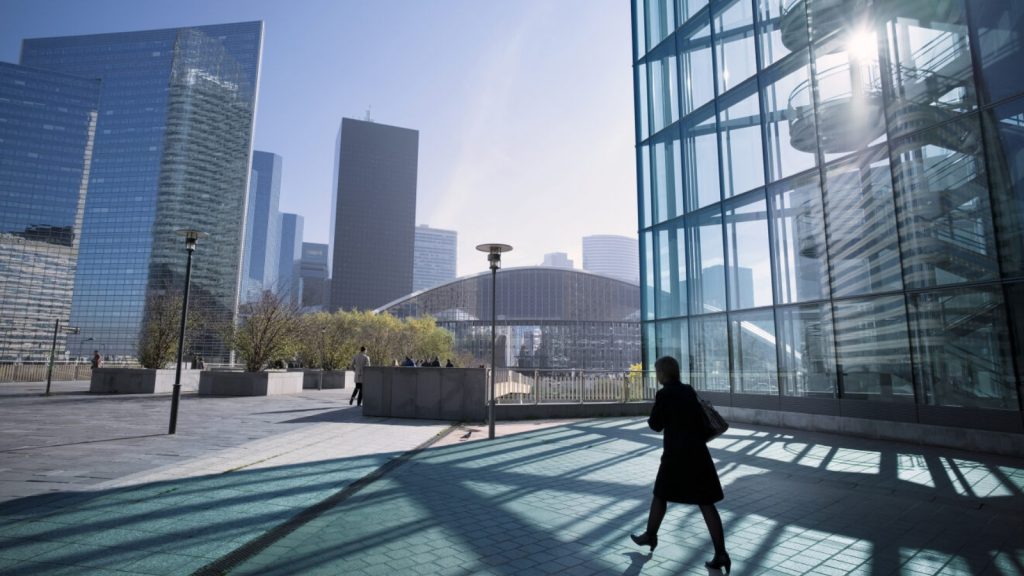
Diversity in forward-looking macroeconomic scenarios
Under IFRS 9, forward-looking information is a key component of Expected Credit Loss (ECL) calculations. However, forward-looking information requires a significant level of judgement, making comparisons difficult to navigate. Indeed, similar to the use of post-model adjustments, forward-looking scenarios have also been reported by stakeholders in the context of the IFRS 9 impairment post-implementation review […]
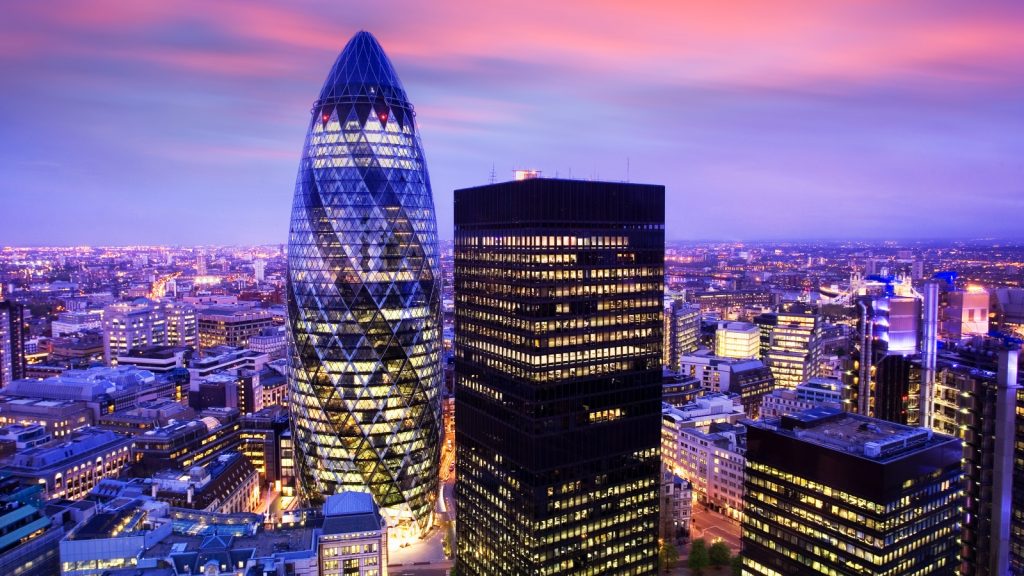
Bank credit risk trends show a relative decrease in high risk exposures
Despite banks emerging from the Covid-19 crisis in reasonably good health, the war in Ukraine combined with a global energy crisis and an uncertain economic landscape have once again put the spotlight on credit risk exposures. To better understand credit risk trends, Mazars conducted an analysis of 26 banks in 11 European countries in May […]
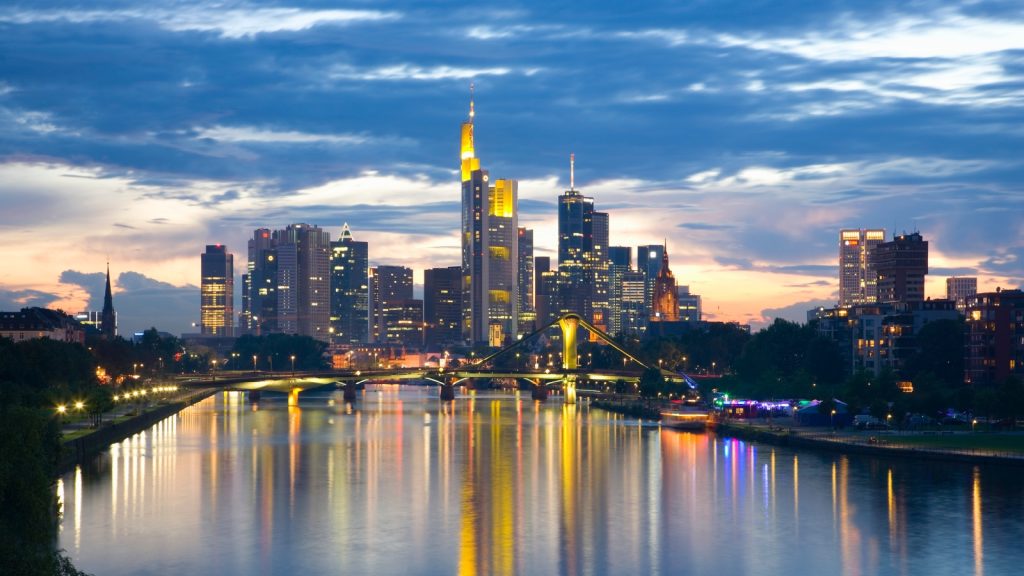
The use of post-model adjustments to capture emerging risks
Since the Covid-19 pandemic, post-model adjustments1, or management overlays, have become an increasingly common and accepted mechanism used by banks to manage expected credit losses (ECLs). The number of post-Covid unprecedented events related to the war in Ukraine, energy crisis and global economic uncertainty has raised a number of questions relating to the consistency and […]
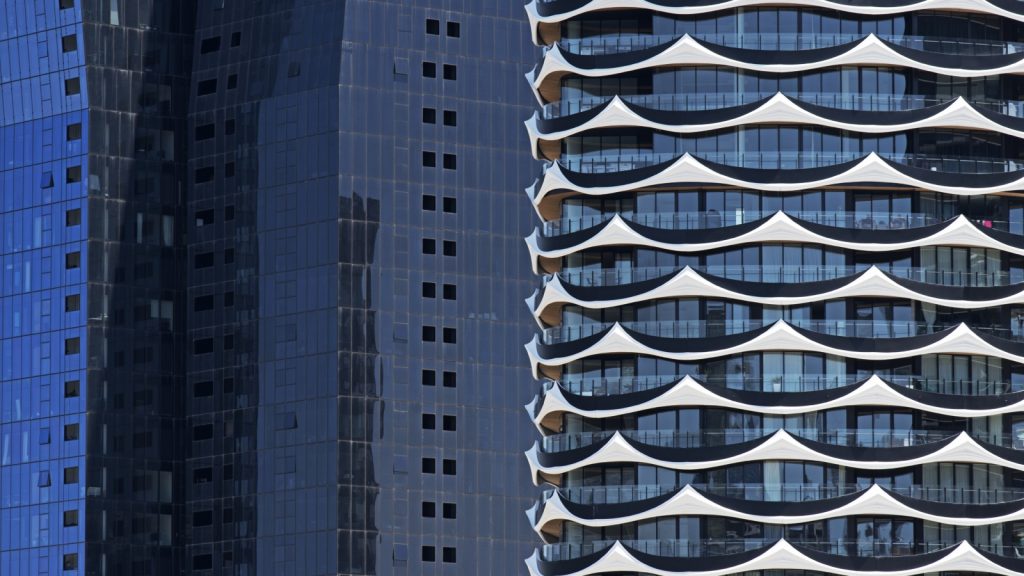
Equipping NEDs to challenge private investment valuations
A recent major board reshuffle in one of Europe’s largest listed investment companies has focused attention on private investment valuations. It follows concerns raised by an ex-director over the robustness of the directors’ processes for approving investment valuations. The issues primarily question whether the Board of Directors has sufficient training and experience and whether governance […]
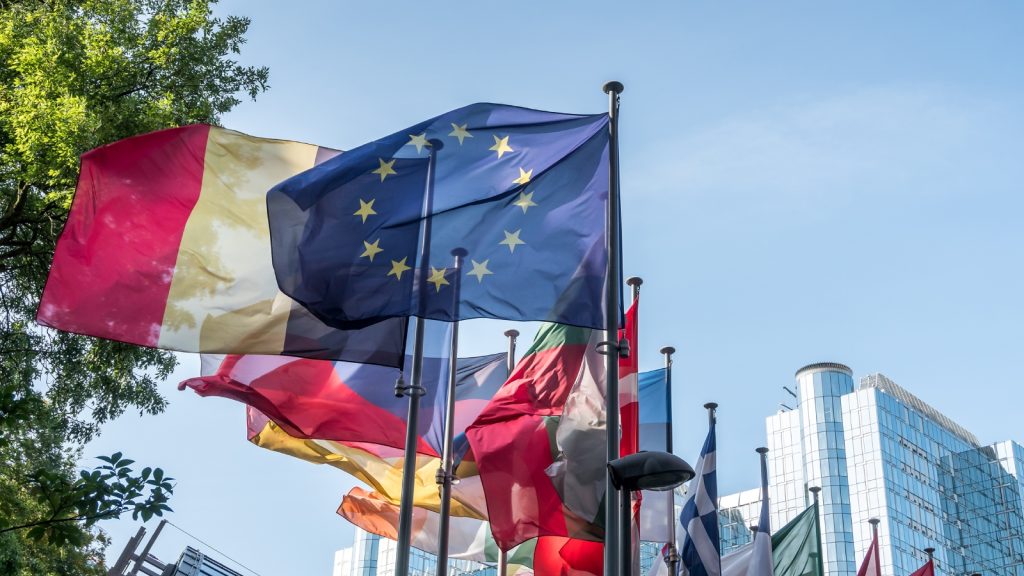
EU bodies update country lists of uncooperative and high-risk countries for financial services
Revised list of uncooperative countries and territories for tax purposes published by the European Council The list of uncooperative countries and territories about tax is an important part of the external tax strategy of the EU. Globally, this strategy intends to contribute to the ongoing efforts to advance good governance practices in the tax domain. […]
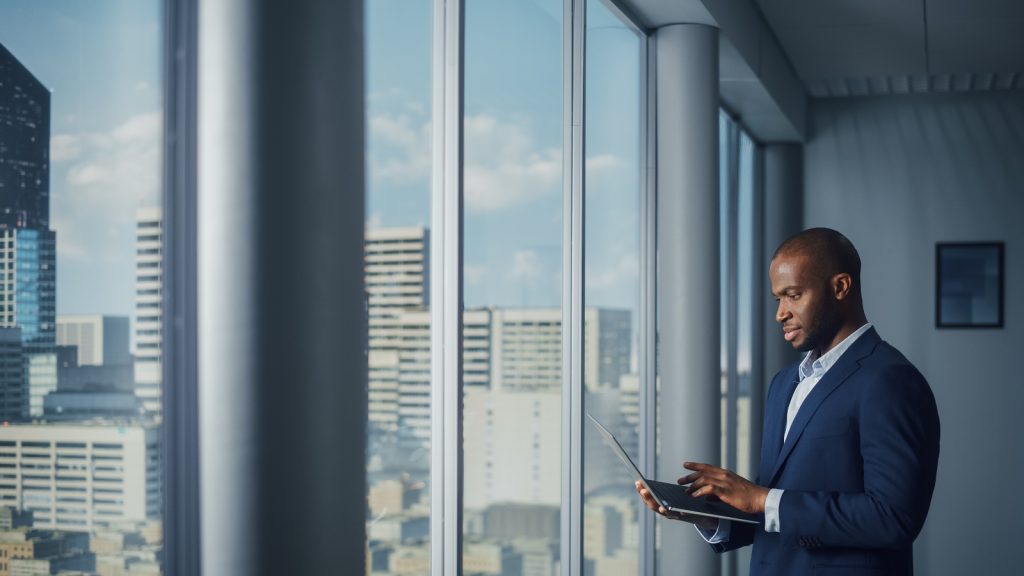
New reports on transaction monitoring systems and risk analysis published by the ACPR and COLB
ACPR publishes report on automated AML/CFT transaction monitoring systems In 2022, the ACPR conducted a comprehensive thematic review, focussed on the automated systems utilised by the entities under its supervision. This entails entities implementing their obligations in terms of transaction monitoring. The primary objective of this review was to assess the efficiency of the operation and […]
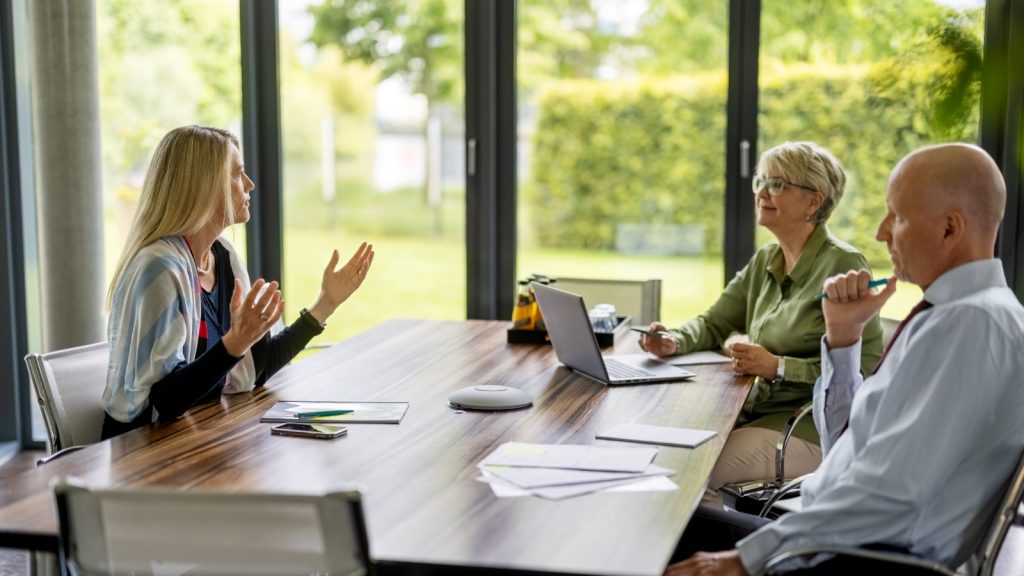
EUROFI financial forum: strengthening economic union and European competitiveness
The Eurofi financial forum is a setting for exchanges between European Union (EU) economic and financial regulators and senior financial sector executives from the industry. It occurs bi-annually alongside the Economic and Financial Affairs Council configuration (ECOFIN) meetings. This summary takes stock of the Eurofi discussions, as well as recent publications by the EU Commission […]
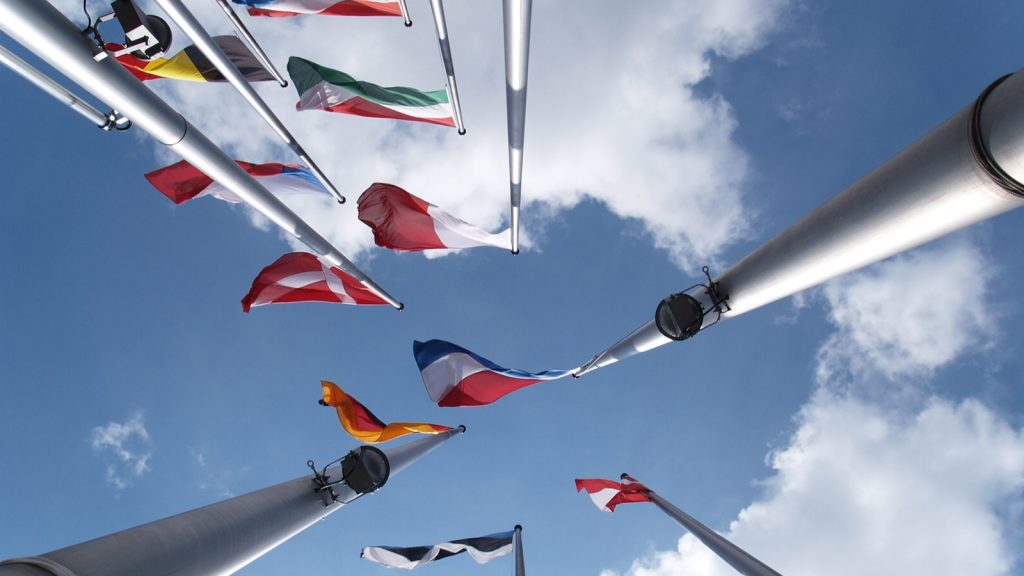
The Council of Europe provides updates on combatting the financing of terrorism
2022 AML-CFT Committee report available The MONEYVAL Committee, an entity of the Council of Europe which is tasked with addressing challenge of money laundering and terrorist financing (ML/FT), has recently published the AML-CFT report 2022. The findings of the report are primarily centred around adherence to compliance with global sanctions, notably in freezing or confiscation […]
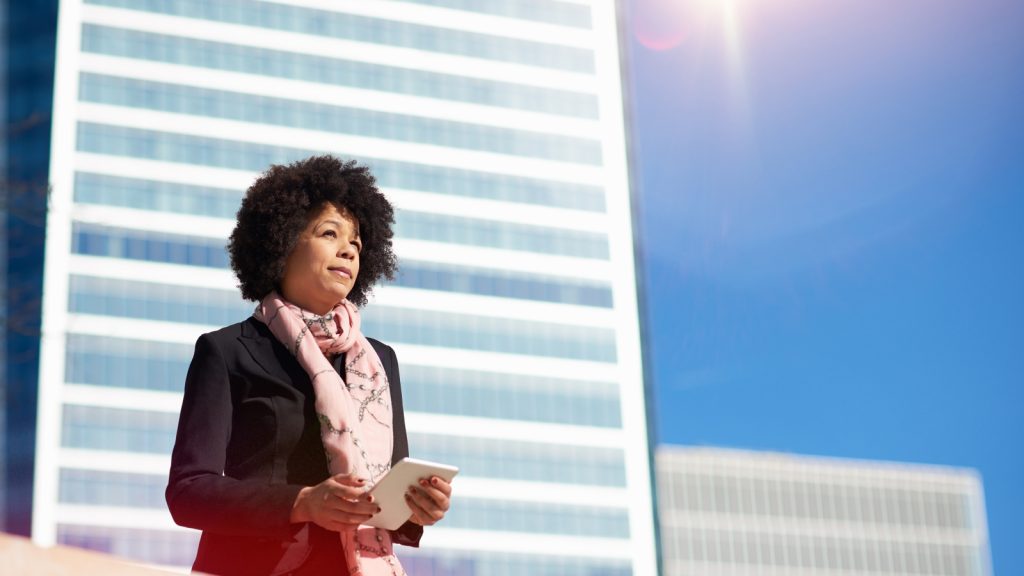
EU regulatory framework on the establishment of the digital euro: from investigation to realisation
With the approaching end of the investigation phase of the ECB’s digital euro project in October 2023, and the expectance of a decision on starting a realisation phase by the end of this year, the pros and cons of a potential digital euro have been widely discussed in the past months. The topic became even […]
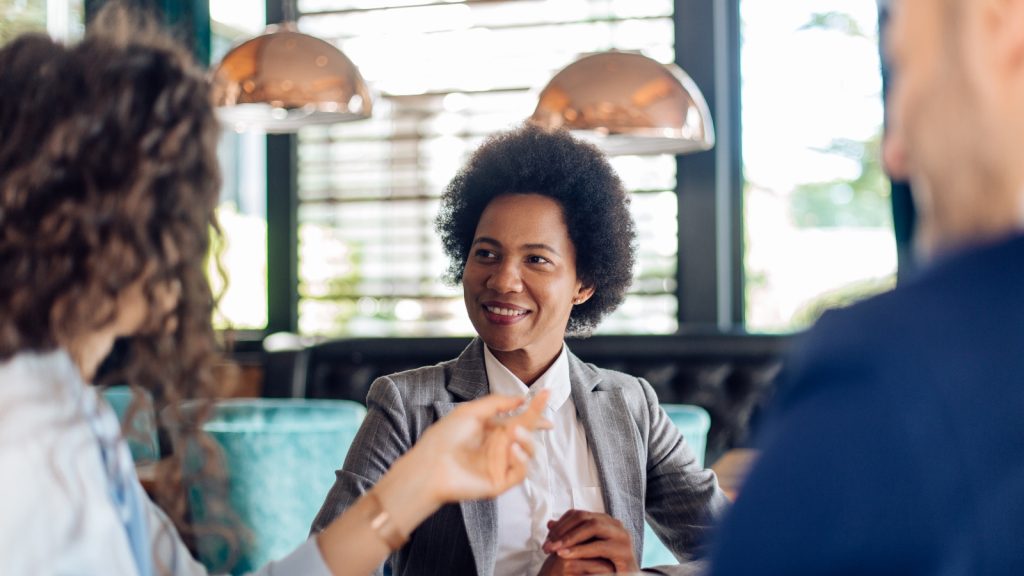
The EBA publishes new report and guidelines in response to risk within the financial services sector
New report on AML/CFT risks in payment institutions In accordance with the European Union regulations, the European Banking Authority (EBA) has been mandated to assess the management of the most significant risks in the fight against money laundering and terrorist financing (ML/FT). The entity’s analysis is centred around the identification and management of ML-FT risks […]
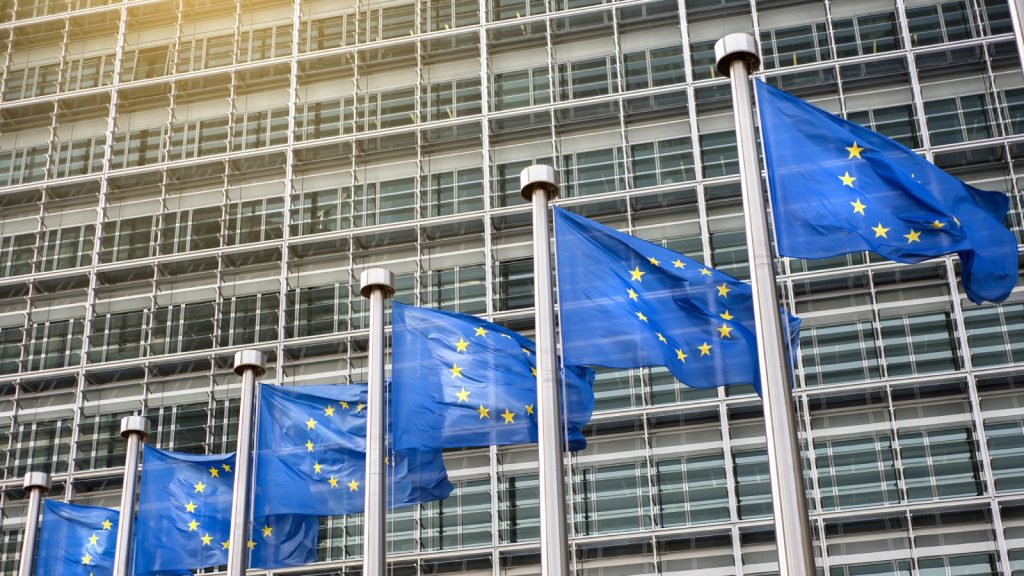
The European Parliament devises a new agreement to restrict access and abuse of financial services information
New measures to combat money laundering and terrorist financing The European Parliament has adopted a set of stringent measures to strengthen the fight against money laundering and terror financing, alongside circumventing sanctions within EU. These regulations are presented by EU in the form of a “legislative package” comprising three key measures which provide various practical […]
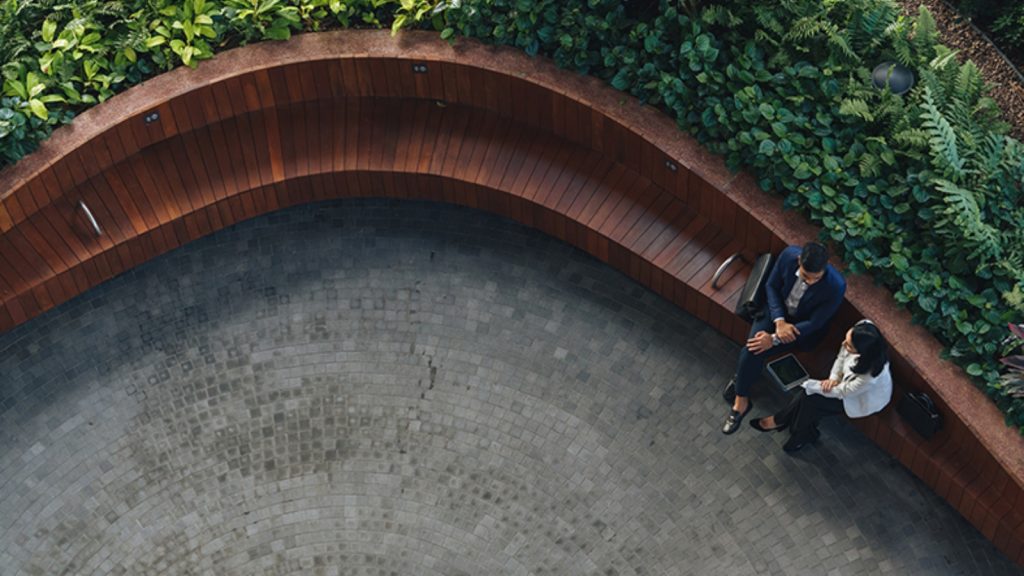
Asset managers and ESG implementation: turning regulatory and operational compliance into commercial opportunities
ESG-related regulatory requirements, and scrutiny, show no signs of abating. Asset managers have a pivotal role in financing the transition towards low-carbon economic systems. Hence, governments have introduced several ESG-related regulatory requirements that apply to asset managers. Some examples of these are the Sustainable Finance Disclosure Regulation (SFDR) in the EU and the mandatory disclosures […]
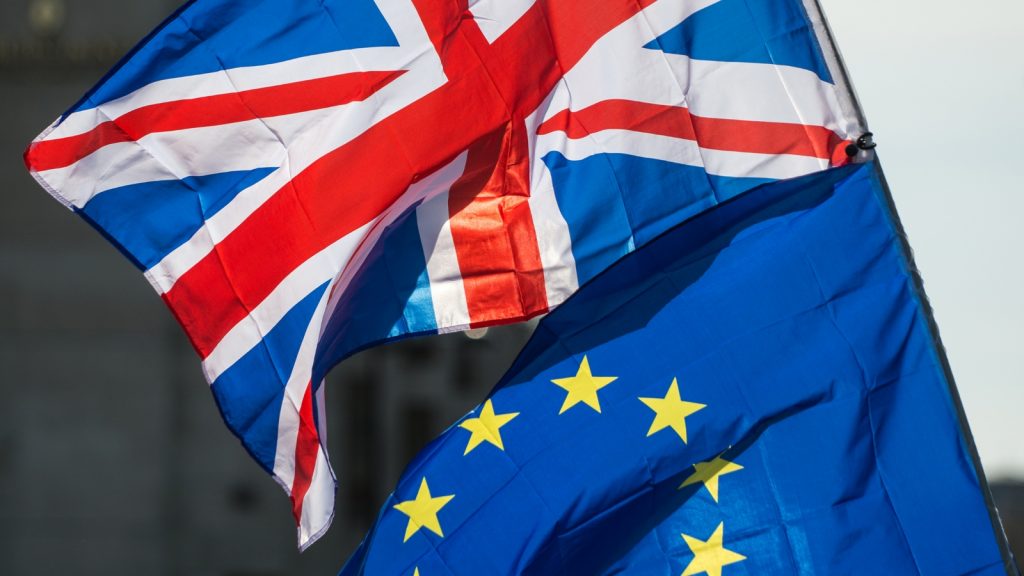
Basel reforms: similarities and divergences between the UK and EU
Both the UK and the EU are consulting on the next wave of iterations to the Basel reforms. Whilst the focus of these consultations has been relatively broad, the rules around the computation of Pillar one Credit Risk RWAs have seen the most significant change. This article outlines some of the main differences between the […]
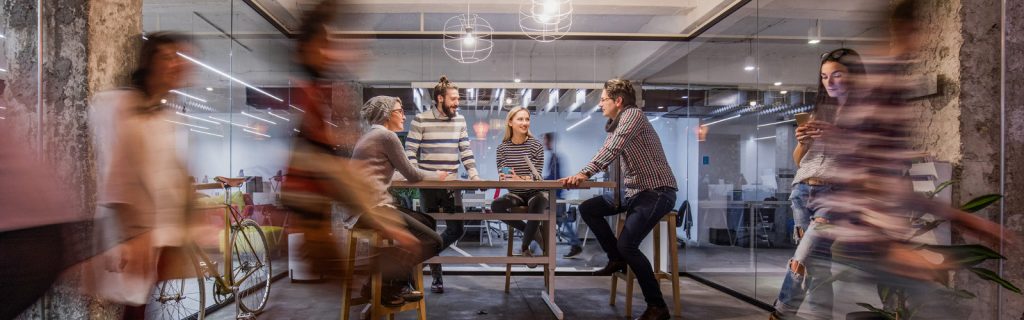
European Commission to strengthen regulatory framework for bank crisis management
The European Commission published on 18 April 2023 a new legislative package aiming to adapt and strengthen the framework for crisis management and deposit insurance (CMDI), with an acute focus on small and medium-sized banks. This proposal, which follows the announcement of the Eurogroup finance ministers inviting the Commission and the European co-legislators to review […]
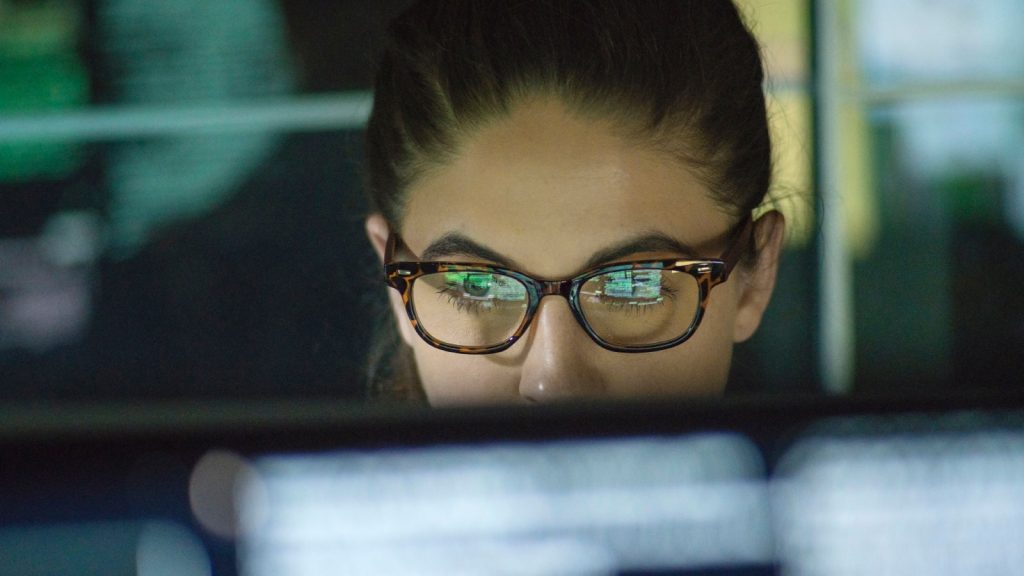
Greater insight into the AMF certification exam, the AMF-ACPR report and ROSA
On 16 December 2022, the AMF published additional information[1] on its website about the AMF exam, more commonly known as the “AMF certification” exam, since it certifies only the entities that organise the exam, rather than the people who pass it. The AMF lists the various functions within ISPs and FIAs that are covered by […]
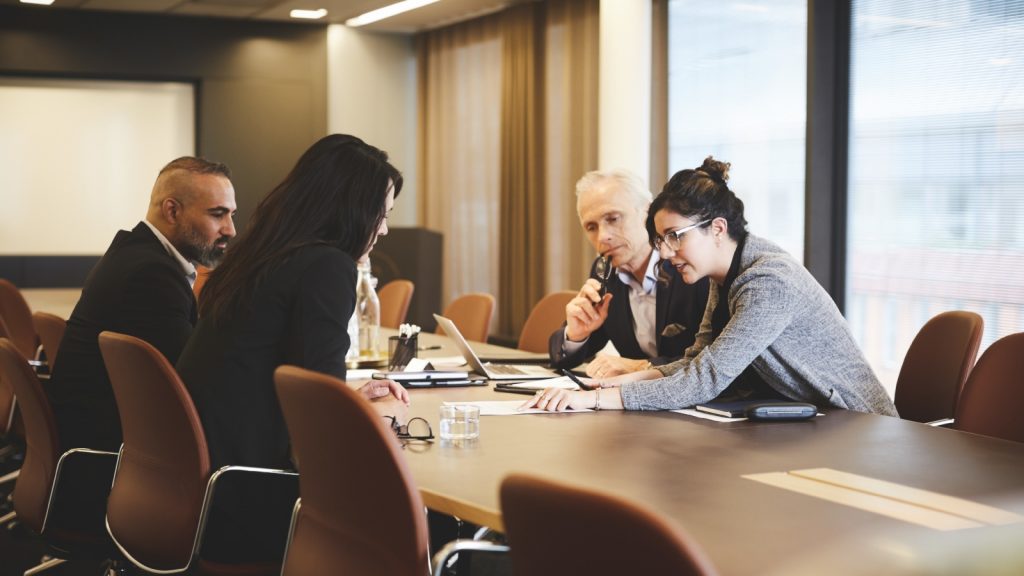
How does the 2023 Finance Act provide clarification on tax-favoured schemes and extend their validity?
The tax system applicable in the overseas public authorities includes a set of investment incentive schemes designed to promote their economic and social development. One factor to their successful implementation in practice is to provide as much visibility and sustainability for an investor as possible over time. Another key is to have a broad scope […]
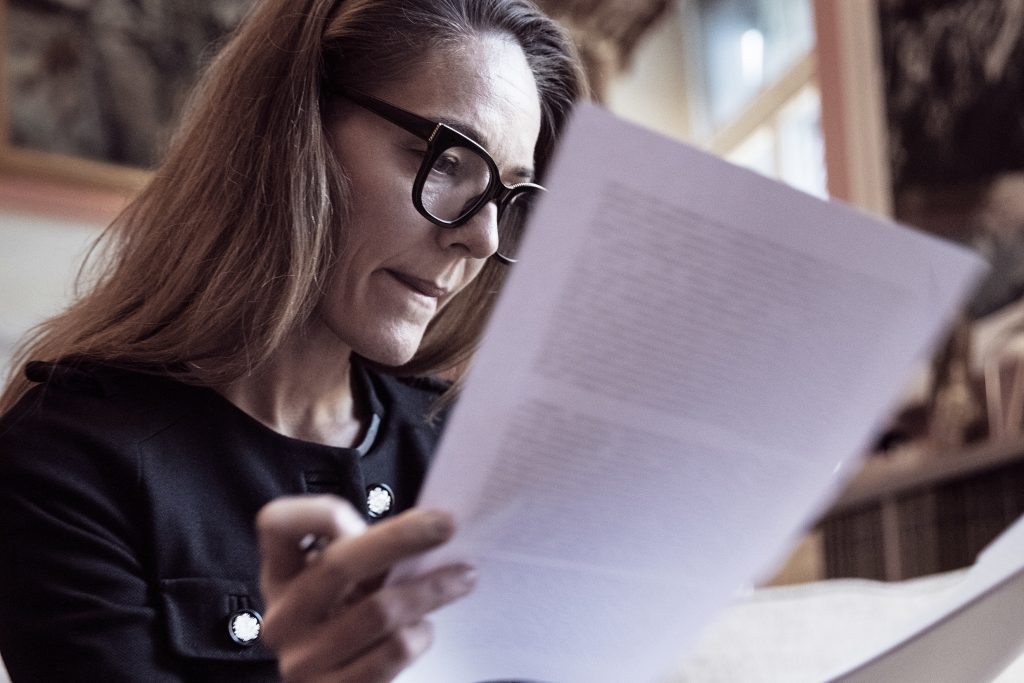
ESMA’s latest Q&A: which key topics are covered and how has it impacted the AFG?
The European Securities and Markets Authority (ESMA) published on 17 November, together with EBA and IOEPA, a Q&A on the RTS of the SFDR Delegated Regulation (Commission Delegated Regulation (EU) 2022/1288), just six weeks before coming into force. The Q&A clarifies a number of points, including those relating to principal adverse impacts (PAIs) and taxonomic […]
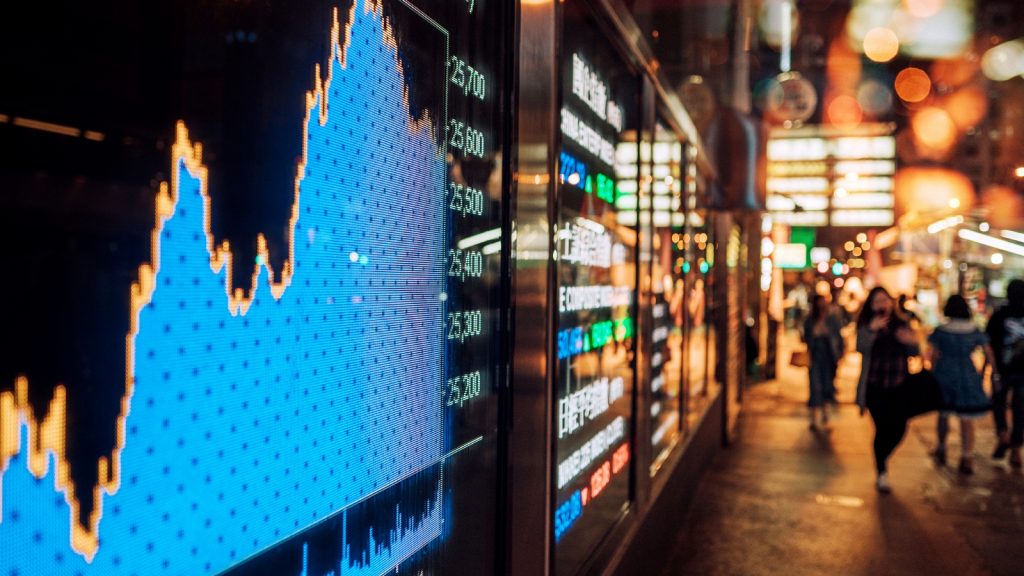
What lessons can be drawn from recent events in the banking sector?
In recent weeks, we have witnessed the successive bankruptcies of three banks in the United States, as well as the hasty takeover of Credit Suisse by UBS. This chain of events inevitably calls into question whether this trajectory echoes the leadup to the financial crisis of 2008. In reality, whilst these crises have stark similarities, […]
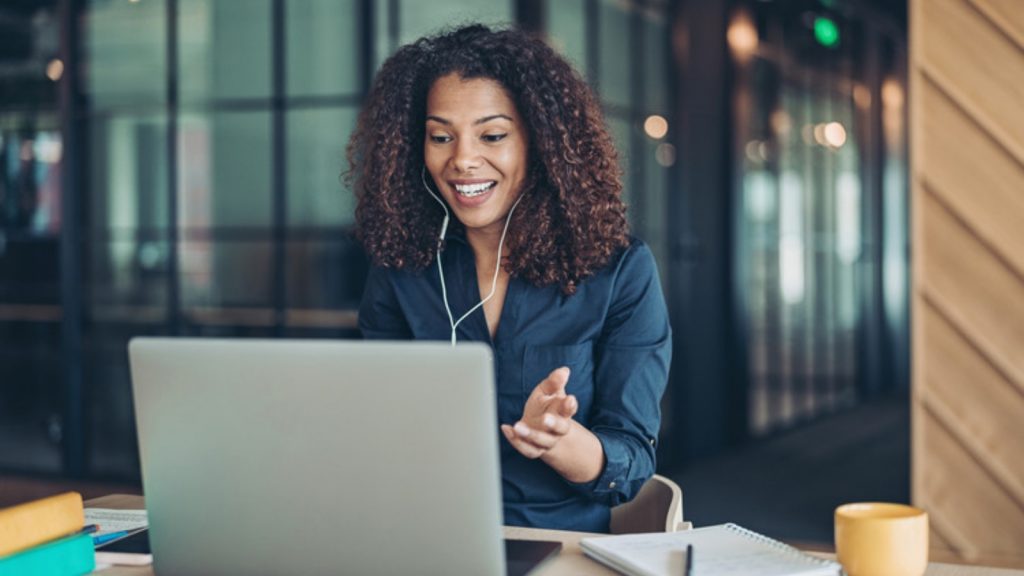
New DORA regulation: the challenge for insurers to strengthen their IT and cyber risk management
Since the onset of 2023, regulatory news has been adorned with the latest European legislation, under the acronym DORA, adopted on 10 November 2022 by the European Parliament. Standing for the Digital Operational Resilience Act, it will apply to the members of the European Union from 2025, and concerns companies in the financial sector specifically. […]
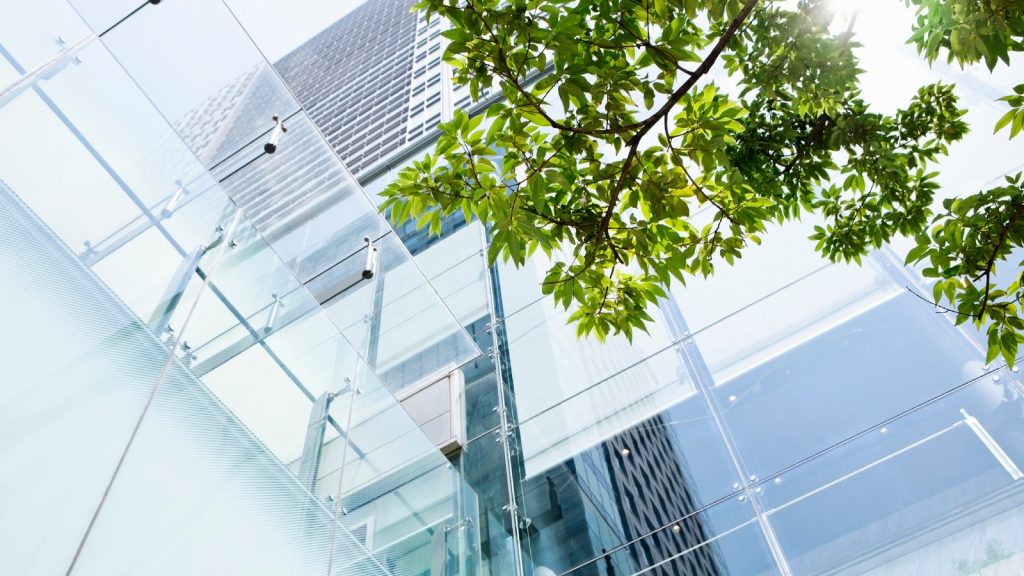
Sustainable finance series: Why does sustainable finance matter?
The momentum towards a low-carbon economic system is only set to grow. Financial services firms are pivotal actors in the transition; consequently, increasing demands are being put on them to demonstrate their sustainable finance activities and credentials. This blog explains what sustainable finance is and why it matters to financial services firms. What is Sustainable […]
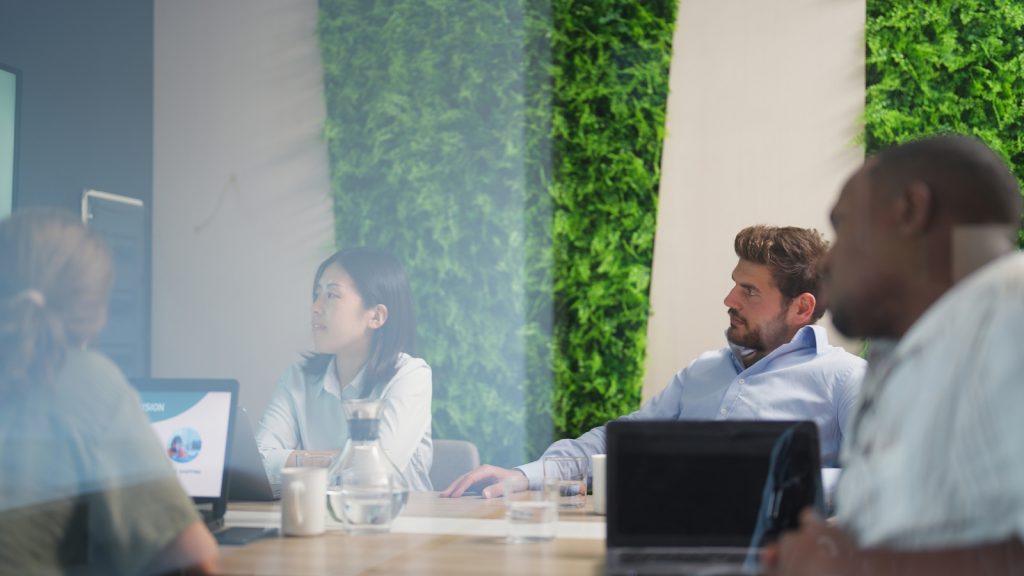
Sustainable finance series: Driving credible ESG actions
Implementing credible environmental, social and governance (ESG) actions requires successful enablers. So how can firms identify these enablers and, crucially, remove barriers to implementation? If we take our latest C-Suite Sustainability Barometer, we can see that out of the over 1,100 businesses accounted for in the survey, 75% are planning to increase their investment in […]
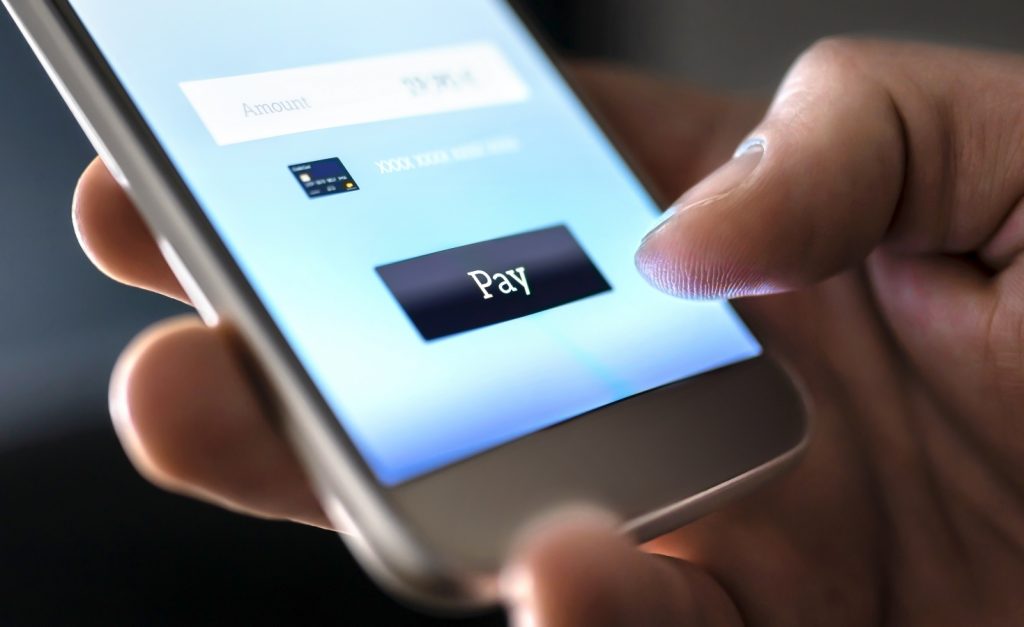
The digital euro as we know it today
“I see digital as the future of finance”. These are the words of the Executive Vice President of the European Commission (EC), Valdis Dombrovskis, voiced in the summer of 2020. He has undoubtedly been proven right as governments and central banks around the world have heightened their efforts to keep oversight of the digital transition […]
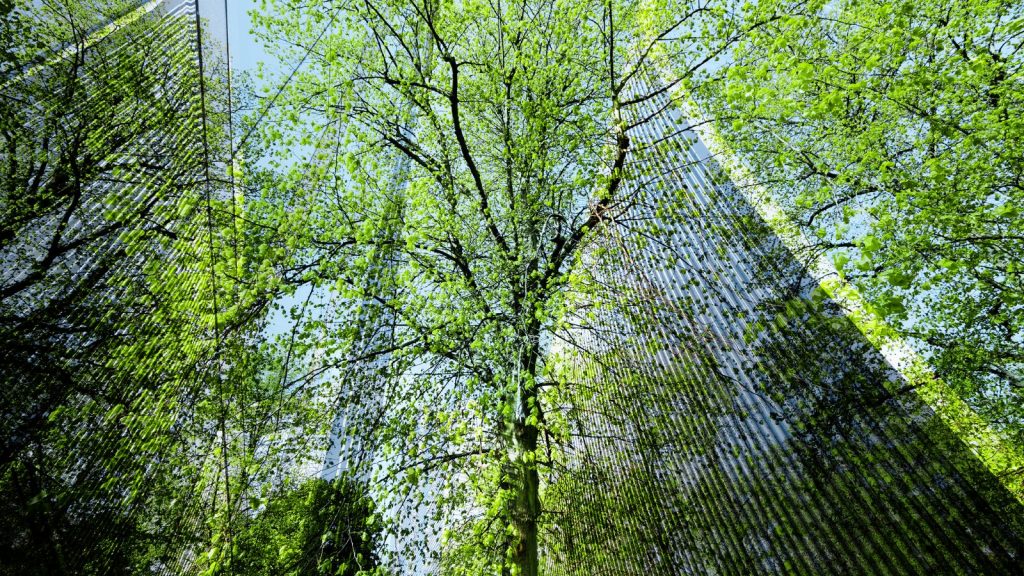
Climate change valuation adjustment: introducing a climate change scenario extrapolation to long dated CDS curve
The global climate crisis has triggered the financial sphere to address the way in which it conducts business. Climate risk consideration is currently growing in the banking industry but should also be considered by banks in the Credit Valuation Adjustment (CVA) when pricing derivatives. The credit risk for long dated derivatives (beyond 10 years), reflected […]
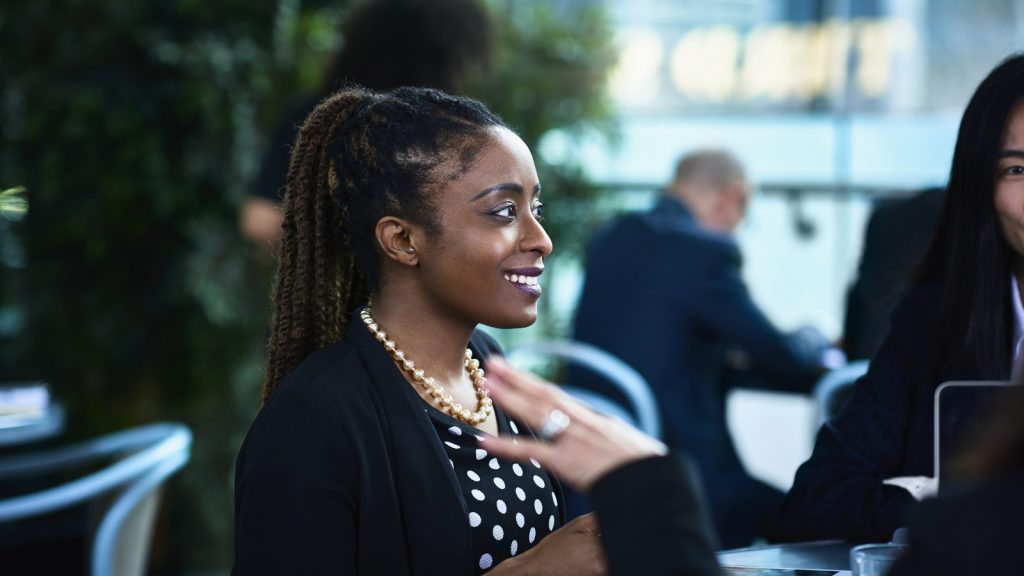
The Fed shares instructions on its first pilot climate scenario analysis exercise
The Federal Reserve Board (Fed) has shared instructions on its pilot climate scenario analysis exercise (CSA). Six of the largest U.S. banks, i.e., Bank of America, Citigroup, Goldman Sachs, JPMorgan Chase, Morgan Stanley, and Wells Fargo are participating in the exercise and are requested to submit their results along with documentation by July 31, 2023. […]
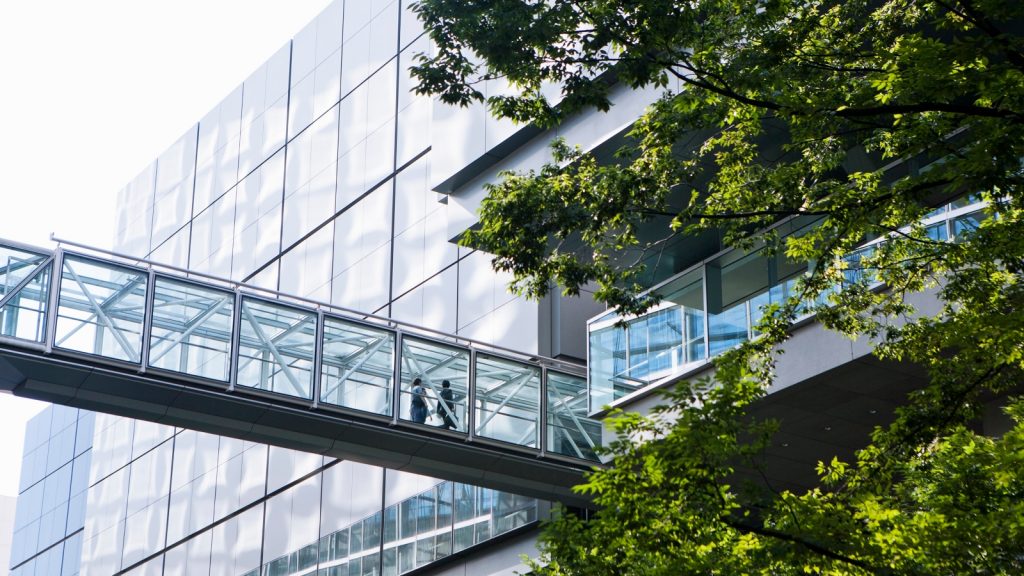
Why ESG-linked features impact financial assets classification under IFRS?
In our last article on sustainability-linked financing, we highlighted the accounting issues related to these contracts that are currently being debated between stakeholders. The most critical issue is the classification of loans or bonds that reference the borrower or issuer’s environmental, social and governance (ESG) key performance indicators (KPIs) on the balance sheet of lenders […]
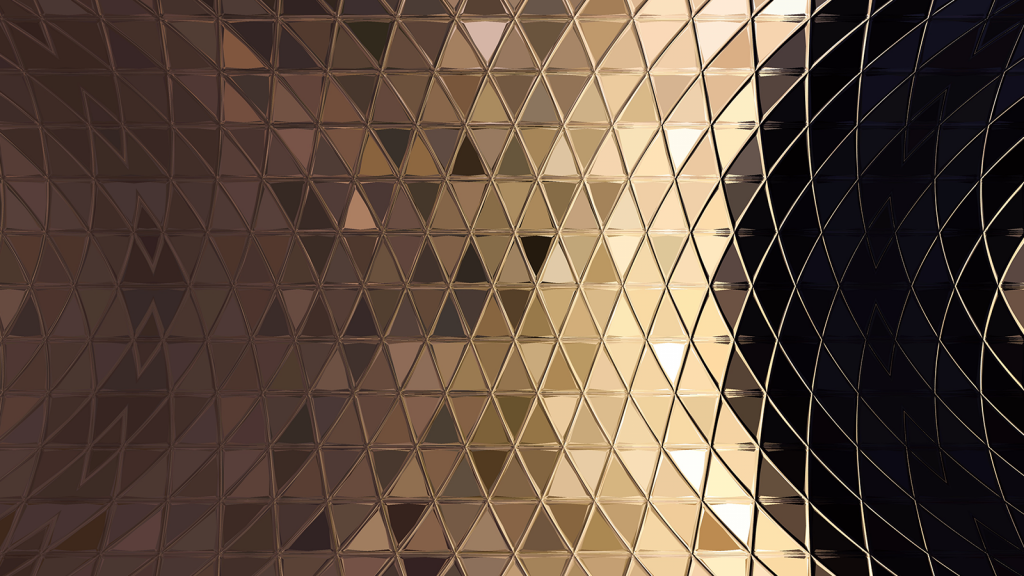
Spotlight on main European banks’ credit risk
After two years marked by the Covid-19 crisis, the first half of 2022 offered the prospect of a return to a certain economic normality. However, the outbreak of war in Ukraine combined with a deteriorating economic environment have reshuffled the cards and once again brought banks into a zone of turbulence and uncertainty. So how […]
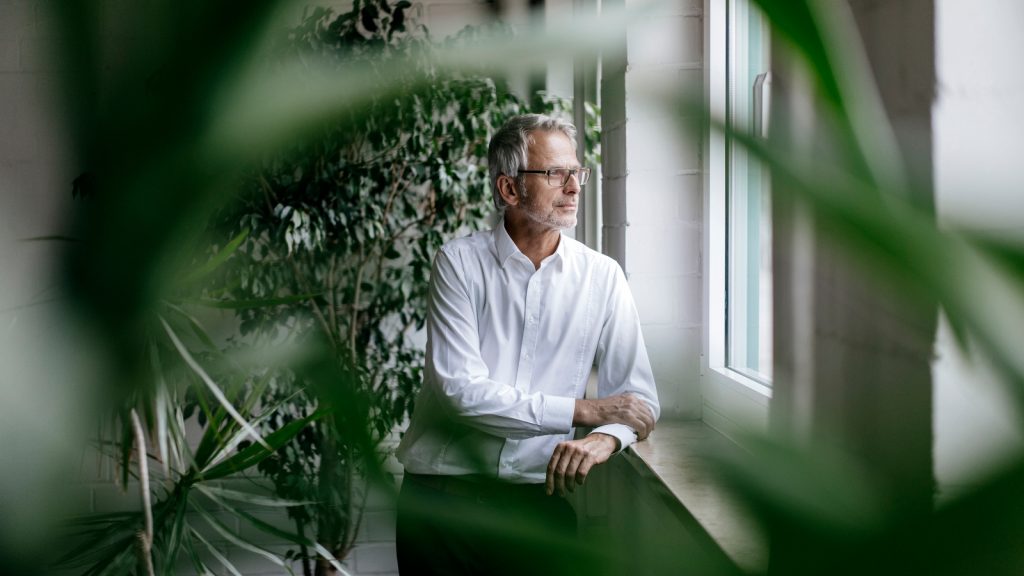
COP27: stepping up implementation and the role of finance
A lot of attention at COP27 was focused on the likelihood of keeping global warming to 1.5 °C, in line with the goals of the 2015 Paris Climate Agreement. The consensus was that we are in real danger of falling off track. Few nations revised their nationally determined contributions to reduce their carbon footprint compared […]
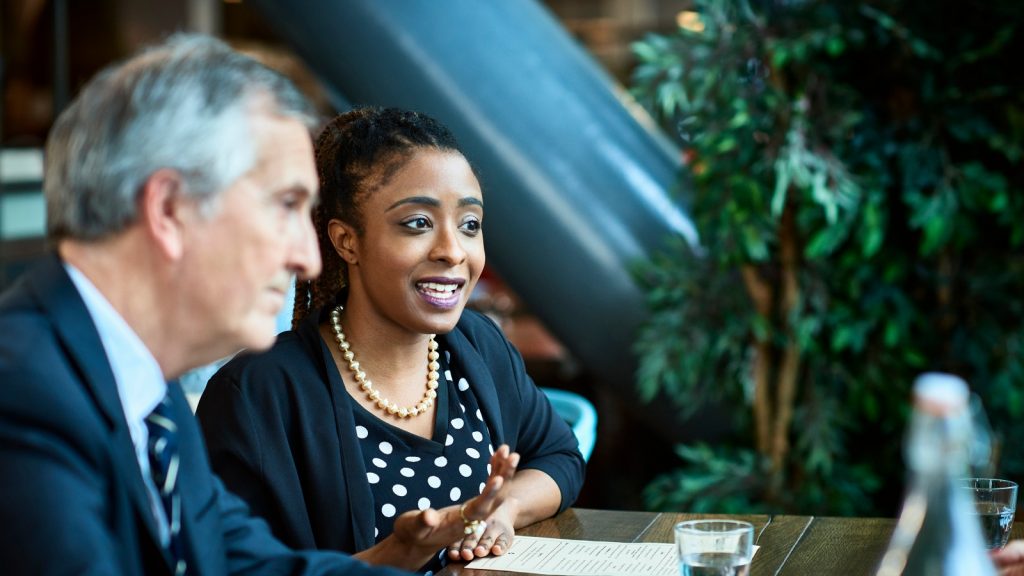
Results of the ECB 2022 thematic review on climate-related and environmental risks
The European Central Bank (ECB) has expressed a significant supervisory concern surrounding more than half of supervised banks in terms of the progress made on fulfilling the expectations specified in the Guide on climate-related and environmental risks. The ECB recently concluded its 2022 thematic review of the banking sector’s alignment with supervisory expectations. This review […]
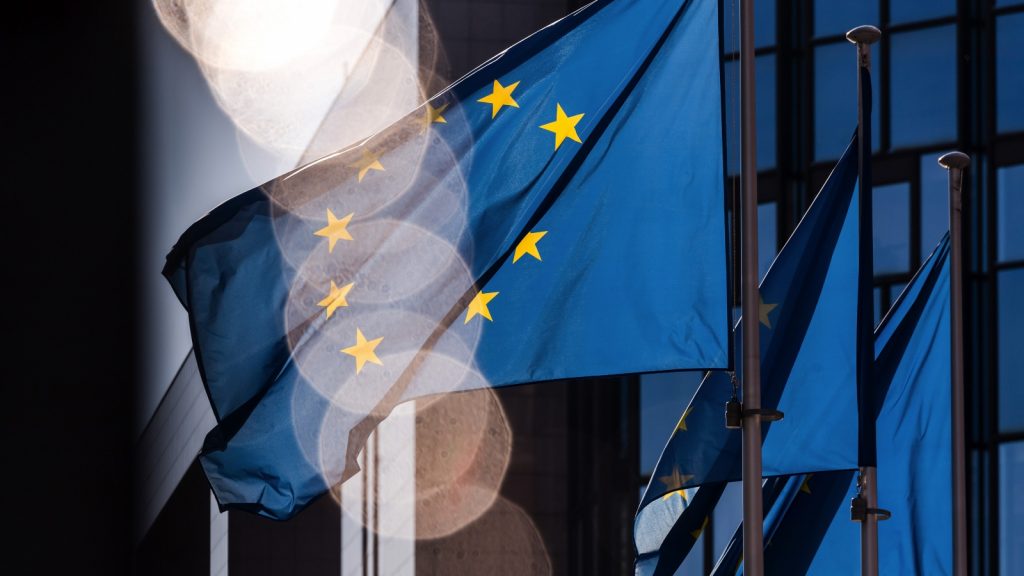
EBA considers bottom-up stress testing with top-down elements
The European Banking Authority (EBA) is tasked, in cooperation with the European Systematic Risk Board (ESRB), to initiate and coordinate biennial EU-wide stress testing exercises to assess the resilience of institutions to adverse market developments. The objective is to provide supervisors, banks, and other market participants with a common analytical framework to consistently compare and […]
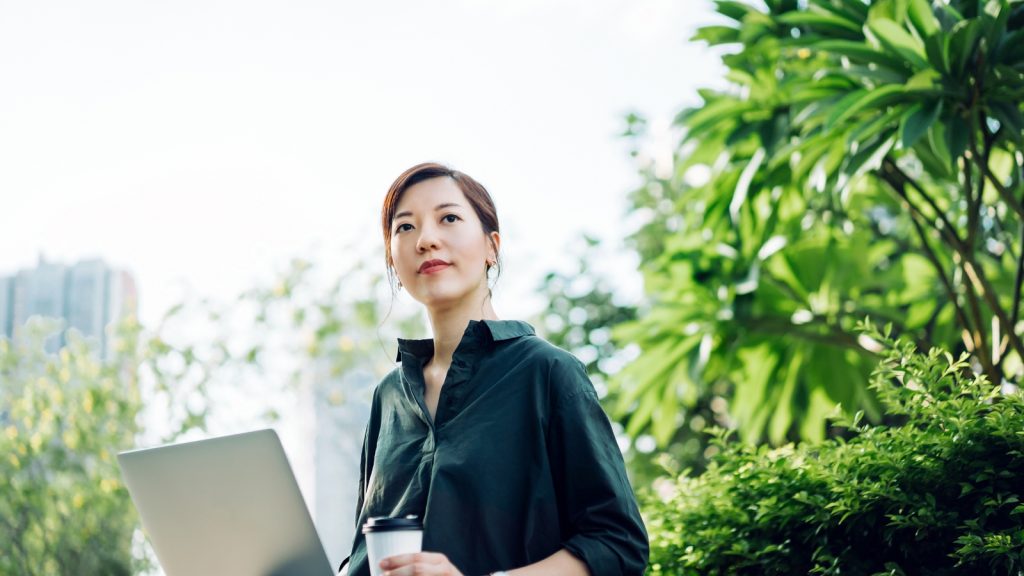
IFRS series on sustainability-linked financing
As environmental, social and governance concerns are becoming more and more prevalent, sustainable finance is now under the spotlight. The financial sector has a key role to play in achieving the ESG transition. One of the levies developed by the financial industry is to propose new kinds of financing that promote ESG practices and projects […]
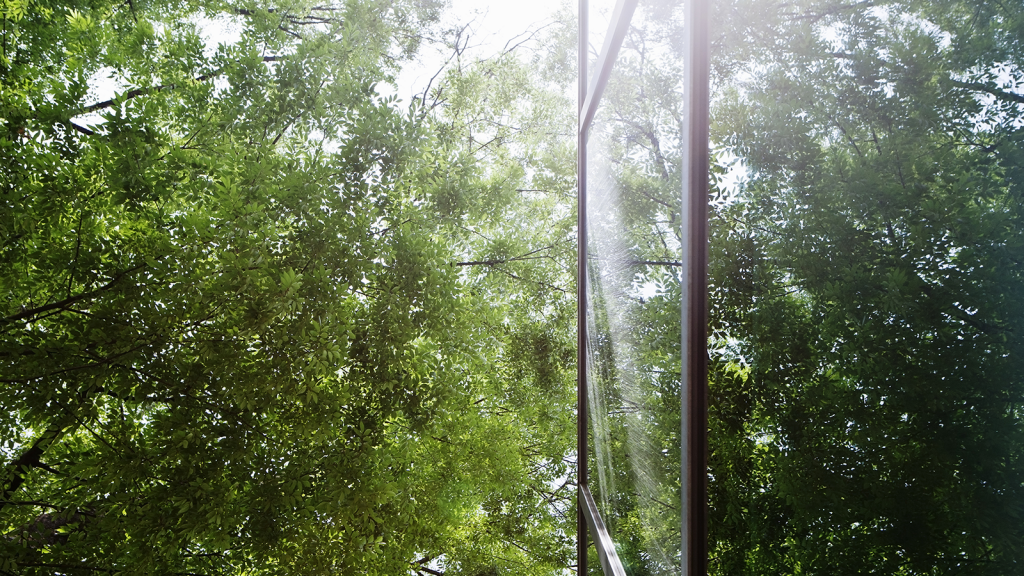
Green taxonomy trends facing the real estate sector
The European Union (EU) has taken the first step in directing capital investments toward so-called sustainable activities with the introduction of the Green Taxonomy on 1 January 2022. For the real estate sector, the objective is to measure the share of eligible activities contributing to the first two climate objectives – the mitigation of climate […]
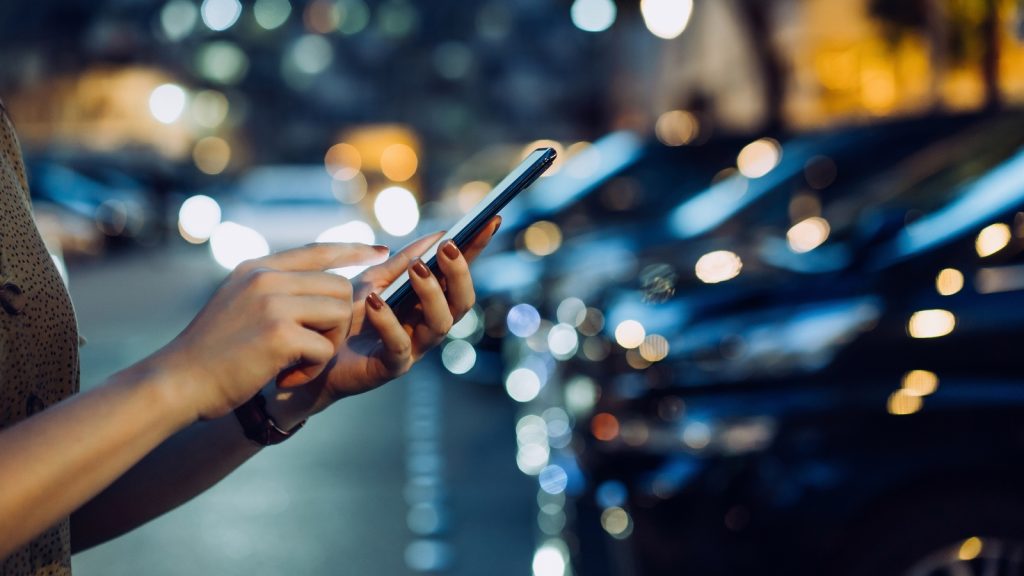
Can BIS develop a cryptoasset regulatory framework without limiting the innovation process?
In summer 2022, the Bank for International Settlements (BIS) published its second consultation paper on the prudential treatment of cryptoasset exposures. The guidelines outlined in the proposed document follow an initial discussion paper released in 2019 and a first consultative document issued in 2021. The complete text is set up as a new standard to […]
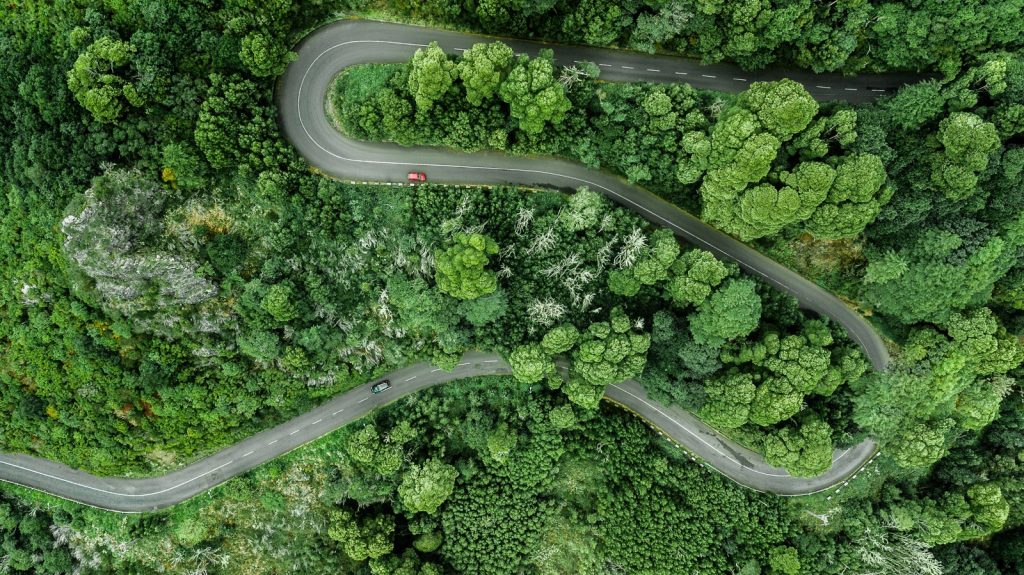
The FED announced a pilot climate scenario analysis exercise for early 2023
The Federal Reserve Board (FED) will commence its first bottom-up climate scenario analysis exercise at the beginning of 2023, as announced on 29 September. The exercise will be exploratory in nature and will not result in extra capital requirements. The list of designated participants consists of six of the largest U.S. banks, i.e., Bank of […]
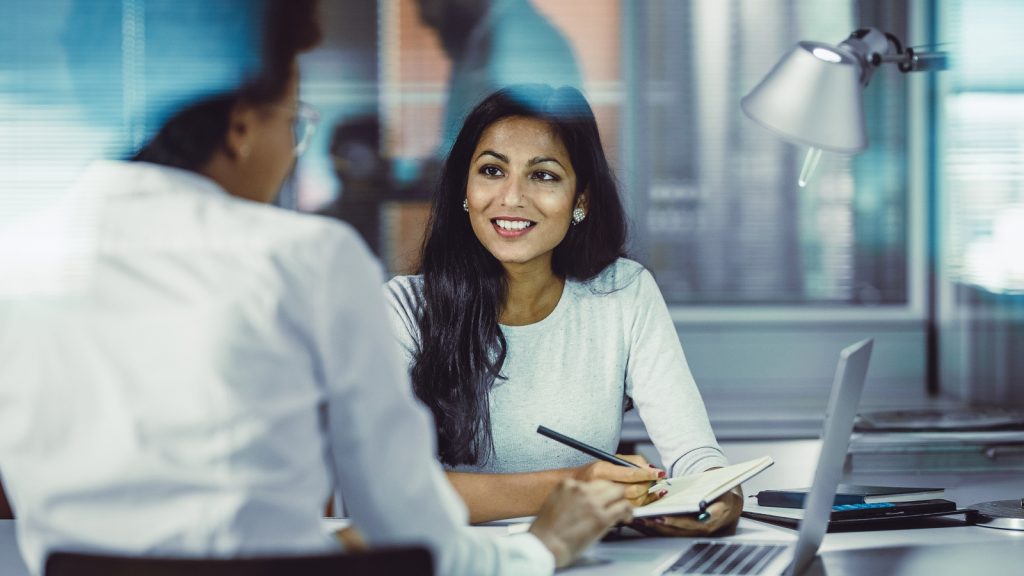
Reliable information key to the insurance sector’s ability to apply Green Taxonomy
The objective of the European Union’s Taxonomy regulation, in force since 1 January 2022, is twofold for the insurance and reinsurance sector. First, to measure the share of investments devoted to financing economic activities eligible for the taxonomy, known as the Investment Ratio. Second, to measure the share of gross premiums written in eligible non-life […]
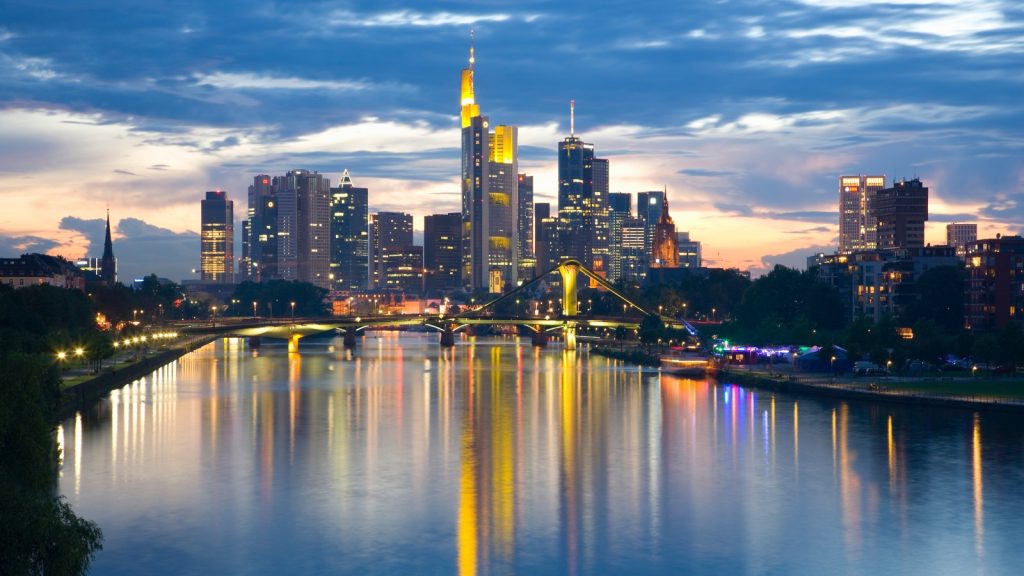
Banks grapple with GAR objectives
In force since 1 January 2022, the European Union’s Taxonomy regulation aims to support the market for green finance. More specifically, greater transparency in the market will help prevent greenwashing by providing information to investors about the environmental performance of assets and economic activities of financial and non-financial information. For the banking sector, the target […]
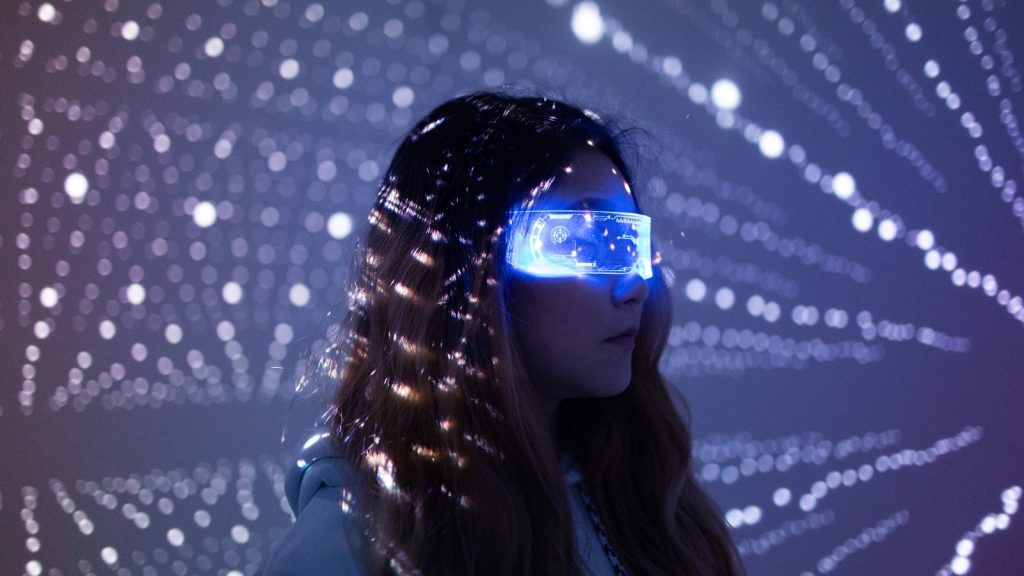
New pilot scheme opens pathway for blockchain technology
A new regulation introducing a pilot scheme based on blockchain technology is set to come into force on 23 March 2023. The new European regulation1 is an experiment to develop secondary markets for financial securities based on distributed ledger technology (DLT). Authorised participants in the scheme will be able to provide trading services and settlement-delivery […]
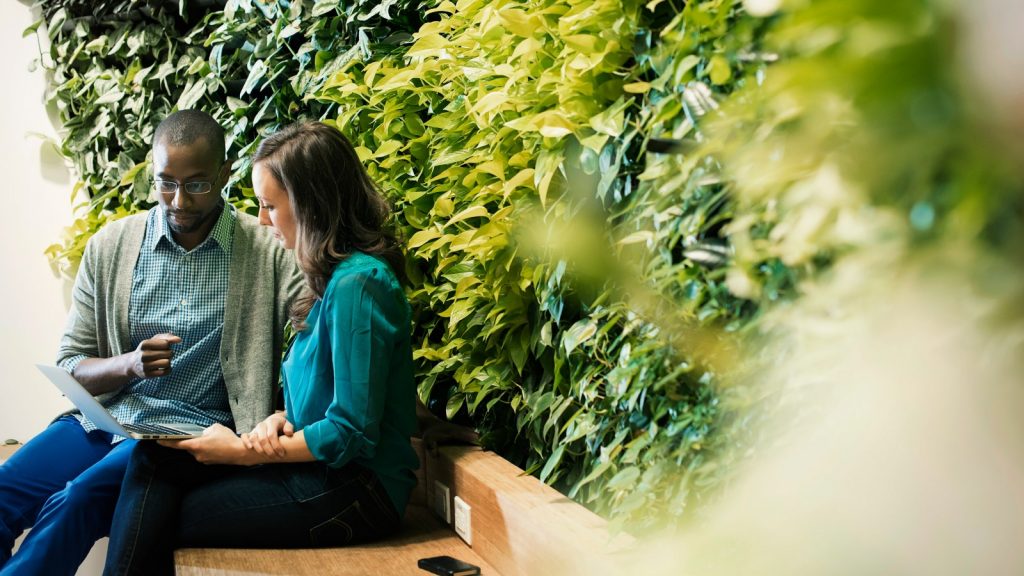
Results of the ECB 2022 climate risk stress test
The first supervisory climate risk stress test (2022 CST) conducted by the European Central Bank (ECB) has concluded with official results and findings made public on 8 July 2022. The exercise has complemented the broader ECB’s agenda to assess the readiness of banks in Europe to manage climate-related and environmental risks. The 2022 CST was […]
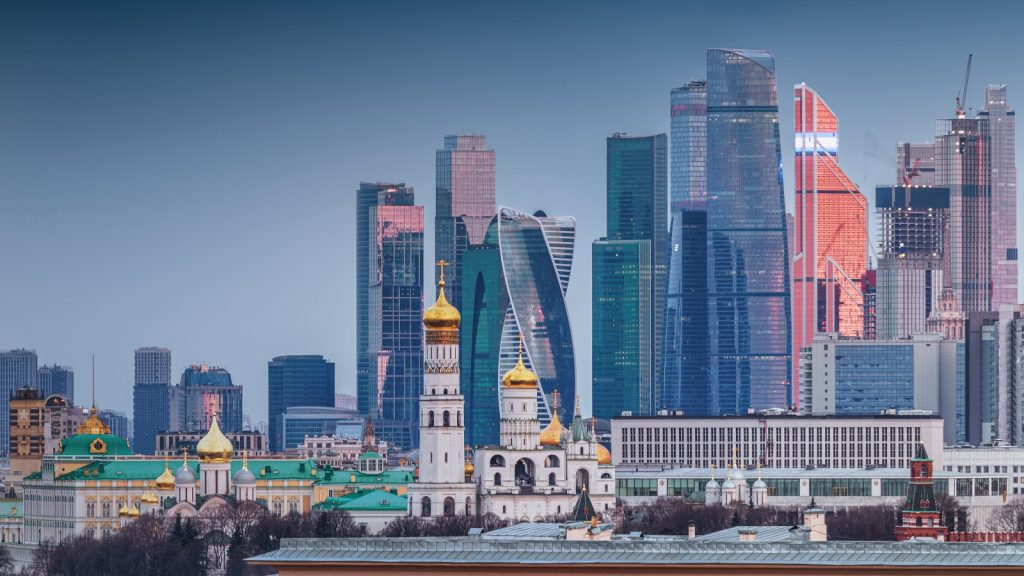
Russian sanctions: what implications for financial institutions?
Following Russia’s annexation of Crimea in March 2014, the United States (US) and the European Union (EU), together with other countries, imposed mainly economic sanctions on Russia. Since Russia’s recognition of the self-proclaimed autonomous republics of Donetsk and Lugansk, followed by Russia’s attack on Ukraine on 24 February 2022, these sanctions have taken on new […]
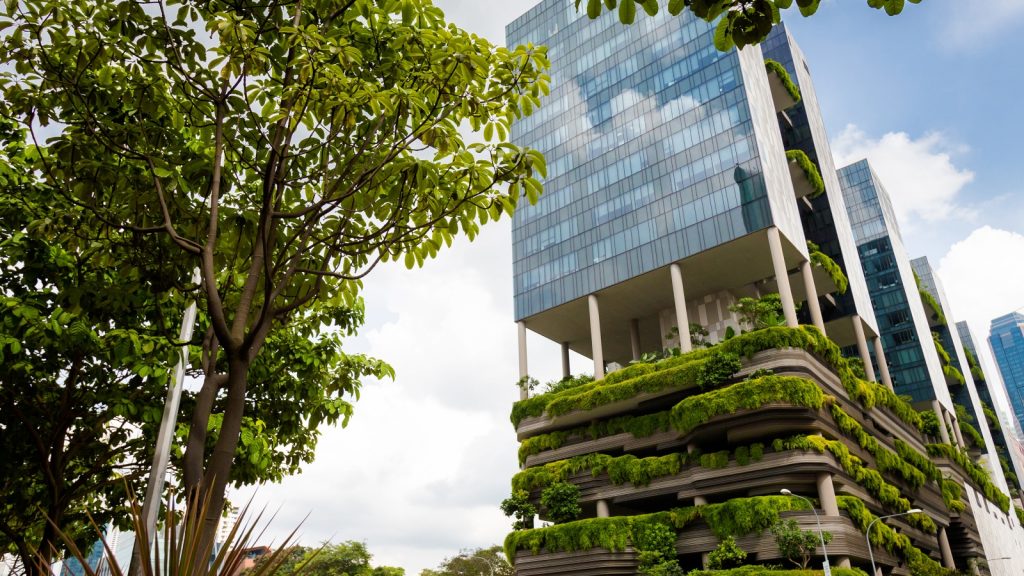
How to address climate risk in the banking prudential framework
Climate change is now firmly in the focus of prudential regulators and supervisors across the globe. Against this background, the European Banking Authority (EBA) is mandated to assess whether a dedicated prudential treatment of exposures related to assets or activities associated substantially with environmental and social objectives would be justified. Based on its findings, the […]
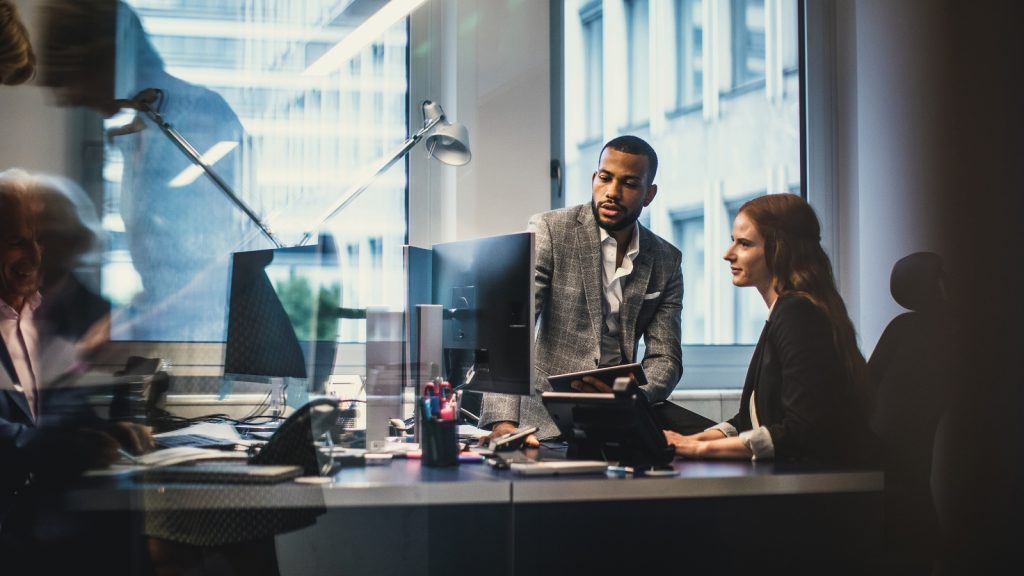
Quarterly SSM briefing: stable supervisory priorities and the ECB’s green agenda
The last few weeks have been marked by an ongoing review of the supervisory priorities initially listed by the Single Supervisory Mechanism (SSM) for 2022-24, and developments in the climate agenda outlined by the European Central Bank (ECB). ECB’s supervisory priorities for 2022-24 remain stable despite geopolitical instabilities and challenges At the beginning of 2022, […]
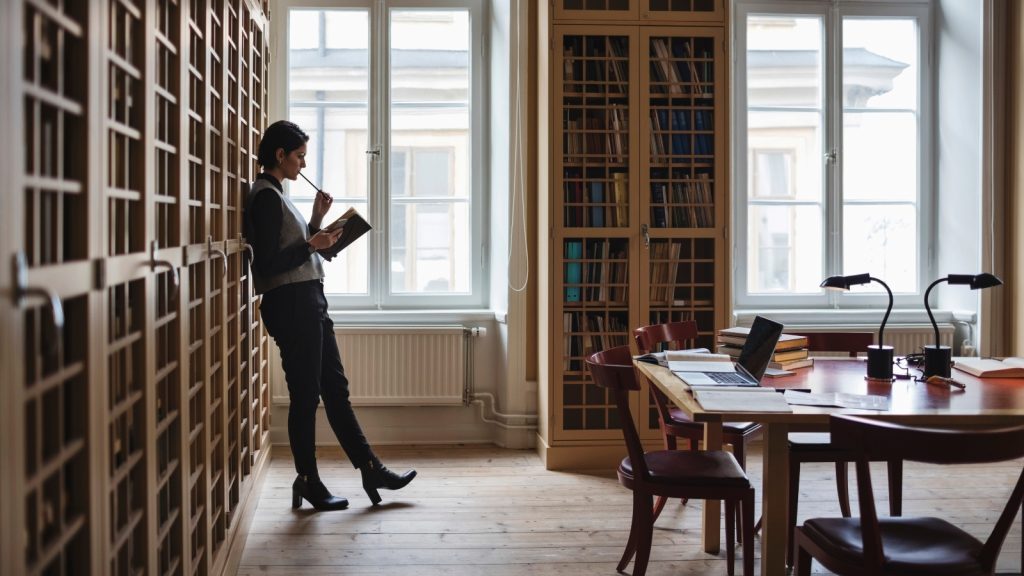
The return of inflation: what consequences for banks?
For several months now, we have been in an economic and financial environment that we have not seen for some years. In May, inflation in the Eurozone reached 8.1%, with six countries exceeding 10%, while the United States recorded an 8.6% year-on-year price increase. The short-term reasons for the return of inflation are well known, […]
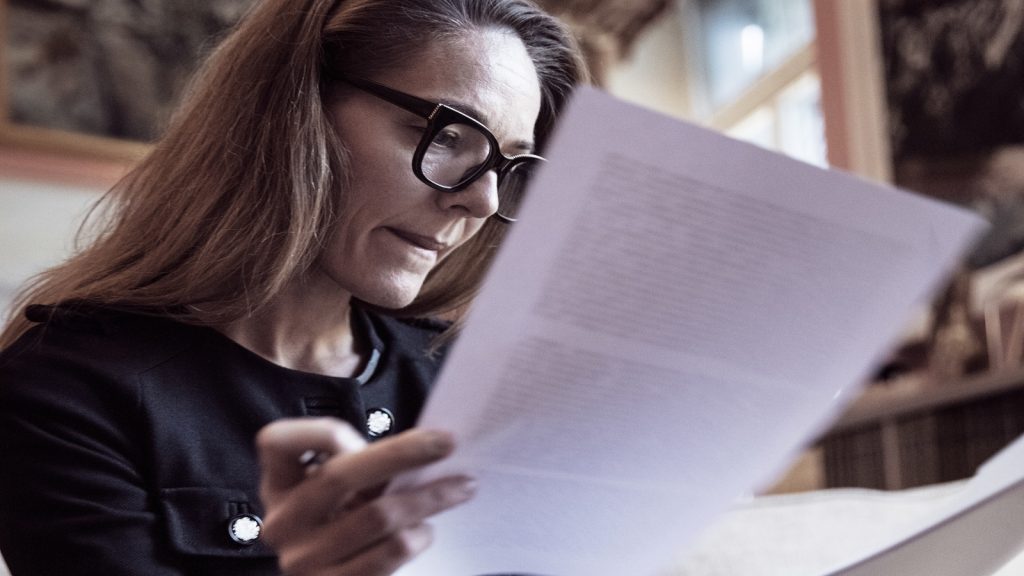
Real estate share deals: survey and assessment from a tax perspective
The years between 1848 and 1918 are considered the relevant building period for the Gründerzeit-Zinshaus buildings in Vienna. In this period Viennese housing construction underwent a decisive development which shaped the city’s characteristic flair to this day: the Zinshaus buildings – so typical for Vienna – came into existence. Viennese Gründerzeit-Zinshaus buildings have not lost […]
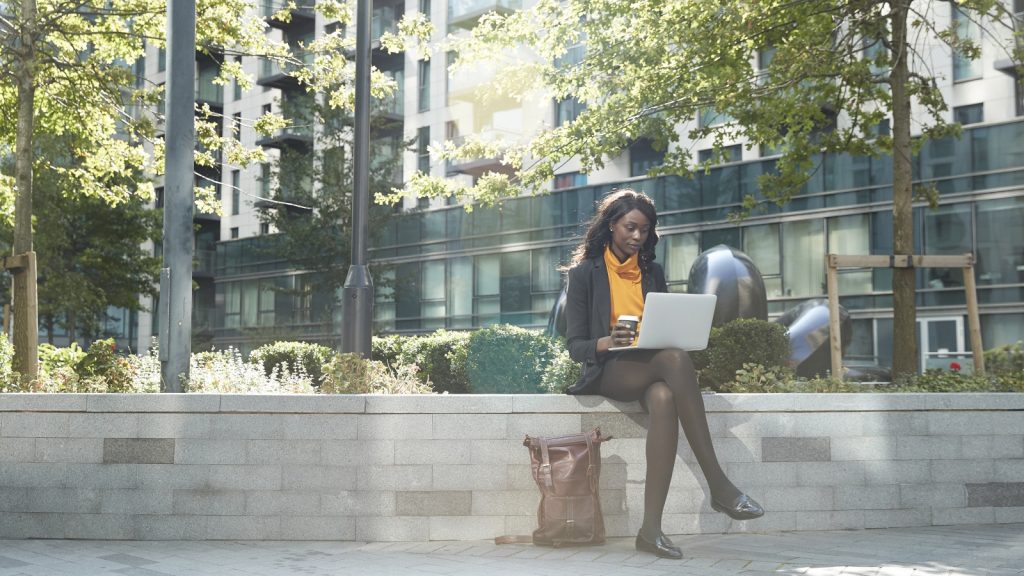
France steps up sustainable transformation with mission-led business law
France’s innovative and incentivising Action Plan for Business Growth and Transformation (PACTE) law lays the legal foundations for corporate social responsibility. With more than 400 companies established as “sociétés à mission” – mission-led businesses – by the end of 2021, this new scheme is an undeniable success. The number of mission-led companies has doubled in […]
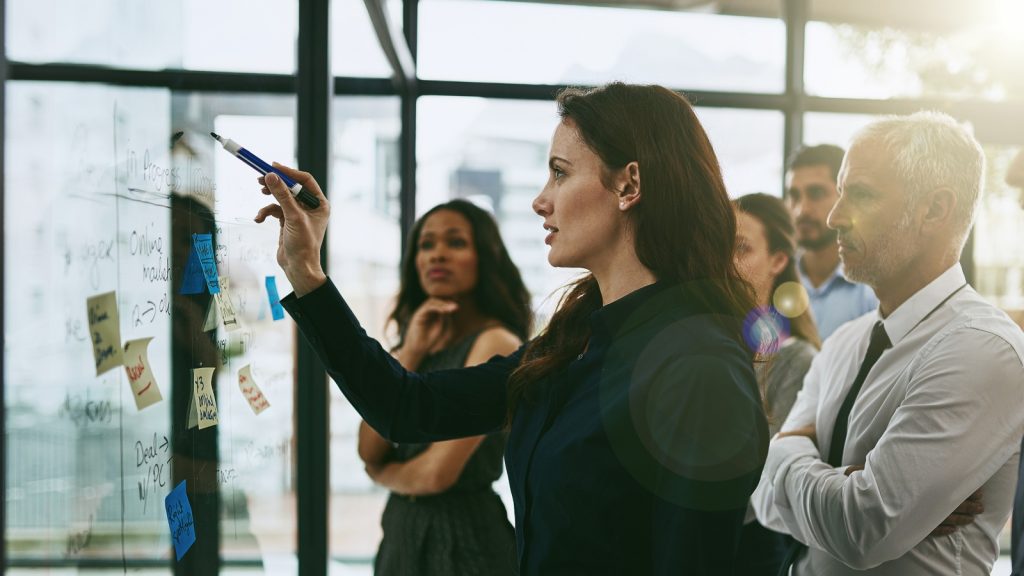
The digital euro: the future of central banking in Europe?
Central Bank Digital Currencies (CBDCs) continue to receive increasing attention not only from the ECB but all over the world. So far, 10 countries [1] have already deployed CBDC programmes with another 15 countries [2] currently conducting pilot programmes. In total, 105 countries are considering using CBDC programmes, representing over 95% of global GDP and […]
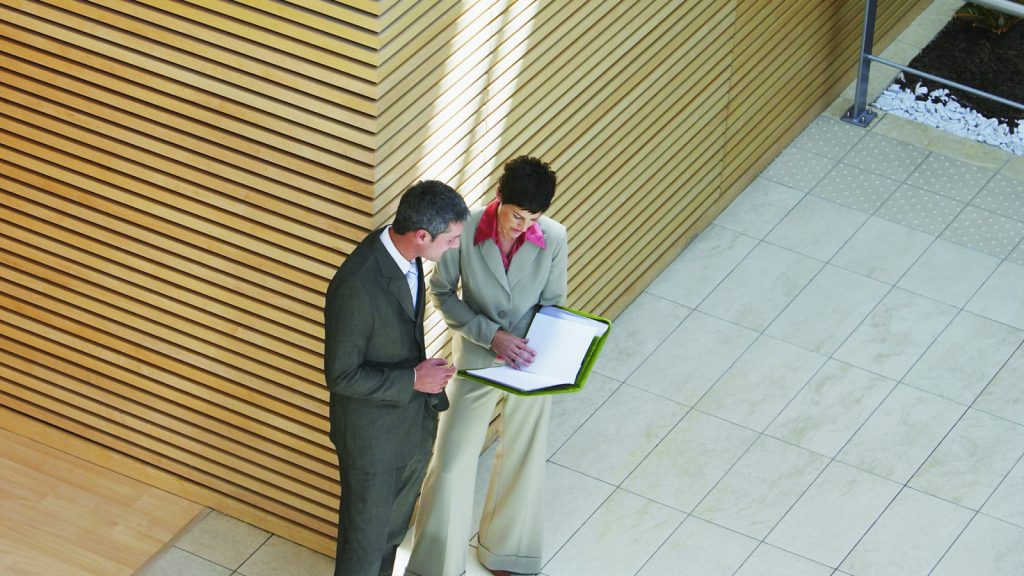
Leveraged transactions: supervisory expectations in the Eurozone
The chair of the European Central Bank’s Supervisory Board, Andrea Enria, has voiced several times in the past months the supervisor’s concern with the increasing growth of the leveraged finance sector, which deals with loans to highly indebted borrowers. By mid-2021, the combination of a strong global loan moratoria policy and the long-standing low interest […]
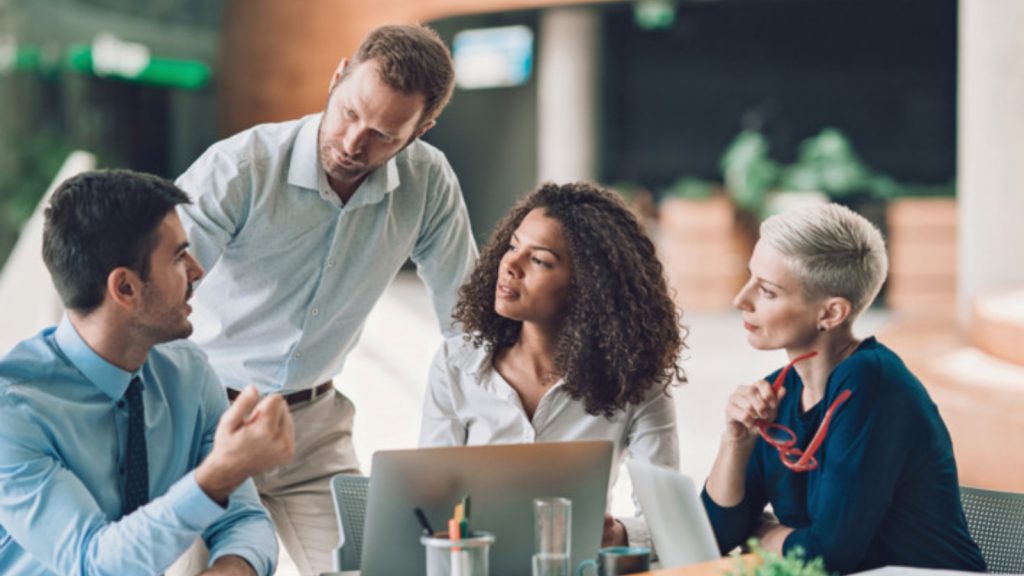
Impacts and consequences of the war in Ukraine for banks and insurance companies
The war in Ukraine, as well as the unprecedented sanctions imposed by the European Union, the United States and their partners against Russia have had major consequences for financial services institutions. For foreign companies operating in Russia or Ukraine, the first concern was the safety of their staff. They had to make difficult choices to […]
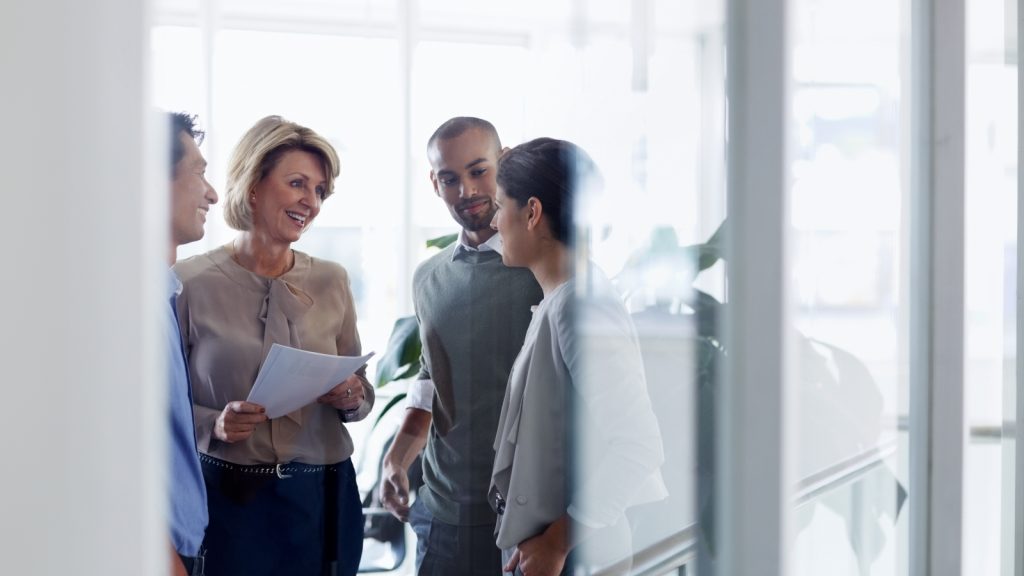
Solvency II Directive measures to aid European economic recovery
While the European Commission’s most recent opinion on the review of the Solvency II Directive is broadly in line with the final European Insurance and Occupational Pensions Authority (EIPOA) opinion issued in December 2020, some measures have now been amended. These amendments are designed to strengthen the capacity of European insurers to contribute to the […]
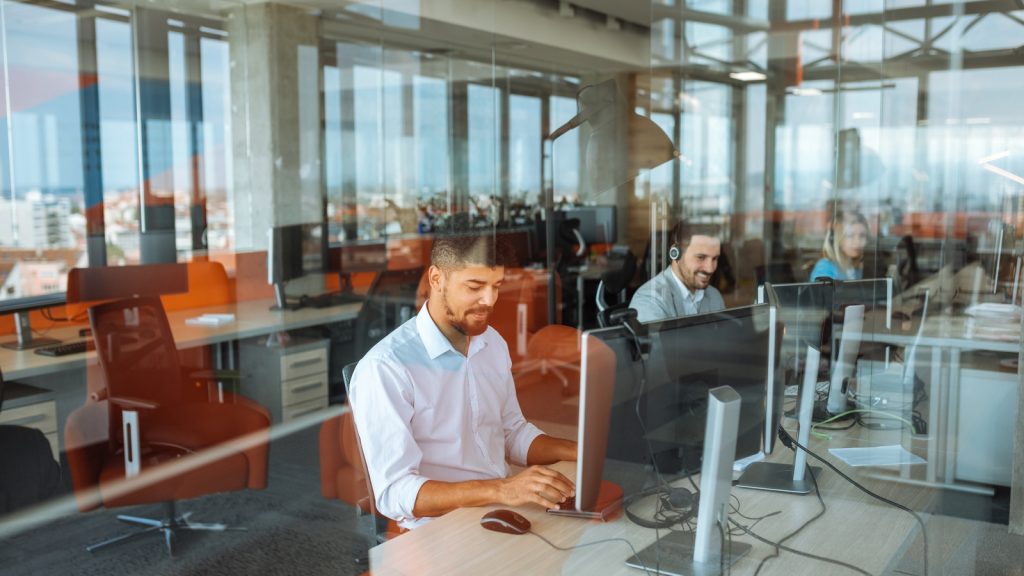
New-style cyber insurance policy models on the rise
Regardless of geography or business sector, many groups and companies have taken out cybersecurity insurance policies in recent years. These policies cover companies against new threats to information systems, including ransomware and data theft incidents that have been making the headlines. For a long time, the risks identified in these policies were on the borderline […]
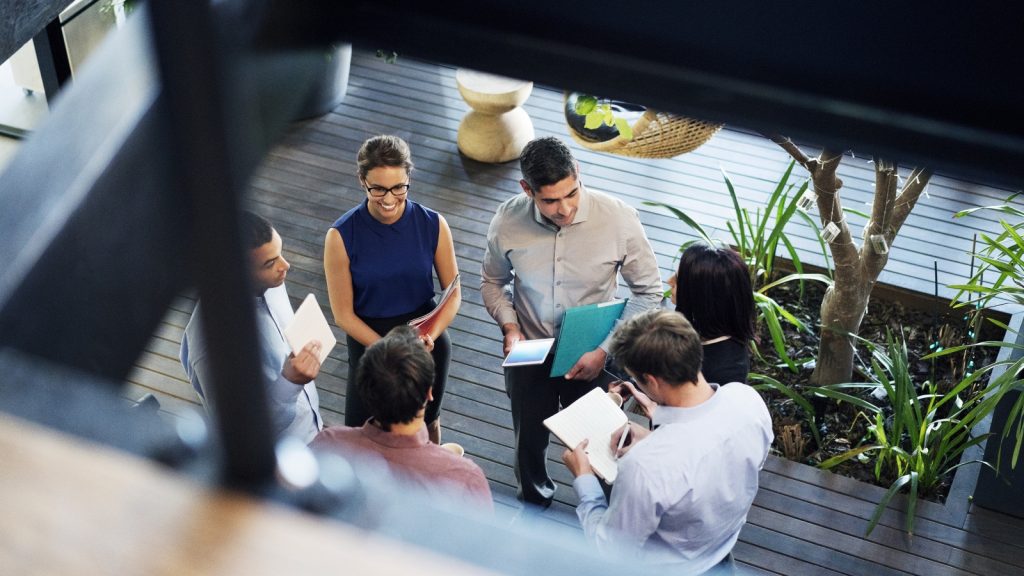
Sustainable finance regulations signal a sea change for insurance sector
The European Green Deal aims to achieve climate neutrality by 2050 and create a modern, competitive and resource-efficient economy. To meet its objectives, the European Commission has begun to restructure the non-financial reporting requirements for companies. Although some of the requirements were partially implemented in 2021, this is only the beginning of a real sea […]
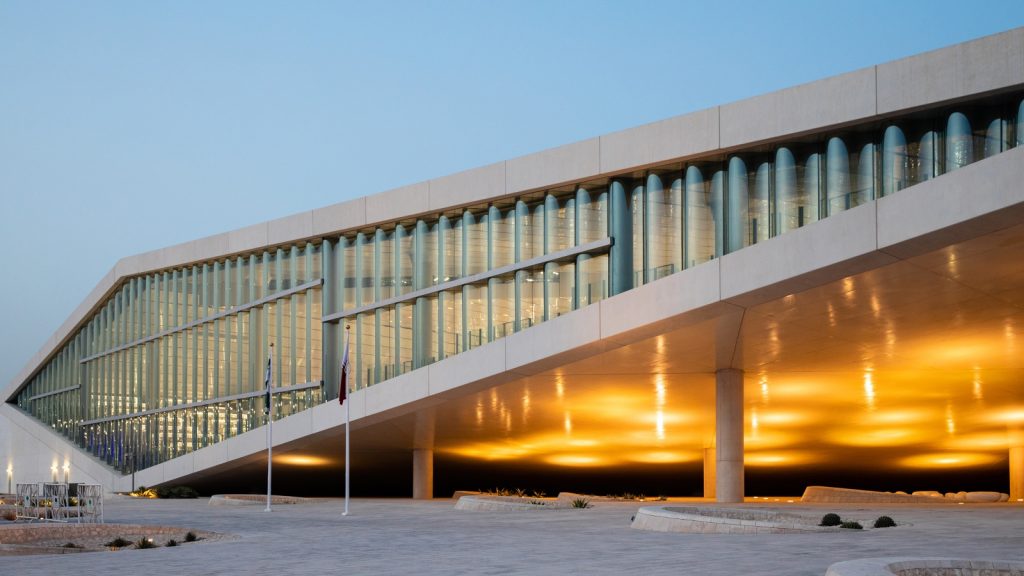
European crisis management framework: ripe for reform?
Since the European crisis management framework was established in 2014, there have not been many failing banks in Europe. However, the recent global health pandemic, combined with the ongoing conflict in Europe between Russia and Ukraine, could easily change this. The EU crisis management framework was established in response to the global financial crisis and […]
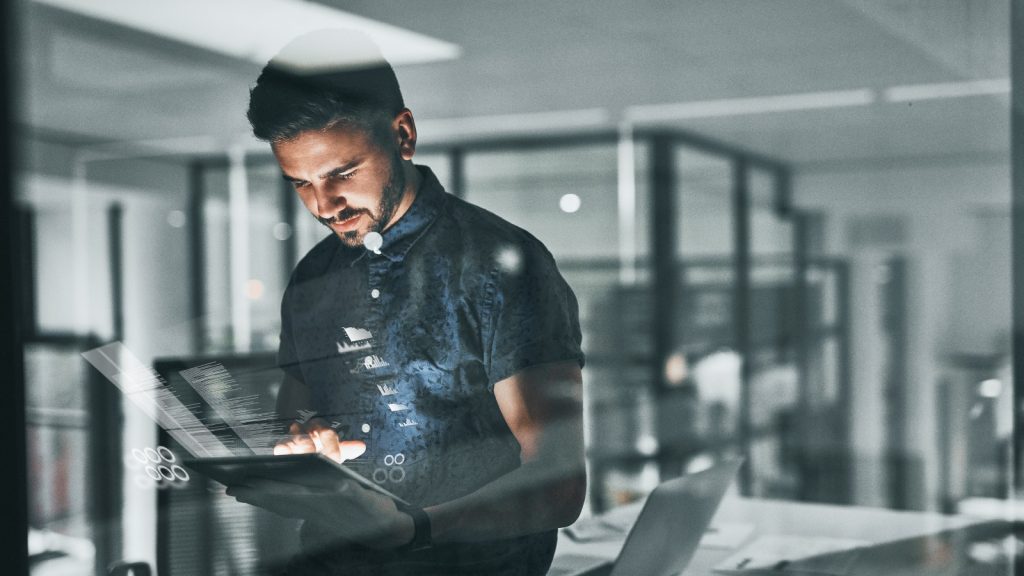
Can markets in crypto-assets (MiCA) give banks a regulatory edge?
Crypto-asset markets have been on banks’ radar for some time. While interest and involvement have varied, regulatory developments have been a driving force. In September 2020, the European Union (EU) published a proposal for the regulation of Markets in Crypto-assets (MiCA), offering a uniform legal framework for crypto-assets in the EU. On 14 March 2022, […]
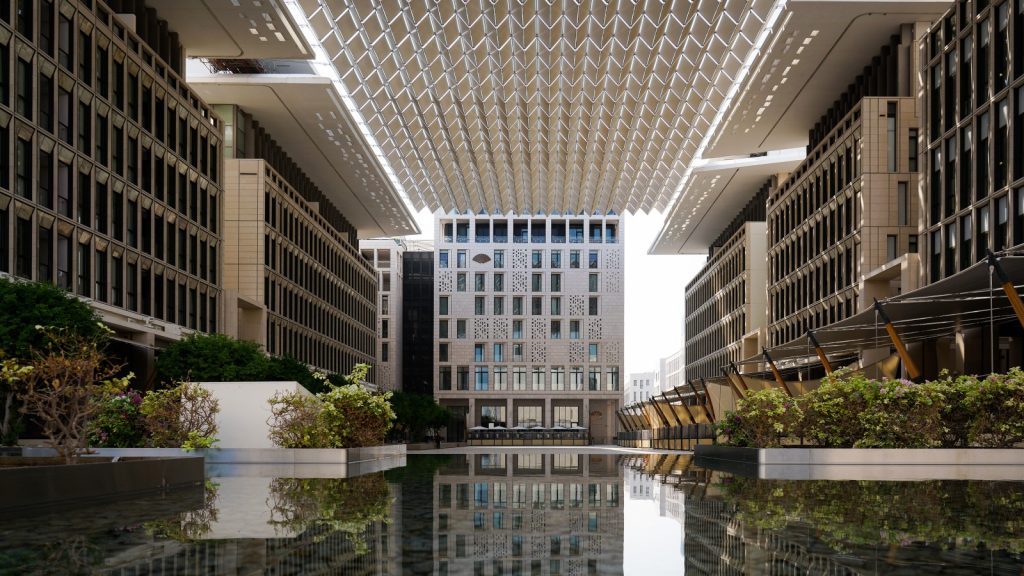
Eurofi financial summit addresses EU’s ecological and digital transition
As a setting for exchange between European Union (EU) economic and financial regulators and senior financial sector executives from the industry, one of the world’s largest financial services conferences, Eurofi, took place in Paris in February. Established in 2000, the Eurofi meetings occur bi-annually* alongside the Economic and Financial Affairs Council configuration (ECOFIN) meetings. The […]
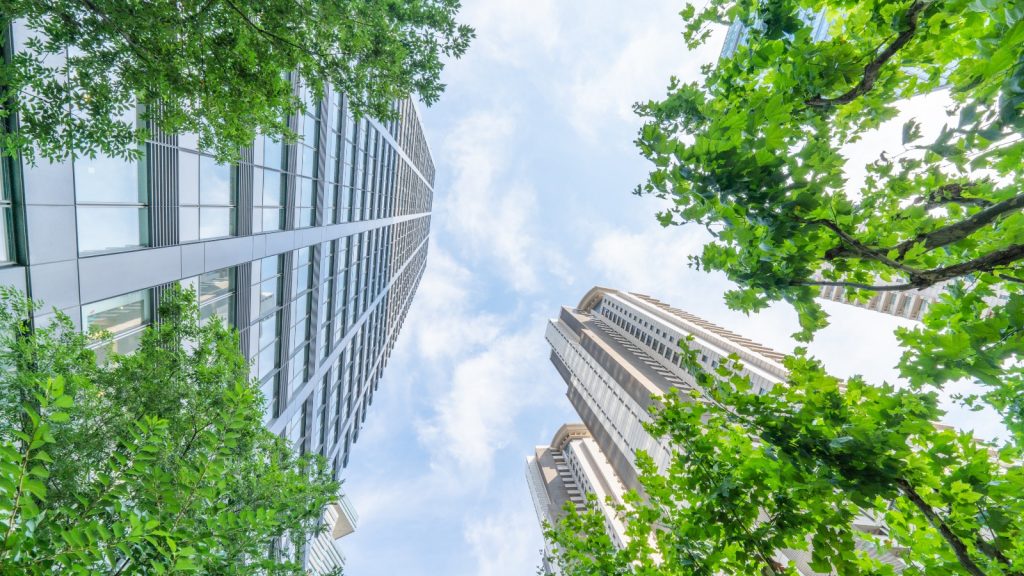
Banks need to step up efforts on climate and environmental risk disclosures
In March 2022, the European Central Bank (ECB) published its second snapshot of climate-related and environmental risk disclosure levels among significant institutions under its direct supervision. In line with the results of the first snapshot published in November 2020 – regarded as the baseline measurement – none of the institutions in scope for this second […]
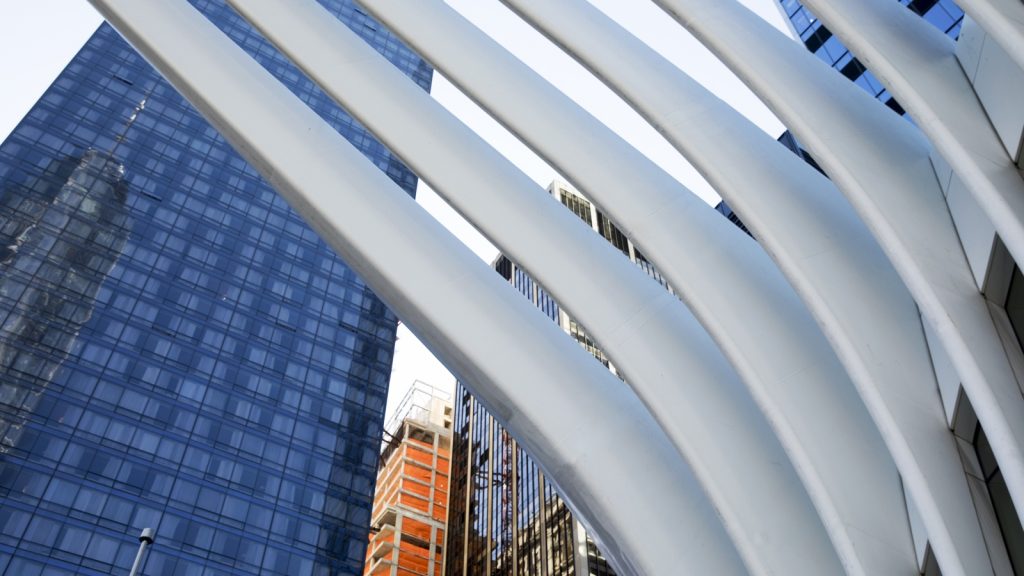
Prudential risks for banks with a Russian presence
The invasion of Ukraine by Russia on 24 February 2022 is considered the most significant geopolitical event since the Second World War. While there is no question of military intervention by the European Union (EU) at the moment, the EU has nevertheless decided on a major package of sanctions that will have a heavy impact […]
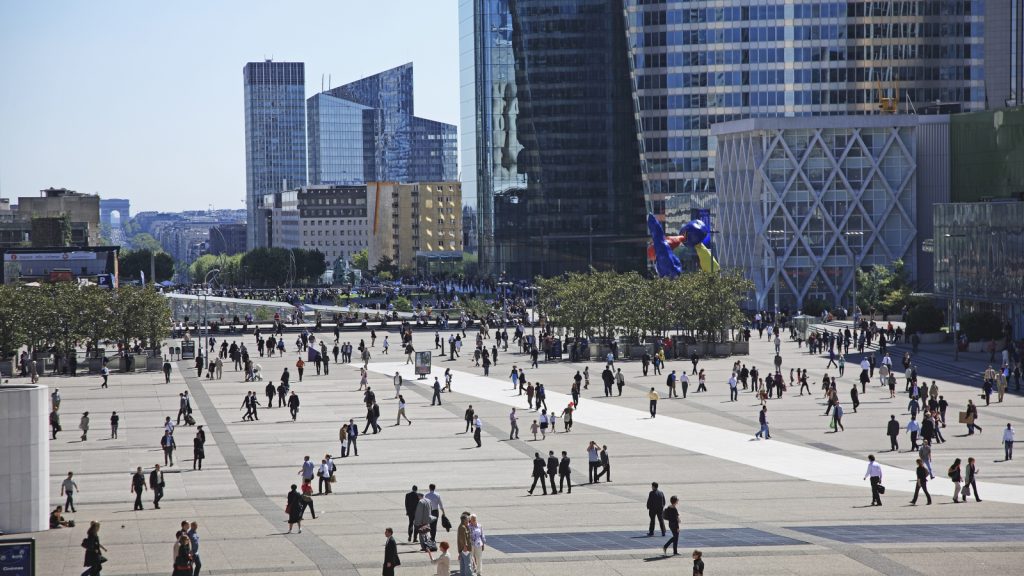
EBA launches a central database for AML/CFT
A central database to strengthen the anti-money laundering and counter-terrorist financing (AML/CFT) framework was launched by the European Banking Authority (EBA) on 31 January 2022. Called EuReCA, the new database will be essential to coordinate efforts by national competent authorities and the EBA to prevent and fight money laundering and terrorist financing (ML/TF) risks throughout […]
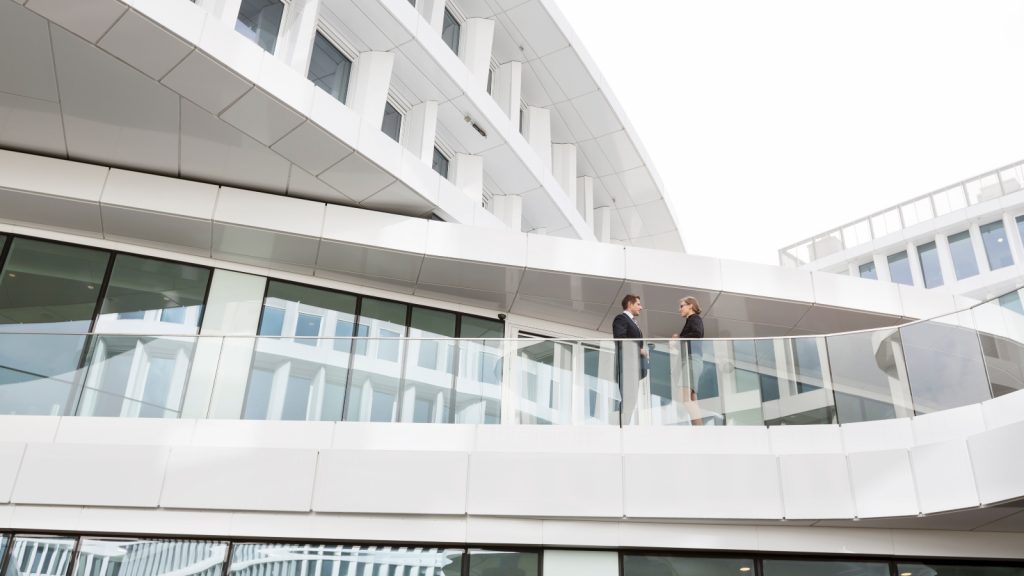
GDPR has controls over subcontractors in its line of fire
Like all industries, the real estate sector has to implement a range of legal, technical and organisational measures to protect the personal data of its employees, customers, prospects and suppliers. Processing must comply with several regulations related to data protection, including, for example, the General Data Protection Regulation (GDPR), applicable since 25 May 2018. Same […]
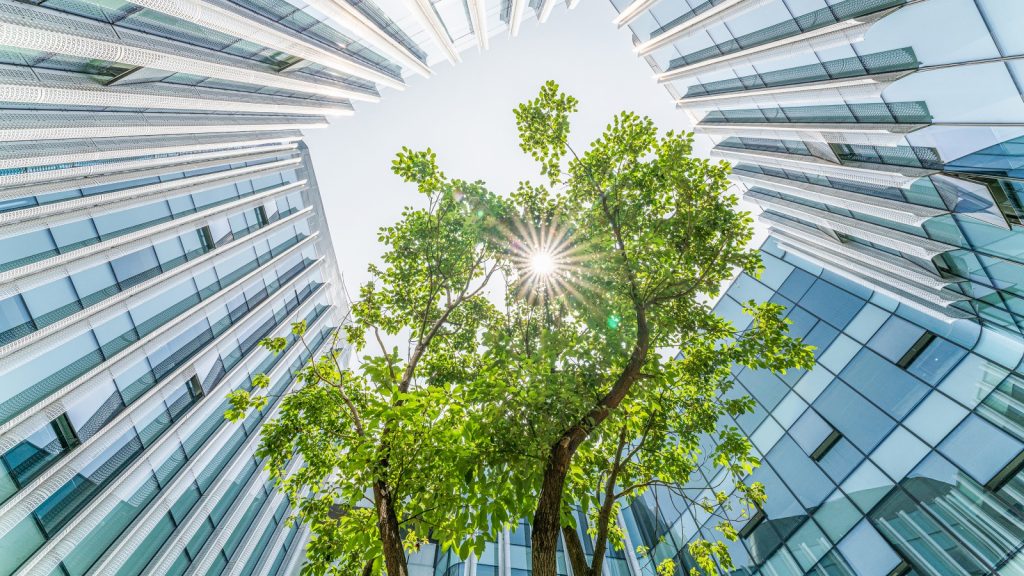
Embarking on an ambitious common climate framework
As part of the European Green Deal, the European Union intends to encourage green investments and prioritise the revision of the Non-Financial Reporting Directive (NFRD). The European or Green Taxonomy, which sets out a precise classification of sustainable activities with the strategic objective of redirecting capital flows towards those activities from 2022, is a result […]
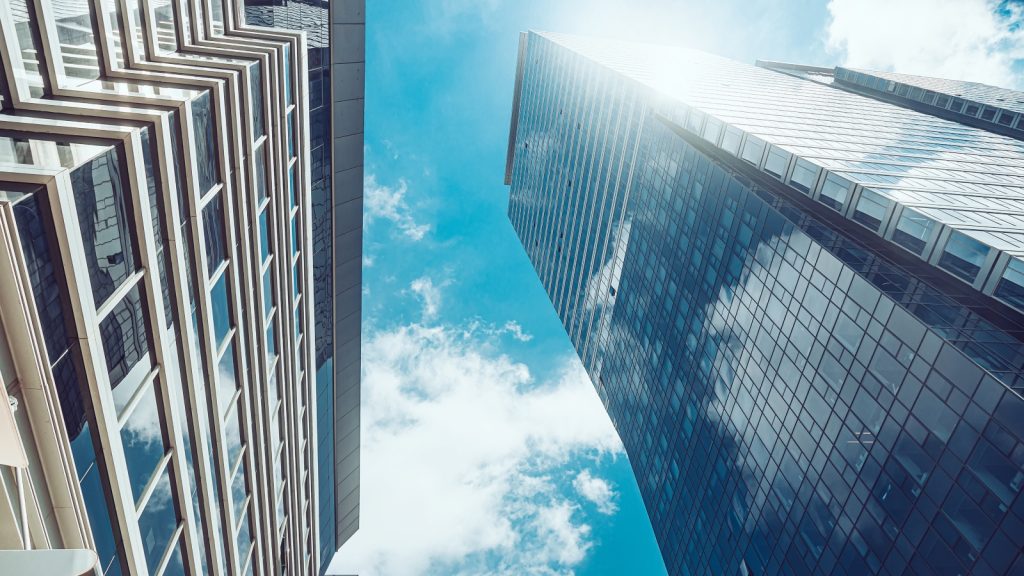
Fast close implications for real estate management companies
The market is seeing a growing trend for real estate management companies seeking to speed up the process of closing their accounts. This is largely due to the positioning of real estate funds in life insurance contracts requiring increasingly shorter valuation intervals. Furthermore, as the regulatory obligation to publish Net Asset Values (NAVs) within the […]
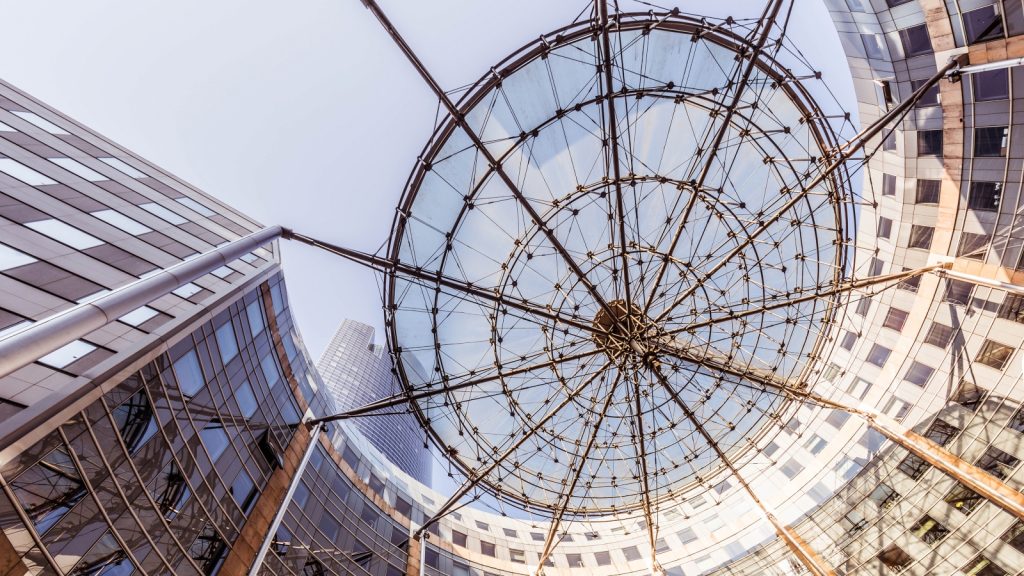
France’s EU Council Presidency to focus on new growth model
As part of its rotating Presidency of the EU Council for a six-month mandate, France chaired its first EU Council of Finance Ministers (ECOFIN) meeting on 18 January with a view to getting certain current legislative work finalised. The ECOFIN meeting was mainly dedicated to introducing the Presidency’s roadmap for the months to come. Beyond […]
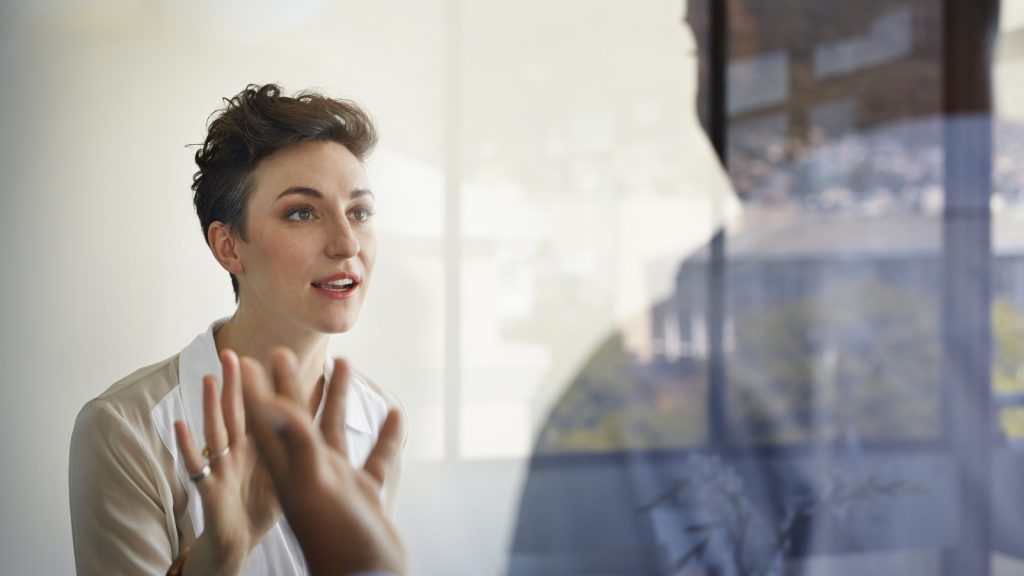
Positive behavioural and cultural change: the implementation of an accountability framework
As regulated entities execute their post-Brexit strategies and relocate their European Union (EU) operations from the UK to other EU states, a key issue to be addressed for those relocating to Ireland remain to be the impending legislative changes surrounding increased accountability standards for executives and non-executives. Not least, the breaking of the participation link, […]
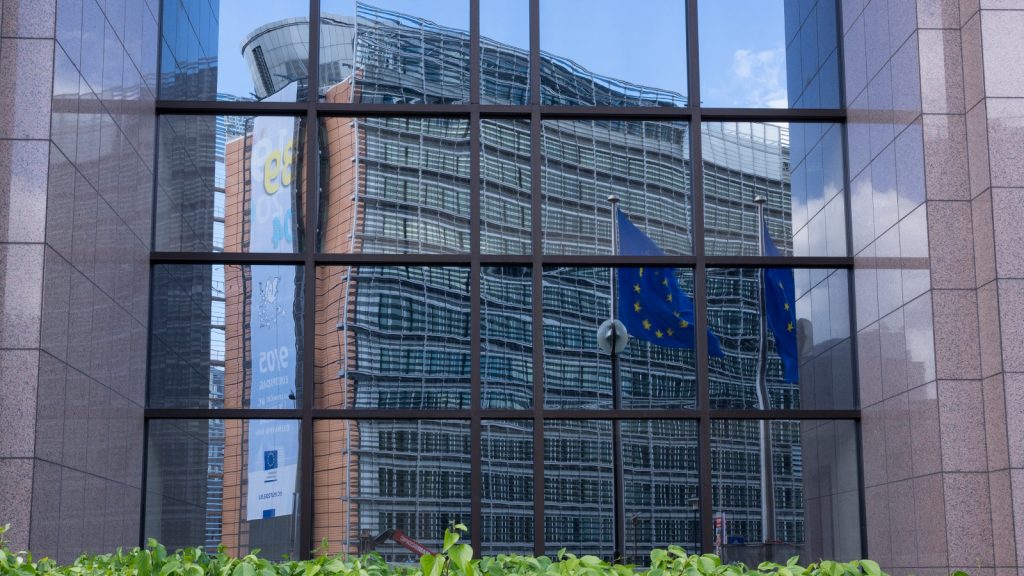
The long road to proportionality in prudential regulation and supervision
The great financial crisis triggered a massive wave of bankruptcies in the worldwide banking sector, affected not only large international banks such as Lehman Brothers but also local ones such as Northern Rock in the UK. Basel prudential standards are designed to cope with financial risks stemming from the global banking system without taking into […]
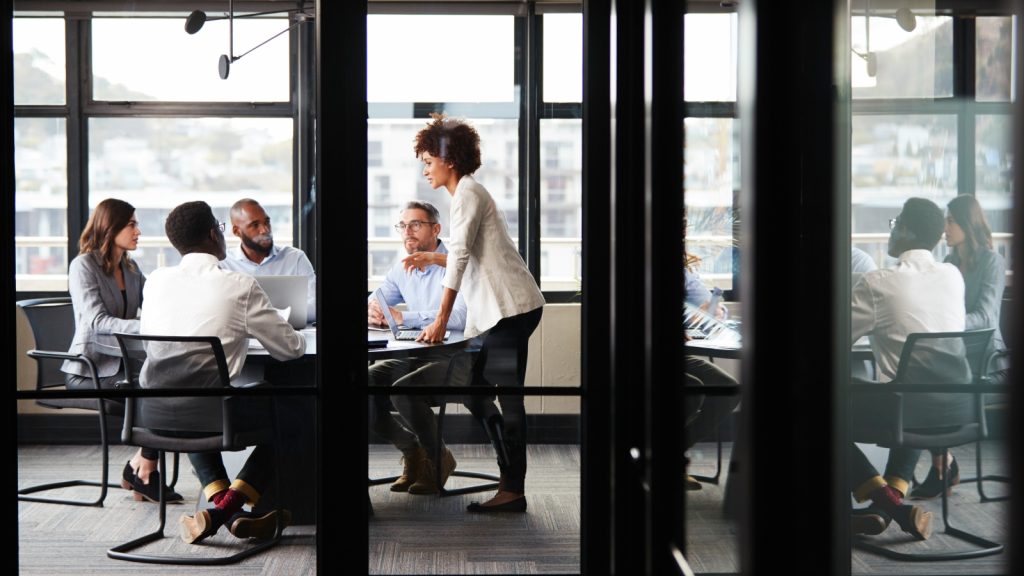
Quarterly SSM briefing: spotlight on supervisory priorities, banking union and liquidity ratio
Supervisory priorities 2022-2024 In December 2021, the European Central Bank (ECB) and the national supervisory authorities of the Eurozone countries published their supervisory priorities for 2022-2024. The three-year coverage enables the ECB banking supervision to achieve good progress in addressing the identified vulnerabilities while at the same time affording enough flexibility in any corresponding actions […]
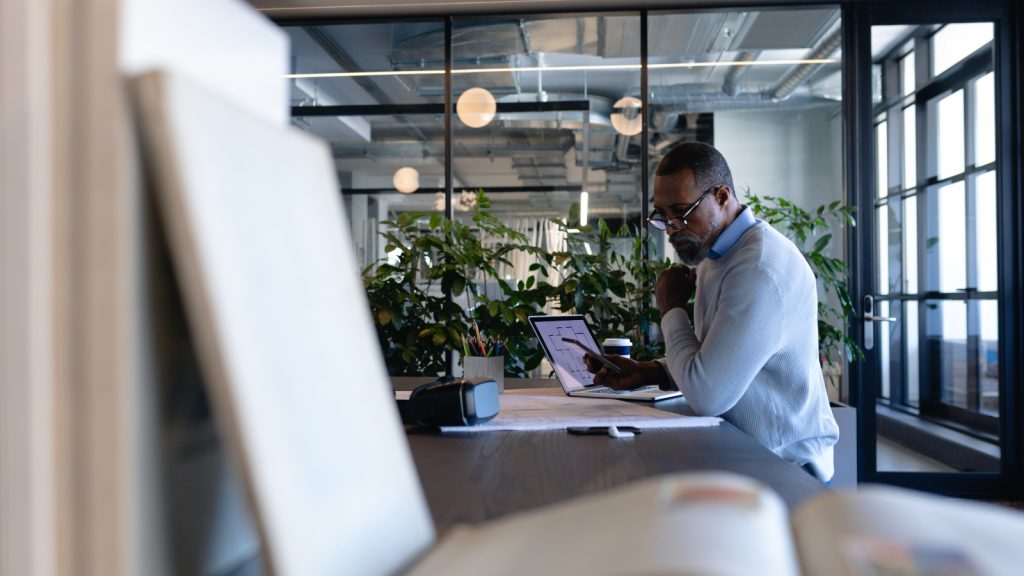
Sustainability and climate risk: what can banks expect?
The growing importance of sustainability issues and the role of credit institutions in financing transformation places climate and environmental risks at the core of regulatory and supervisory scrutiny today. For some years now, the Network for Greening the Financial System (NGFS), comprising central banks and national supervisory authorities, has been working to enhance sustainability and […]
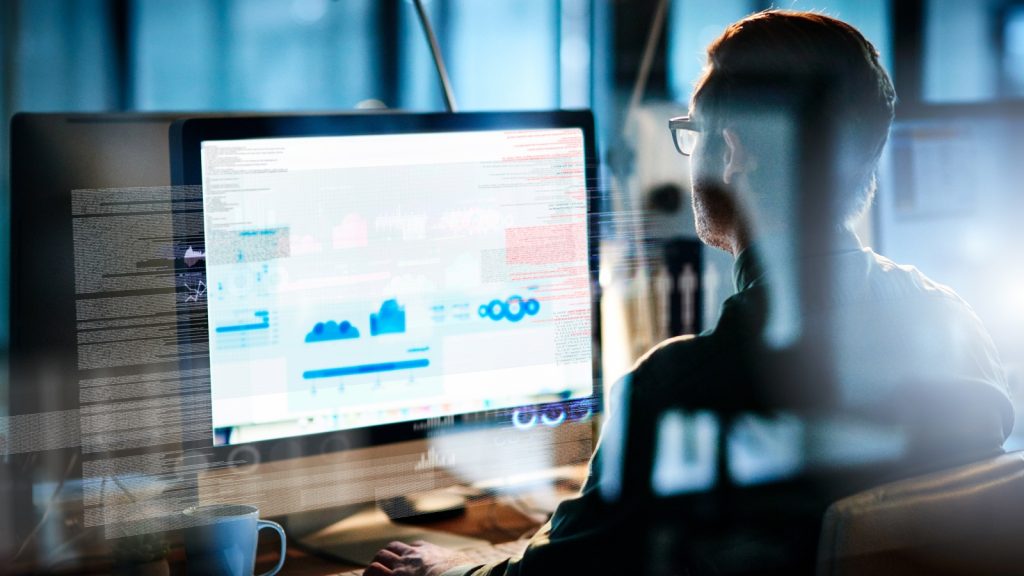
The imperative of expanding the traditional MRM function
Financial institutions and non-bank financial technology companies (FinTechs) alike make extensive use of various machine learning models (MLOps) in core and non-core areas of their business. Banks, for example, rely on such models for a range of risk assessments, including predictive underwriting, credit risk management, suspicious and/or fraudulent activity management, fair lending compliance, derivative and […]
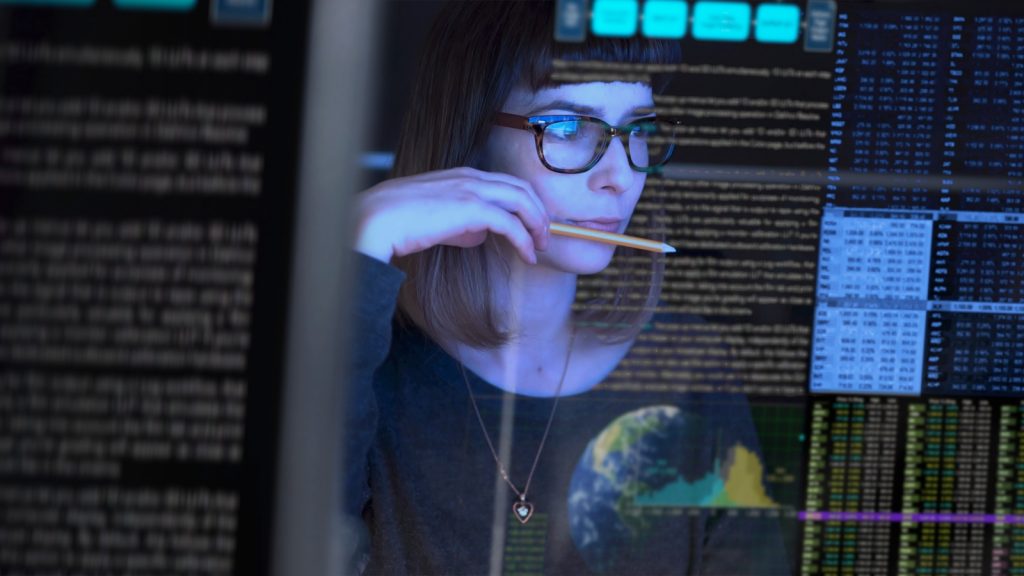
Can banks balance the opportunities and challenges of digitalisation?
The Covid-19 pandemic has amplified technology’s impact on the banking sector, helping to prove that technology now stands at the core of business sustainability for banks. In their constant search for convenience, digitally-savvy customers have pushed banks’ focus towards providing global business solutions more than ever. A new normal has emerged: an environment where banks’ […]
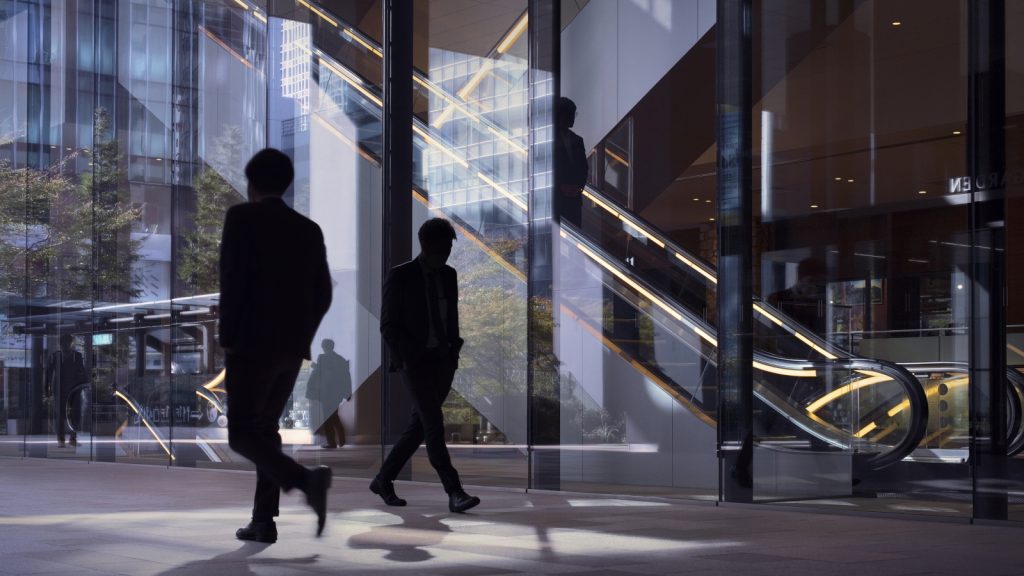
New European authority aims to strengthen framework to fight money laundering
The creation of a new Anti-Money Laundering Authority will transform the supervision of money laundering and financing terrorism (AML/CFT) in the EU. Proposed reforms also extend the AML/CFT rules to all crypto-asset service providers, as well as include specific rules concerning due diligence on customers and beneficial ownership. It is expected that some of these […]
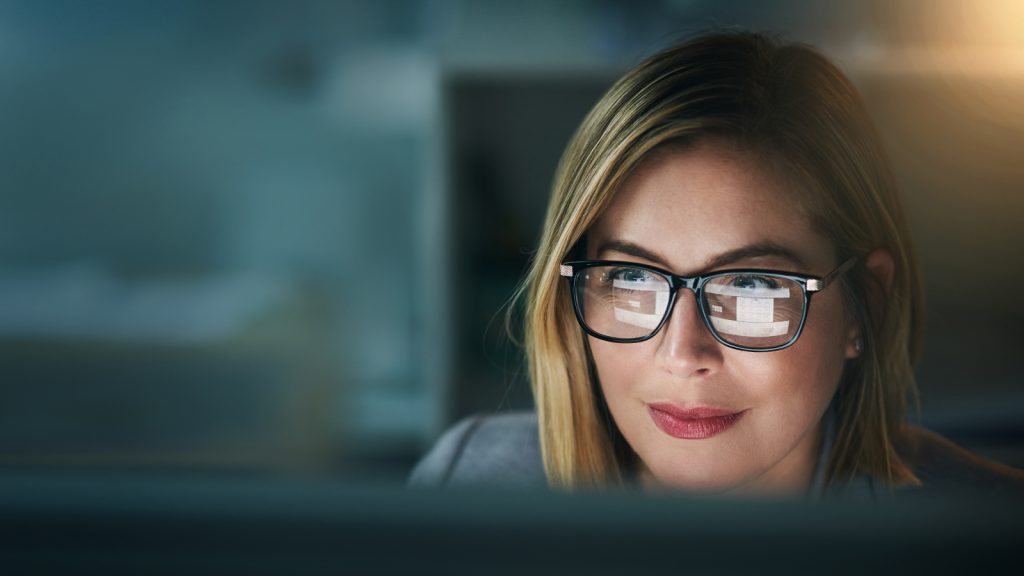
European Commission adopts review of Solvency II
On 22 September, the European Commission adopted a review of Solvency II following the consultation launched by EIOPA in 2020, whose final guidance was published in December 2020. As the Commission notes, the 2020 review of the directive met several objectives: • remove the obstacles to long-term financing of the economy and redirect investment by […]
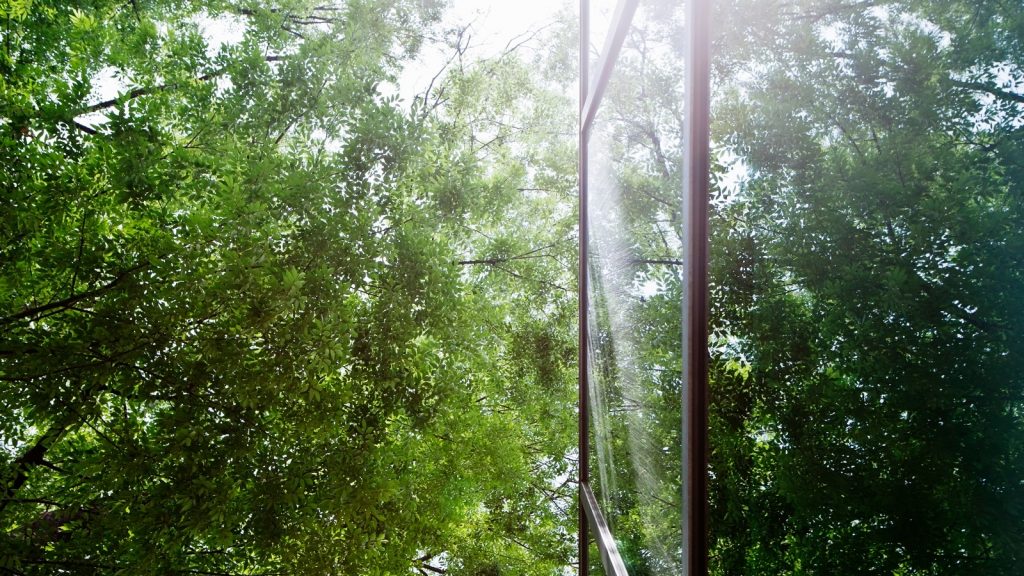
The FSOC weighs in on climate risk
The Financial Stability Oversight Council (FSOC) was established under the Dodd-Frank Wall Street Reform and Consumer Protection Act as a result of the 2007-2008 US financial crisis. A first of its kind, the 15-member council is tasked primarily with identifying growing systemic risks to US financial stability and proposing coordinated regulatory responses to both preempt […]
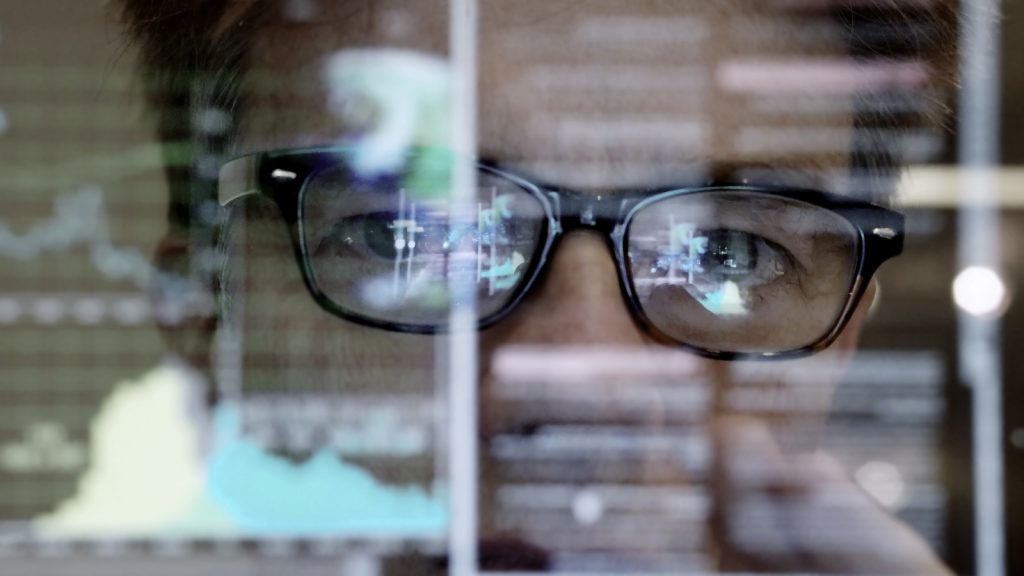
Remote working: A growing target for hackers
The widespread use of working from home (WFH) during the pandemic, regardless of sector or geographical location has required organisations and their information systems (IS) management to be very agile in deploying or increasing their capacity for remote collaboration. Some institutions were already prepared – for example, following the wave of strikes at the end […]
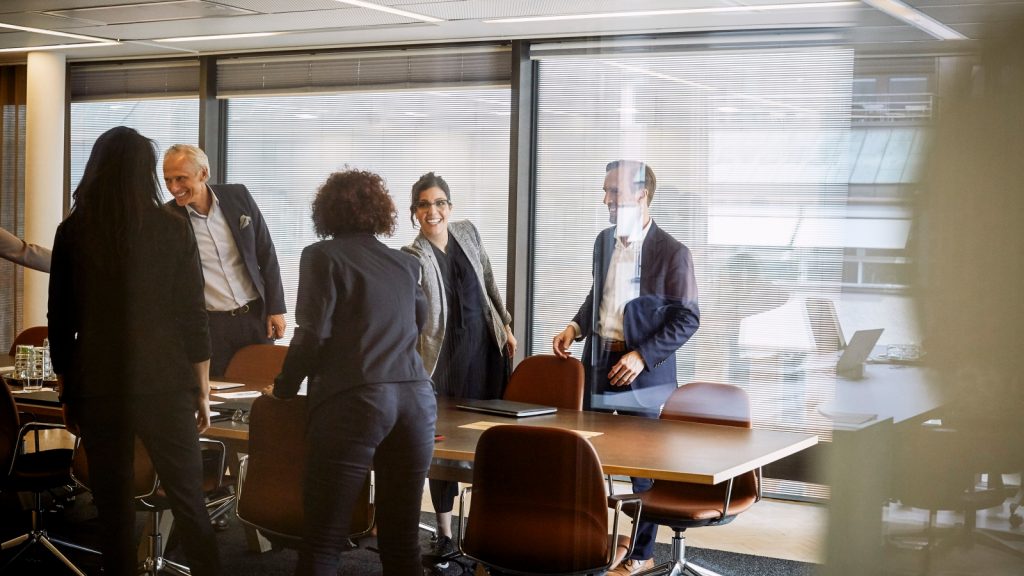
Regulated firms: A matter of life and death
As the PRA transitions from a “rule-taker” to a “rule-maker”, small and medium-sized banks operating in the UK can expect to benefit from a more “streamlined” regulatory regime that could be easier to interpret, implement and maintain; but at the same time, they can also expect the PRA to be progressively more involved in scrutinising […]
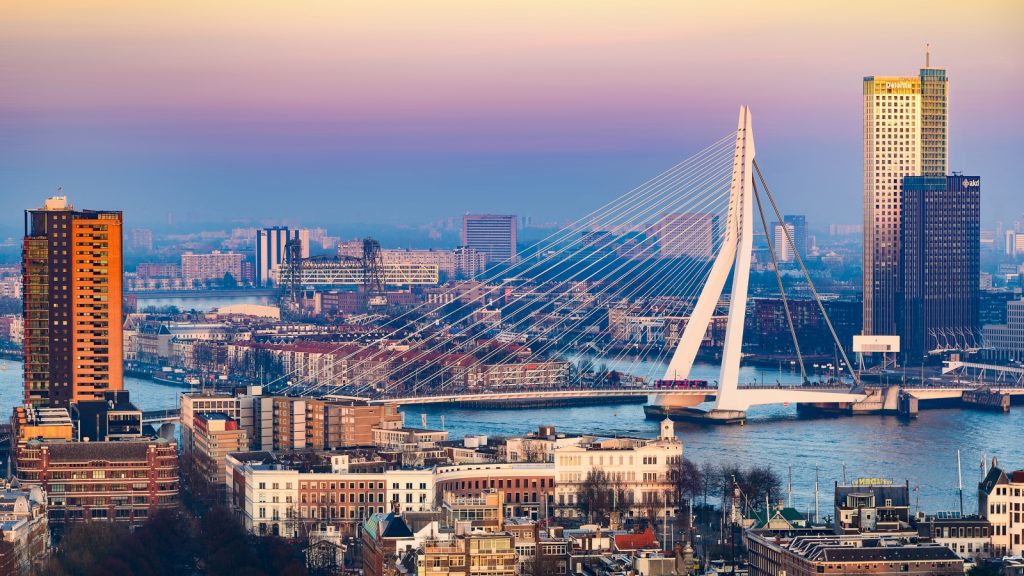
NPL secondary market may solve the increase in credit risk
The identification and management of non-performing loans or NPLs as early as possible by banks are among supervisors’ current high-level priorities. Indeed, when prudential, monetary, and fiscal crisis mitigation mechanisms are tapered, the weakening of borrowers’ creditworthiness could materialise, along with increasing credit risks and therefore NPLs. This expected rise of new NPLs in European […]
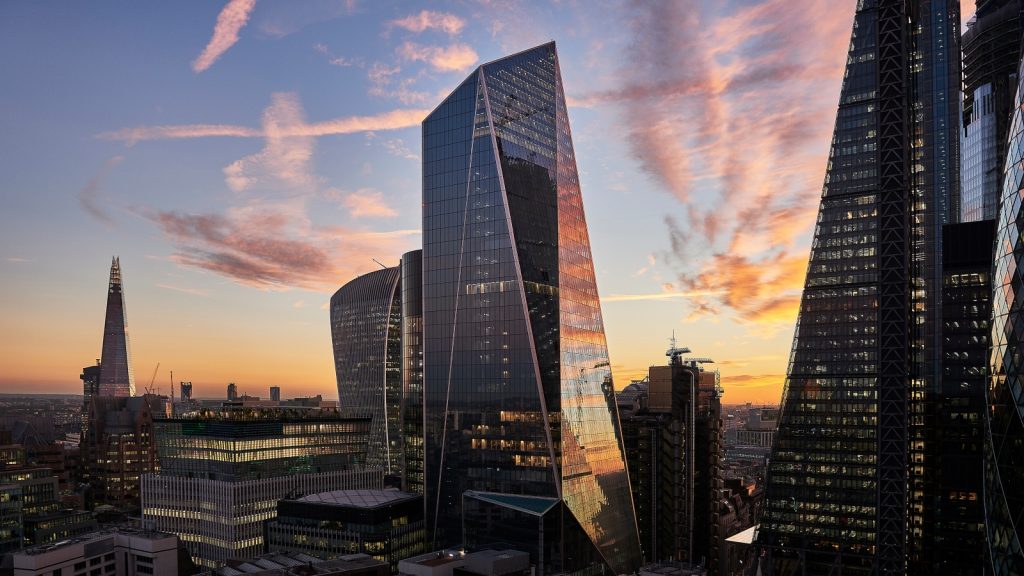
The road to implementing the final Basel agreements
The unveiling of the new banking package “CRR3 – CRD6” on 27 October 2021 presents a further landmark on the road to implementing the final Basel III agreements. The regulatory scheme will also focus on the revision of the market risk framework from January 2019, as well as the latest developments in pillar 3 requirements. […]
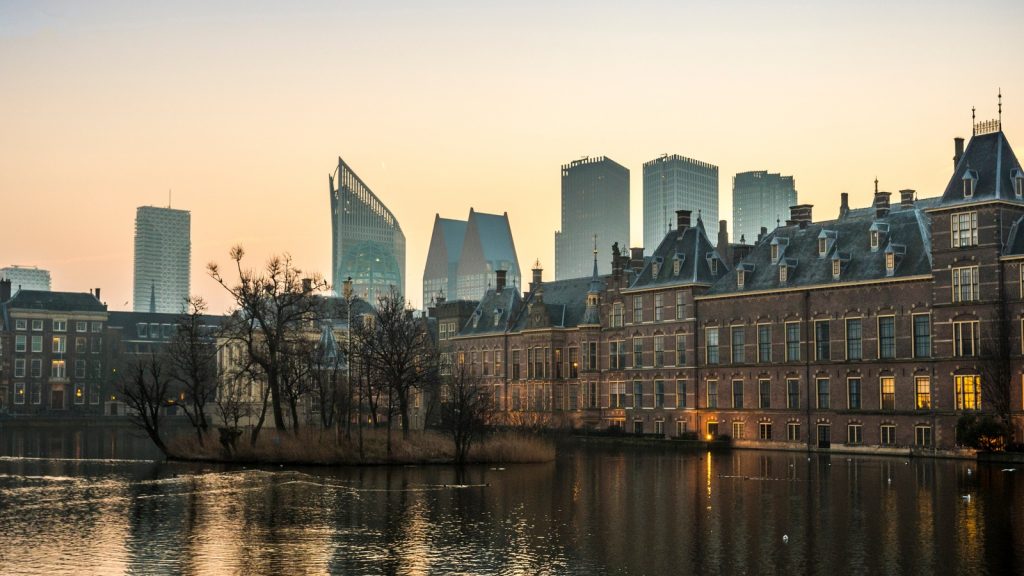
Banking consolidation in Europe: What can we expect?
The low level of banking consolidation in Europe compared to other countries is raising concerns among the supervisory community in Europe. It is a trend further reinforced after the financial crisis of 2007/2008 that produced a noticeable slowdown in consolidation operations in the EU. So what has been the impact, and what can we expect […]
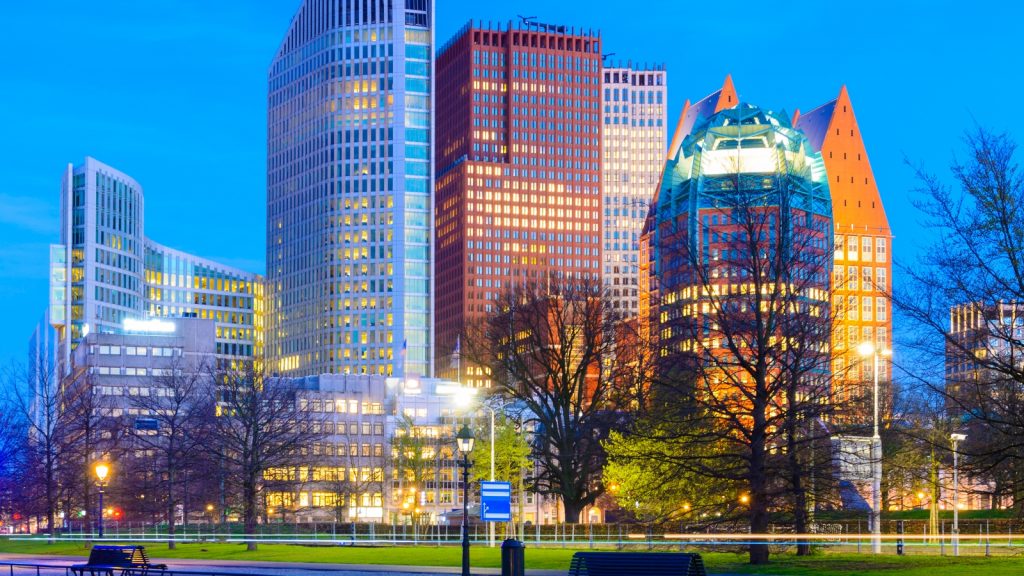
First ACPR climate stress test pilot exercise results
Climate change introduces considerable economic challenges. On the one hand, financial institutions must contribute to the transition to a low-carbon and balanced economy to effectively combat global warming. On the other hand, the financial sector is exposed to climate-related and environmental risks and therefore needs to implement appropriate risk management practices within a financial stability […]
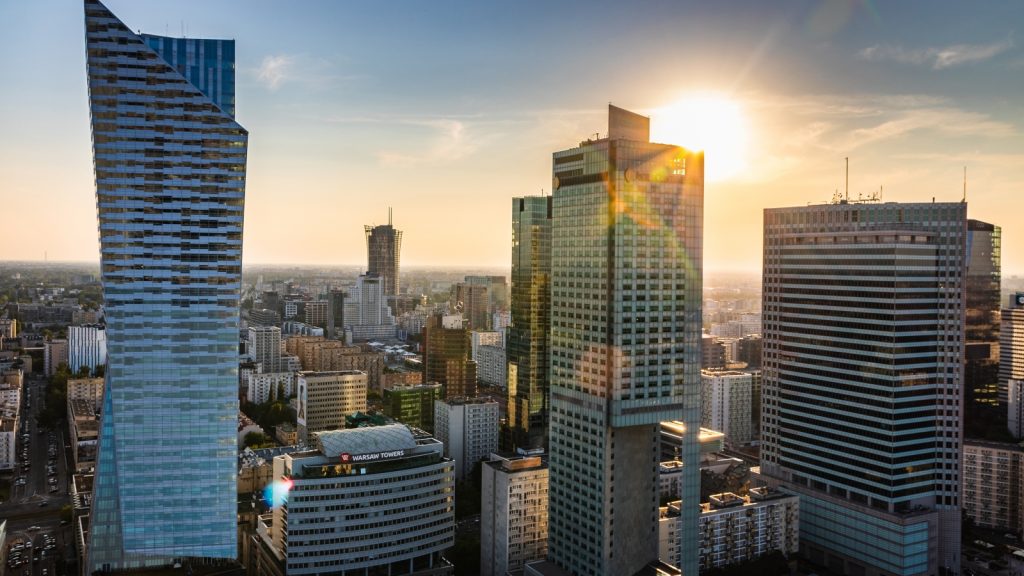
The Single Supervisory Mechanism: Post-pandemic actions and expectations
On 30 July, the European Central Bank unveiled the 2021 supervisory stress test results, which demonstrated that the region’s banking system is resilient in an unfavourable environment. The Common Equity Tier 1 (CET1) ratio has fallen 5.2% to 9.9% under the 3-year adverse scenario, while under the baseline scenario the CET1 ratio will reach 15.8% […]
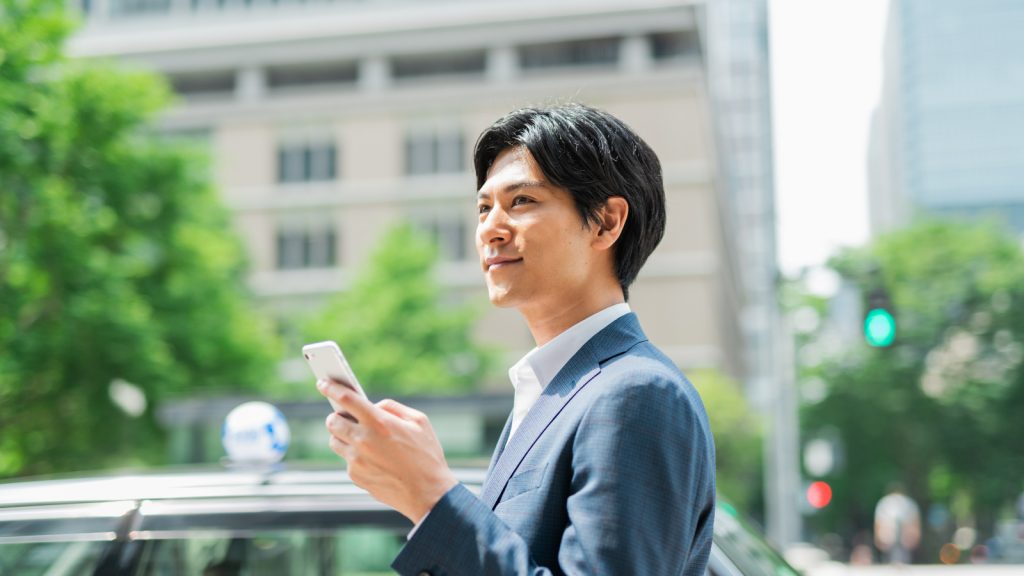
ESG investing: Three risks to consider
The continued popularity of funds with an environmental, social and governance (ESG) focus has put global ESG assets on track to exceed $53tn by 2025, up from nearly $38tn at the end of 20201. As growth continues, expectations for effective compliance policies and controls in place are expected to become more rigorous as political and […]
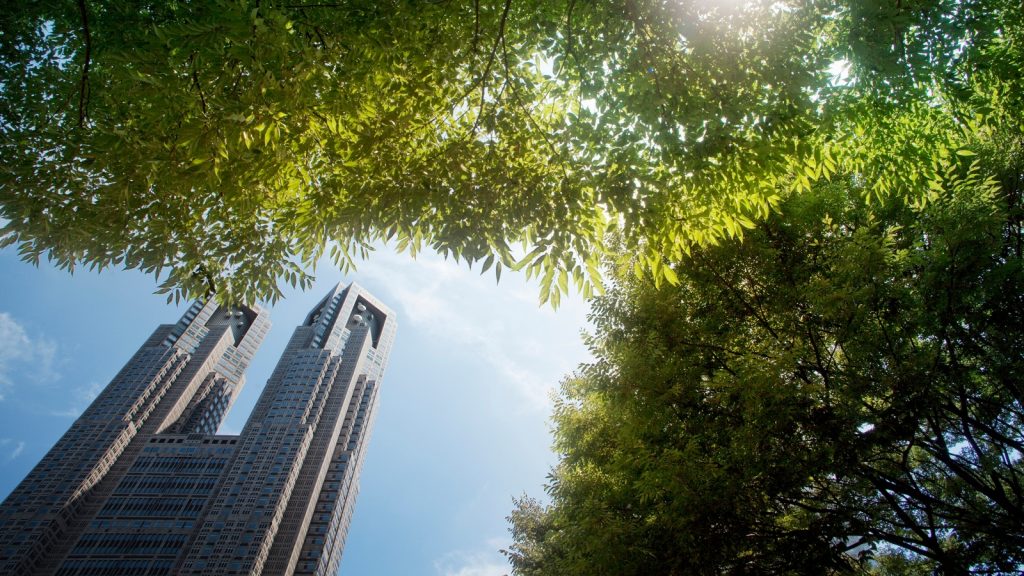
ESG investing: From buzzword to mainstream
A growing interest in environmental, social and governance (ESG) issues is driving record inflows into the ESG-led investment sector. During 2020, sustainable funds available to European investors attracted net inflows of €233bn1, which saw assets under management hit the $1.1tn milestone, accounting for almost 10% of total European fund assets. A similar growth story in […]
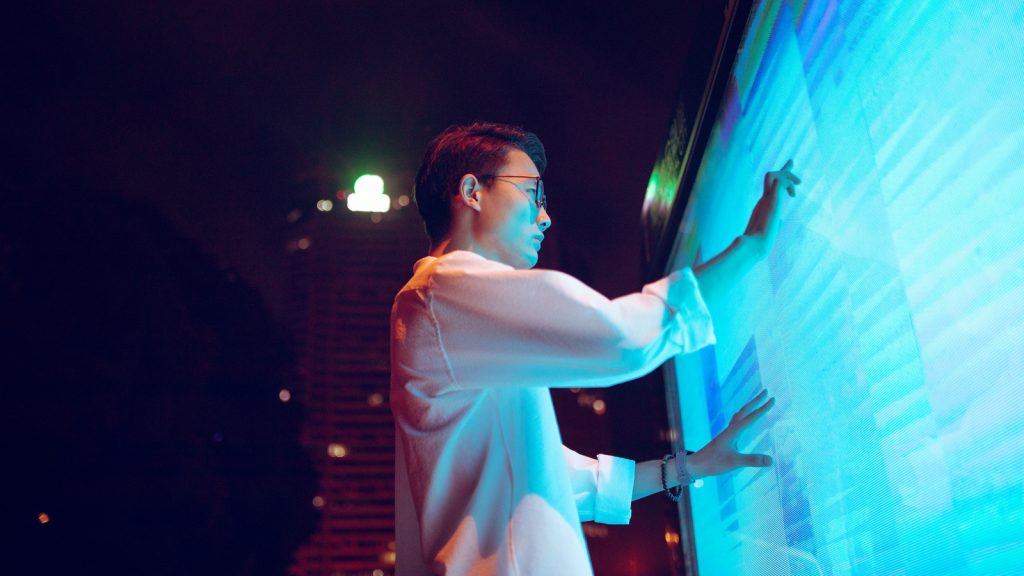
Deep hedging: application of deep learning to hedge financial derivatives
The recent breakthrough of data science and deep learning make a model independent approach for hedging possible. This hedging approach known as deep hedging is a robust data-driven method able to consider market frictions as well as trading constraints without using model-computed greeks. This article gives the main theoretical tools to understand the methodology and […]
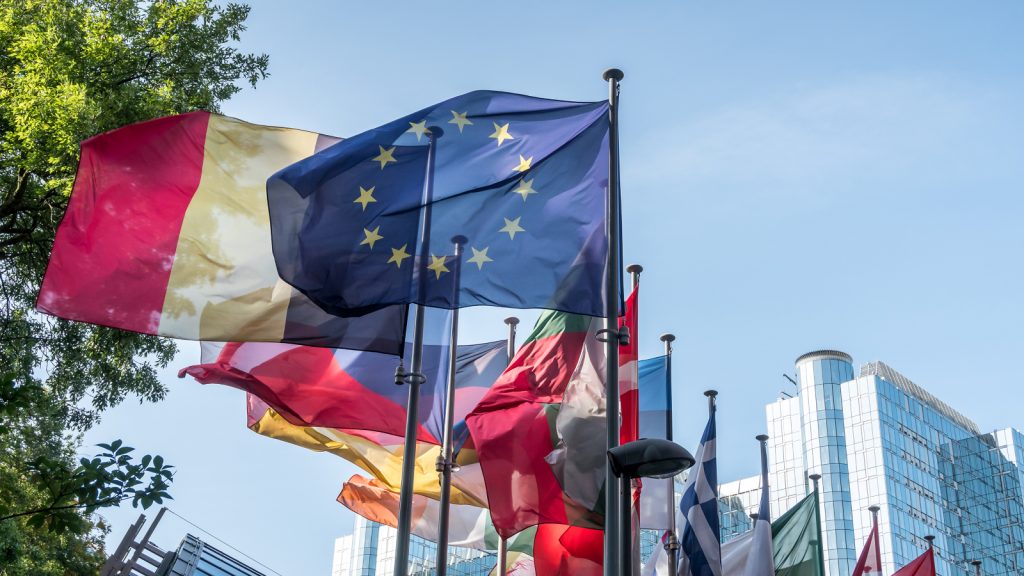
How the new prudential regime will impact EU investment firms notably French
A major step forward has been taken in implementing the European “investment firms” package in France*. The transposition of the package into local legislation, alongside EU Regulation 2019/2033 on prudential requirements for investment firms (IFR), came into full effect on 26 June 2021. In accordance with the proportionality principle, the IFR and investment firms directive […]
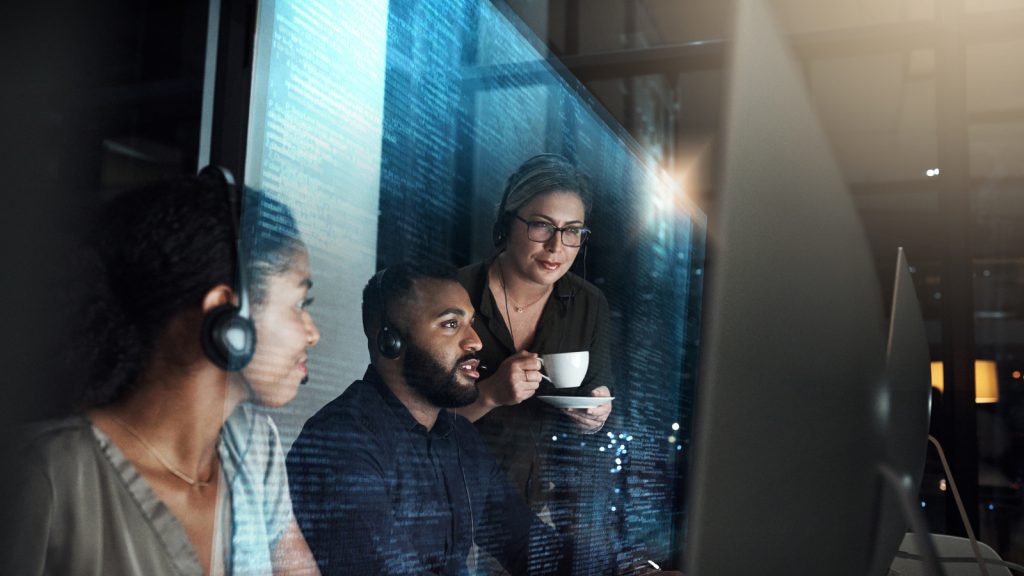
How banks can address supply chain risk
Local and international trends have transformed the way banks operate, affecting their capital positions and profitability. In particular, ongoing digitalisation programmes and technological innovation continue to add pressure on traditional banking models, including the supply chain. While management’s focus on capital preservation, profitability and growth for shareholders remains, risks from an operational perspective have intensified. […]
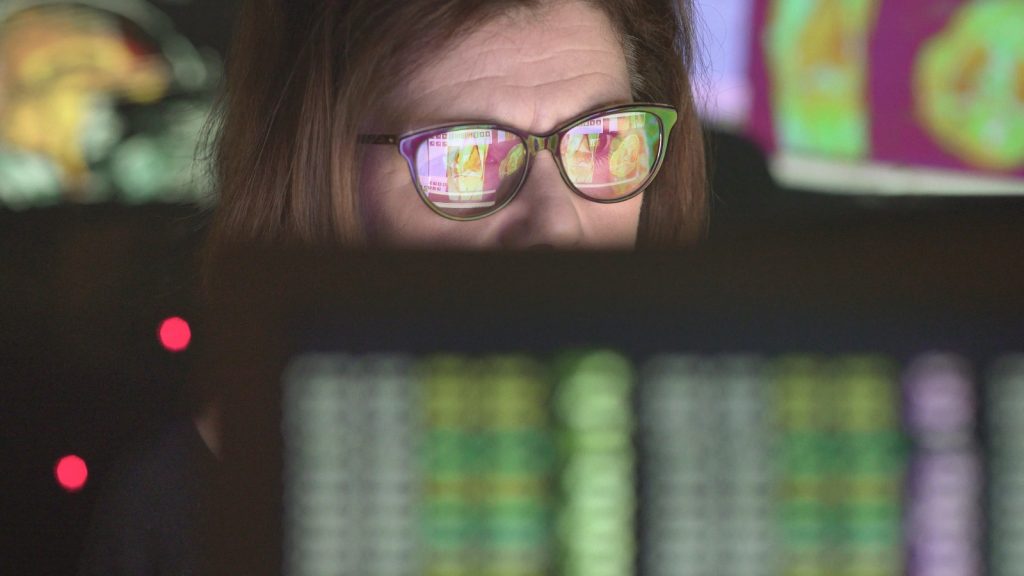
Five steps to transforming banking operating models
With the current ultra-low interest rate environment and market volatility having a negative impact on banks’ returns and, ultimately, their capital positions, operating models must quickly adapt and become more cost-efficient to maintain profitability. This drive for cost-efficiency has become more apparent as innovation in technology and ongoing digitalisation have further upended traditional banking systems […]
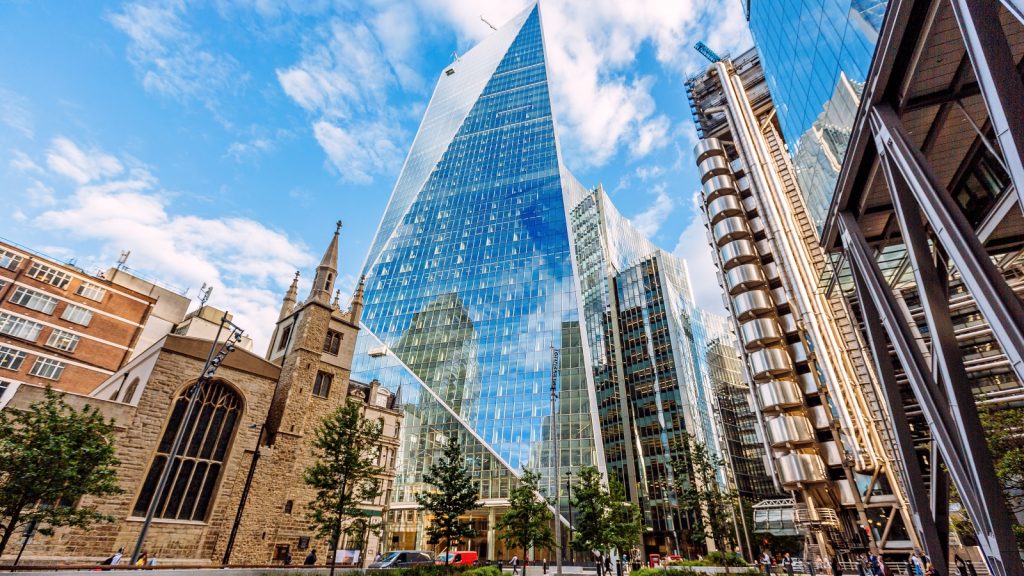
2021: The year of Brexit for banks
Brexit, or the UK’s departure from the European Union, became a reality on 1 January 2021. In terms of the regulatory impact for the financial sector, and the banking sector in particular, the UK being a third country, UK banks can no longer benefit from the European passport for their continental activities. Therefore, they can […]
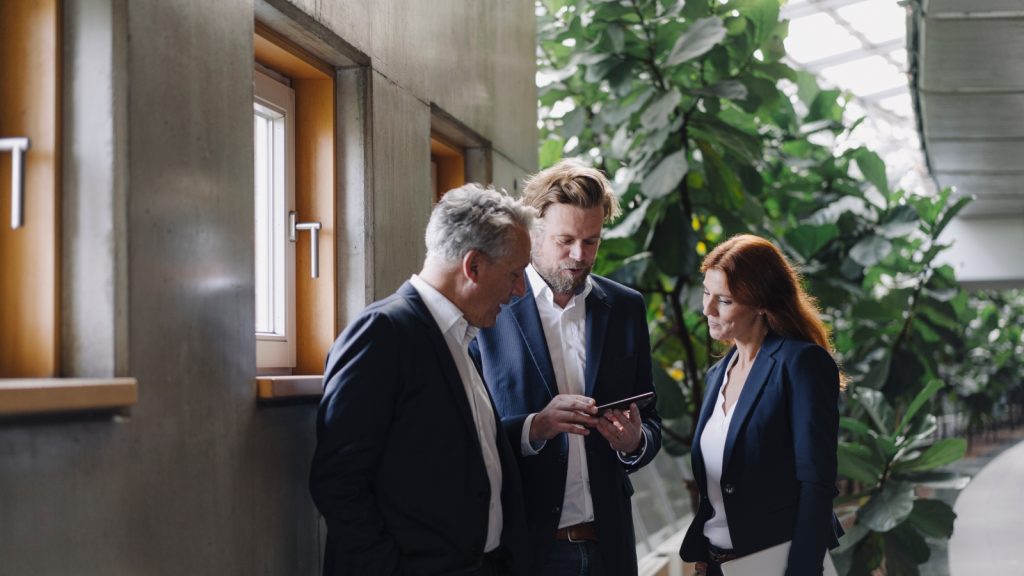
EBA: draft technical standards on Pillar 3 disclosures of ESG risks
On 1 March 2021, the European Banking Authority (EBA) launched a public consultation on draft implementing technical standards (ITS) for Pillar 3 disclosures of environmental, social and governance (ESG) risks, under its capital requirements regulation (CRR) mandate. The consultation will end on 1 June 2021. Large banking institutions with securities traded on a regulated market […]
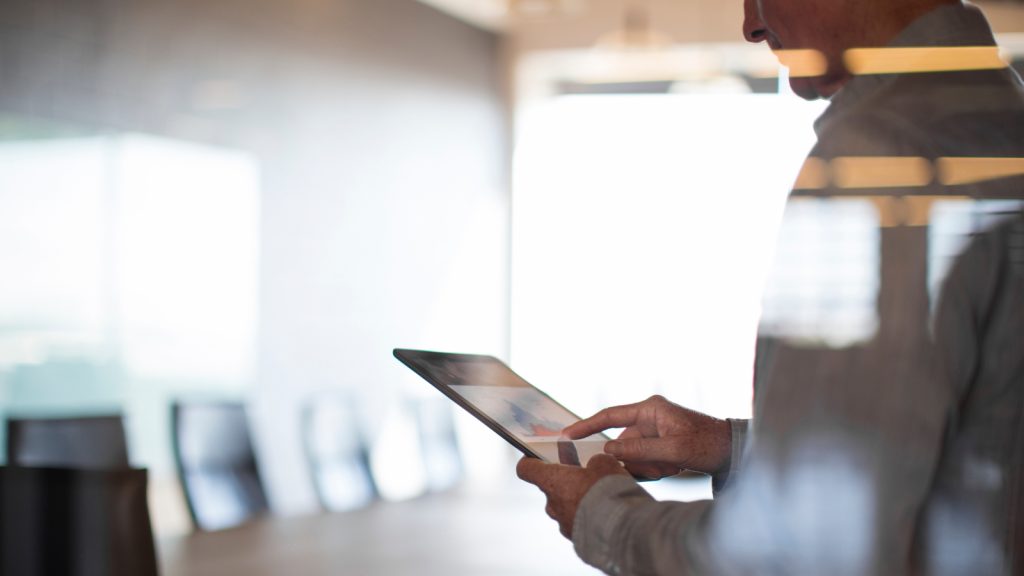
Achieving digital operational resilience
The digitalisation of banking processes and the introduction of AI-led technology impact the central and strategic role of information systems within the banking system. The growing use of information and communication technology (ICT) exposes all financial institutions to an increasing level of digital risk that could weaken their operational resilience, in particular, due to more […]
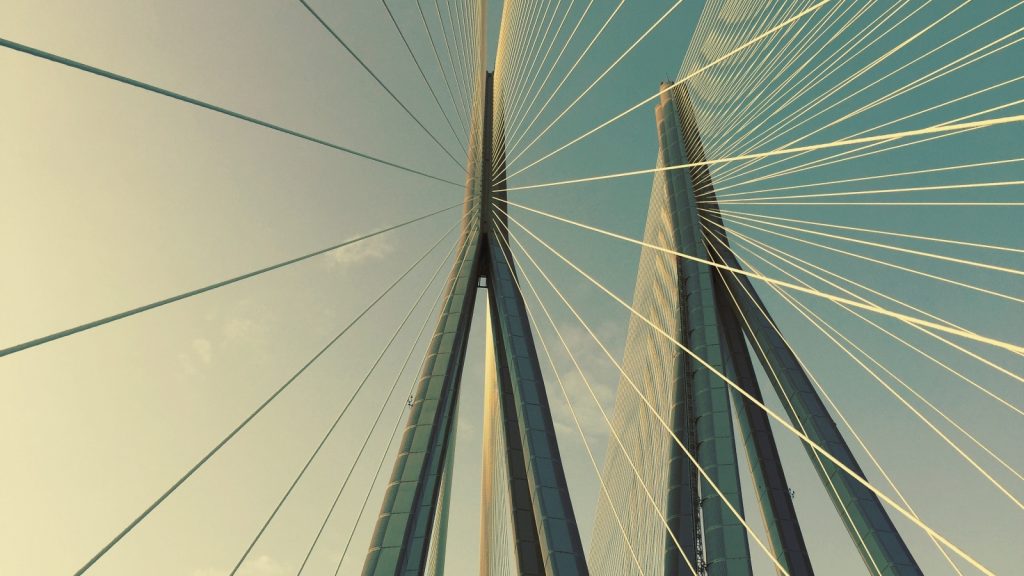
Key takeaways & industry challenges following the ECB TRIM project – a focus on credit risk (Part 2)
The Targeted Review of Internal Models (TRIM) was one of the largest projects by the European Central Bank (ECB) aimed at identifying potential sources of unwarranted or non-risk based variability in Significant Institutions (SIs) risk-weighted assets (RWA) from the use of Pillar 1 internal models such as Probability of Default (PD), Loss Given Default (LGD) […]
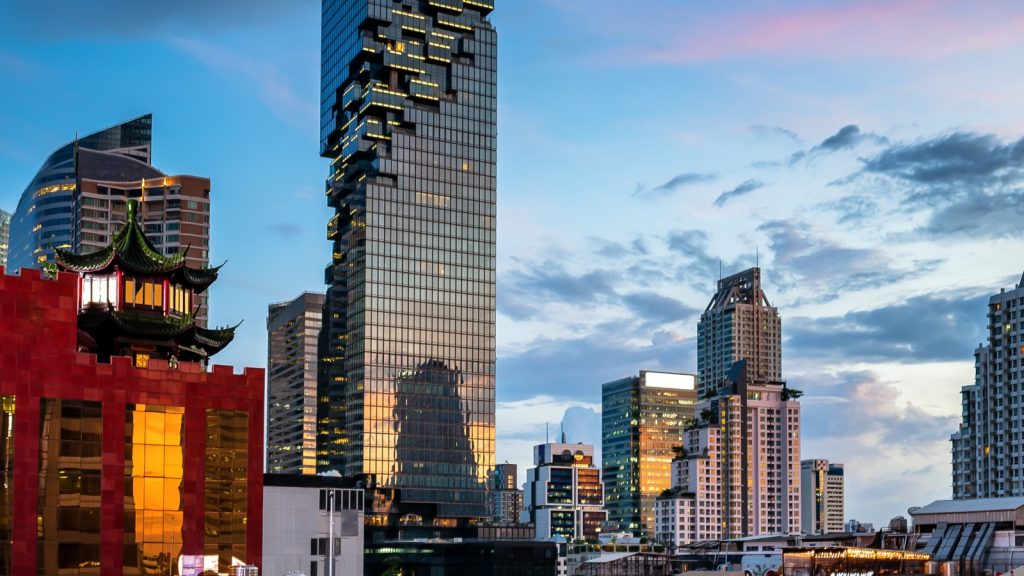
Key takeaways & industry challenges following the ECB TRIM project – a focus on CCR (Part 1)
Click here to read ‘Key takeaways & industry challenges following the ECB TRIM project – a focus on credit risk (Part 2)’ As articulated by the ECB in its recent TRIM reporting, the 236 findings cover different key aspects of supervised entities Internal Model Method (IMM) models & frameworks. Remediation actions are underway in all […]
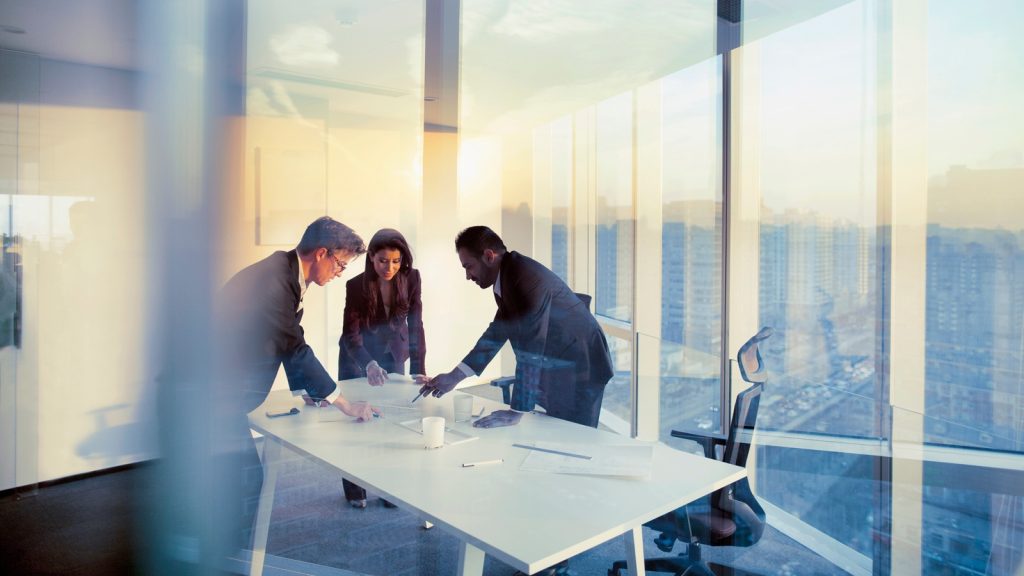
The Basel Committee: updated guidance on the external audit of banks
Against the background of a new year still severely affected by the persistence of the pandemic throughout the world and economies facing an unprecedented global macro-economic shock, the Basel Committee has felt it necessary to address the audit of the expected credit loss (ECL) accounting estimate within the overall financial statement audit. With IFRS 9 […]
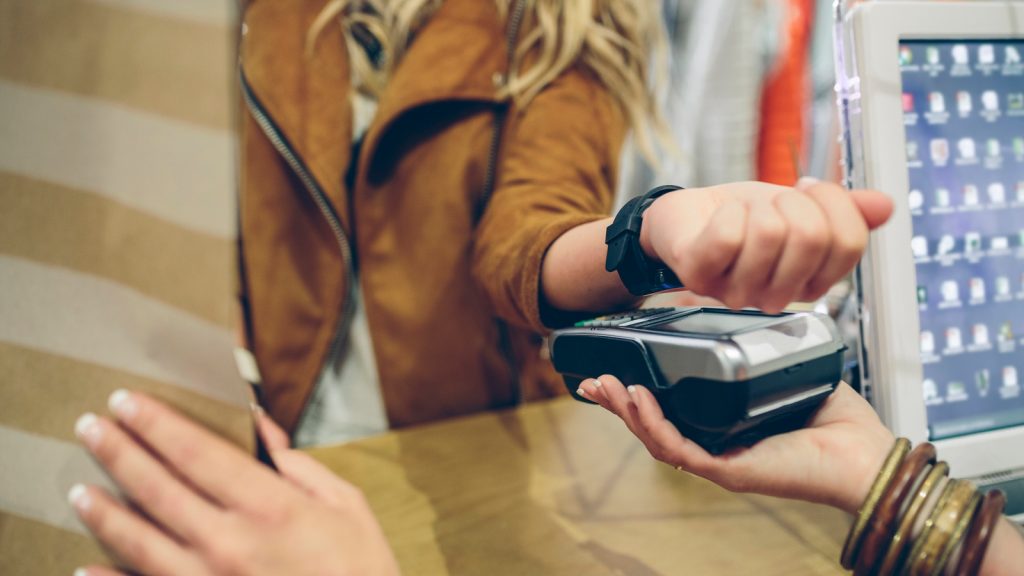
Shaping the future of banking with 5G
Over the past decade, the financial services industry has been disrupted by the arrival of new players whose rise to prominence has pushed traditional banks – previously faced with little competition – to transform themselves. In this context, technology and innovation, particularly 5G, will allow the most skilful and agile banking organisations to take advantage […]
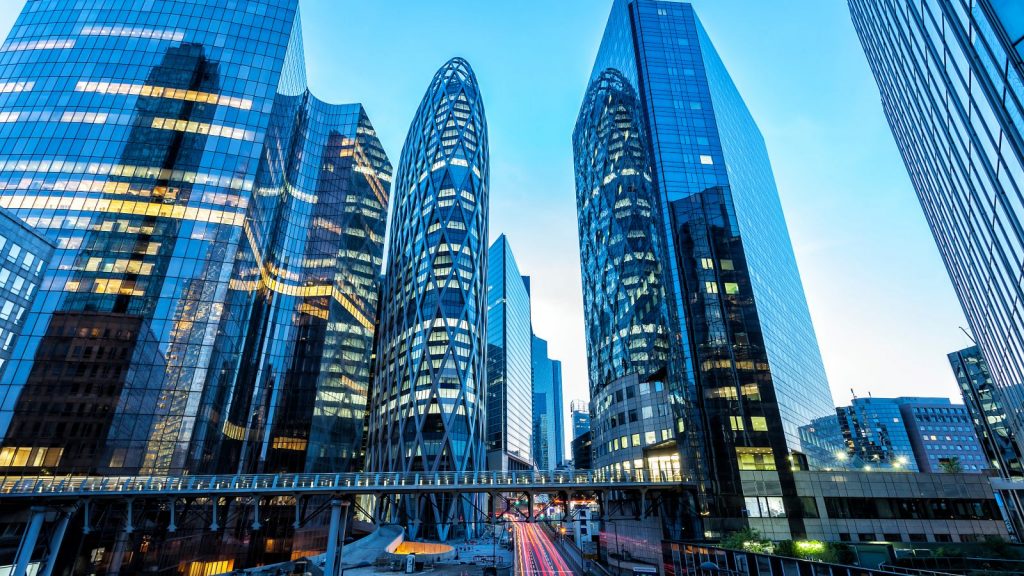
Managing an increase in bank credit risk
While 2020 went relatively smoothly for the banking sector, uncertainties remain on the potential effects of Covid-19 on the real economy. Any negative impact could lead to heavy losses for the sector, especially when support measures are gradually phased out. These measures have not only contained the anticipated increase in credit risks, but have also […]
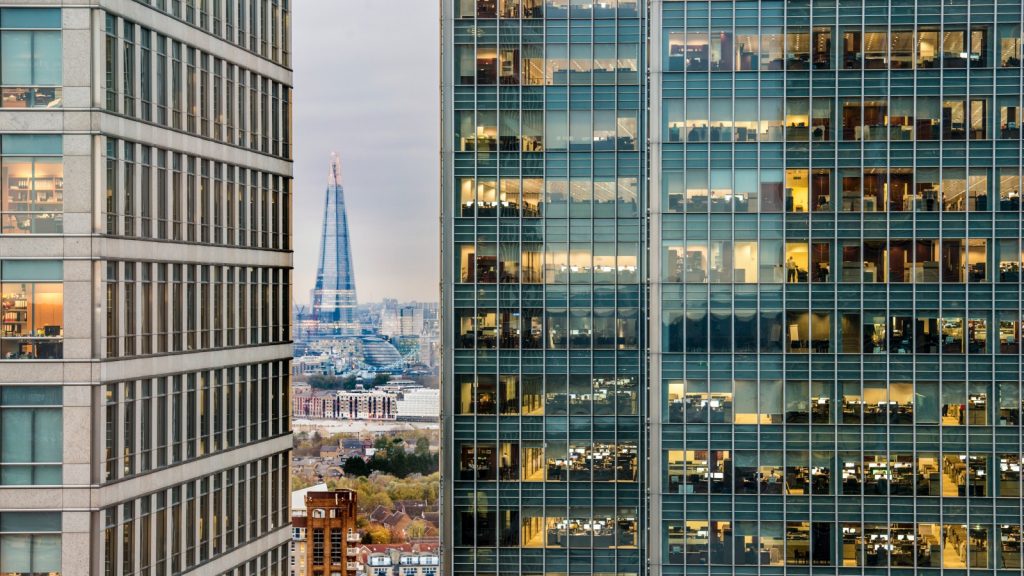
The impact of credit risk on 2021 stress tests
On 13 November 2020, the EBA published the final methodological note for the 2021 EU-wide stress-testing exercise. The aim of the stress tests is to assess the resilience of financial institutions to adverse economic and financial developments, in particular in the event of an increase in credit risk due to the default of the borrower. […]
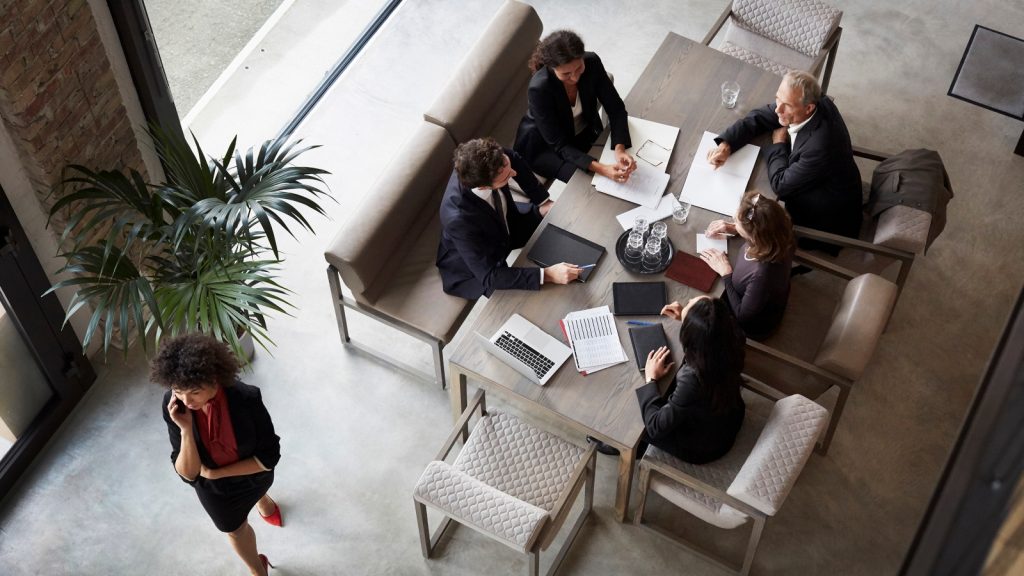
2021 stress tests planned as banks face worsening crisis
The publication on 29 January of baseline and adverse scenarios, output templates, instructions and market assumptions required to carry out stress tests signals the go-ahead by the European Banking Association (EBA) for the 2021 regulatory exercise. Because of the Covid-19 pandemic, these tests, originally planned for 2020, will take place between now and 31 July […]
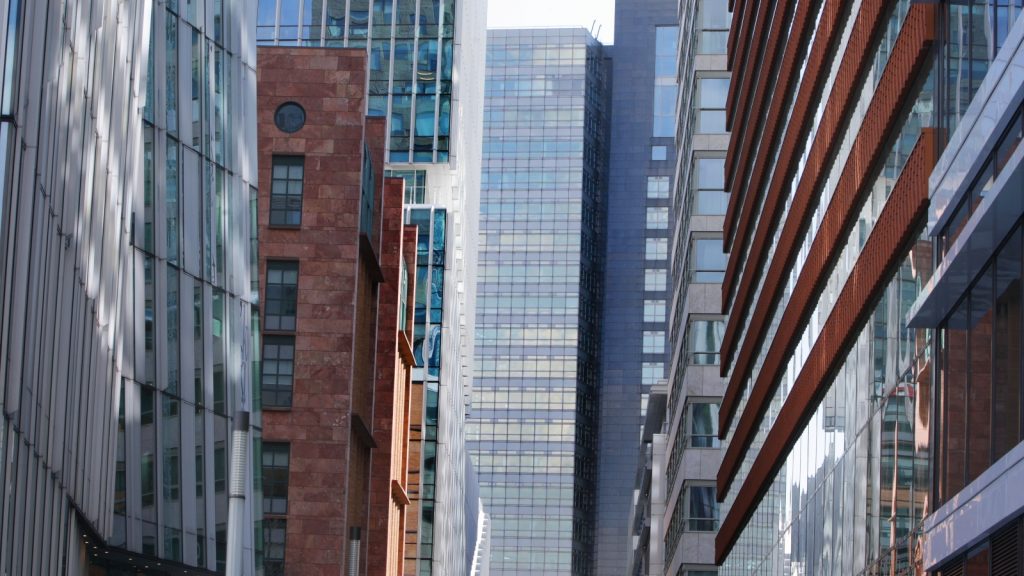
Resolvability is now the SRB’s key focus
With the economic repercussions of the Covid-19 crisis yet to be fully assessed, a robust resolution framework is essential to ensure the stability of the banking system. While the banks were given leave to postpone the reporting of some less urgent information in spring 2020, the Single Resolution Board (SRB) has reiterated the importance of […]
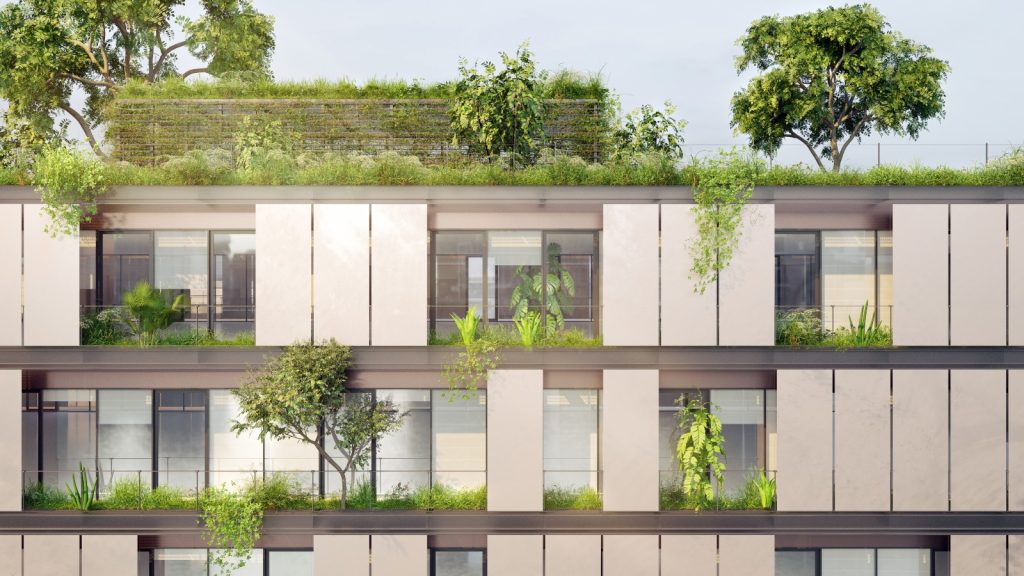
Smart buildings that benefit the city, people and the environment
While there is a consensus about the smart building concept, there are still challenges to overcome if tomorrow’s buildings are enhanced to benefit the environment, cities and people. Starting with their design and throughout their useful lives, buildings have a huge impact on the environment and the quality of life of their users. So how […]
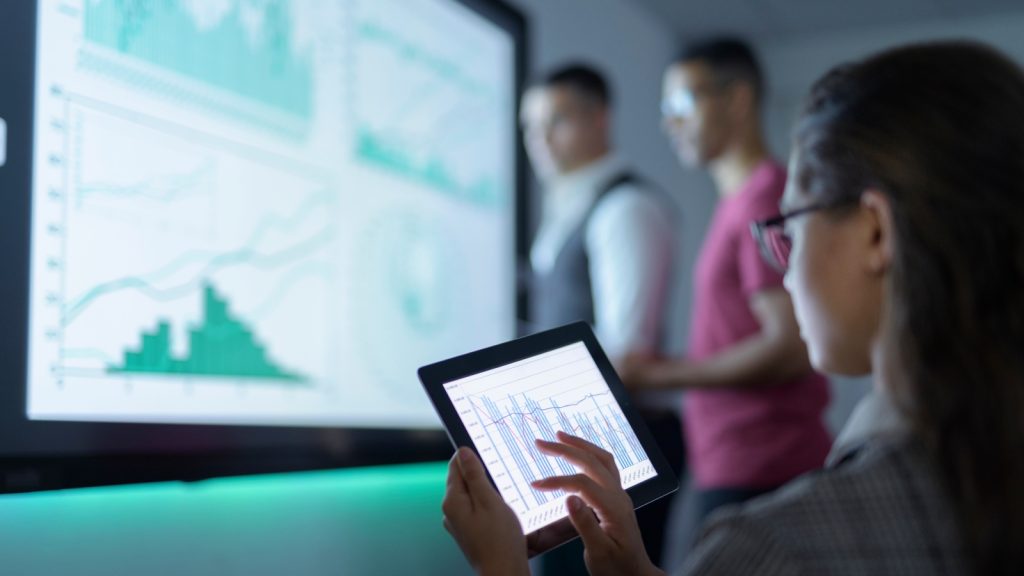
Digitalising the real estate industry
While digital transformation has been disrupting all business sectors for many years, 2020 will be remembered for its particular impact on real estate administrators. A third of French respondents believe that artificial intelligence can be more efficient than a real estate agent. Moreover, 40% of those under 50 years old are convinced that the property […]
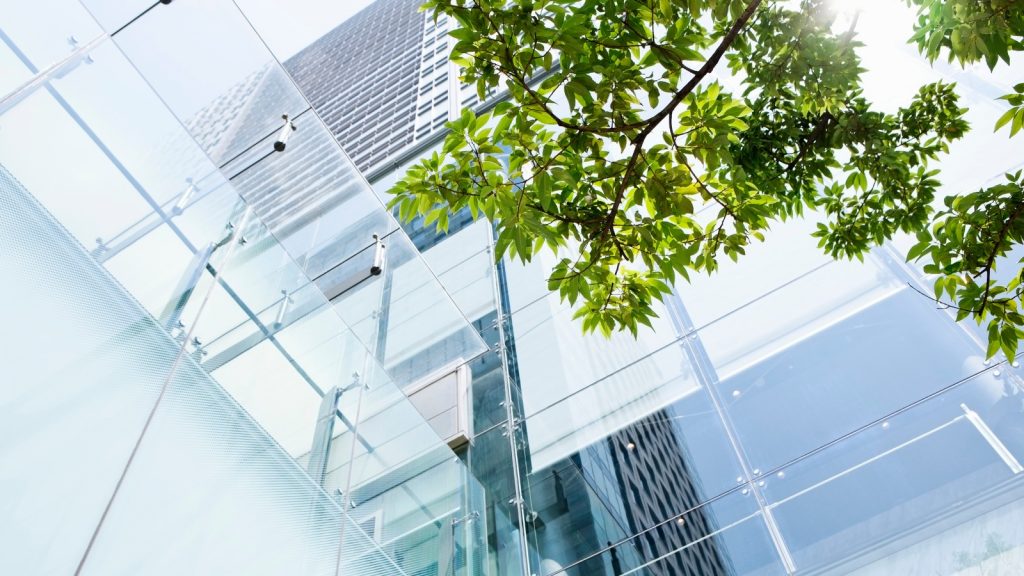
EBA discussion paper on the management and supervision of ESG risks
European sustainable finance regulations evolved considerably in 2020, and the European Banking Authority (EBA) is continuing this trend into 2021. It recently published a discussion paper assessing the potential inclusion of Environmental, Social and Governance (ESG) risks in the supervisory review and evaluation process (SREP) performed by national competent authorities (NCAs)[1]. What firms need to […]
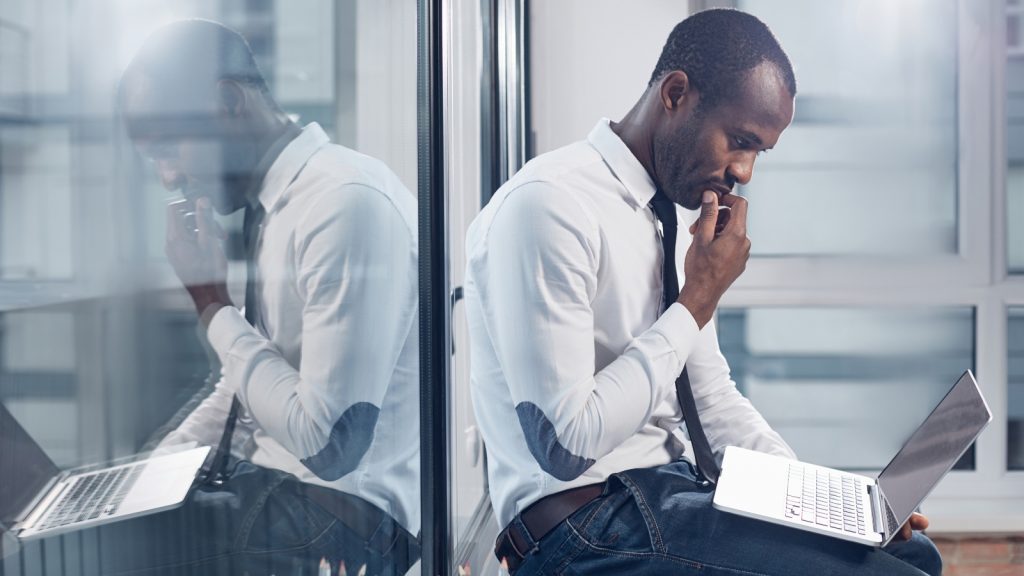
Can Africa’s banking sector maintain its growth momentum?
With more than half of the world’s fastest-growing economies located in Africa1, the continent’s economic outlook is a positive one. Average annual GDP growth since 2000 is over 5%, placing Africa as the second-fastest growing economy behind Latin America. Real GDP growth, estimated at 3.4% for 2019, is projected to accelerate to 4.1% in 2021. […]
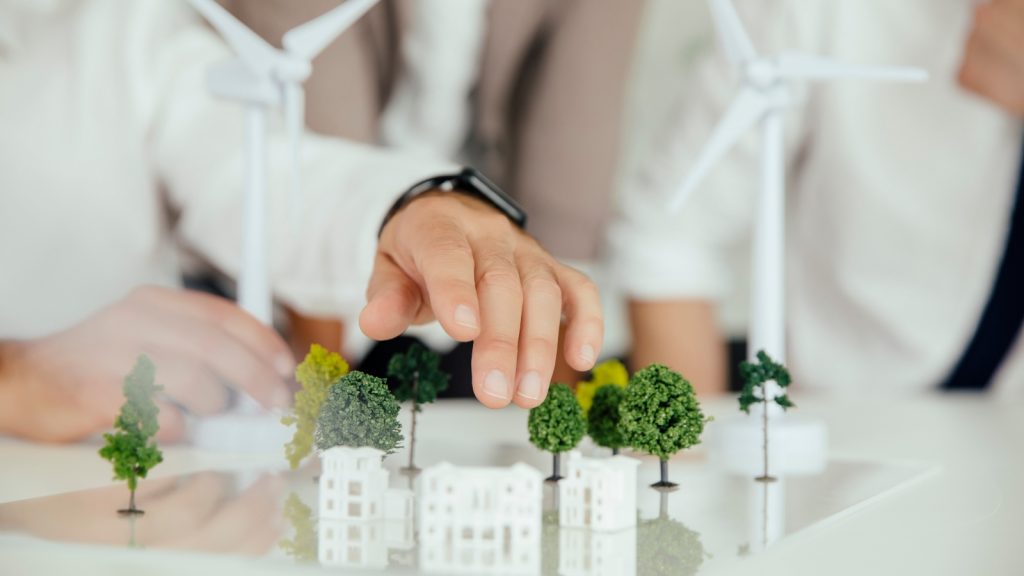
Developing a toolkit for responsible investment decisions
This article is part of the series covering the impact of sustainable finance on the insurance sector. Read further:Part 1: Assessing the impact of sustainable finance on insurance entitiesPart 2: How the insurance sector is meeting ESG challenges Clarity of information provided to various stakeholders is a growing issue for financial organisations. Despite the efforts […]
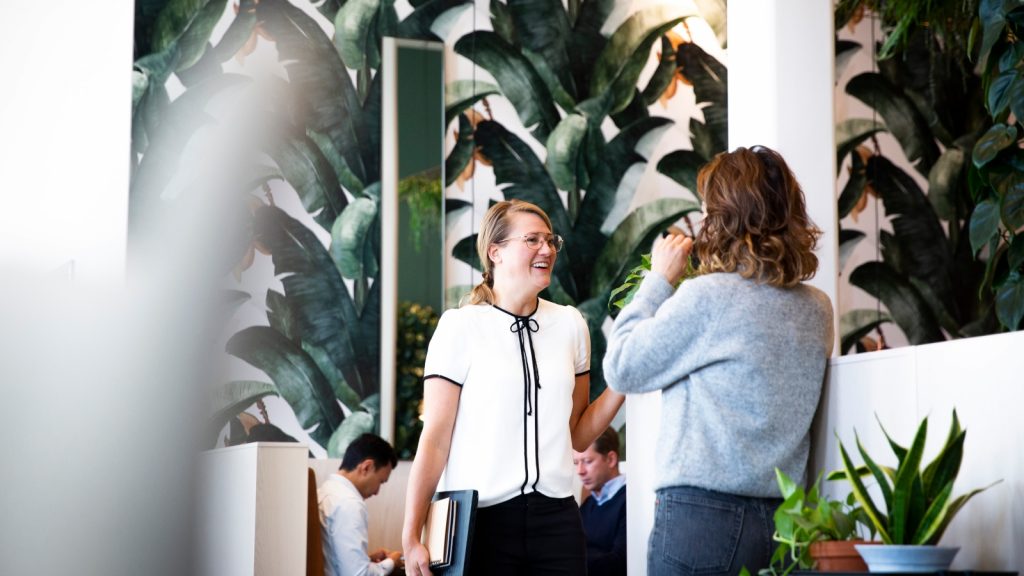
How the insurance sector is meeting ESG challenges
This article is part of the series covering the impact of sustainable finance on the insurance sector. Read further:Part 1: Assessing the impact of sustainable finance on insurance entitiesPart 3: Developing a toolkit for responsible investment decisions When taking environmental, social, and long-term asset portfolio issues into consideration, insurance companies must assess the specific risks […]
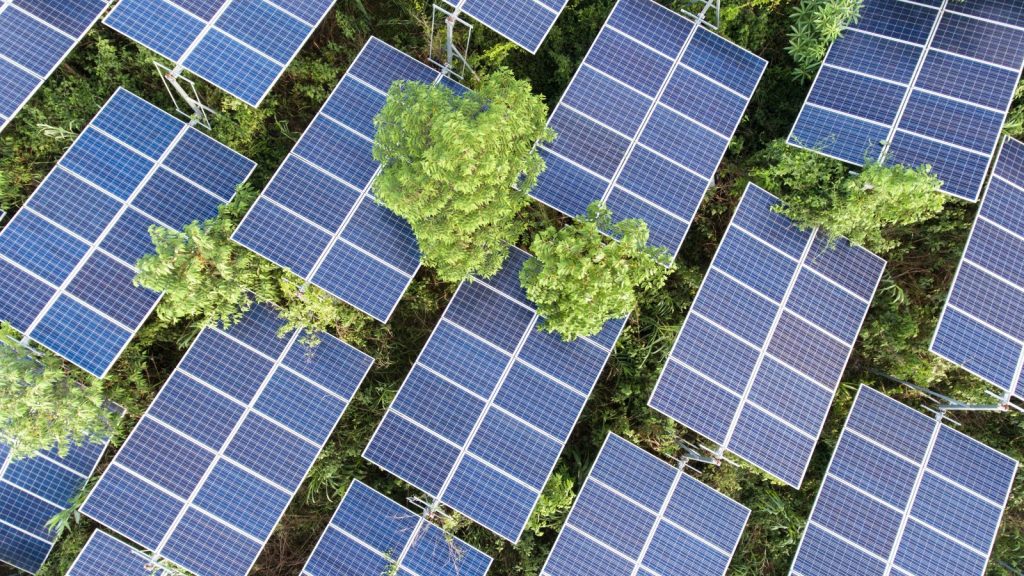
Assessing the impact of sustainable finance on insurance entities
This article is part of the series covering the impact of sustainable finance on the insurance sector. Read further:Part 2: How the insurance sector is meeting ESG challengesPart 3: Developing a toolkit for responsible investment decisions Amid a global pandemic and a rising threat of climate change, today’s society expects financial organisations to uphold strong […]
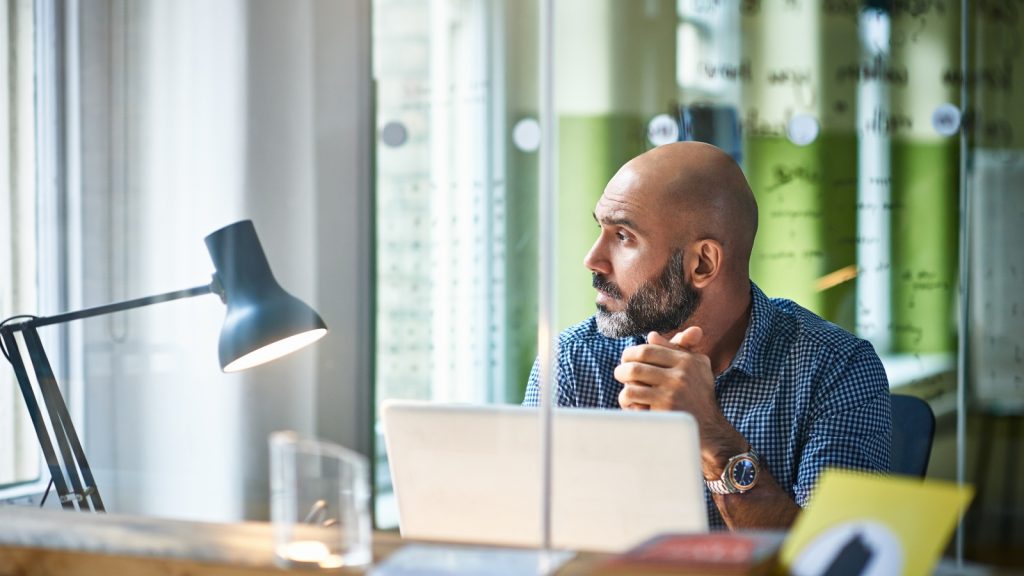
Reimagining the office in the work from home era
The working environment has always been an important factor for companies’ performance and their ability to attract talent. With the rise of remote work, this aspect has become critical: companies now have to ‘seduce’ staff to return to the office. Therefore, real estate services will play a crucial role in helping businesses upgrade working environments […]
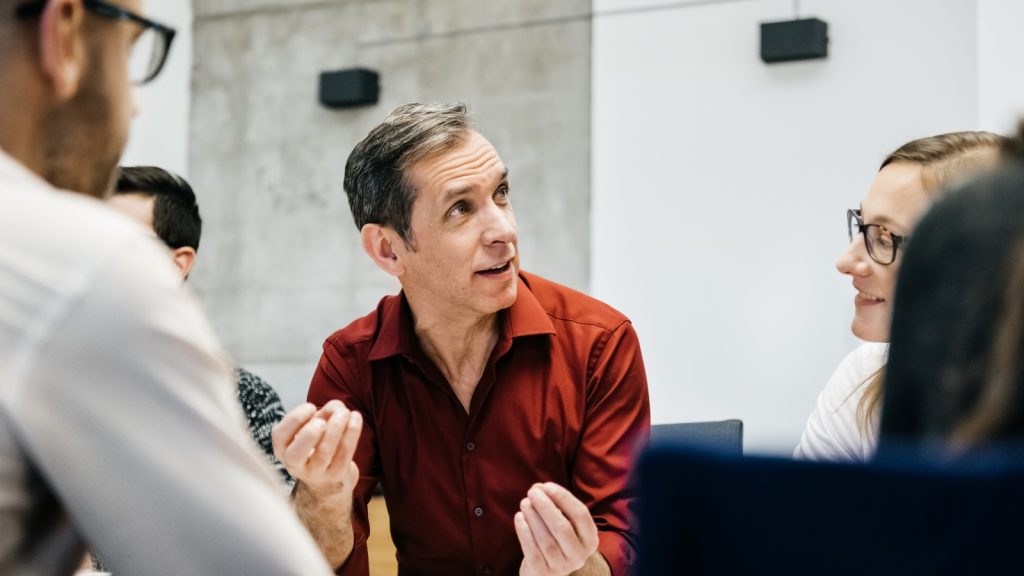
Maintaining Confidence in Real Estate Crowdfunding
The global real estate crowdfunding market was estimated at USD13.2bn in 2018 and is expected to reach USD868.9bn by 20271. In addition, the market is expected to grow at a compound annual growth rate (CAGR) of 58.3% from 2019 to 20271. In Europe, excluding the UK, real estate crowdfunding transaction value was USD600.1m in 20182. […]
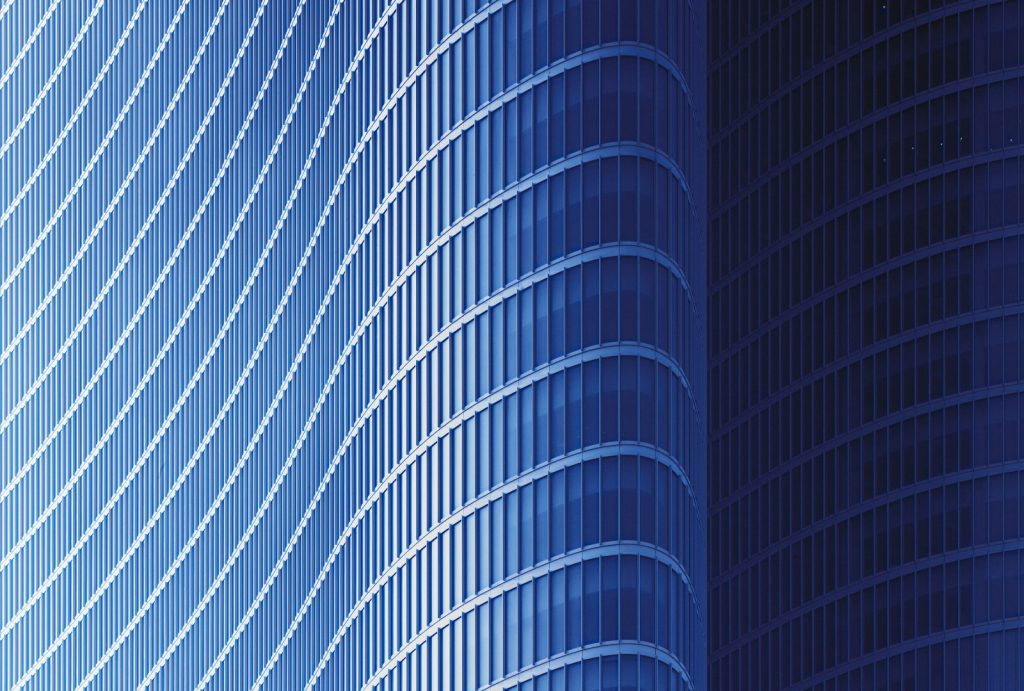
Mazars’ banking regulatory radar: 2020-2025
In this edition of our Banking Regulatory Radar, we cover the key regulatory developments in the banking sector for 2020-2025. The latest version of the Mazars’ Regulatory Radar has been updated with all the Level 2 legislation published in 2020, as well as the measures that were taken in the context of the Covid-19 pandemic. […]
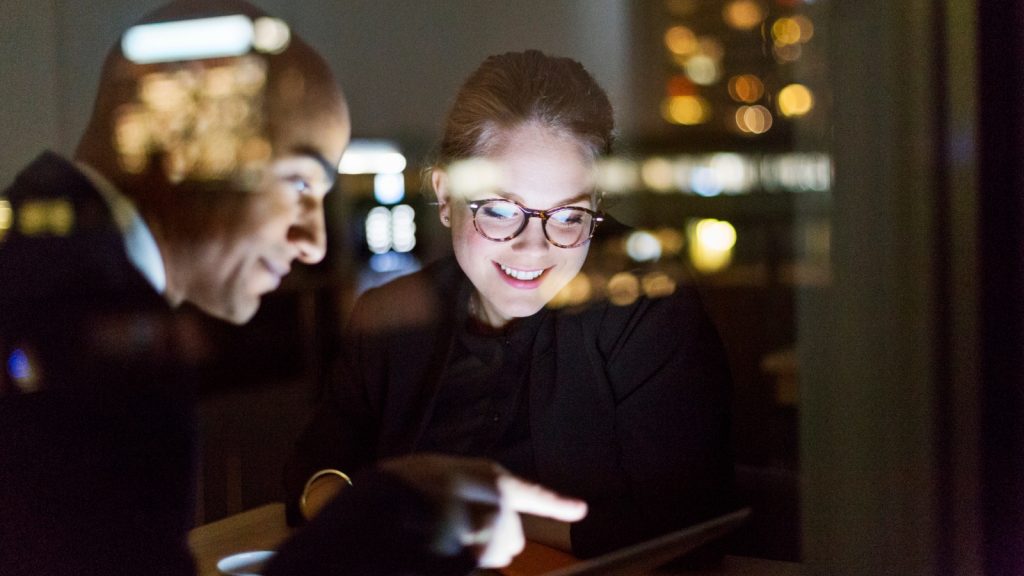
Can regulatory systems come to terms with Facebook’s stablecoin?
Facebook’s ambition to create a transferable global digital coin between users on the social media giant’s messaging platforms WhatsApp and Messenger has been controversial from the outset. Perhaps not surprisingly, the backlash from regulators around the world was substantial from day one. The world’s leading economies were less than enthusiastic of the possibility of a […]
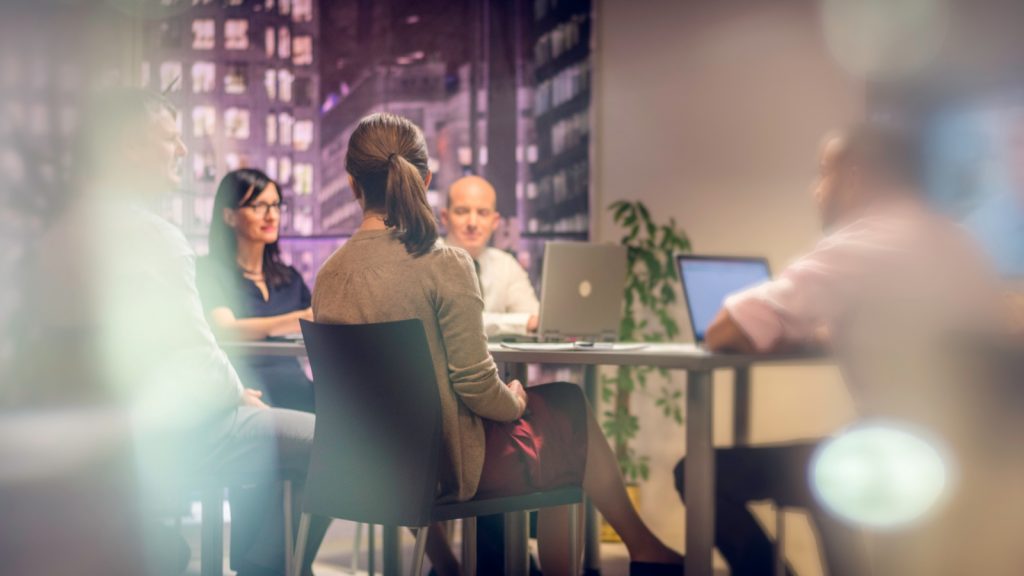
Why rethinking CSR is vital for real estate strategies
The pandemic has had a significant impact on our entire ecosystem. We have seen global CO2 emissions fall by a record 7% in 20201 benefitting the environment. Yet, at the same time, we have seen adverse consequences for the mental health of staff working remotely, with almost half (49%) of those working from home saying […]
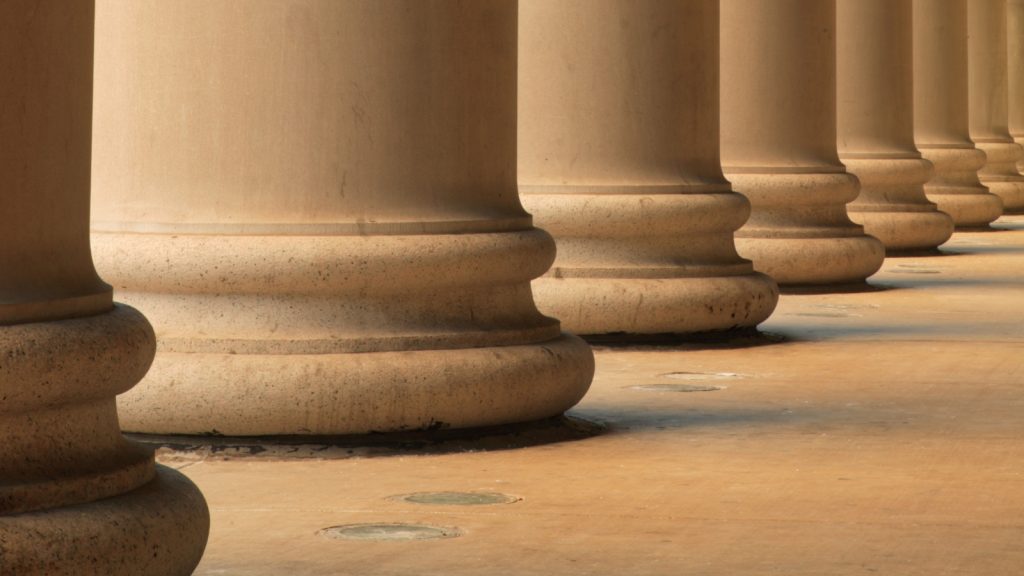
2021 Stress testing the UK banking system: the Bank of England’s approach
March 2020 marked the first time – since its inception in 2014 – that the Bank of England (BoE) cancelled its annual stress tests for the UK’s biggest lenders. Instead, they undertook a desktop analysis of the UK banking sector resilience. In late 2020, the Financial Policy Committee (FPC) judged that most banks have capital […]
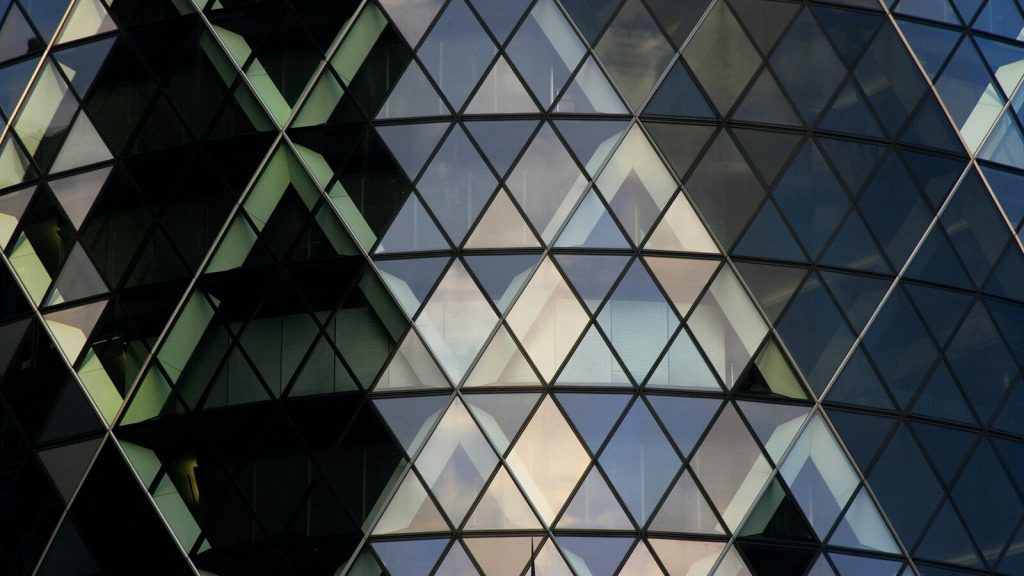
UK supervision of international banks post Brexit
Around one-fifth of global banking activity is undertaken in the UK. Almost half of the UK’s banking assets are held by international banks. The PRA currently supervises approximately 250 international banks, both branches and subsidiaries, which are part of around 180 international groups. Background On 11 January 2021, the PRA shared in a Consultation Paper […]
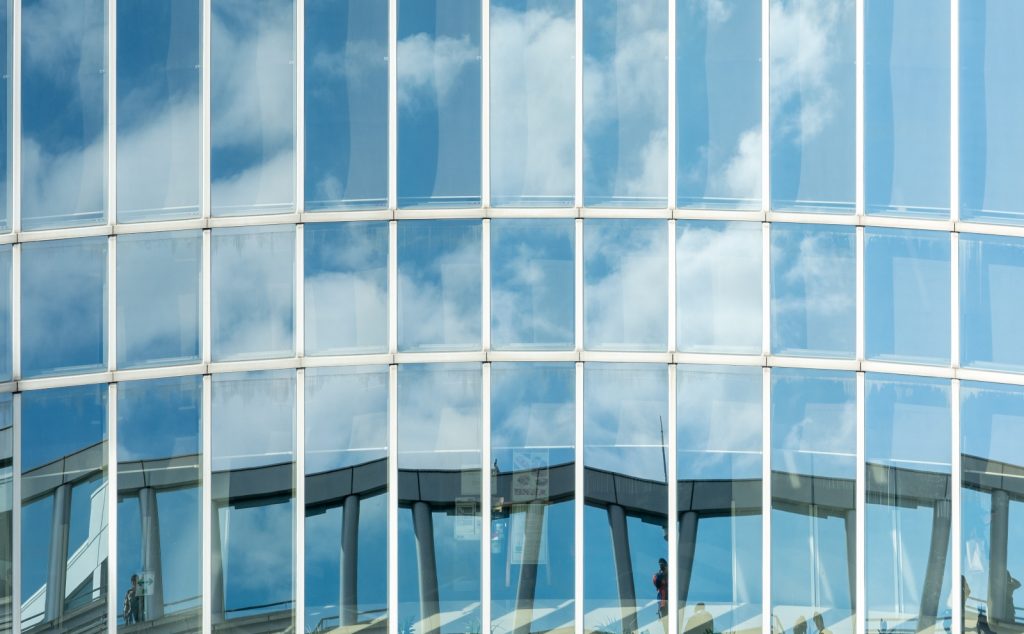
IBOR Transition: Modelling RFR term rates to price IR derivatives
One of the anticipated challenges in the transition from IBOR rates to risk-free rates (RFRs) is the management of its impact on quantitative models. The ones currently used for pricing IBOR-linked financial instruments account for term rates which are “forward-looking”. The RFRs replacing the IBORs are all overnight rates. This means that a term rate […]
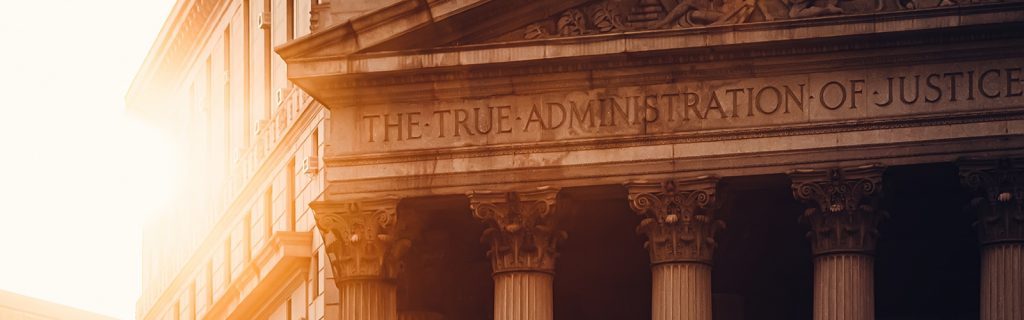
Reducing reporting burden for European banks while increasing data quality: a challenge for the EBA
Under article 430c of the updated Capital Requirements Regulation (CRR 2), the European Parliament and the Council of the European Union mandate that the European Banking Authority (EBA) perform a feasibility study on reducing the reporting burden for the European banking sector while ensuring data collection for monetary policy, resolution and supervisory purposes and take […]
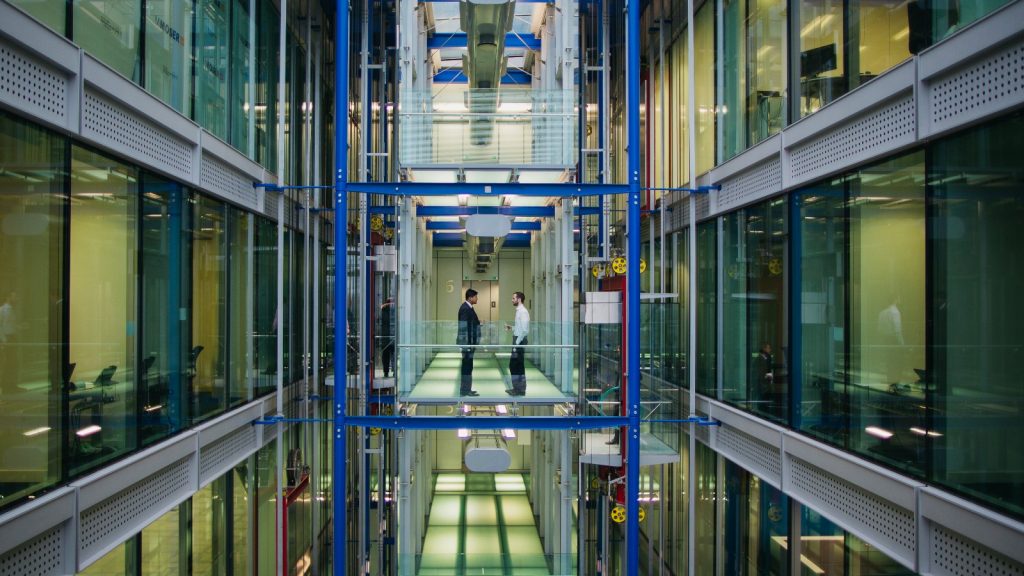
How the insurance industry can emerge stronger
There is little doubt that Covid-19 has had a significant impact on insurers, but there were already factors in play that were adding pressure on the insurance industry. What covid-19 has done is to create new challenges, as well as bringing existing challenges to the foreground. Looking ahead, the actions insurers take now to deal […]
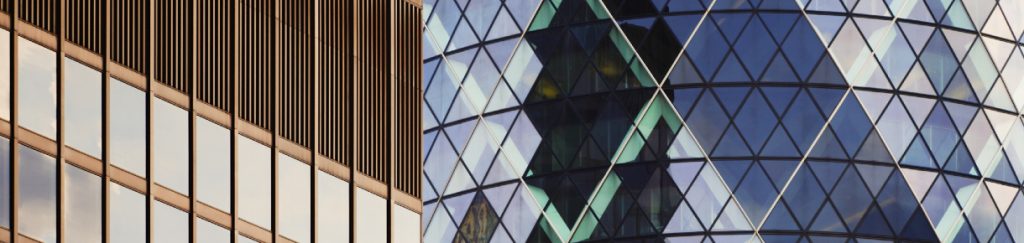
IBOR transition: impacts of the SOFR discounting switch
An important milestone in the IBOR transition is the change in rates used by LCH and CME for discounting and Price Alignment Interest (PAI) calculations for USD OTC cleared swaps. Indeed, on October 16, 2020, they moved from using the daily Effective Federal Funds Rate (Fed Funds) to the Secured Overnight Funding Rate (SOFR) for […]
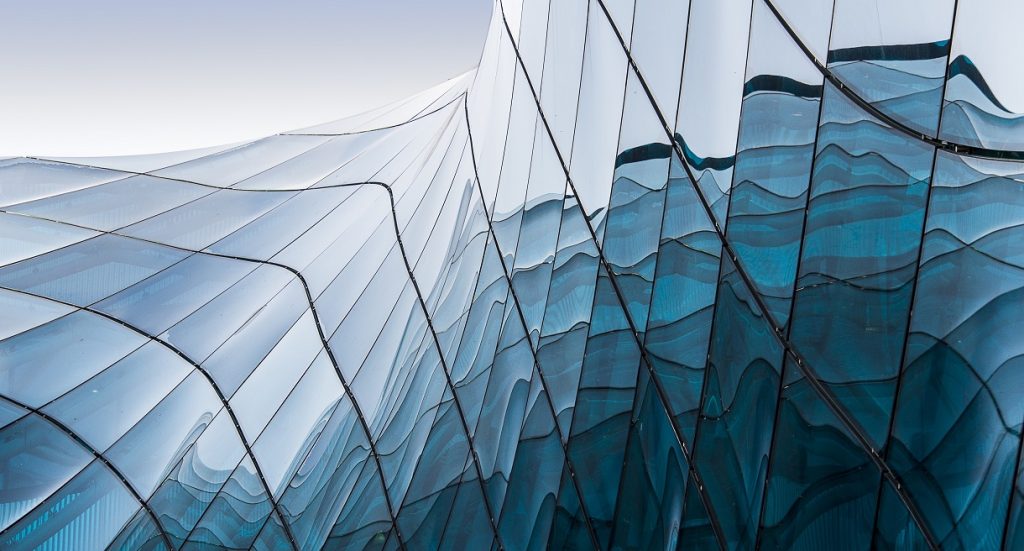
Addressing the challenges of the new sustainable finance regulations
As the world gears up for the transition to net-zero, the European Union is setting ambitious targets with respect to its own environmental footprint. For instance, by 2030 the EU is looking to reduce European greenhouse gas emissions by at least 55% compared to 1990 levels; increase the share of renewables within Europe’s total energy […]
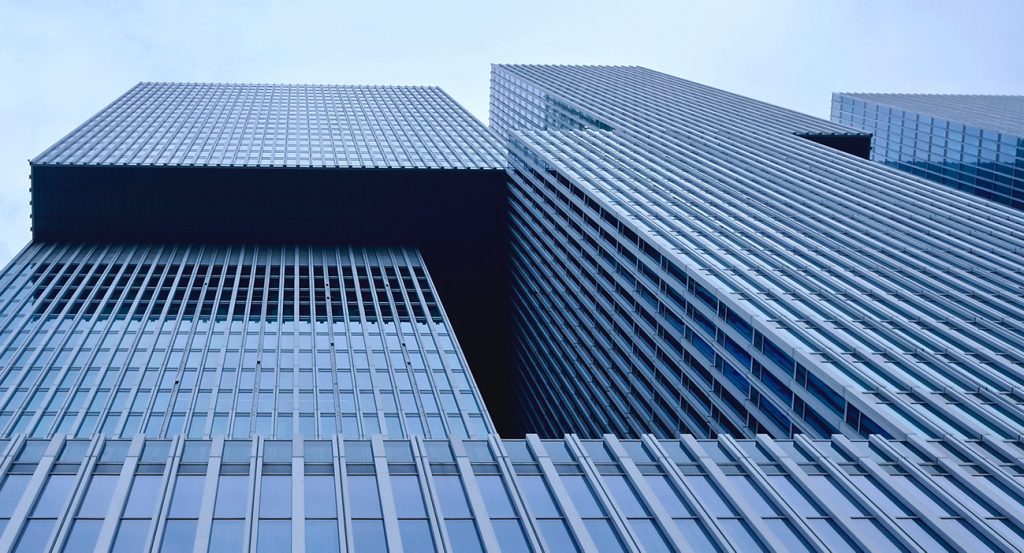
Bank stress tests – the post Covid agenda
In the early 1990s, stress tests became a popular internal tool for international banks to examine risks and gain a better understanding of threats to the institutions’ balance sheet. From there, the Basel Accord was amended in the mid- ’90s and required banks and investment firms to conduct stress tests. However, these were more internal […]
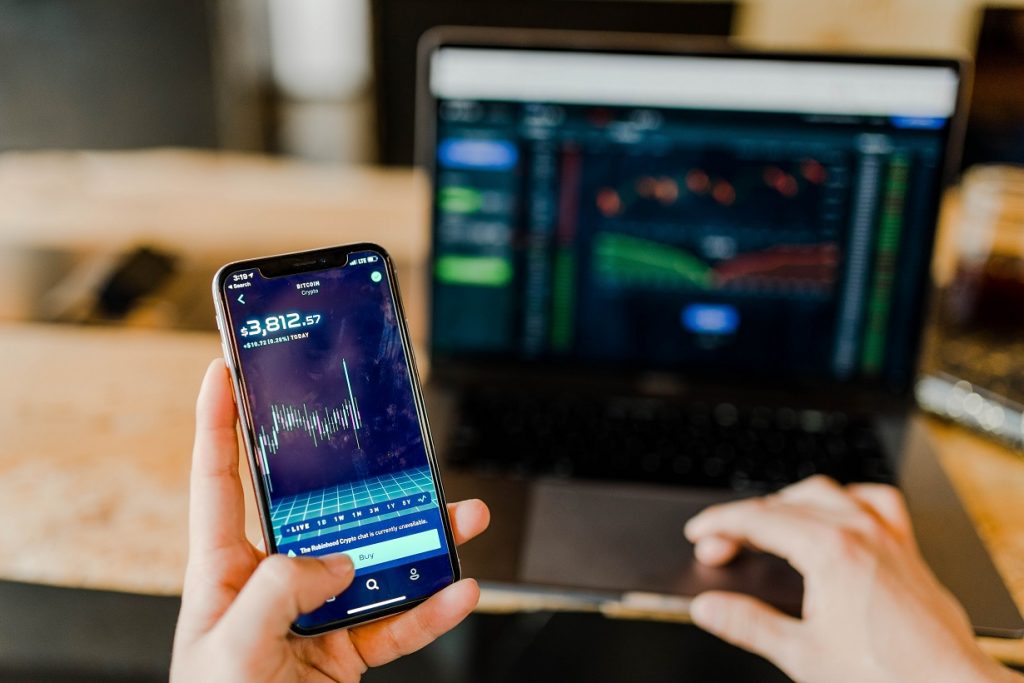
IBOR transition and FRTB cross dependencies highlighted
The revised market risk framework – also known as the Fundamental Review of the Trading Book (‘FRTB’) – not only impacts an institution’s regulatory capital charge calculation for market risk, but also affects operational, governance and business strategies. FRTB brings significant change. With the aim of harmonising capital standards for market risks across jurisdictions and […]
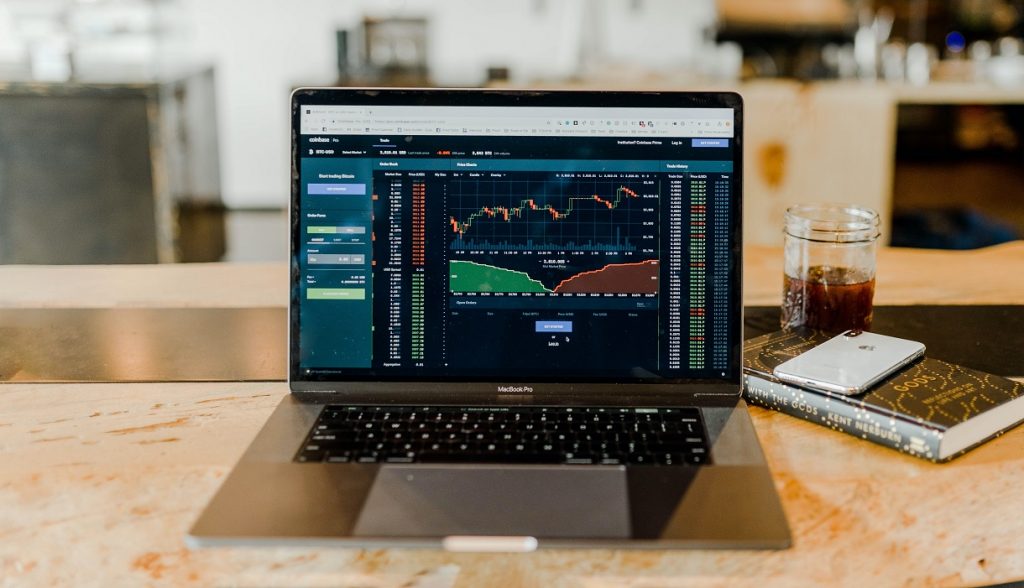
IBOR Transition: modelling of SOFR risk factors
One of the major challenges of IBOR transition is the availability of historical data on alternative risk-free rates (RFRs) required to implement interest rate model changes or re-calibration. With the Secured Overnight Financing Rate (SOFR) only published since April 2018, the available time series do not provide enough observations for risk modelling. Adding to that, […]
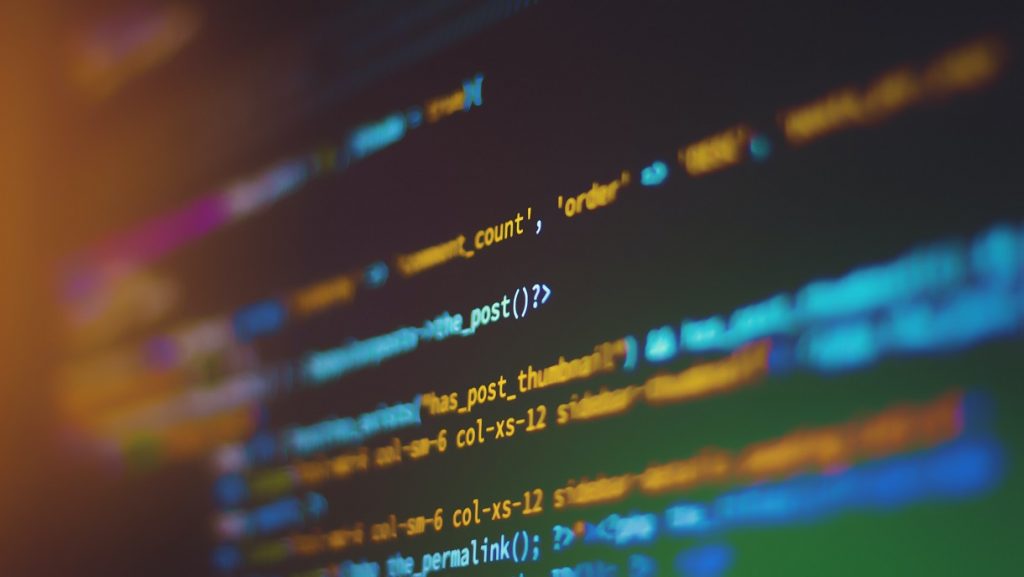
Acceleration in changing the prudential treatment for Software Assets: Covid-19 impact
Over recent years, technology and software have become strategic assets for competitiveness and resilience in the banking sector. Institutions have no choice but to invest to develop and deliver innovative services whilst managing ever greater IT and cybersecurity risks. The pandemic and announcement of lockdown measures posed a significant challenge for banks’ technology teams as […]
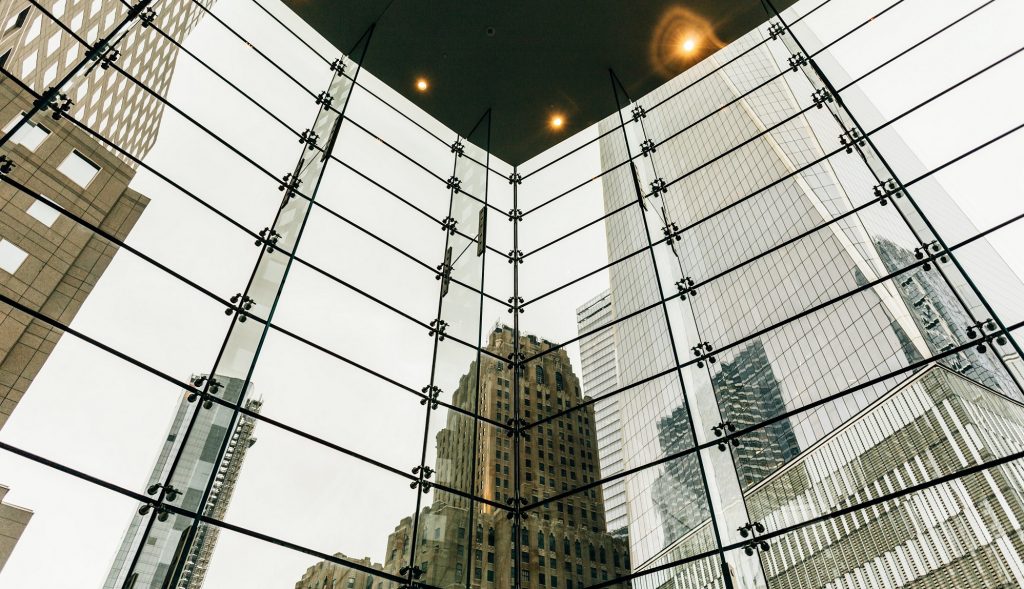
Federal reserve board publishes 2020 stress testing results and additional sensitivity analysis
The Federal Reserve Board released stress test results for DFAST 2020 including additional sensitivity analysis, considering the COVID19 outbreak, to assess the resiliency of large banks under three hypothetical recessions, or downside scenarios, that could result from the coronavirus event. Furthermore, the Board provides guidance for large banks to maintain resiliency during economic uncertainties from […]
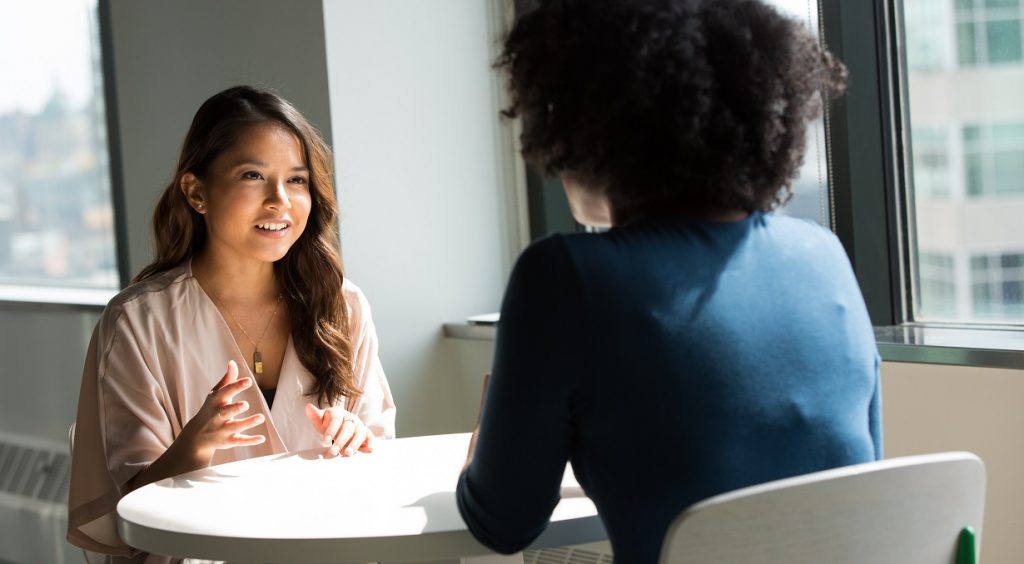
New measures by the European Central Bank aim to improve gender diversity
American entrepreneur, Malcolm Forbes, once described diversity as “the art of thinking independently together”. Today, diversity is beginning to emerge as a quintessential workforce norm and institutions have started to acknowledge the differences in their staff compositions that are deeply ingrained in the fabric of their organisational culture. With many challenges remaining, the independent think […]
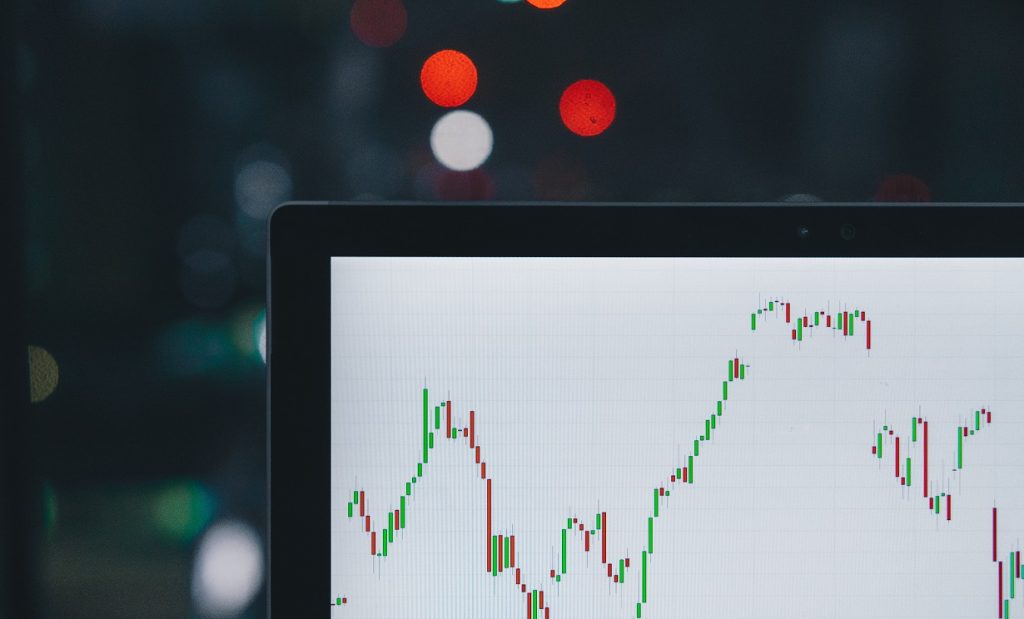
Is SOFR a strong enough USD LIBOR alternative?
With COVID-19, being declared a pandemic on March 11, 2020, financial institutions have had to shift most of their resources to mitigate the risks that have arisen. This has adversely affected important activities, one of which is market participants’ efforts to detach from LIBOR before its cessation at the end of 2021. As a result, […]
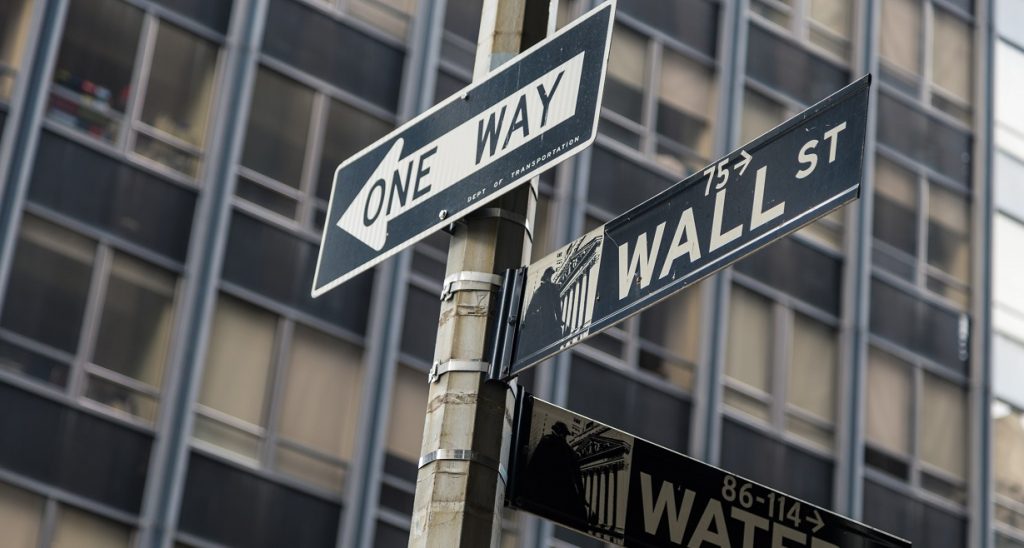
Covid-19 US policy changes: what banks need to know
Impacts from the COVID-19 pandemic have reverberated across every part of the global economy. Small businesses are struggling to pay their employees, banks are grappling with collapsing local economies, and many borrowers across the nation cannot meet their monthly mortgage payments. Banks will play a critical role in supporting their communities through this crisis, and […]
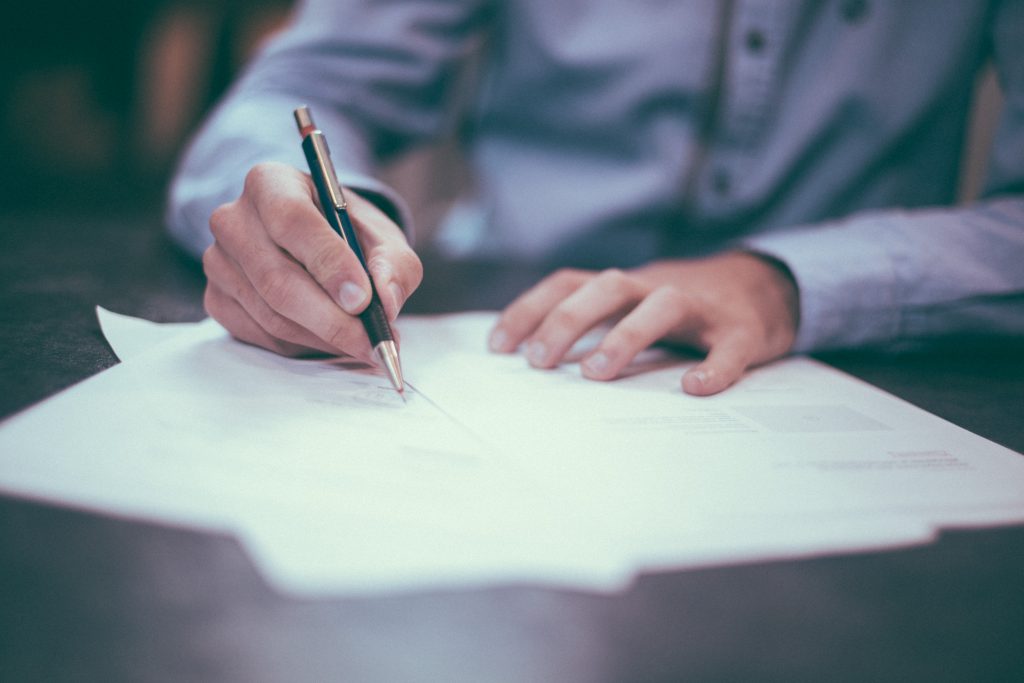
Are banks underestimating the risks of Covid Emergency Loans?
During the last few weeks, the volume of loans issued by banks has snowballed as governments release programmes to bail out businesses affected by Covid-19. As a result of these higher volumes, the exceptional increase in underwriting activity raises several issues for banks. Most notably, banks, like all commercial institutions, are also having to cope […]
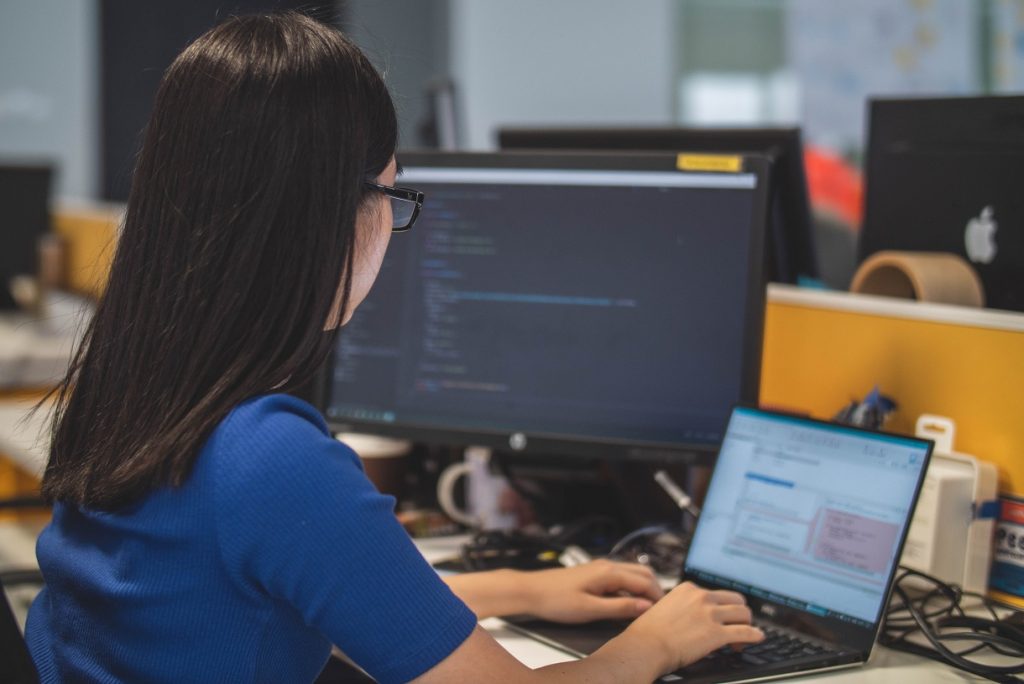
Are more stringent gender diversity measures required?
Gender equality, while not systematically embedded in national laws, is clearly set in European law. The Capital Requirements Regulation (CRR) requires financial institutions to adopt a policy promoting diversity within their management bodies and, for the most significant ones, to set targets to reach gender-balanced boards. Despite these regulatory requirements, the conclusions of the European […]
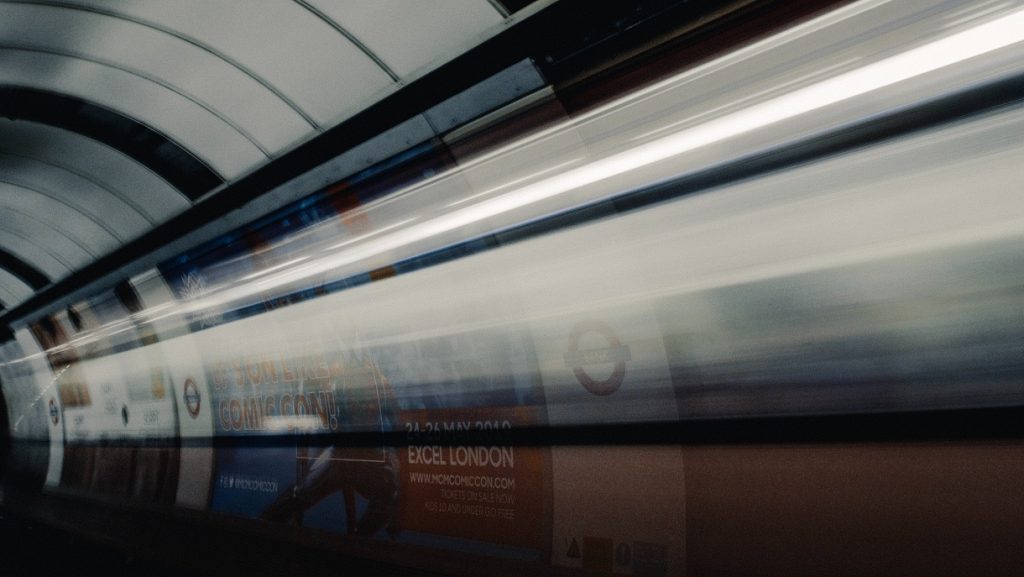
Progress on transitioning to SONIA
The Risk-Free Rates Working Group (RFRWG) published an update on the impact of COVID-19 on the timeline for firm’s plans to transition away from GBP LIBOR on the 29th April. While the central assumption of LIBOR’s publication being ceased after the end of 2021 remains intact, the Working Group has amended the timeline for the […]
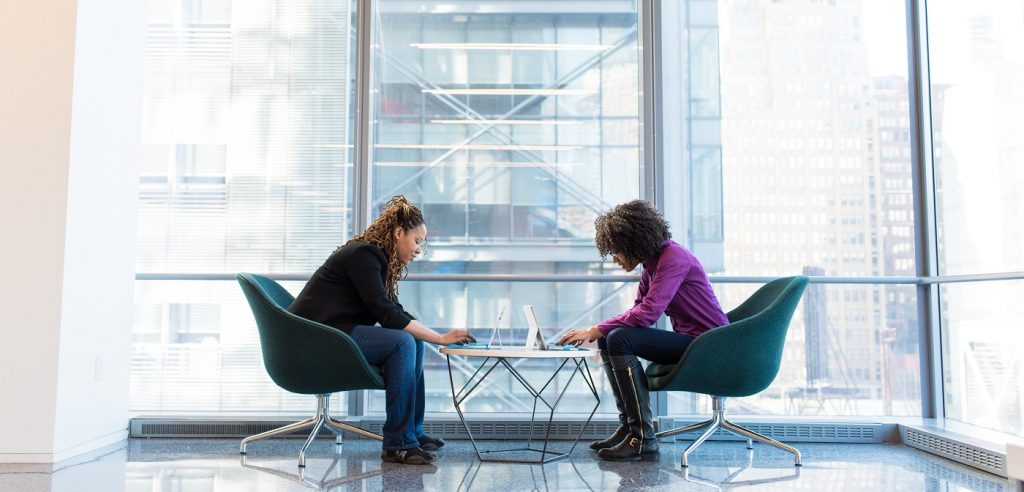
How banks can demonstrate responsible banking during the pandemic
What is the purpose of a bank? Does it have a responsibility to society at large, over and above its duties to its shareholders, customers and employees? The purpose of banks – to make a profit or be socially useful? What is the purpose of a bank? Does it have a responsibility to society at […]
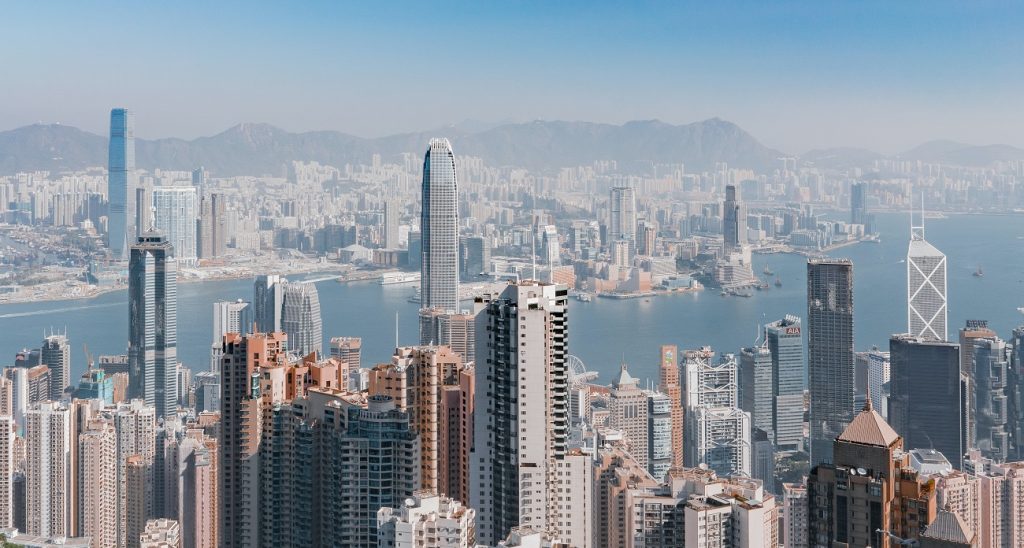
HKMA Support Measures and the Impact to the Banking Industry
Pierre Latrobe at Mazars discusses recent HKMA initiatives taken in response to Covid-19 and their implications for Hong Kong banks, highlighting credit risk as a growing threat. The Hong Kong economy has been confronted by several downside factors over the last two years. The first hit was the initiation of the US trade war with […]
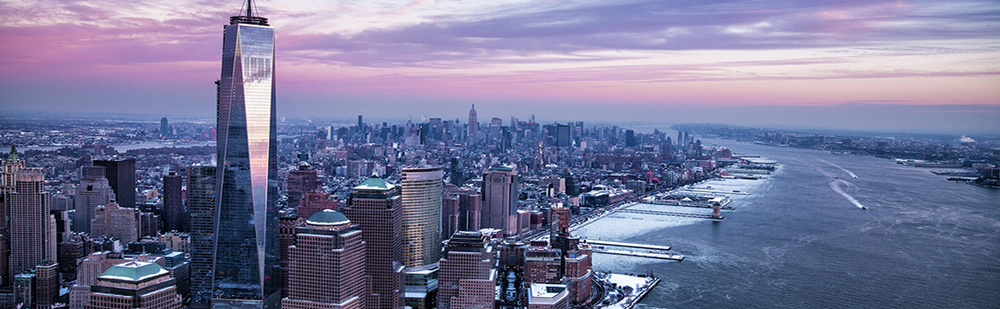
Key considerations on institutions’ credit IRB and IFRS 9 models
Mazars provides an update on recent developments affecting financial institutions’ credit capital and provision models with focus on the EBA IRB Roadmap and COVID-19 relief measures. Before the onset of the COVID-19 global pandemic, regulatory bodies across the Eurozone published guidance to financial institutions as part of the European Banking Authority’s (EBA) Basel III implementation […]
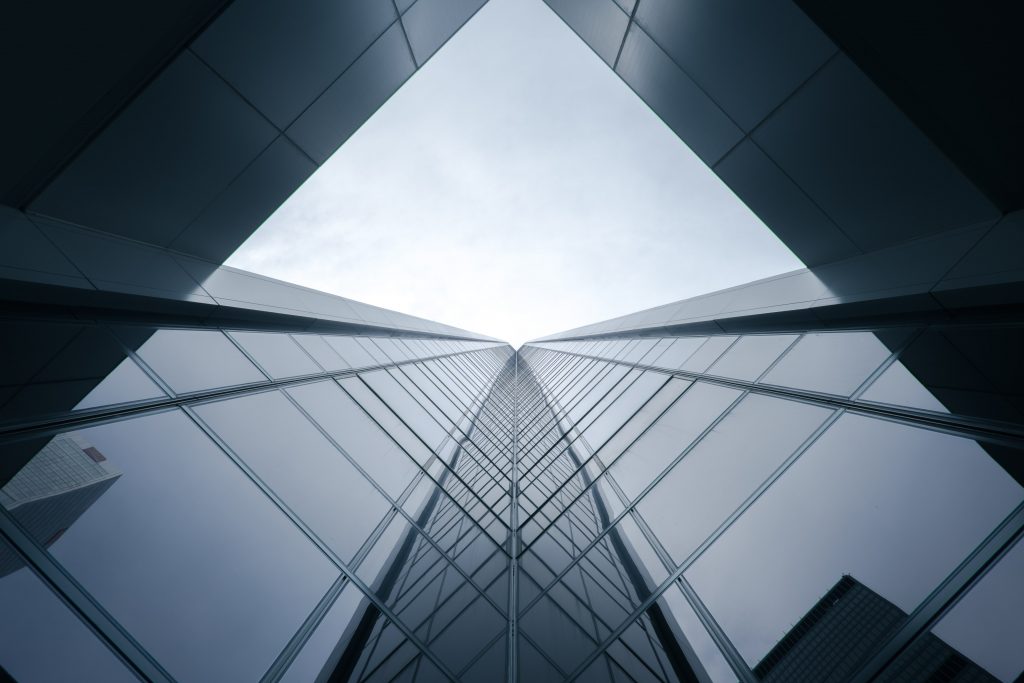
ARRC acts for a smooth IBOR transition
The Alternative Reference Rates Committee (ARRC) continues to support market participants in their efforts to transition from USD London Interbank Offered Rate (LIBOR) towards the Securities Overnight Reference Rate (SOFR). Following the Financial Conduct Authority’s (FCA) March 2020 statement that the expected LIBOR cessation deadline remains unaltered – i.e. end of 2021 – ARRC published […]
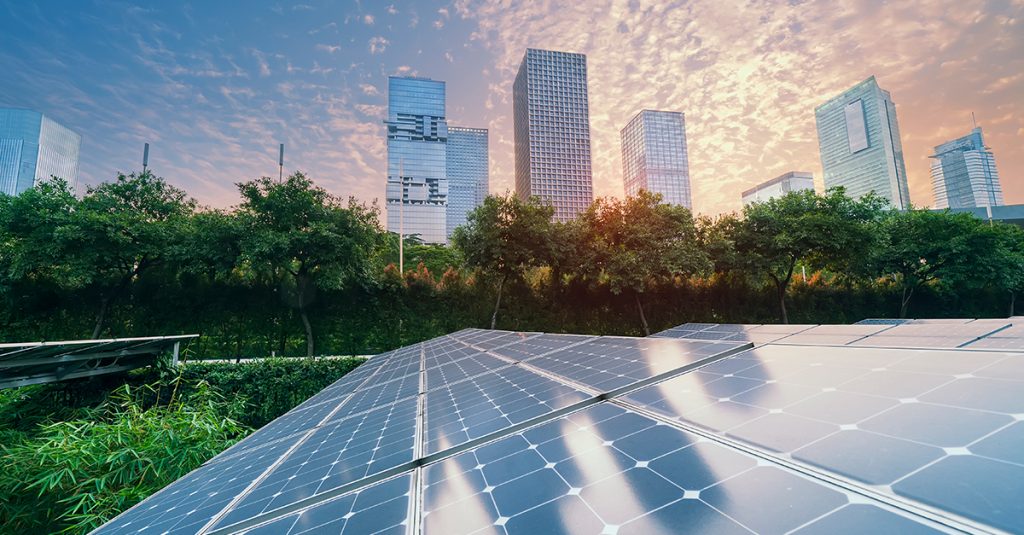
How will COVID-19 affect the financial regulatory response to climate change?
At first glance, regulatory authorities appear to have deprioritised the issue of climate change. However, a closer look would suggest otherwise and climate change in reality remains a key long-term priority of national and European regulators. In some areas, regulatory action on climate change has been delayed Central banks around the world have taken steps […]
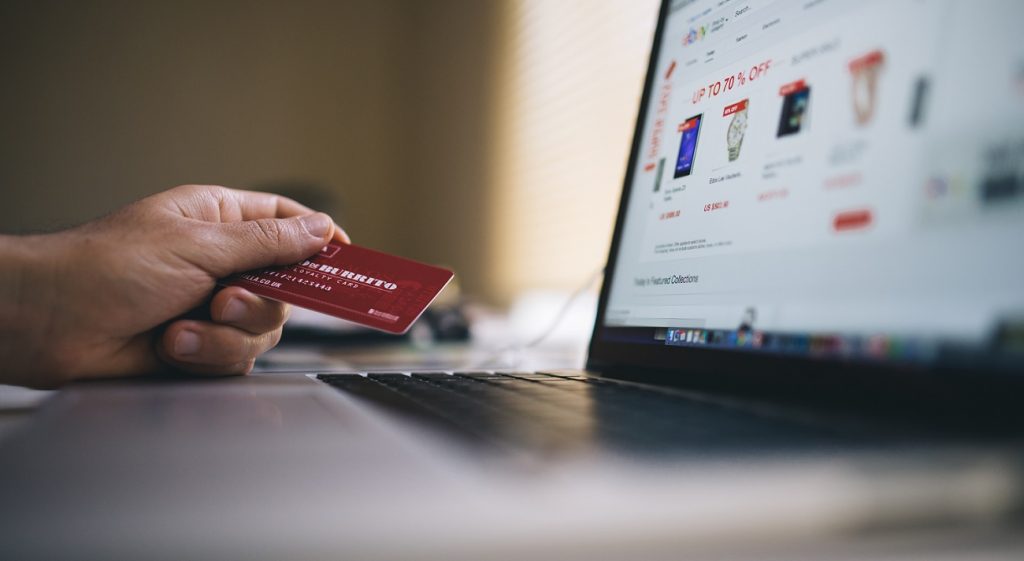
Rebuilding Credit Card Profitability post COVID-19
The current pandemic is having far reaching consequences across all aspects of society. Compared to other industries the impact on the credit card industry is relatively mild and from a customer perspective the value of on-demand liquidity is now clearer than ever. However, there will be significant impacts on industry profitability. Reduced international travel will […]
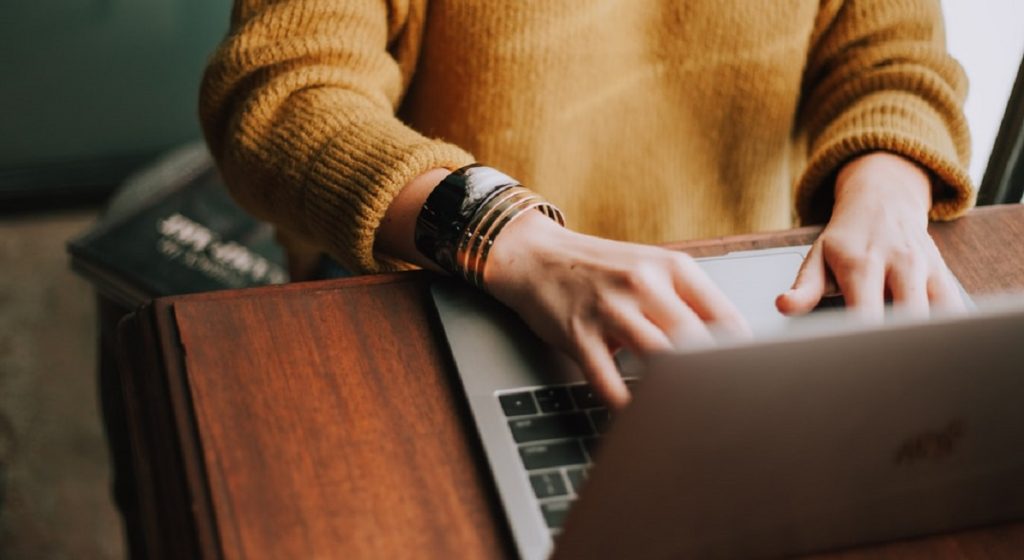
How banks can prepare for life after containment
Covid-19 disruption to the banking sector is widespread, including changes to working patterns, changes in customer behaviour, changes to partner-supplier dynamics and direct impacts on profit and loss accounts. The phase of immediate action to ensure business continuity is now largely complete. As infection curves flatten, restrictions are gradually eased and light starts to emerge […]
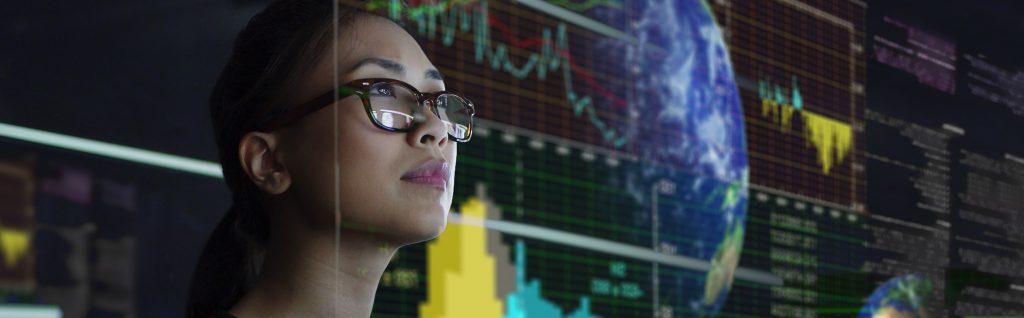
Regulatory flexibility gives banks the tools to support the economy during the Covid-19 pandemic
With banks no longer the weak link in the financial system, they now have a key role to play in supporting the real economy to survive the crisis caused by the Covid-19 pandemic. The significant strengthening of prudential regulation over the past decade since the 2008 financial crisis has enabled banking institutions to post solid levels […]
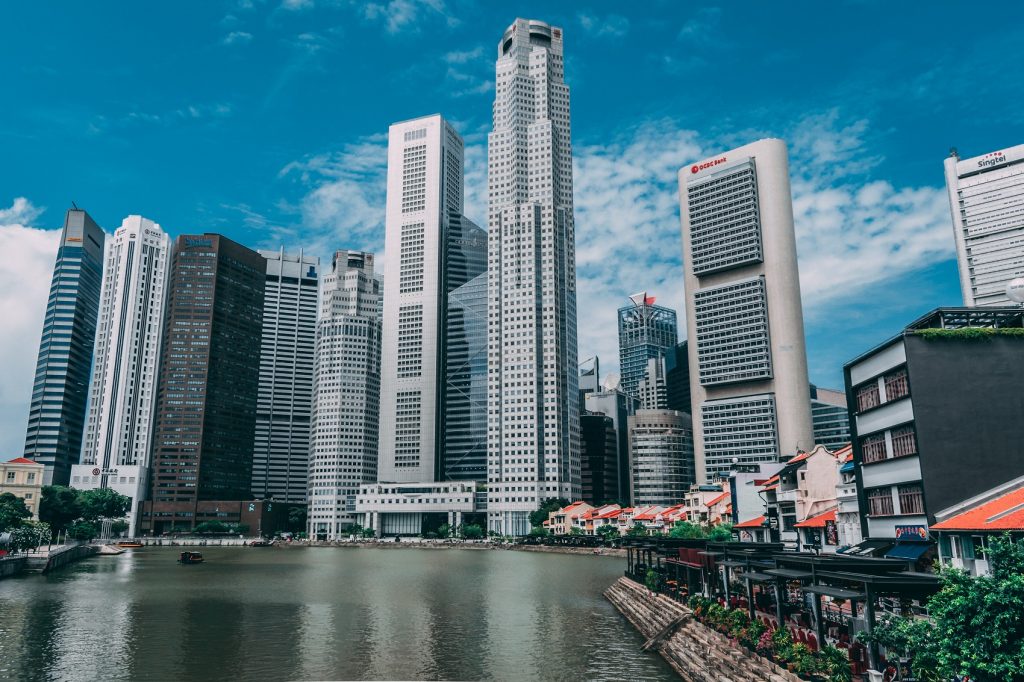
Covid-19: Major risk considerations for the banking sector
As we continue to feel the effects of the global pandemic, the banking sector, like many other sectors, now faces unprecedented uncertainty about the economic outlook ahead. While banks go into this pandemic in a stronger position than the global financial crisis of 2008, the current environment presents particular challenges and disruption to standard accounting […]
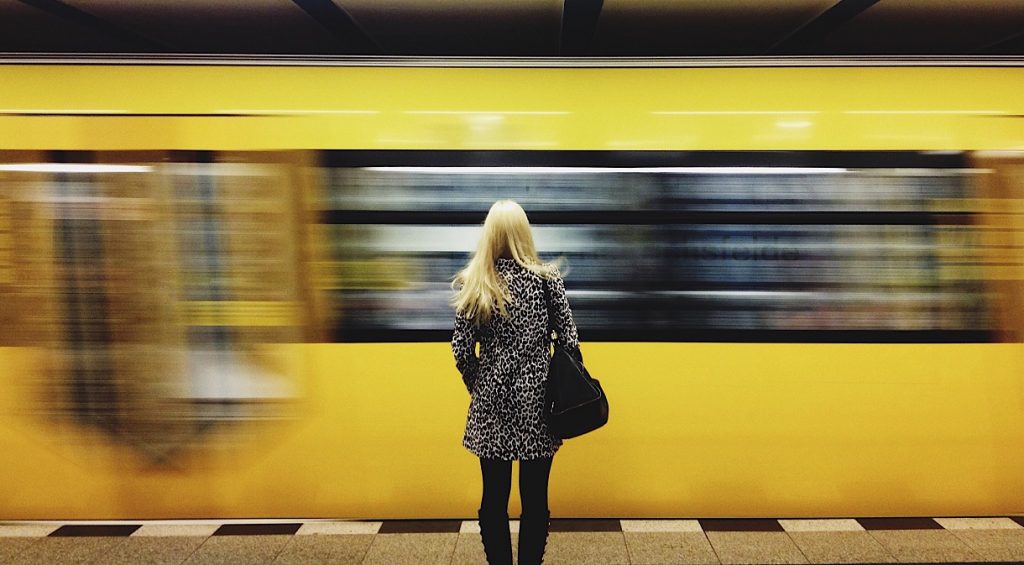
COVID-19: Phase 1 of SFTR delayed
The European Securities and Markets Authority (ESMA) has issued a public statement to announce the delay of the industry’s compliance with phase one of the Securities Financing Transactions Regulation (SFTR). This is in response to ESMA’s awareness of the financial industry’s struggle to devote resources to comply with the new reporting obligation, as firms face […]
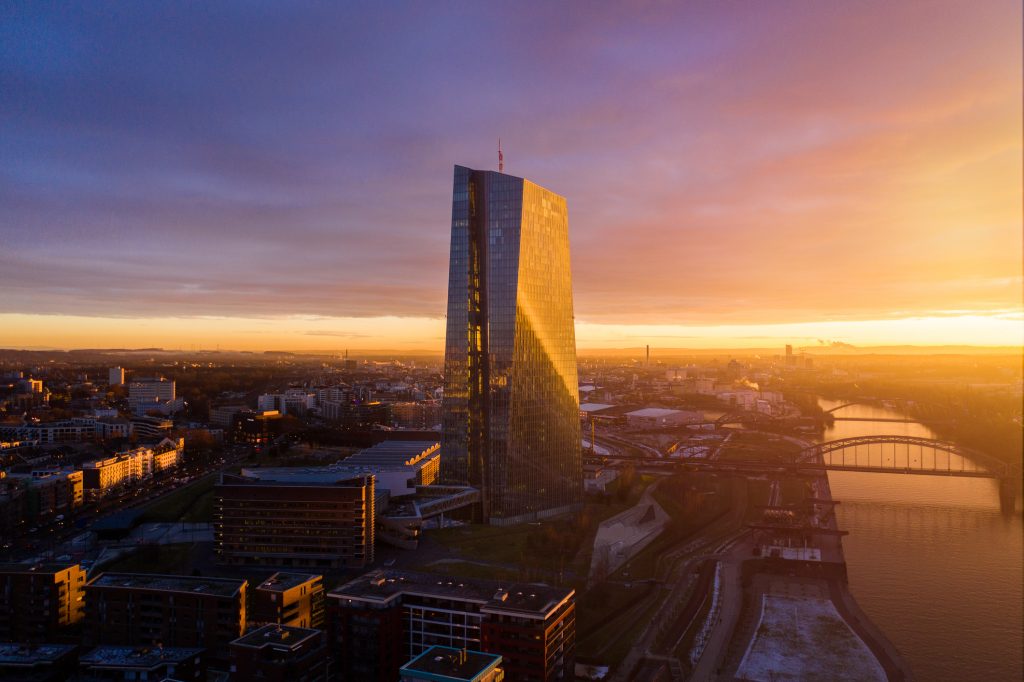
COVID-19, banks and regulation: the road ahead in the UK and Europe
The Covid-19 outbreak and the unprecedented emergency it presents has created a unique threat to the world’s economy. Like all sectors, banking has been impacted, and its stakeholders have felt excessive pressure over the last few weeks to get things right. Regulators in financial markets around the globe have all announced Covid-19 action plans, which […]
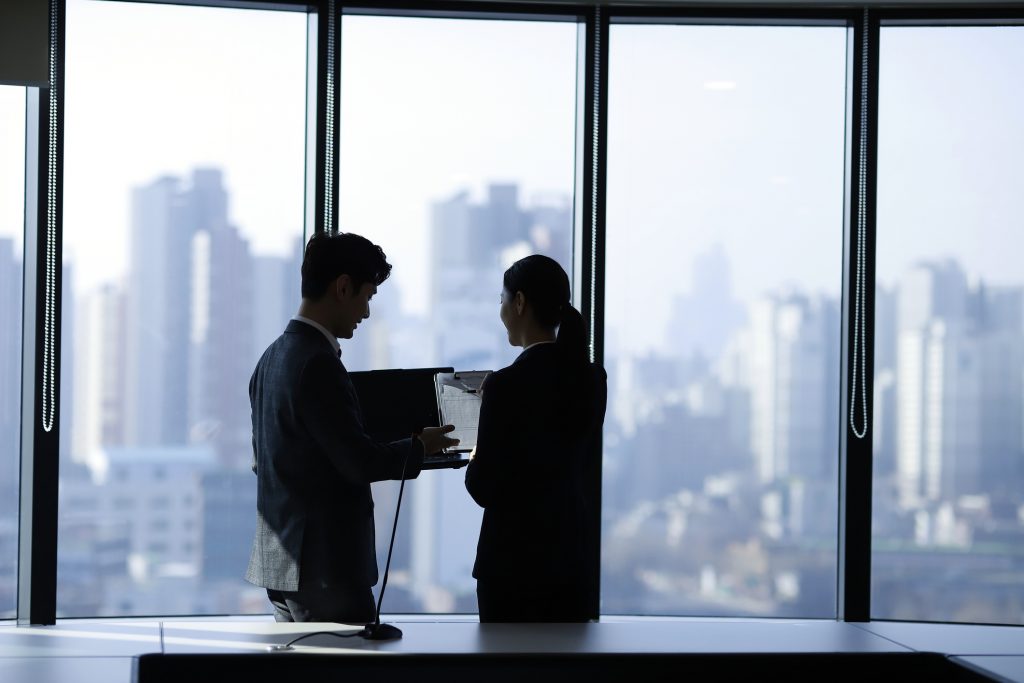
IBOR transition: Fallback language developments
The expected 2021 disappearance of LIBOR requires robust fallback language for cash products and derivatives alike. Industry associations have taken initiatives to reform the historic fallback language of securities, with ISDA proactively leading the way on derivatives and national working groups proposing enhancements for cash products. While the derivatives market is expected to be harmonized […]
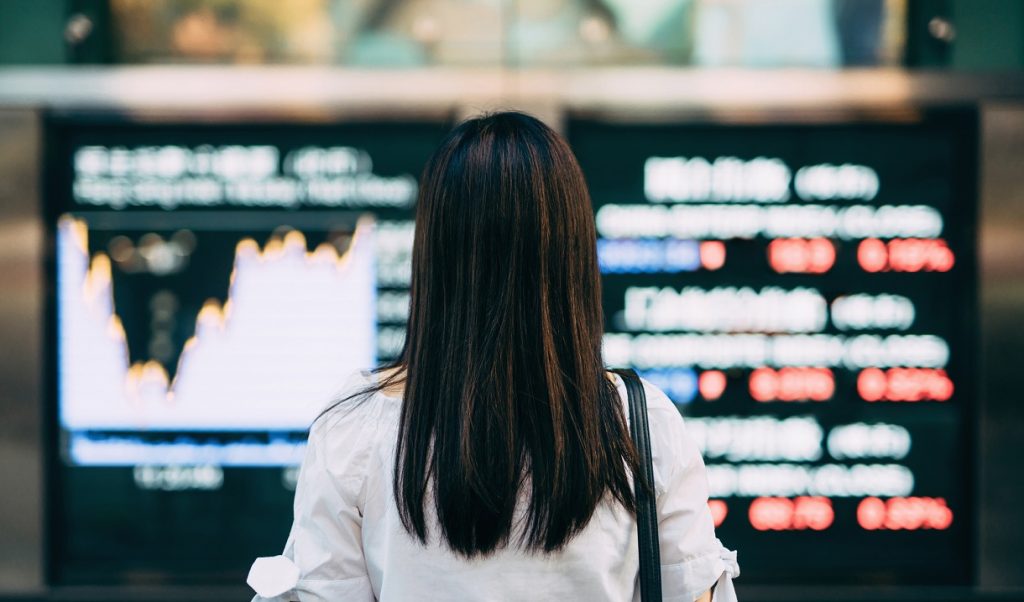
Do Asian market Libor preparations pose systemic risk to world markets?
Since Libor was first used in financial markets in 1986, it has become the foundation of the global interbank funding market. However, regulators ruled that Libor’s volatility during the last global financial crisis (GFC) and a rate-rigging crisis in 20121 involving the world’s largest banks exposed a fundamental weakness with the rate’s publication methodology. Yet, […]
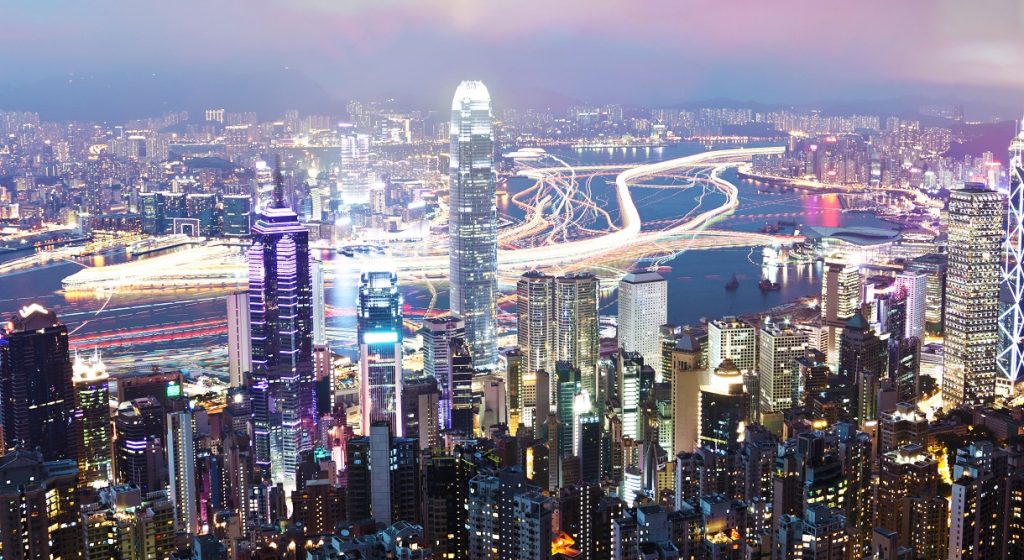
Study highlights significant variations in HKFRS 9 reporting practices
One year on since the HKFRS 9 standard on financial instruments came into force in Hong Kong and two years since the First Time Application (FTA), trends and insights into impacts of the standard are beginning to evolve. As a reminder, the standard introduced numerous changes with regard to the classification, impairment recognition and the […]
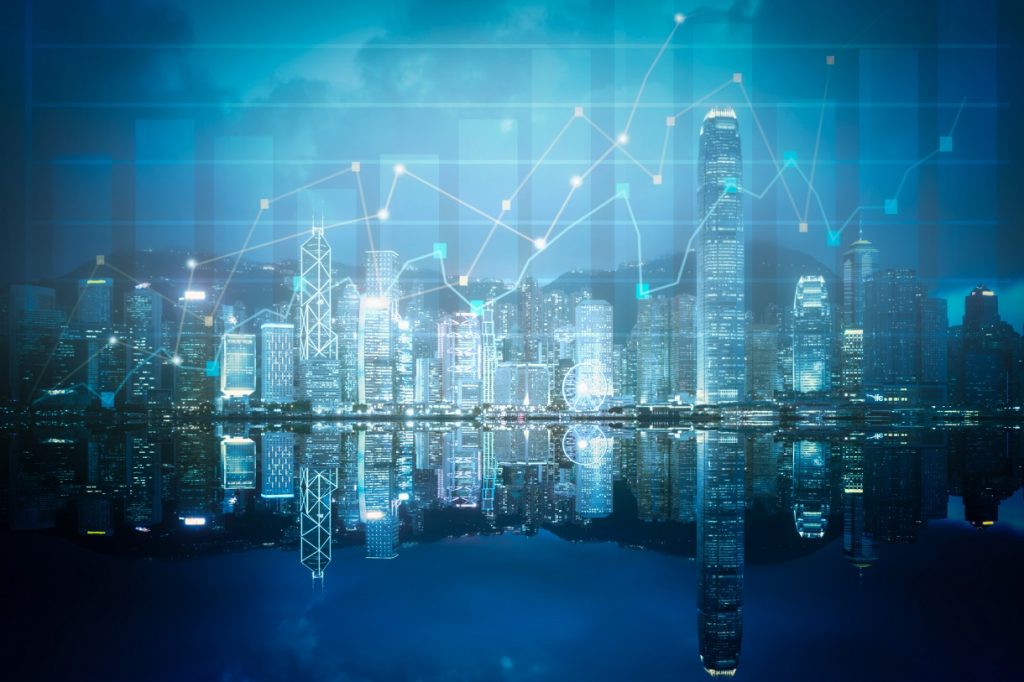
Re-engineering the banking sector
In recent years, disruption to the banking sector has seen an increasing number of partnerships between banks and FinTechs, as banks look to acquire the digital expertise now required for 21st century banking and FinTechs look to tap into the finance knowledge and consumer reach that traditional banks enjoy. More recently, this quest for technological […]
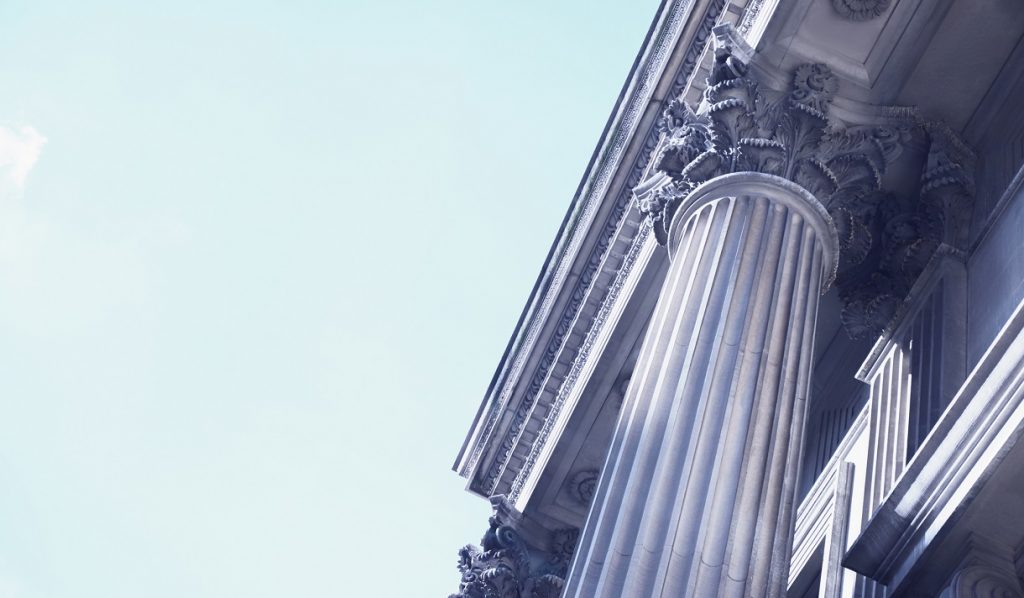
New prudential regulation for investment firms in Europe
At the end of nearly two years of legislative work, the reform of the prudential regulation of investment firms completed its final phase with the publication in the Official Journal of the European Union of two new regulatory texts: Regulation 2019/2033 on the prudential requirements of investment firms (IFR), and Directive 2019/2034 on the prudential […]
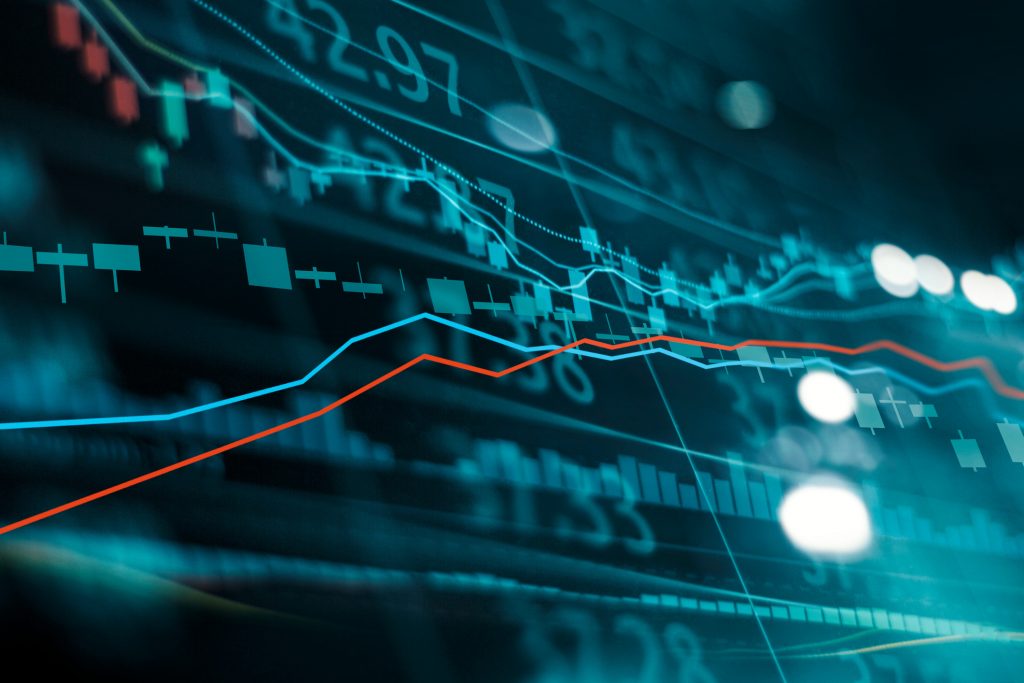
How to predict the results of P&L attribution tests in the FRTB framework?
Under the terms of the Fundamental Review of the Trading Book (FRTB), a bank wishing to apply the Internal Modal Approach (IMA) to calculate its capital charge associated with market risk must carry out: backtesting on the Trading Book (TB) and for each trading desk (TD), two profit and loss attribution (PLA) tests for each […]
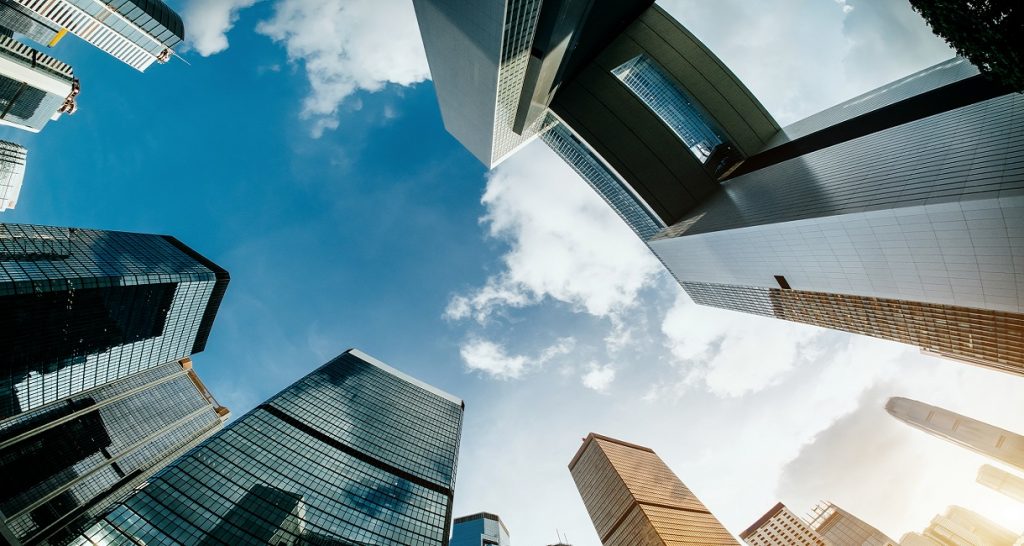
Is Asia on its way to IBOR transition?
With Libor’s cessation date at the end of 2021 looming, global regulators are hastening their IBOR fallback strategies. Yet while market momentum has increased for multiple published RFR indices, among them the GBP SONIA, the EUR €STR, and the USD SOFR, Asian economies, some of which rank among the world’s largest, continue to lag. While […]
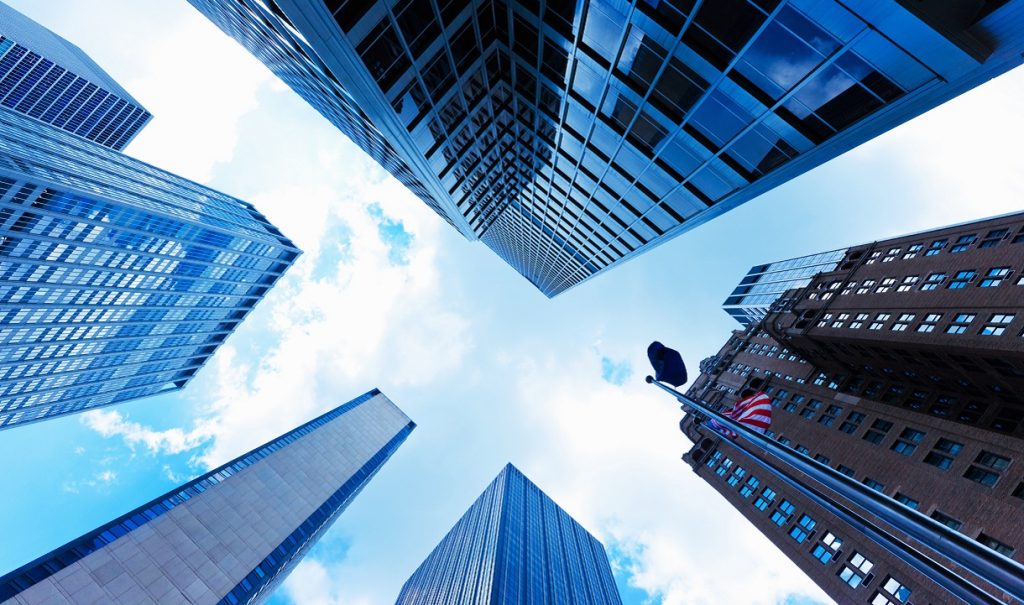
Is SOFR the ultimate replacement for USD LIBOR?
Financial market participants – at least the largest ones – are actively preparing for the expected discontinuation of the London Inter-Bank Offered Rate (LIBOR) after 2021. Transitioning towards a LIBOR-free world is a challenge that requires the involvement and coordination of the whole industry in order to find appropriate solutions to replace the 35 different […]
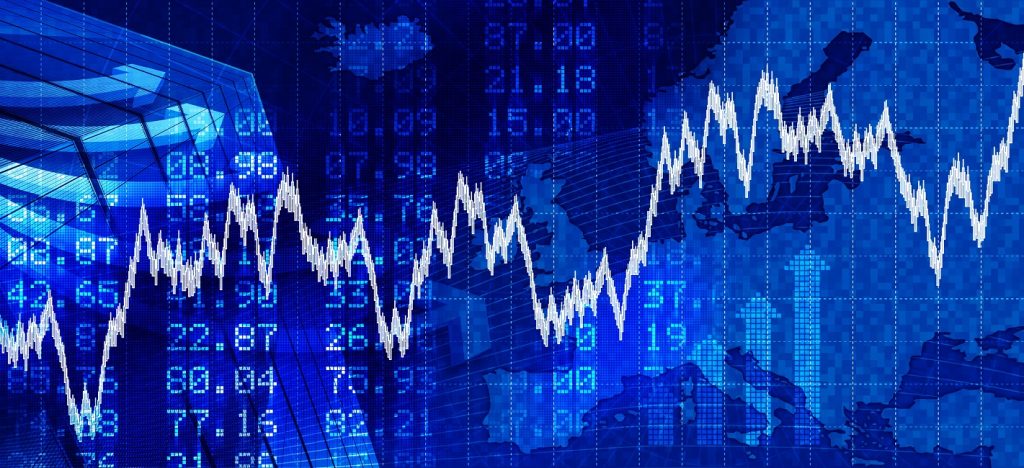
EBA’s Stress Test 2020 Methodology – What’s new for the banks?
The methodology for an exercise to assess the resilience of EU banks to adverse market conditions and test the state of their capital allocations has been released by the European Banking Authority (EBA). The exercise – part of EU-wide stress testing – will apply to broadly 70% of the European banking sector[1]. Some 52 banks […]
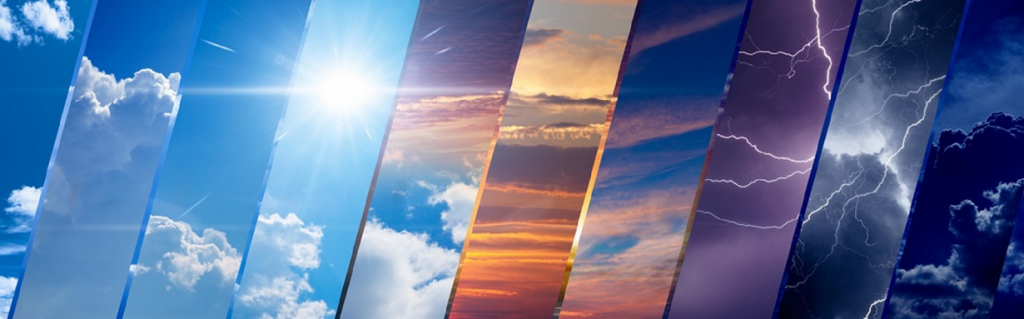
A looming climate crisis?
Persistant negative interest rates, the inherent risk of a trade war between China and the United States, fears of a recession… all worrying signs of an imminent new crisis. However, the real question is not if but when the next crisis will hit. More than ten years after the financial and sovereign debt crisis, it […]
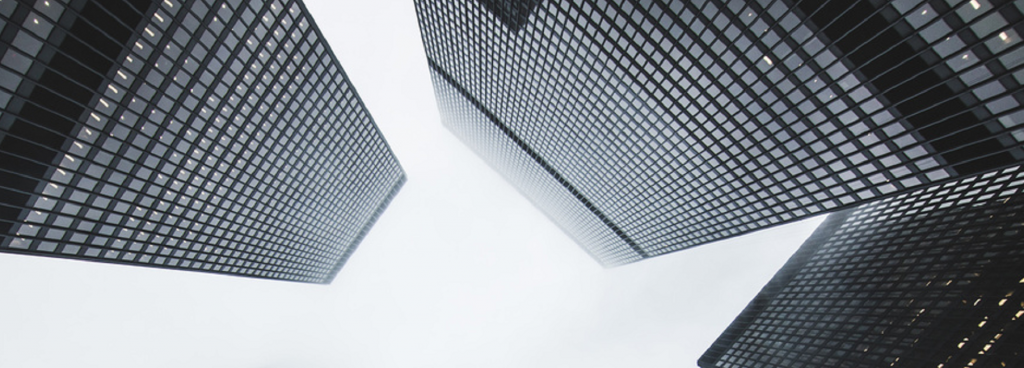
Conduct Risk should not be underestimated during IBOR Transition
With little more than two years to go, Libor’s cessation date continues to near. The voluntary agreement of panel banks submitting to Libor will conclude at the end of 2021, from which risk-free rates (RFR) are expected to replace Libor and similar indices. Are corporates paying enough attention to Libor updates? Libor’s cessation should be […]
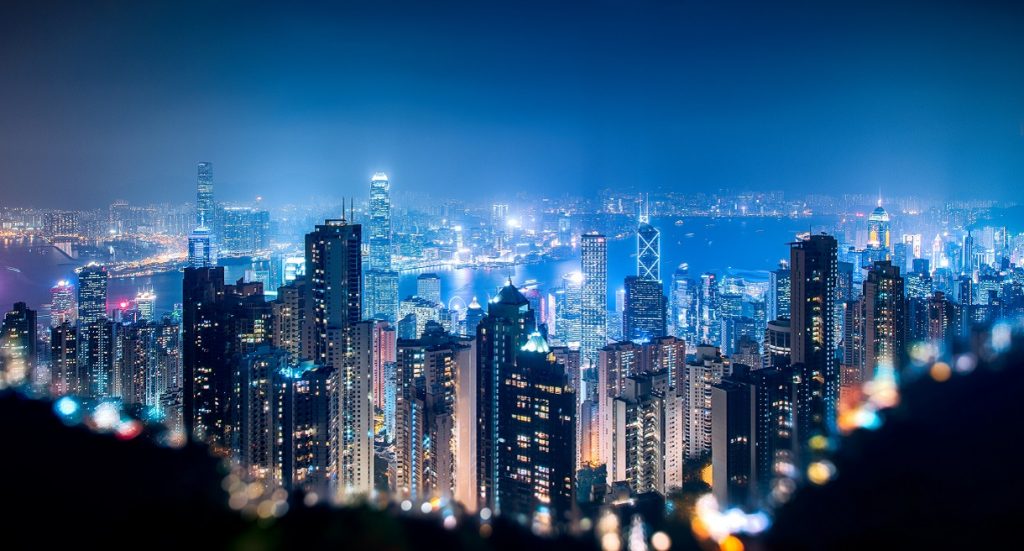
The Supervisory Answer to Hong Kong’s Worsening Economic Performance
Pierre Latrobe at Mazars discusses the measures the HKMA has taken so far to strengthen its macroprudential supervisory toolkit and address potential risks to the wider financial system. The Hong Kong economy is suffering from several lingering negative factors, the US-China trade war, the global economic slowdown and the ongoing protests, to name but a […]
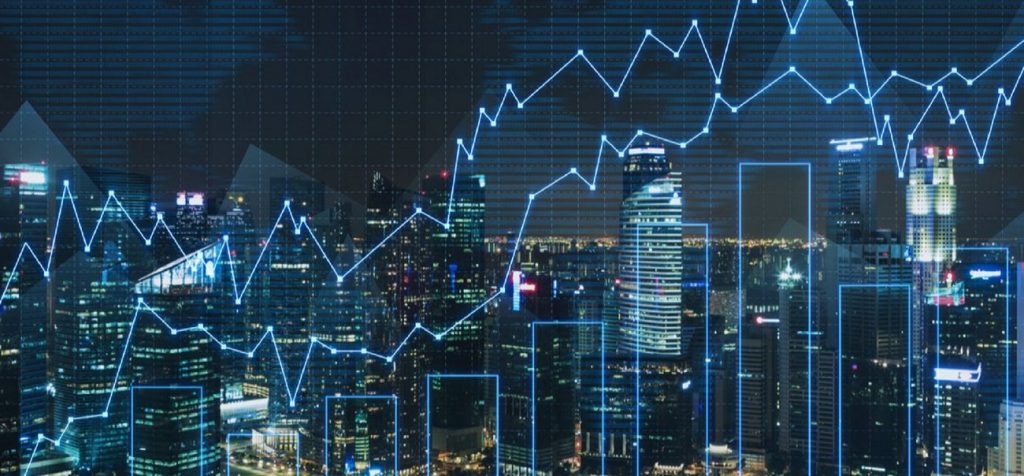
IBOR Reform – key takeaways
With significant IBOR reform on the horizon, Mazars brought together industry experts, practitioners and regulators to discuss the challenges and opportunities they face. Speakers included the Bank of England Market Division’s Alastair Hughes, EFRAG’s Didier Andries and Mazars’ IBOR lead, Pauline Pelissier. From a comprehensive and illuminating session, Pauline sums up the key takeaways: “What […]
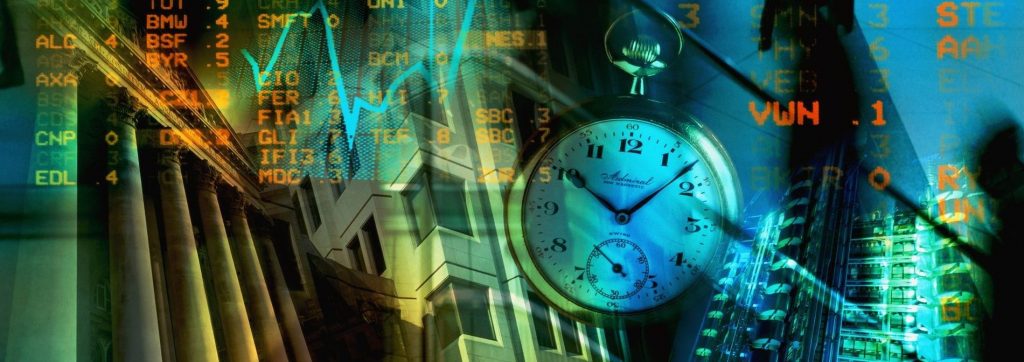
Brexit Watch #6: Seeing past the fog of uncertainty – How are the regulators responding?
The British political landscape has been unsettled and uncertain. Boris Johnson defeated Theresa May in July 2019 to become Prime Minister with his “do or die” conviction for the UK’s exit from the EU, with or without a deal. While the Government has been successful in achieving Parliament’s support for a renegotiated withdrawal deal, MPs […]
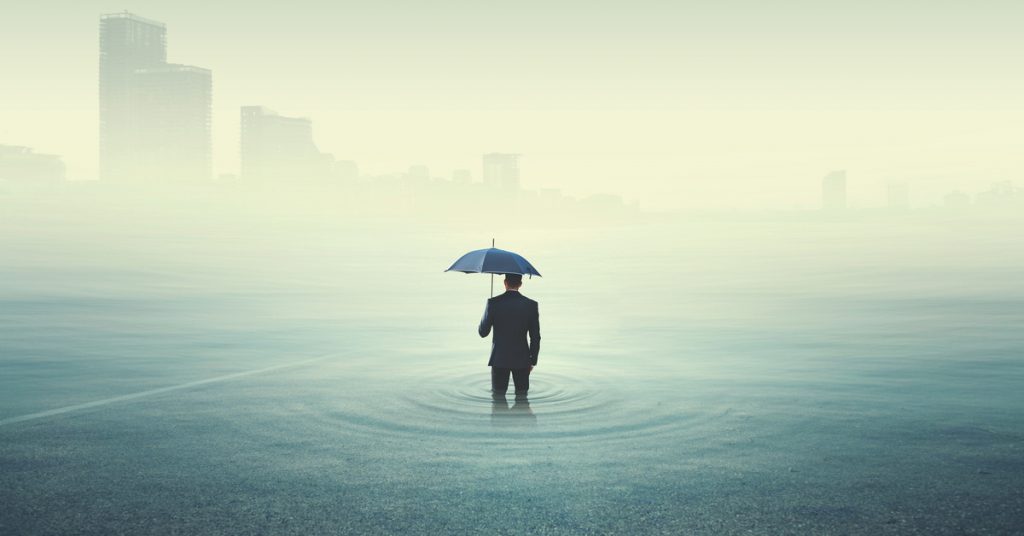
Climate change: the Bank of England’s commitments
In 2018, the Bank of England (the “BoE”) set up a project called “Future of Finance” aimed at anticipating the upcoming changes in financial services for the next decade, and the impact of these changes for market participants, customers and regulators. This research was led by Huw van Steenis, Senior Adviser to the Governor, and […]
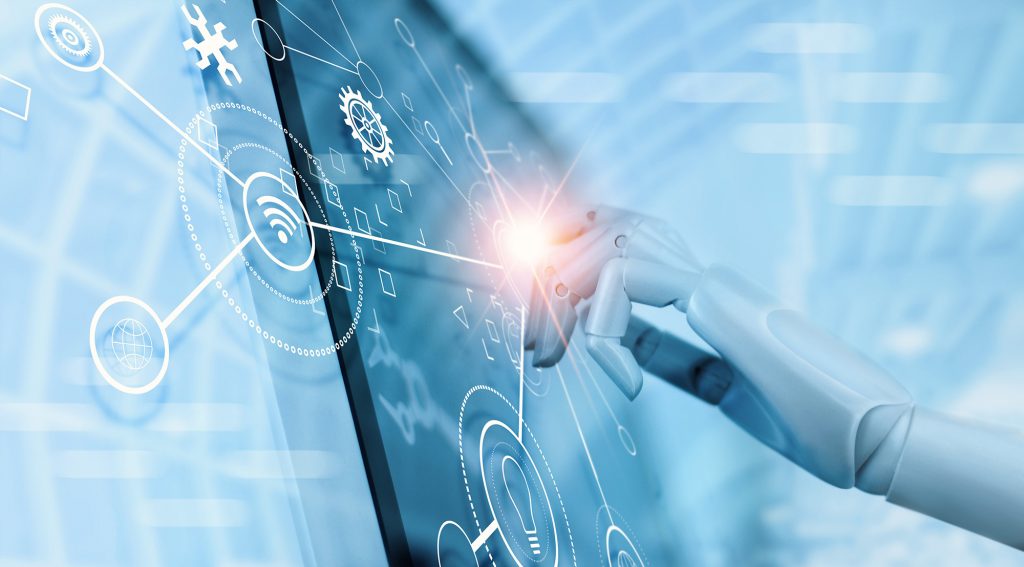
The Impact of Robotic Process Automation in Financial Services
Robotic process automation (RPA) is software that sits on a PC or workstation and is programmed to mimic the activities that a member of staff would perform. It will open applications, copy and paste data, and follow predefined rules. A robot will complete activities three to five times faster than a person. Customer satisfaction can […]
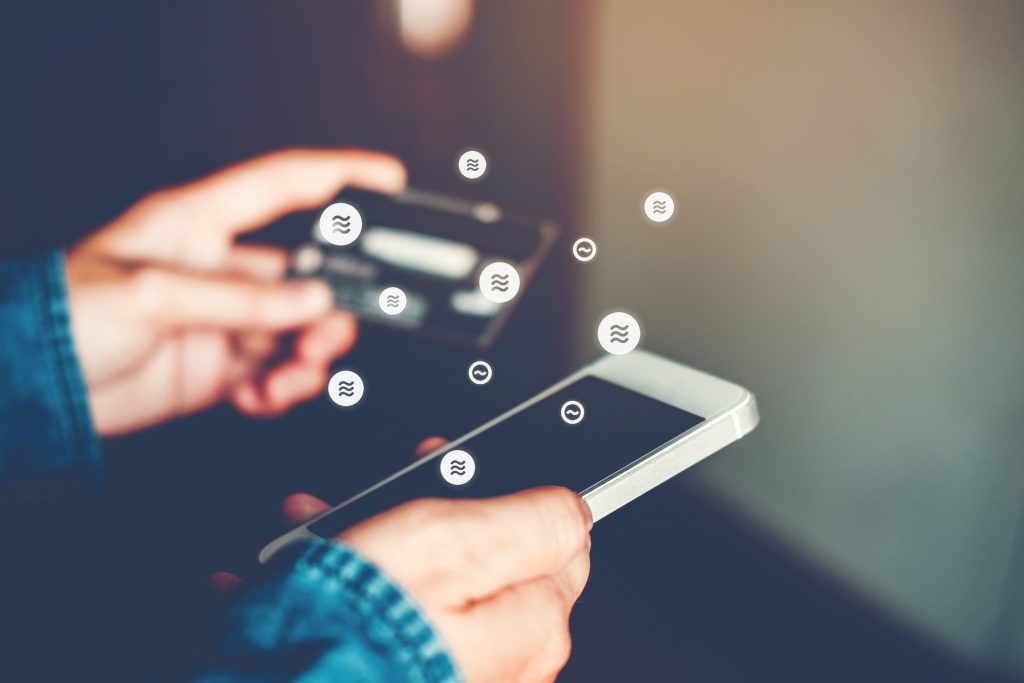
Should we be concerned by Facebook’s launch into cryptocurrencies?
Whether the launch of Facebook’s Libra will threaten global monetary and financial stability is a question that has been on all regulators’ and politicians’ minds since Facebook announced its cryptocurrency project on June 18, 2019. Donald Trump himself tweeted that, as a virtual currency, Libra “will have little standing or dependability” and that Facebook should […]
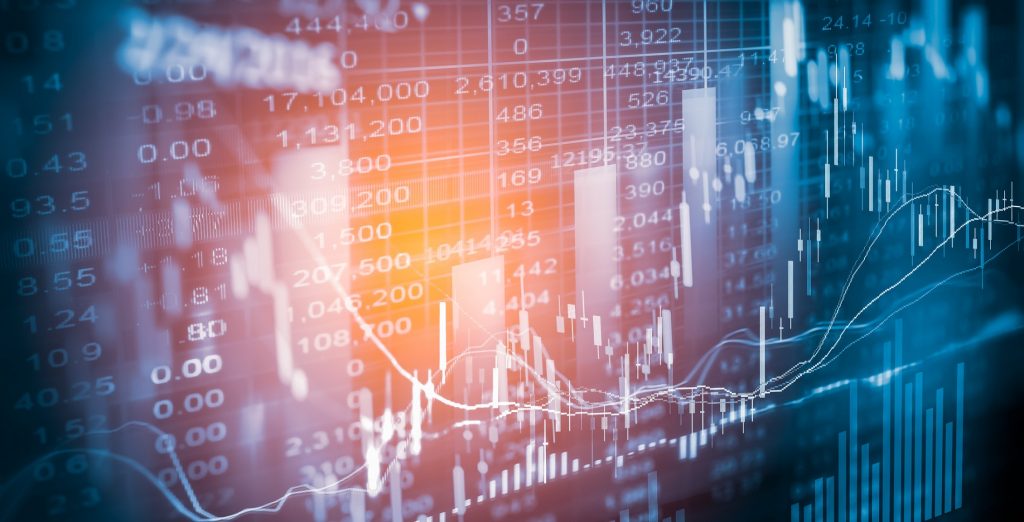
European CIB firms penalised by their regulatory environment
September 2018 saw the tenth anniversary of the collapse of Lehman Brothers, the US corporate and investment bank that was symbolic of Wall Street. Its failure is still fresh in the mind, and marked a turning point for banking and financial regulation. The disappearance of Lehman launched a cycle of “re-regulation” intended to increase the […]
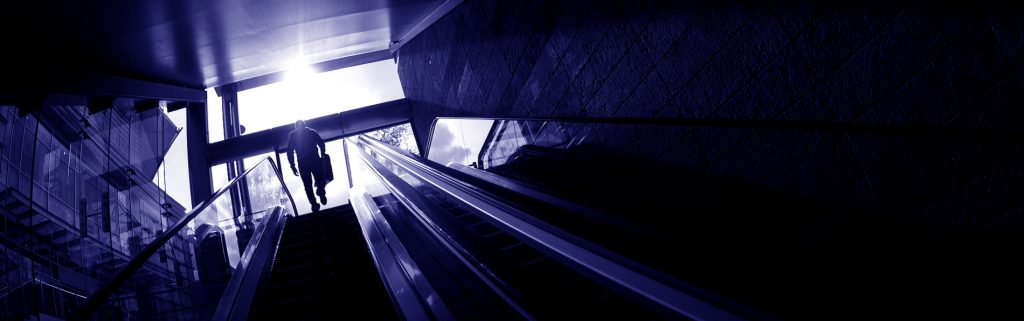
IBOR reform moves forward, but challenges remain
A raft of recent consultations on Ibor reform indicates that we may finally be making some progress. We have seen the International Swaps and Derivatives Association (ISDA) issue another round of consultations for Inter-Bank Offered Rates (IBORs) trying to solve the issue of the spread and term adjustments in fall-back for derivatives referencing IBORs and […]
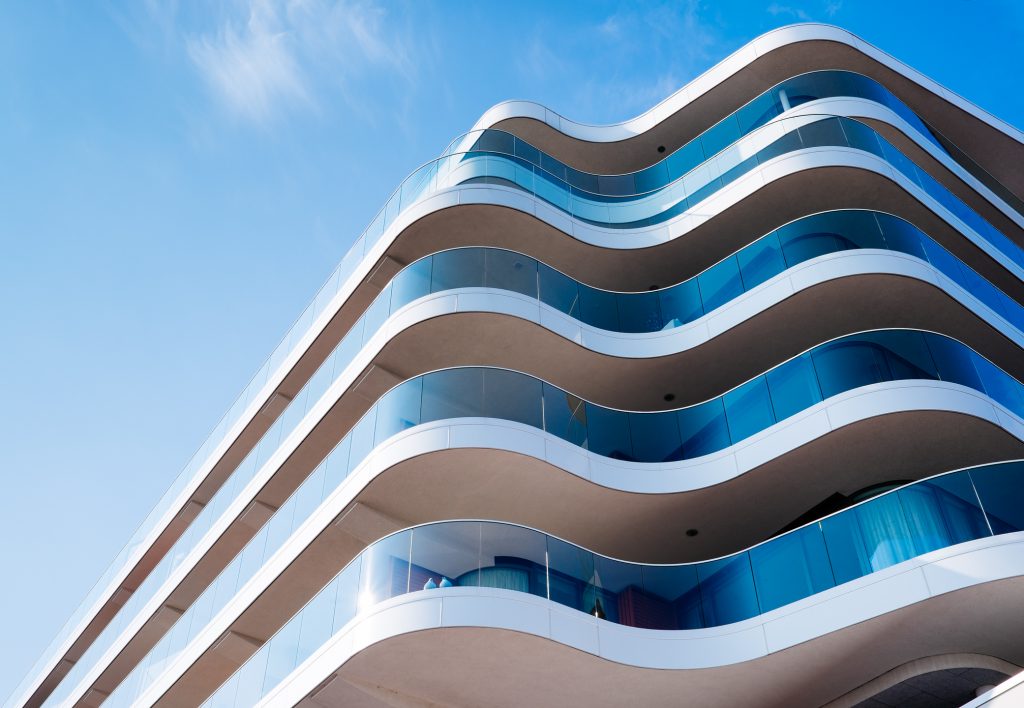
IFRS 16: Potential Changes in Real Estate Strategies
The standard IFRS 16 on leases is applicable to financial periods current at 1 January 2019. It applies to listed companies, their consolidated subsidiaries and entities presenting their accounts under international financial reporting standards (IFRS). The standard seeks to improve the presentation of leases in the accounts, and requires lessees (tenants) to account for leases […]
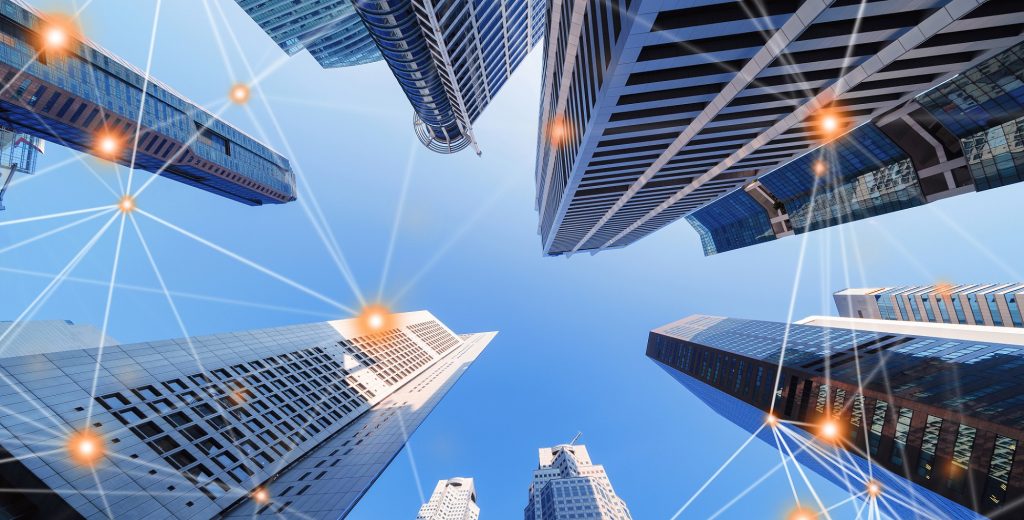
Real Estate Data Lake
Mass data… The real estate sector is gradually going digital, and recent constructions now incorporate equipment and connectivity to allow both use and maintenance to be optimised. However, as the renovation of the stock is naturally slow, the majority of these assets are not “connected”. Nevertheless, a great deal of data is available and constitutes […]
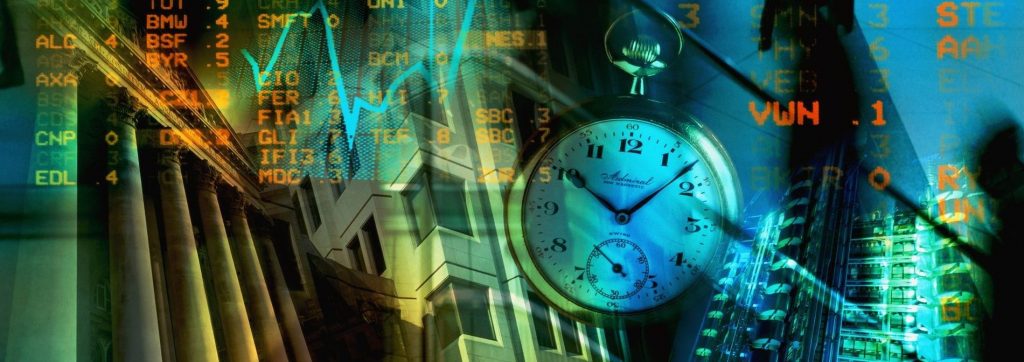
Brexit Watch #5: Brexit Extension – How are the Regulators Reacting?
During a meeting of the Special European Council on the 10 April 2019, EU leaders agreed to a flexible Brexit extension until 31 October 2019, to allow for the ratification of a withdrawal agreement. Despite this extension, there is still great pressure on Theresa May to secure a deal before the EU Parliament elections are […]
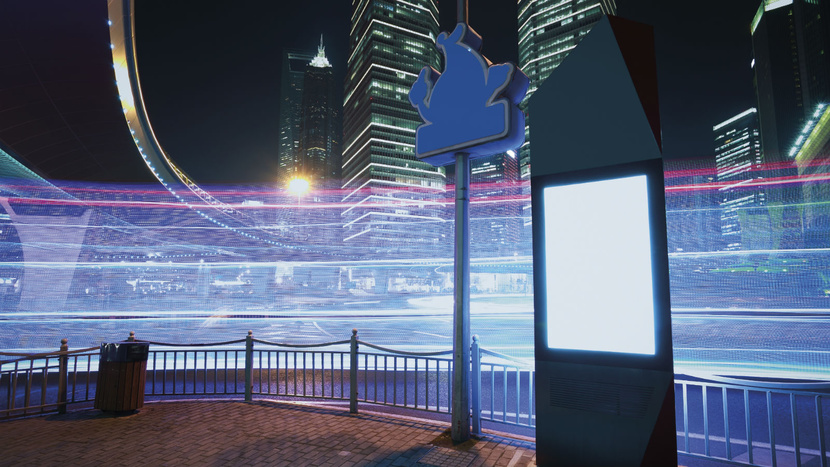
Future-proofing financial services: insight report for board directors
New technology, regulatory developments and societal changes, including those linked to strengthening stakeholder engagement, are substantially impacting financial services as well as the rest of the business community. For businesses to succeed in this fast-changing environment they must be led effectively by their boards and successful in seizing opportunities and managing the risks confronting them. […]
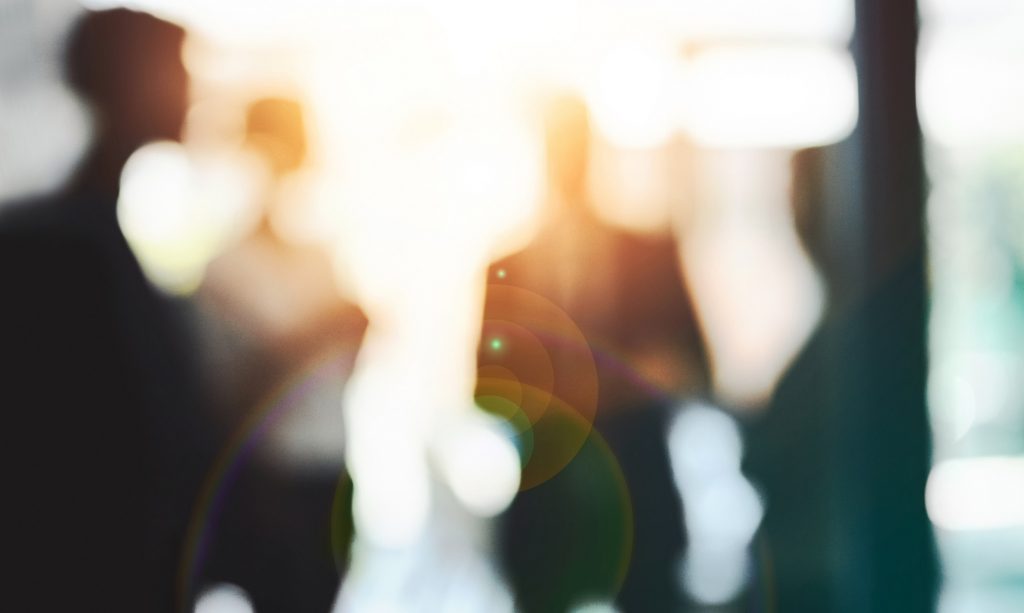
The need for a consistent agenda between regulators, supervisors and legislators
With 2019 marking a move for the European Banking Authority (EBA) to Paris in April, regulatory priorities will see the EBA preparing the work of transposing the last Basel III agreements. Following a quantitative and qualitative study, the Commission will be awaiting its opinion in or after June 2019. Further, in the context of EBA mandates […]
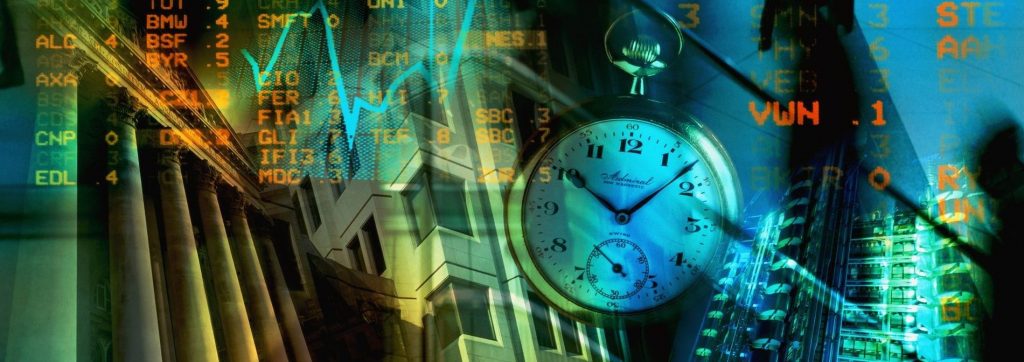
Brexit Watch #4 : A snapshot of how the financial services regulators are reacting
Brexit preparation is one of the largest undertakings that regulators and market participants have ever done, considering the uncertainty and the impact it carries. Many financial institutions have stopped waiting for lawmakers to finalise negotiations over the terms of the exit and are already working towards contingency plans. Banks and brokers are setting up new […]
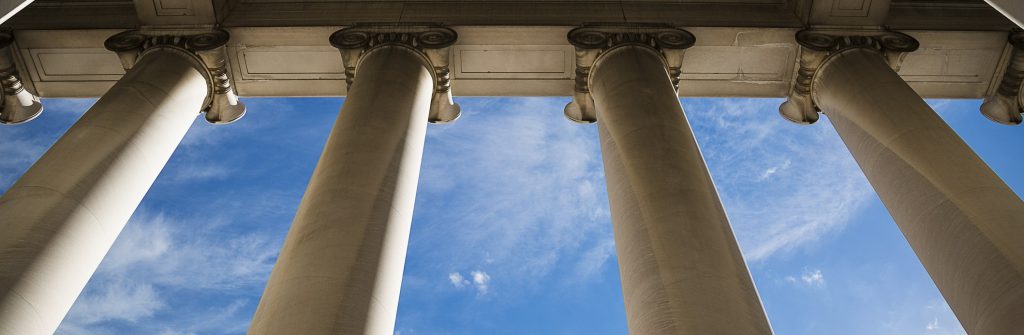
Implementation of post-crisis reforms and remaining challenges in 2019
At the last G20 summit in Buenos Aires, leaders called for the full implementation of all major international financial reforms intended to improve the financial system, in particular, those drawn up by the Financial Stability Board (FSB) and the Basel Committee on Banking Supervision (BCBS), the last being the review of market risk framework published […]
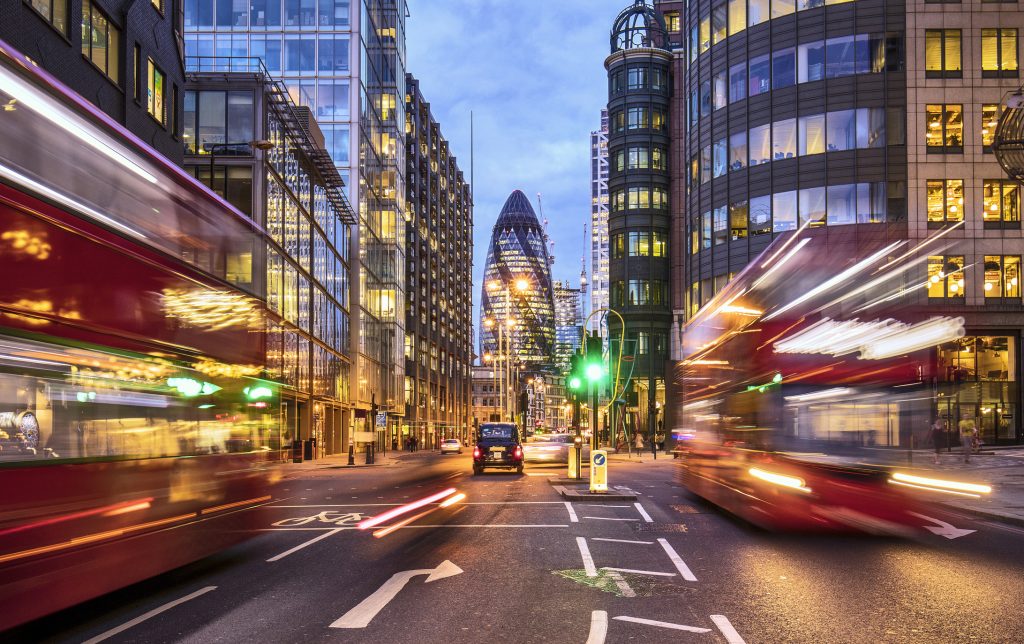
Brexit, Volcker 2.0 and the Bipartisan Banking Bill lead topics of 2019 Institute of International Bankers conference
On March 11 and 12, 2019, Mazars attended the 30th annual conference organized by the Institute of International Bankers (IIB). Agencies and regulators serving as speakers and panelists this year, included: •The Federal Reserve Board (FRB) •The Department of the Treasury •The Commodity Futures Trading Commission (CFTC) •The Securities and Exchanges Commission (SEC) •The Office […]
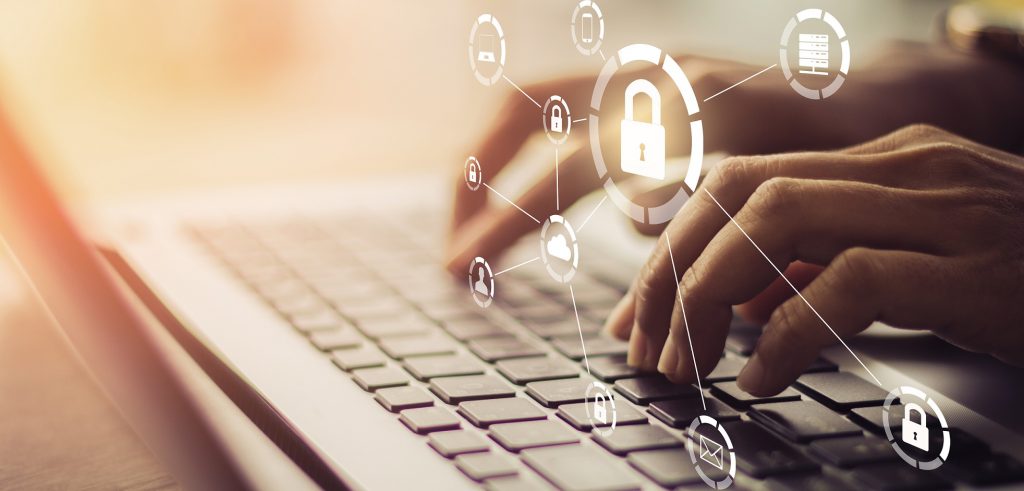
Data Protection: constraint or opportunity?
Today’s world is witnessing an explosion of data, including personal data: your civil status, what you do and don’t like, your holidays, your favourite leisure activities. The exploitation of all this data is multiplying through the use of innovative IT tools. [pukka_pullquote width=”300″ txt_color=”#ffffff” bg_color=”#2d2d2d” size=”24″ align=”left”]”A pessimist sees the difficulty in every opportunity; an […]
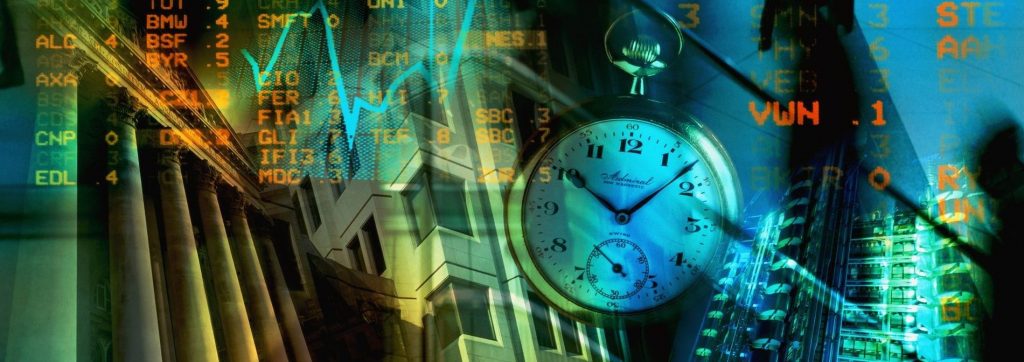
Brexit Watch #3: countdown is on
Following a majority vote against Theresa May’s Brexit deal on 15 January 2019, and with only 3 weeks until the proposed deadline of 29 March, financial services authorities in the UK and EU have been urgently preparing for an increasingly likely no-deal Brexit, announcing further transitional instruments and offering guidance to firms within the sector. […]
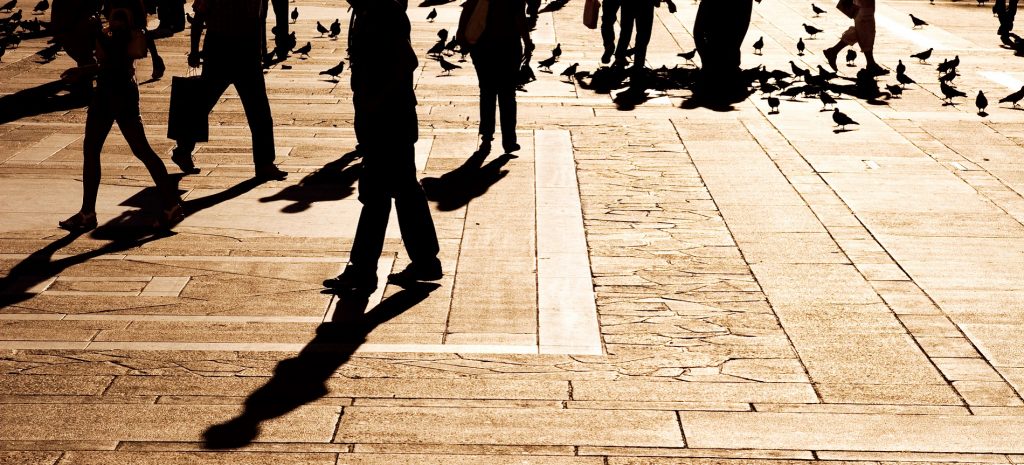
LIBOR reform: Setting the cat among the pigeons
Could the transition period towards the new alternative Risk-Free Rates (RFRs) be more complex than initially envisaged? The speech given by Edwin Schooling Latter, Director of Markets and Wholesale Policy at the Financial Conduct Authority (FCA), on the 28 January 2019, suggests this might be the case. While Mr Latter re-affirmed that the key focus […]
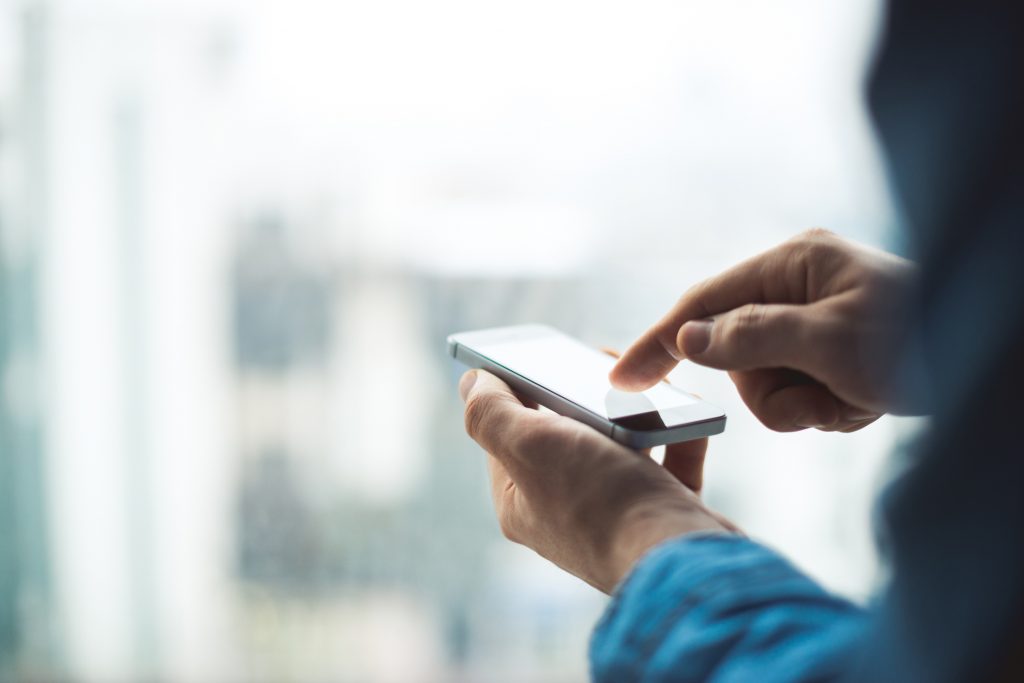
Is one single payment standard the way forward for the banking sector?
The financial sector is bursting with disruptive Fintech start-ups at present who are shaking up the banking and insurance sector with innovations varying from online mortgage banks to online payment services. With innovation occurring at such a rapid pace, how are banks responding? To some extent, the arrival of the revised Payment Services Directive (PSD2) […]
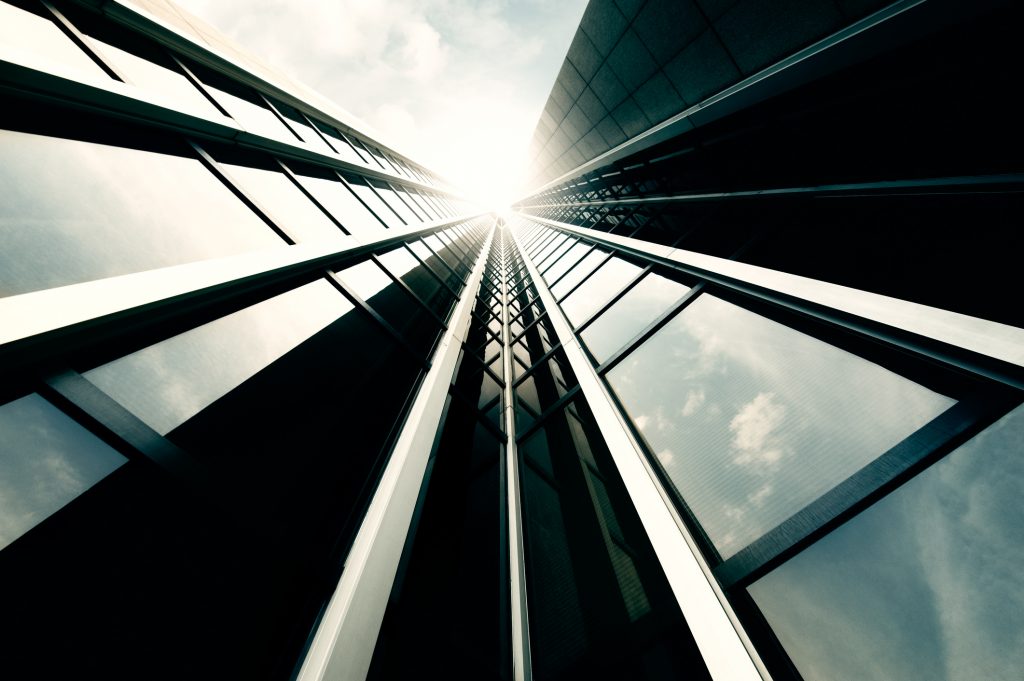
Is there light at the end of the Trading Book’s tunnel?
Seven years after the first Basel Committee on Banking Supervision’s (BCBS) consultative paper on the Fundamental Review of the Trading Book (FRTB) and three years after the publication of the revised Market Risk Framework, is the 14 January 2019 release of the final Minimum Capital Requirements for market risk the end of this particular regulatory […]
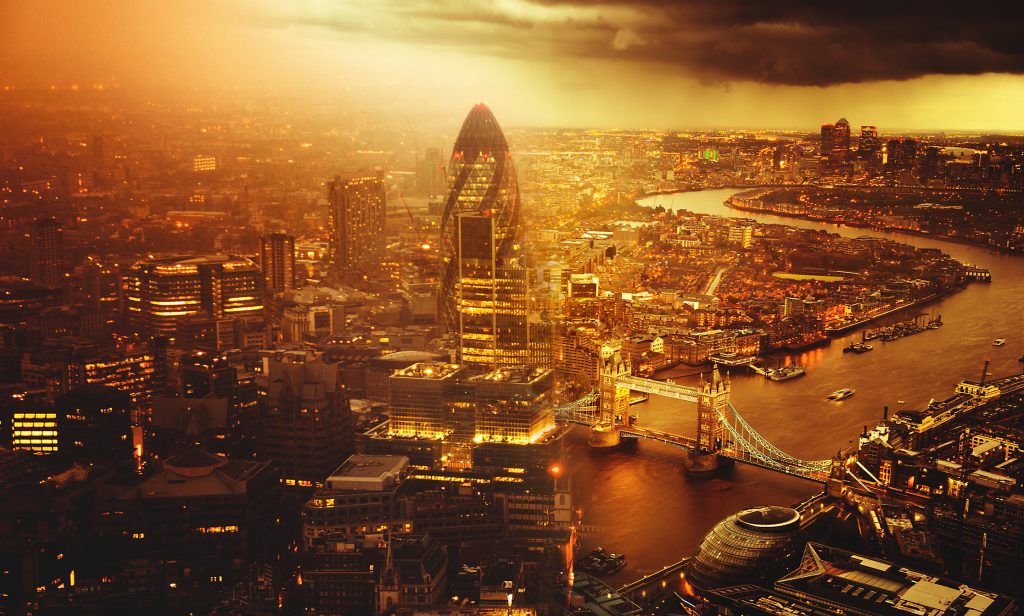
Climate change: a threat to the stability of the financial services
With rising global temperatures[1] comes an ever-growing pressure on the financial services sector to respond and prepare for the far-reaching effects of climate change. The impacts upon the sector are already being felt – extreme weather events are creating significant losses for insurers and credit risks for banks, and pressures on businesses to demonstrate sustainable […]
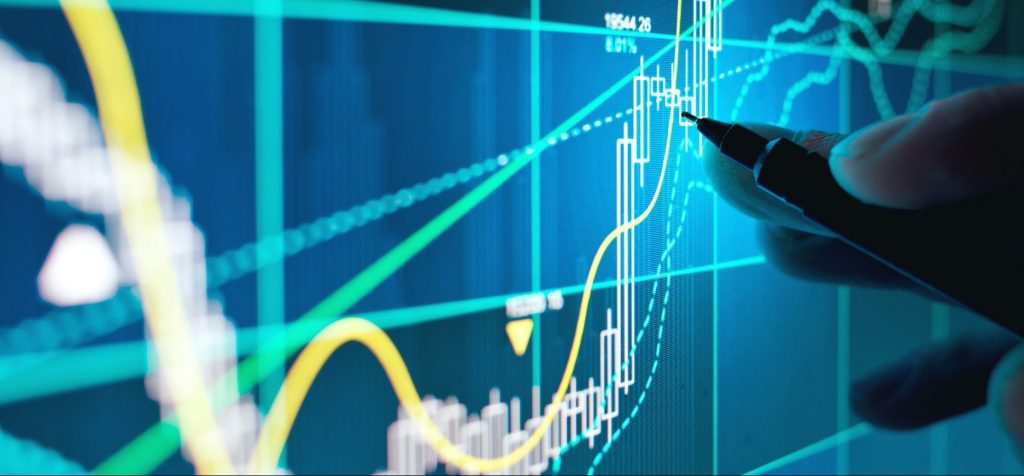
A decade on from Lehman Brothers
Which is the more significant – the tenth anniversary of the collapse of Lehman Brothers, or the tenth anniversary of the opening of the App Store? For the global financial community world, 15 September 2008 is a key date, weighted with as much symbolism as the great stock market crash of 24 October 1929 had […]
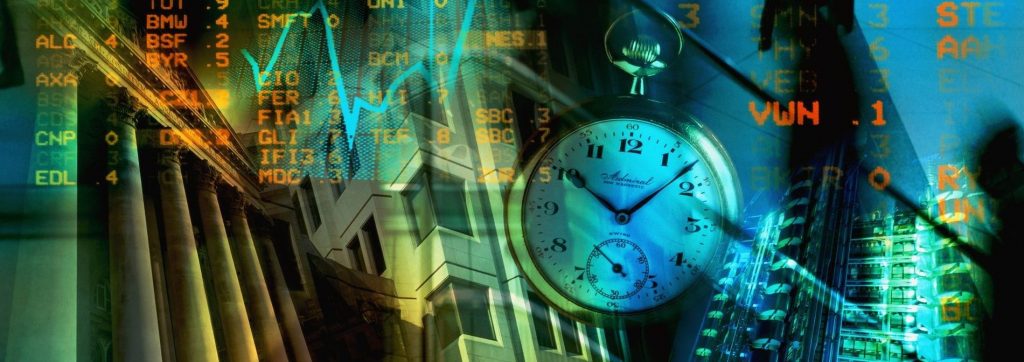
Brexit Watch #2 : Impact of No Deal and Other Regulatory Issues
In the second installation to our Brexit Watch, the delay of the House of Commons vote and subsequent negotiations increases uncertainty, with multiple possible scenarios set to emerge. Brexit preparation has been one of the largest undertakings for regulatory bodies on both sides of the channel. Despite continued unpredictability surrounding outcomes, new directives and amendments […]
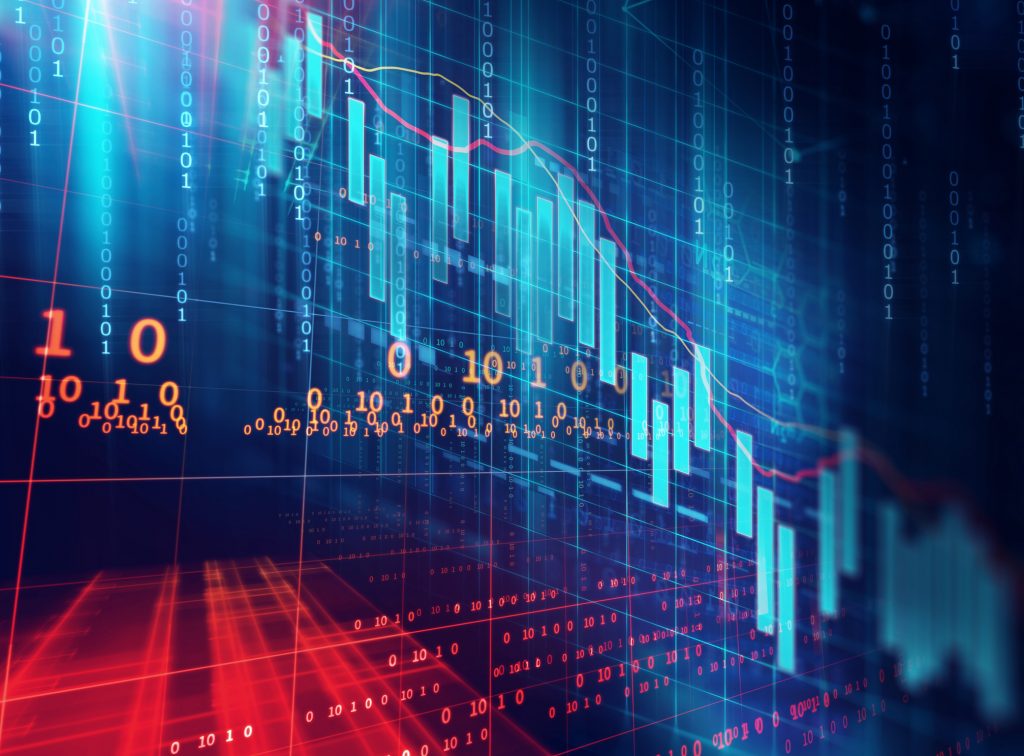
European banks are better armed against macro-economic shocks
On Friday 2 November, as expected, the European Banking Authority (EBA) published the results of the 2018 EU wide stress tests on European banks’ solvency in the event of macro-economic shocks. This was the fourth exercise of the now-biannual testing which has been carried out on European Union banks. Despite more severe tests than in […]
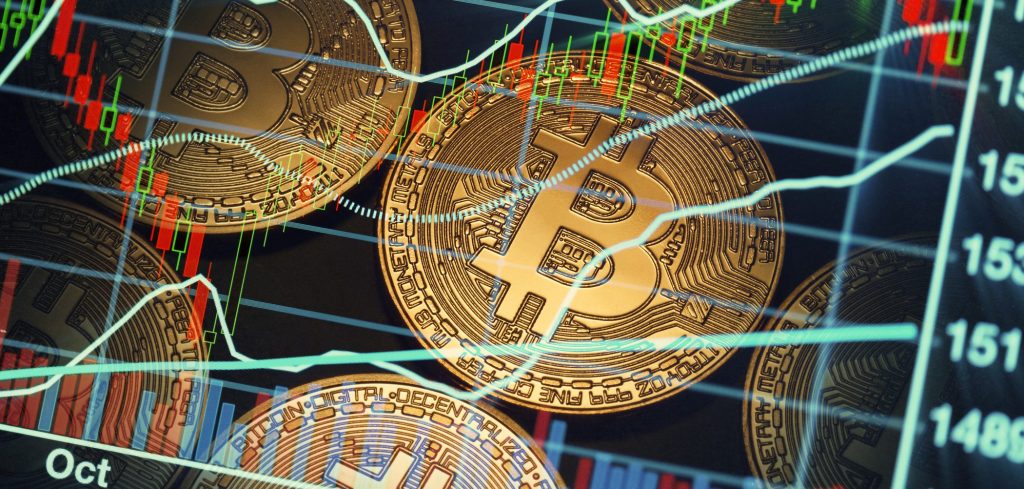
Cryptoassets: Accounting for an emerging asset class
The sweeping growth and prolific collection of technologies that make up cryptoassets today have made it incredibly challenging for regulators worldwide to standardize and issue authoritative guidance. Professional accounting standard-setting bodies, like the Financial Accounting Standards Board (FASB) and the International Accounting Standards Board (IASB) are certainly no exception. What started out as just bitcoin […]
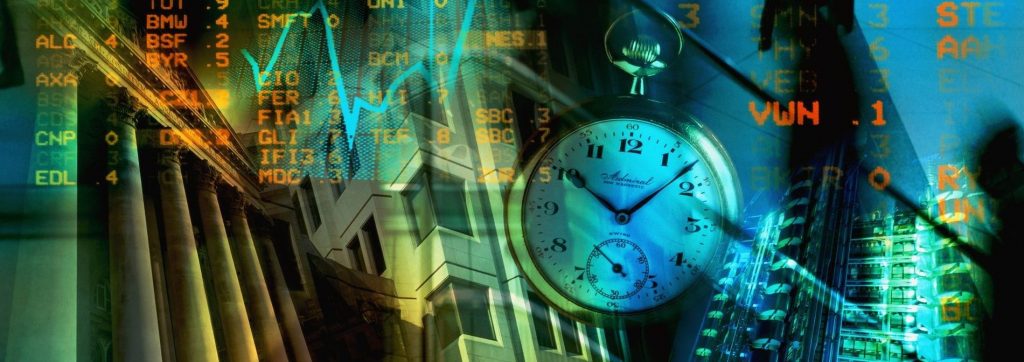
Brexit Watch #1 : Impact of No Deal and Other Regulatory Issues
As negotiations on the UK’s exit from Europe continue, albeit slowly, regulatory bodies in the UK and Europe are beginning to issue regulatory changes to cope with all eventualities, including the UK leaving Europe without a deal. As a result, a number of new directives and amendments to regulations have been issued over the summer […]
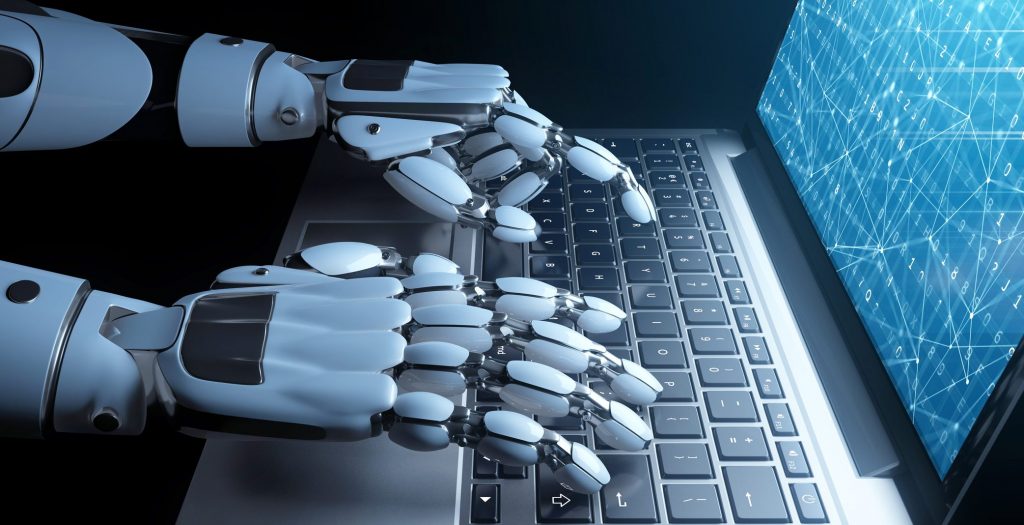
Robo-advisors: turbo-charging the race for unit-linked sales?
With a portfolio in excess of €1300 billion in 2017, euro savings are firmly established as the preferred life-insurance product for people in France, thanks to their threefold benefits of guaranteed capital, attractive returns and instant liquidity. However, recent financial and regulatory developments raise questions as to the legitimacy of this product in the savings […]
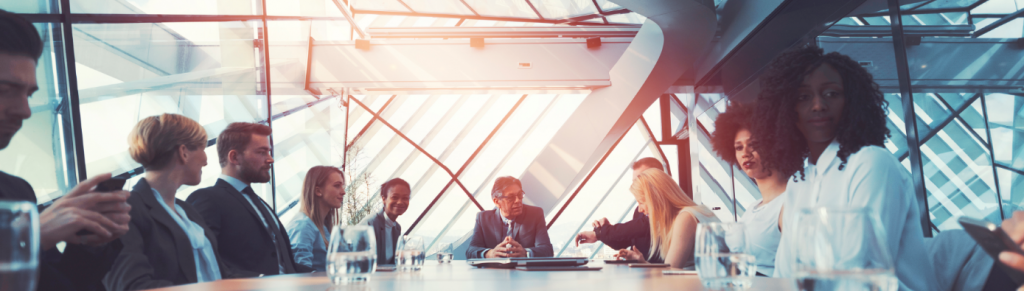
Integrated Reporting: Towards a Global Adoption?
Integrated Reporting applies principles and concepts that are focused on bringing greater cohesion and efficiency to the reporting process, and adopting ‘integrated thinking’ as a way of breaking down internal silos and reducing duplication.The Framework has been tested and assessed during these past three years and significant ‘breakthroughs’ have been achieved. Our present paper, therefore, […]
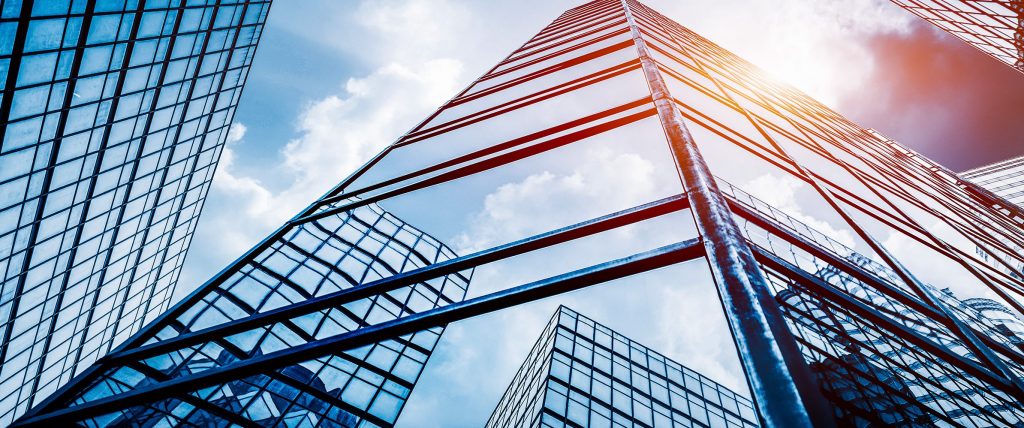
European Insurers’ IFRS 9 Benchmark Study
The new standard IFRS 9 on financial instruments has been effective starting 1st January 2018 for most entities but insurance groups have the possibility to defer its application to 2021, the year when the new standard IFRS 17 on insurance contracts will enter into force. IFRS 9 introduces numerous changes (Phase 1/ classification, Phase 2/ […]
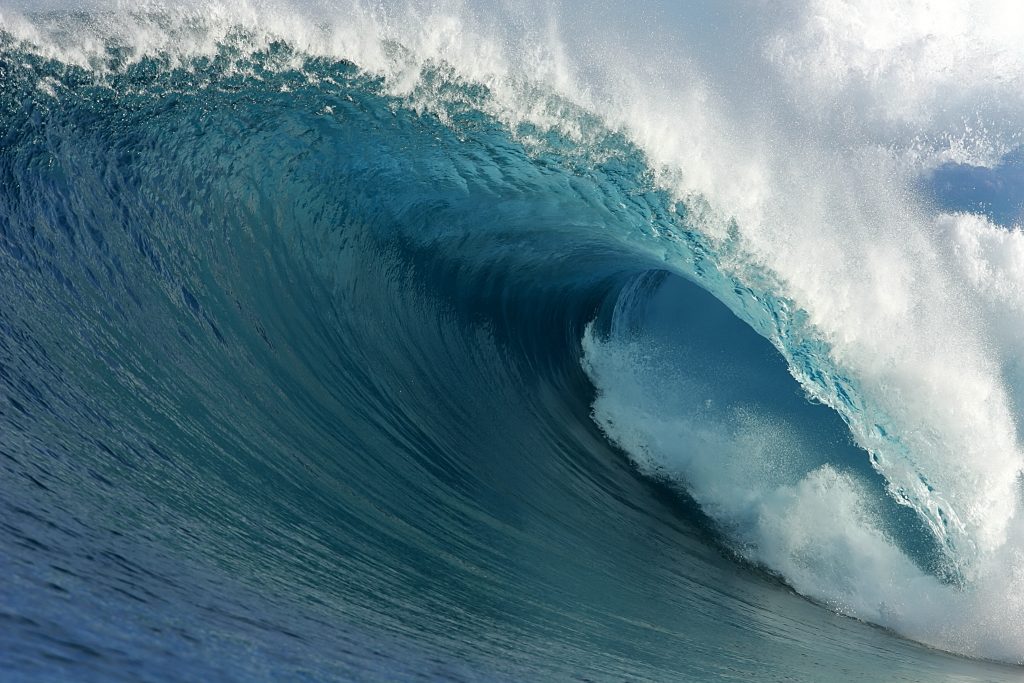
Benchmark Study on Reinsurers’ Financial Communication
Reinsurance, also known as the “ insurers’ insurance ”, plays a key role in the global market economy today. Several factors, such as the strengthening of capital requirements, the increasing level of significant NAT CAT events or the need for optimal coverage is increasing the need for reinsurance. Initially, insurance companies sought partnerships with reinsurers […]
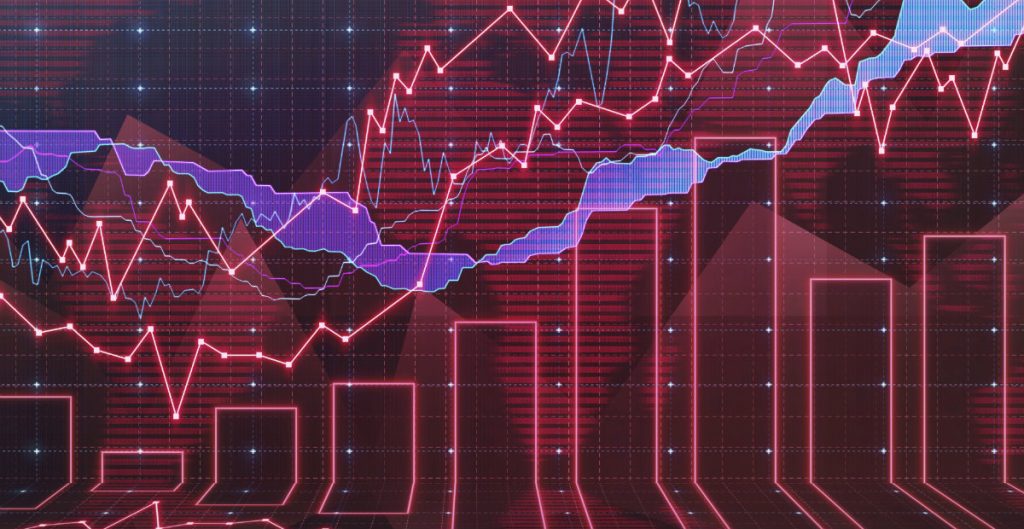
Crypto-Funds: Regulation? Yes please!
While 2018 has witnessed some turmoil in the crypto-currency markets, interest in this new asset class – and also in investment funds which allow easy access to crypto-currencies – remains high. On 26 June 2018 CBOE Global Markets filed an application with the US Security and Exchange Commission (SEC) seeking approval for a Bitcoin Exchange […]
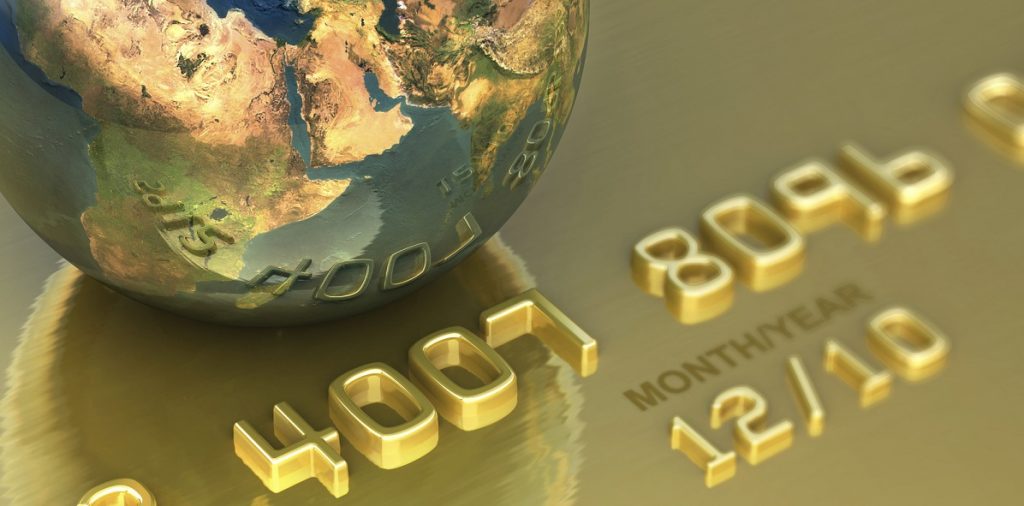
BEAT Could Eat Into Income Tax Savings
Tax legislation generally includes promises to simplify the process of computing taxes. But in the process of transforming legislation into law, those good intentions often are overshadowed by new complexities. The Tax Cuts and Jobs Act of 2017 is no exception, especially for U.S. multinational corporations. Although most corporations herald their much lower 21% tax […]
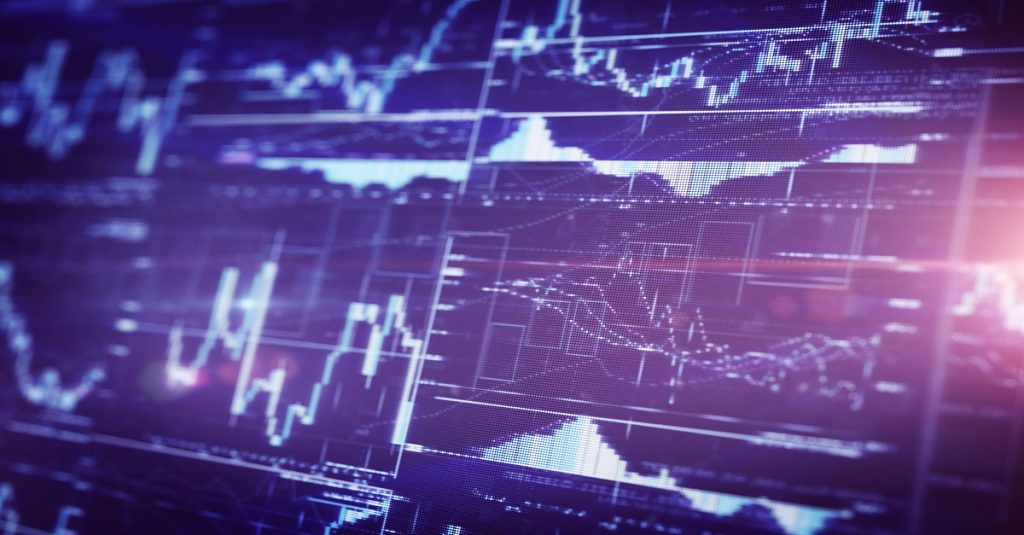
An IBOR revolution is on its way
The whole financial system relies on reference interest rates, more precisely on InterBank Offered Rates (IBORs) whose integrity and reliability have raised some concerns since the 2008 financial crisis and the LIBOR manipulation scandal. These IBORs are used to determine the unsecured short-term funding cost in the interbank market for a combination of currencies, tenors […]
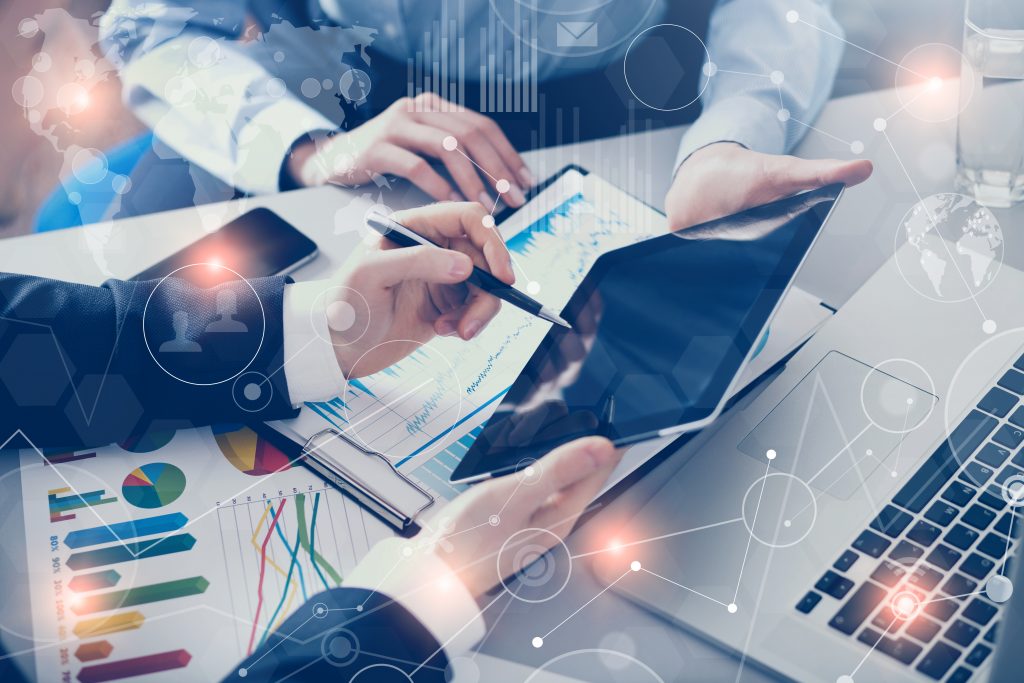
IFRS 17 poses new challenges for the reinsurance industry
After many years of development, in May 2017, the International Accounting Standards Board (IASB) published a new standard for insurance contracts. Coming into effect on January 1st 2021, the IFRS 17 standard introduces a new model for accounting and measuring insurance liabilities with a framework which is substantially different from current accounting practices. While the […]
President Trump Signs the Senate’s Banking Regulatory Reform Bill (S.2155)
S.1255 the Economic Growth, Regulatory Relief, and Consumer Protection Act (the “Senate Banking Bill”) was passed on March 14, 2018 after a strongly bipartisan 67-31 vote. On May 22, 2018, the House passed the Senate Banking Bill with 258 yeas (225 Republicans and 33 Democrats) and 159 nays (1 Republican and 158 Democrats). The bill […]
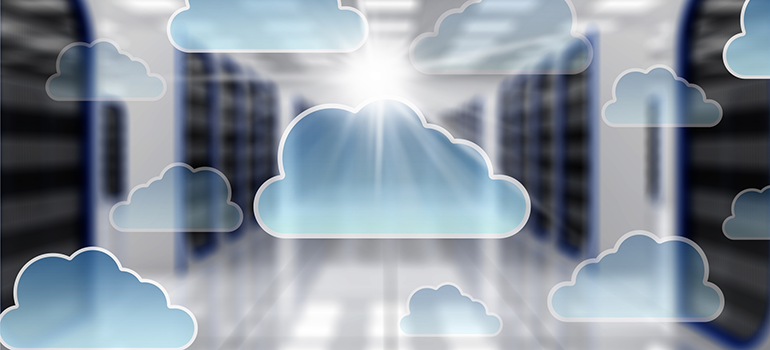
Sizing Up Amazon Web Services
Fintech is prominent in today’s business lexicon, having migrated from the back office to a prominent position in both consumer and commercial finance. Its core functionality on mobile devices and wide application in artificial intelligence (AI) spans blockchain, smart contracts, banking, insurance, regulation and cybersecurity. And Amazon Web Services (AWS), a major cloud player, is […]
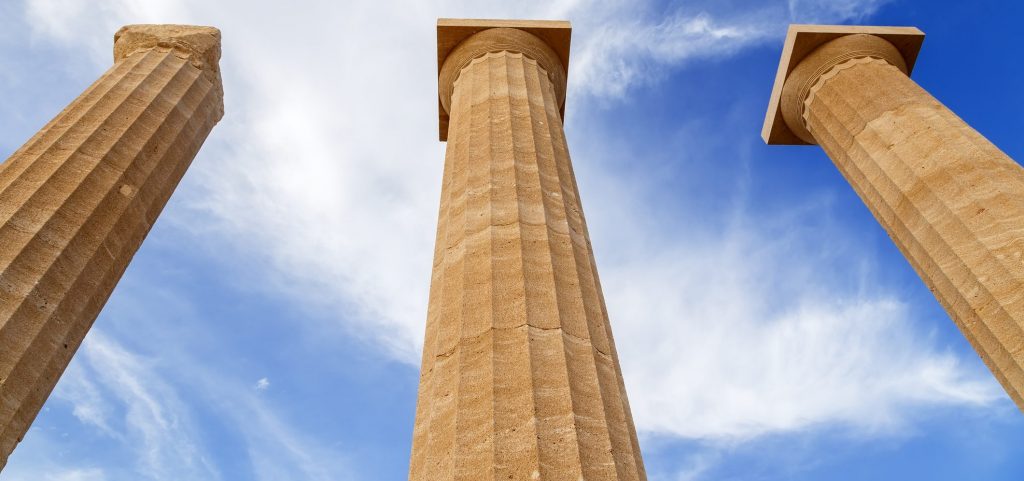
Podcast: Basel III Implementation – May 2018
In this podcast, Greg Simpson discusses the Prudential Regulatory changes proposed by Basel 3. Together with Phuong Gomard and Bowen Lu, regulatory specialists in Mazars’ Banking Consulting practice, they highlight the impact on the standardised approach to credit risk and operational risk. Whilst relevant to all UK banks, it is particularly relevant to smaller challengers and […]
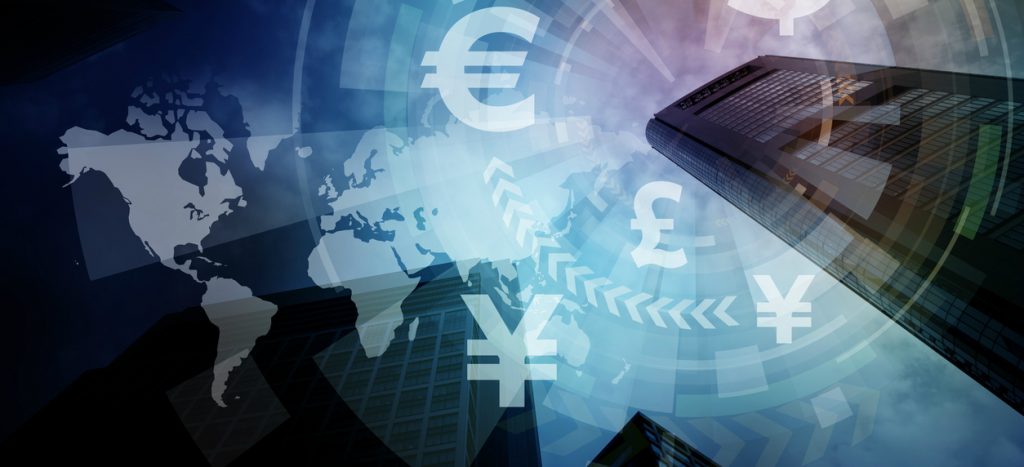
GDPR and PSD2: what are the issues for FinTechs?
The FinTech model is reaching a new level of maturity. The first stage saw them disrupt the traditional banking business models using technology to impact the customer experience and relationship. The second wave offers FinTechs the opportunity to expand their service offering and develop their independence, provided that they understand their relationship with the regulatory […]
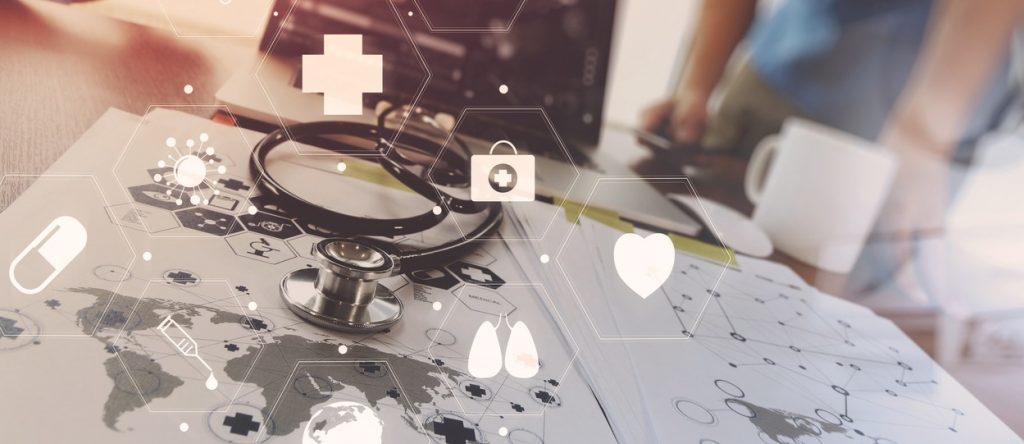
FATCA and the Common Reporting Standard Applied by Life Insurers – a Few Practical Considerations
Within the insurance industry, automatic exchange of information under Foreign Account Tax Compliance Act (FATCA) and the Common Reporting Standard (CRS) is mainly relevant to life insurers, since non-life insurance and reinsurance activities generally do not give rise to classification and reporting obligations under these regulations. Life insurance activities (technically, the fact of issuing or […]
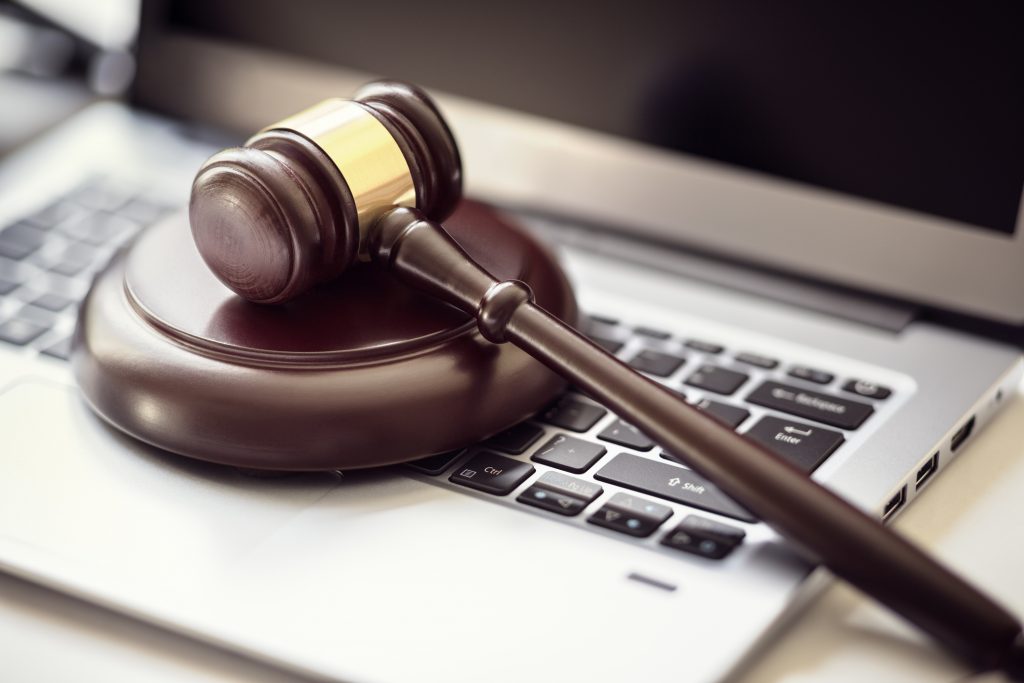
How companies in the financial services sector can measure their human rights footprint in four steps
The complexities of creating and implementing a due diligence framework to monitor and measure the impact financial services have on human rights can be overwhelming. The EU recommended UN Guiding Principles on Business and Human Rights Reporting Framework (www.UNGPreporting.org) – co-authored by Mazars and Shift – , as well as the EU Non-Financial Reporting Directive […]
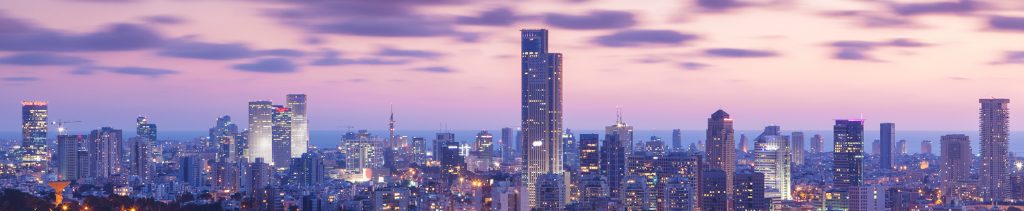
Israeli Debt-Based Financing For Real Estate in the USA
Prominent real estate owners and developers are sourcing an attractive form of capital—bonds issued and traded on the Tel Aviv Stock Exchange (TASE). It’s a creative concept, with benefits for both sides of the transaction, and is also attractive for U.S. listed Real Estate Investment Trusts (REITs), enabling them to issue bonds in Israel while […]
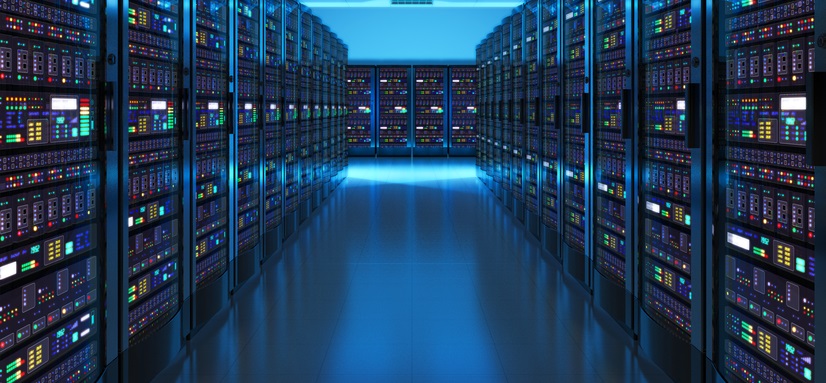
Will the emergence of big data analytics lead to a new wave of regulation in the financial sector?
Big data analytics is one of the most discussed topics in the world of finance. Application of these methods has led to better assessment of credit quality, improved pricing of insurance contracts as well as automation of client interaction. Processes that were previously cumbersome, such as customer on-boarding, have been streamlined and their costs drastically […]
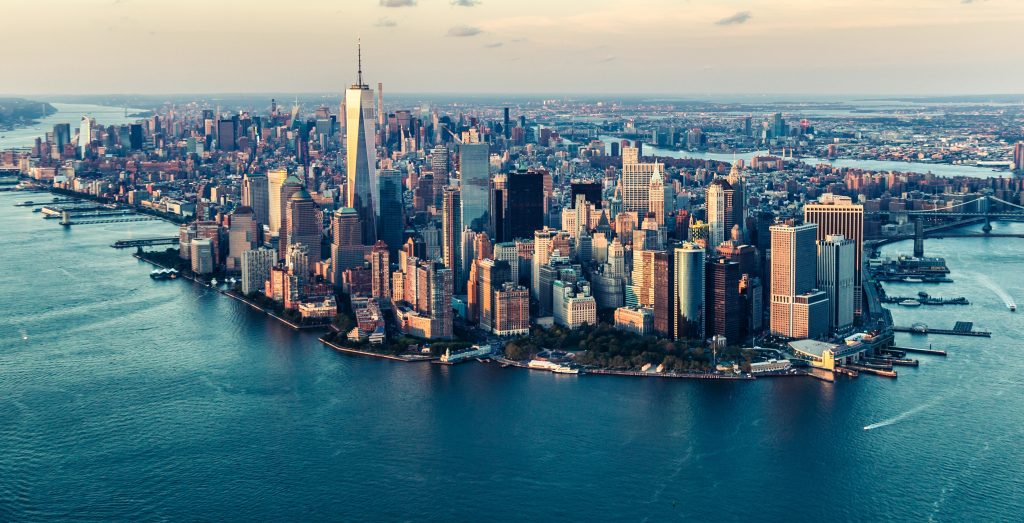
Chinese Investment In NYC Hotels Remains Strong, But Shows Signs Of Slowing
By Bisnow, sponsored by Mazars New York City was both the most liquid hospitality market in the country[i], and global hotel investors’ destination of choice in 2015, according to JLL. That year, its transaction volume exceeded $6.6B, outpacing London by 40% and eclipsing its own 2011 record of $3.1B. Although total hotel deal volume decreased […]
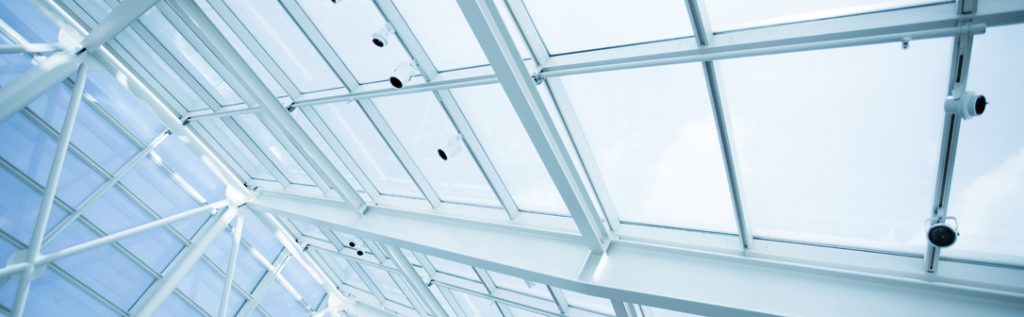
US Tax Reform: Tax Cuts and Jobs Act Imposes New Limitations on Deductibility of Trade or Business Interest
The Tax Cuts and Jobs Act (the “TCJA”), signed into law in December 2017, and applicable to taxable years beginning after December 31, 2017, contains several provisions that will have a significant impact on specific types of entities and investments. One of the most radical departures from current law involves new limitations on the deductibility […]
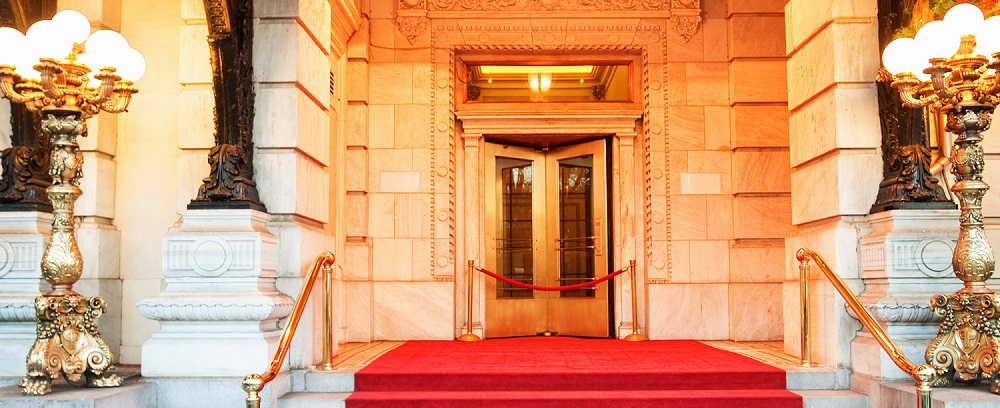
3 Great Ways Hotels Can Cater To Millennial Guests
By Bisnow, sponsored by Mazars Millennials have surpassed baby boomers as the largest living generation[i], and they are spending a larger share of their incomes on experiences[ii] like travel and dining than on possessions. Winning over this generation, which controls a growing share of the nation’s disposable income[iii], is important for hotels’ profitability and long-term […]
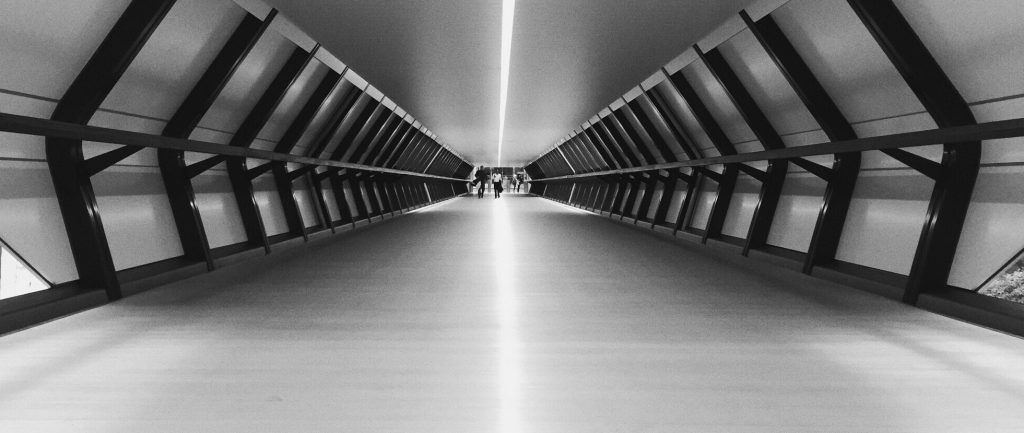
FRTB: has the journey finally begun or has it just ended?
It is truly amazing the turmoil 88 pages can produce. Yet while the whole Banking Industry is strongly opposed to some of the new Fundamental Review of the Trading Book (FRTB) requirements, such as the Profit & Loss Attribution (PLA) test, could the EU Council now be considering to only include reporting requirements in CRR2? […]
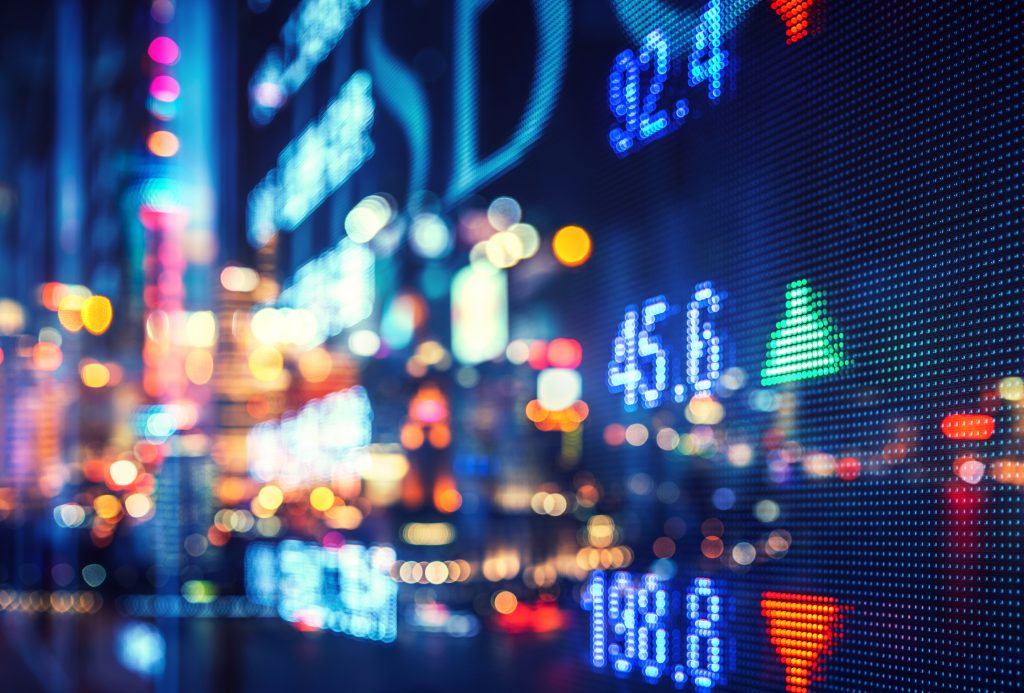
Overview of the US Stress Test Scenarios Vs. the European Scenarios
On February 1, 2018, just one day after the European Banking Authority (EBA) officially kicked off its EU-wide stress test exercise, the Federal Reserve Board (FRB) released the applicable scenarios for its own US stress test. 2018 US Stress Test Scenarios Overview The annual stress test exercise is required by a provision of the Comprehensive […]
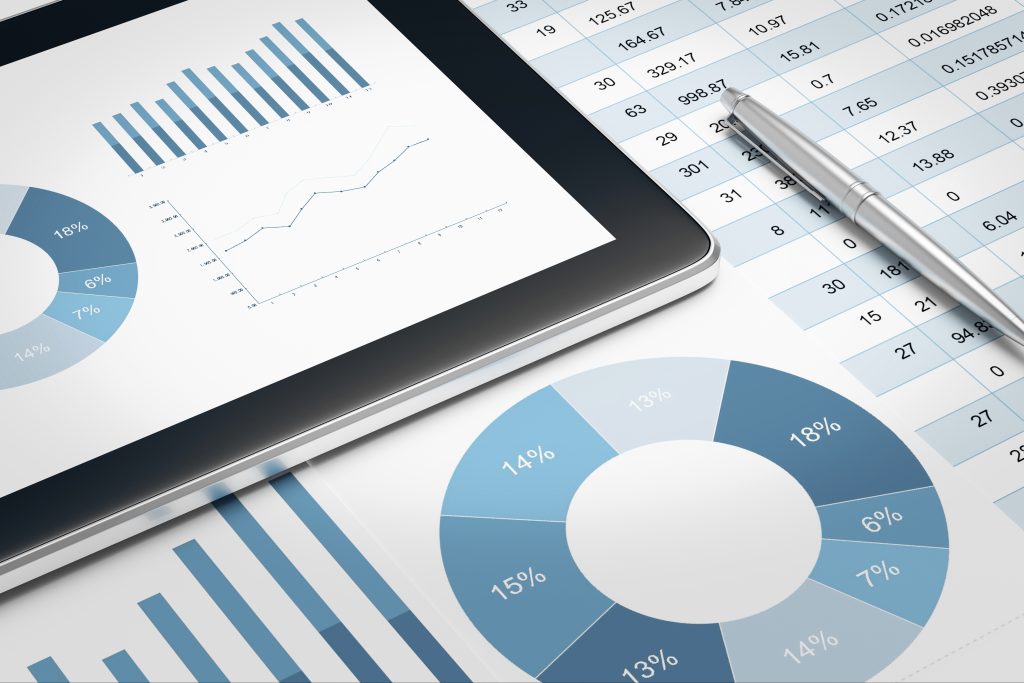
SFCR: Review of narrative reports, good practices and EIOPA recommendations
The Solvency II Directive increases the requirements for transparency vis-à-vis both the regulatory authorities and the stakeholders, including policyholders, financial analysts and investors. In this context, insurance undertakings and groups were, for the first time, required to publish a narrative report no later than 19 May 2017, known as the Solvency and Financial Condition Report […]
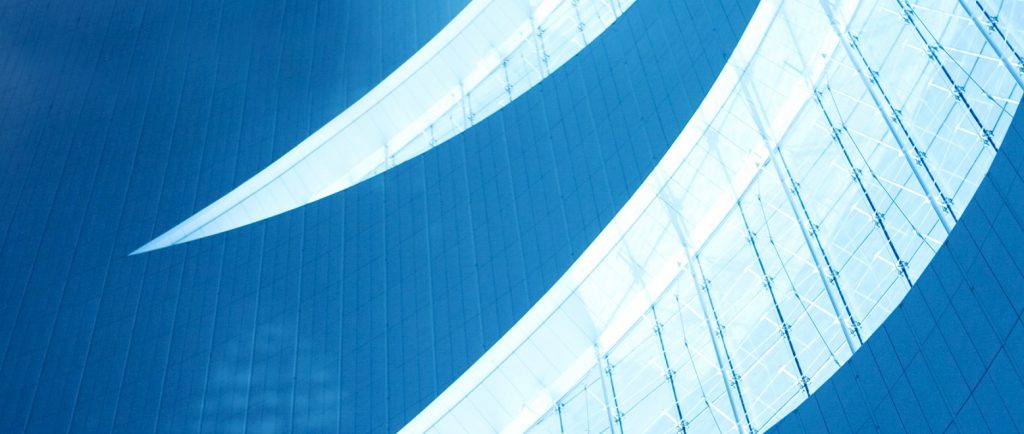
Quantified impacts of IFRS 9 : initial findings
At the end of February 2018, all the major European banks published information on the impact of the implementation of the new standard IFRS 9. IFRS 9 introduces numerous changes (classification, impairment, hedging, etc.). Their impacts at the transition date vary widely from one bank to another. They are negative in most cases, but for […]
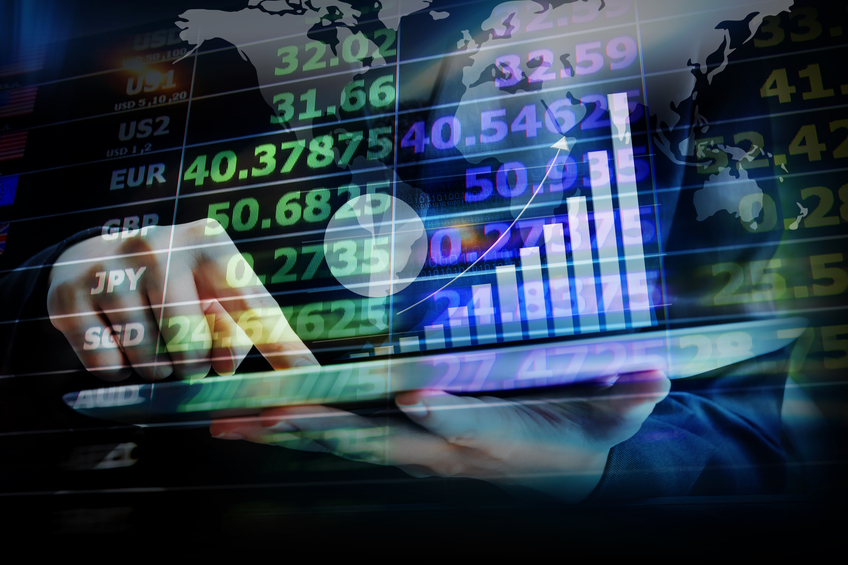
Ultimate Forward Rate (UFR): Why we are seeing a change to the rate curve
On 6 February 2018, EIOPA published its latest risk-free interest rate curve to be taken into account for the purposes of Solvency II calculations. Based on calculations for January 2018, the curve is slightly different from previously published curves. This is reflecting significant changes in the long-term expectations of interest rates in recent years which calculates […]
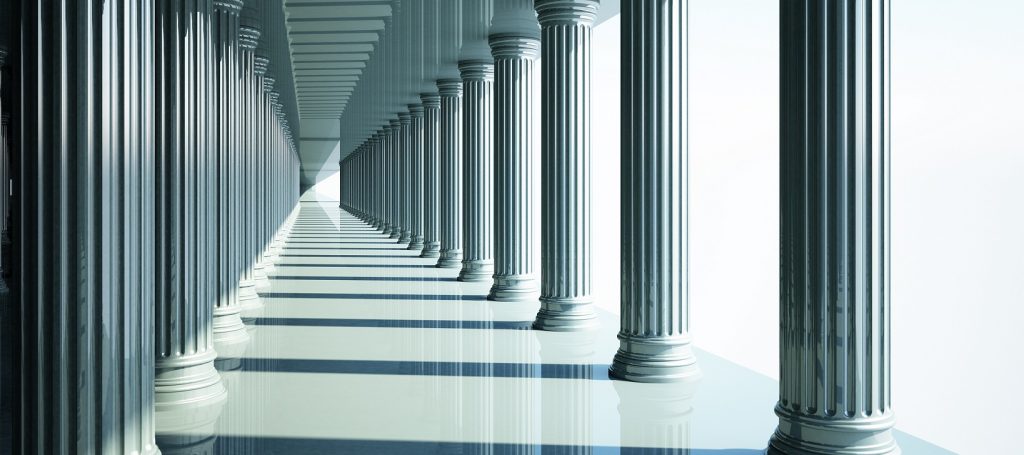
Tackling the issue of NPLs: a European perspective
Because of the 2008 financial and economic crisis, banks have accumulated billions of non-performing loans (NPLs) on their balance sheets. Even 10 years after this major economic event, the situation is not yet back to normal in some countries. In the European Union, and in the Eurozone particularly, NPLs are a real concern as they […]
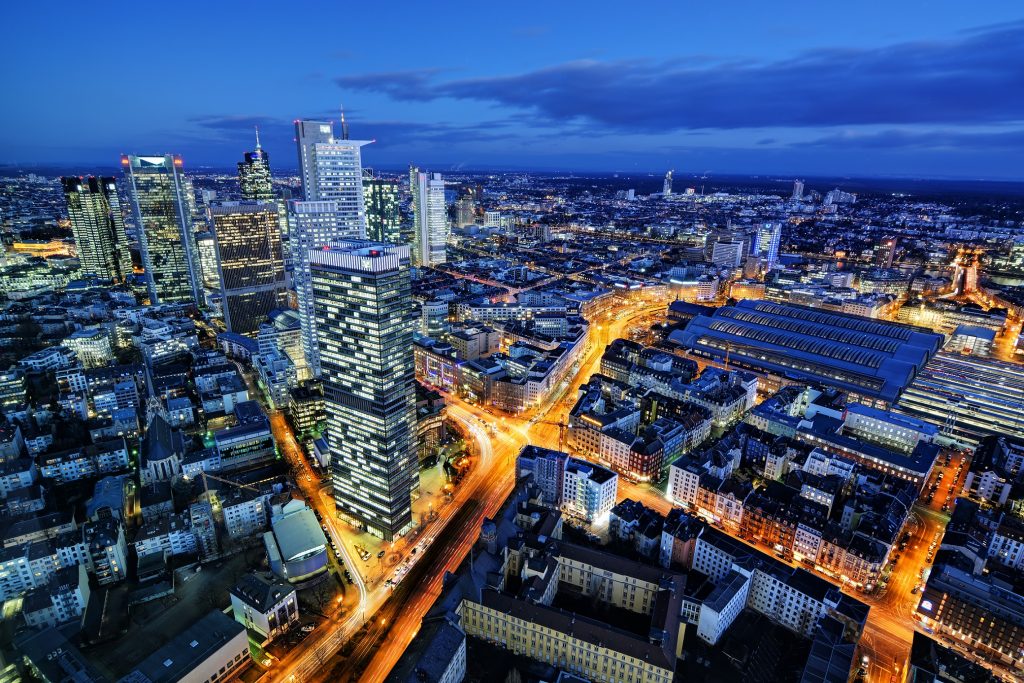
New regulatory requirements for insurance companies – focus on Germany
Because of its importance in society, the insurance industry has always faced a considerable amount of regulatory requirements at the national (BaFin) and international (EIOPA) level. Although this is generally something to be welcomed, this also presents a range of different challenges – not least because the regulations have indirect effects and unintended side effects. […]
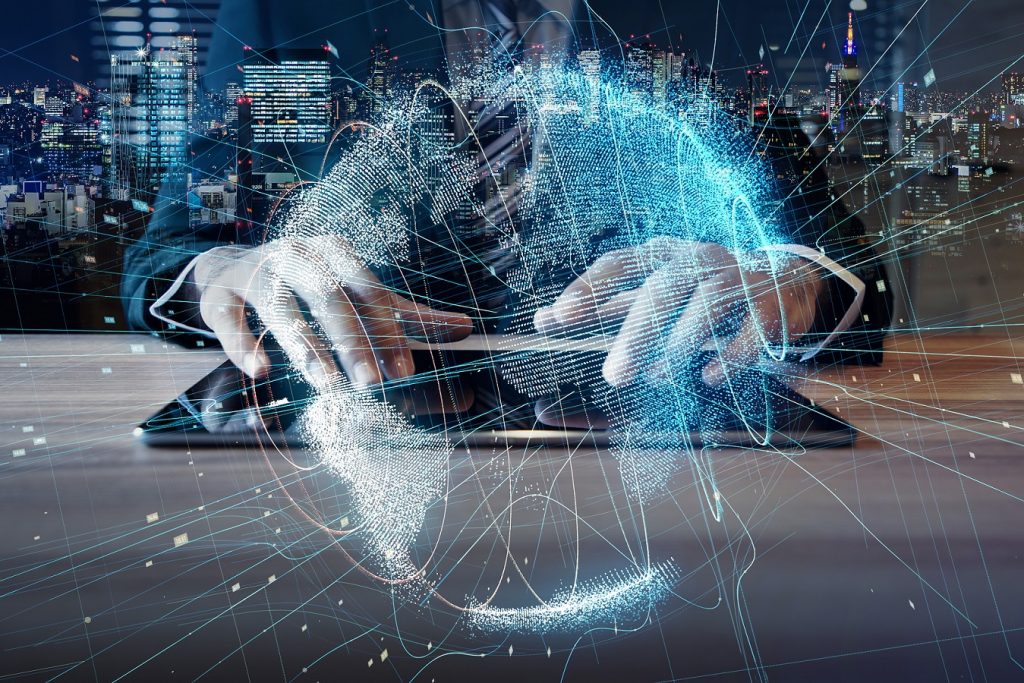
Digital transformation and integration with Enterprise Risk Management
Digital transformation has expanded the need for security, continuity and resilience. Today’s business must embrace an enterprise risk management strategy that includes legal, regulatory and political considerations. Enterprises today face a significant level of security challenges across their organizations. IT is no longer a secondary priority; it is now at the very heart of the […]
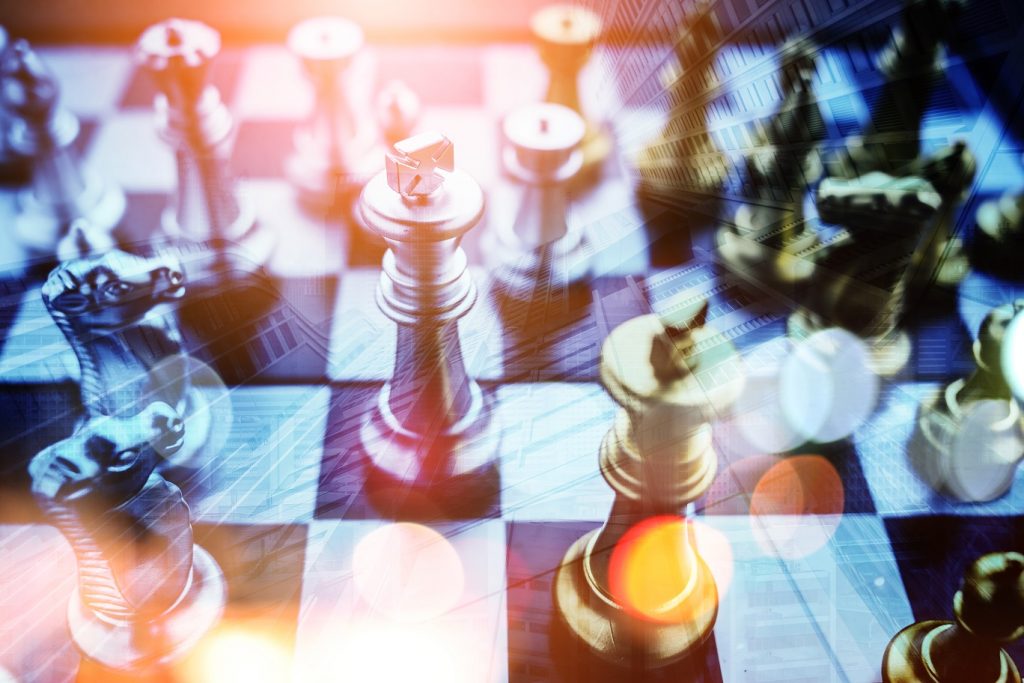
Managing Operational Risk
In recent years, we have seen a tremendous surge of interest in measuring and managing operational risks, both as a result of regulatory developments in corporate governance and capital adequacy, as well as due to a growing realisation that an enterprise-wide view of risk management is simply good business (those familiar with ISO9001 Quality Management […]
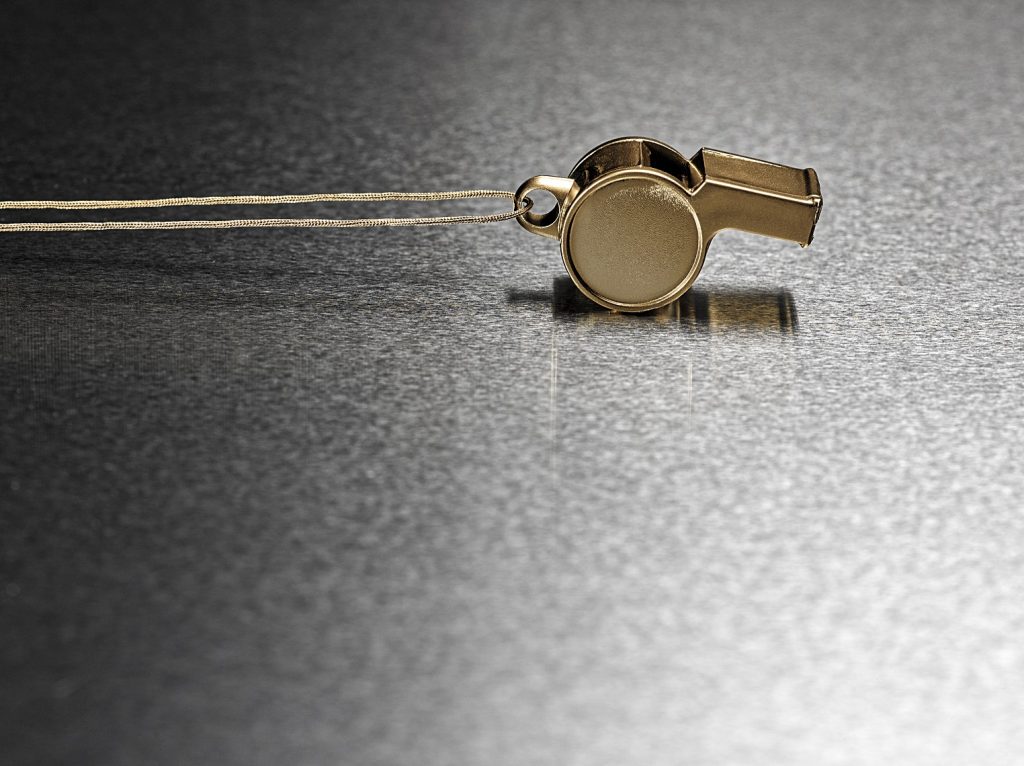
Whistleblowers: a path to combatting fraud
While whistleblowing laws and initiatives might differ from country to country, whistleblowers in the financial industry are now well recognized and have gained international attention. Here we analyse the steps taken in the USA to strengthen whistleblowing procedures and practices. Recent allegations of impropriety on several levels have rekindled whistleblowers as a mechanism for identifying […]
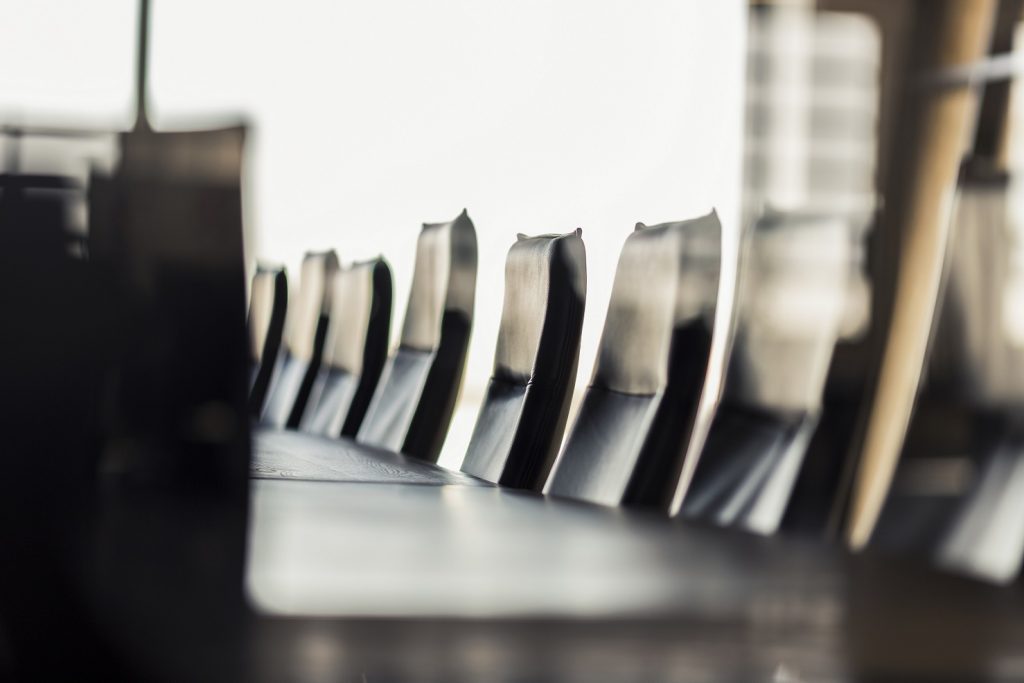
The Basel Committee finalises the Basel III agreements
On 7 December, after several years of negotiations (starting in 2012 with the market risk review), Mario Draghi, chair of the Basel Committee’s Group of Governors and Head of Supervision (G-HOS), announced the finalisation of the Basel III agreements. These represent the final stage in the post-financial crisis reforms to the prudential regulations. For the […]
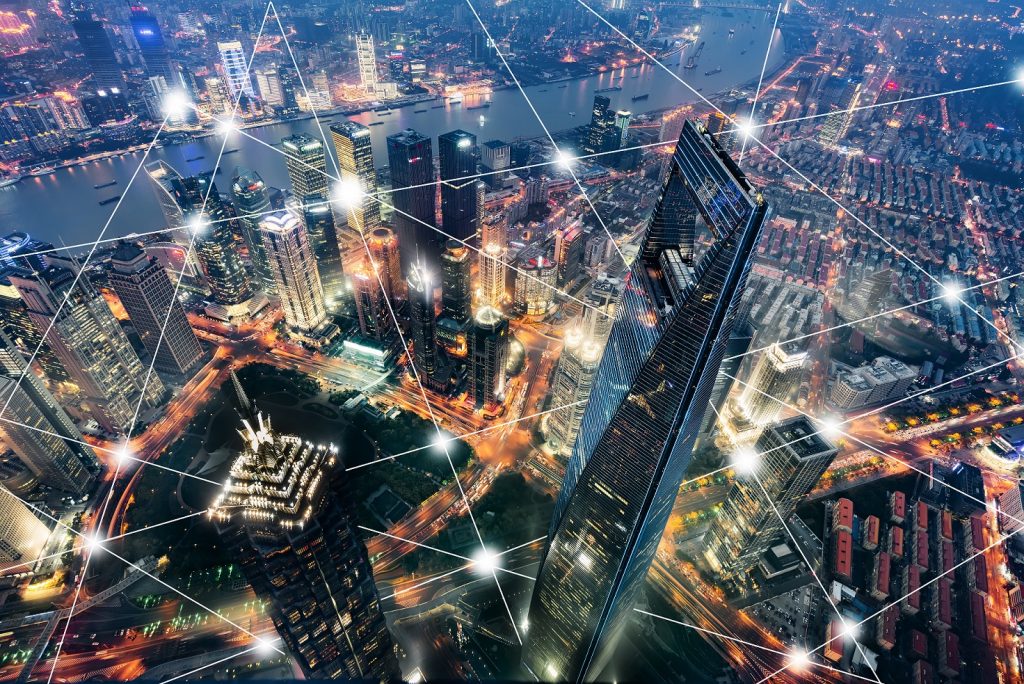
A sector undergoing transformation – changes in insurance companies’ business models
In the financial industry in general and the insurance sector in particular, traditional business models are increasingly coming under pressure – a trend that reflects technological, economic and social changes. At first glance, this represents a risk for insurance firms, since conventional business models such as life assurance are hardly profitable in this new environment. […]
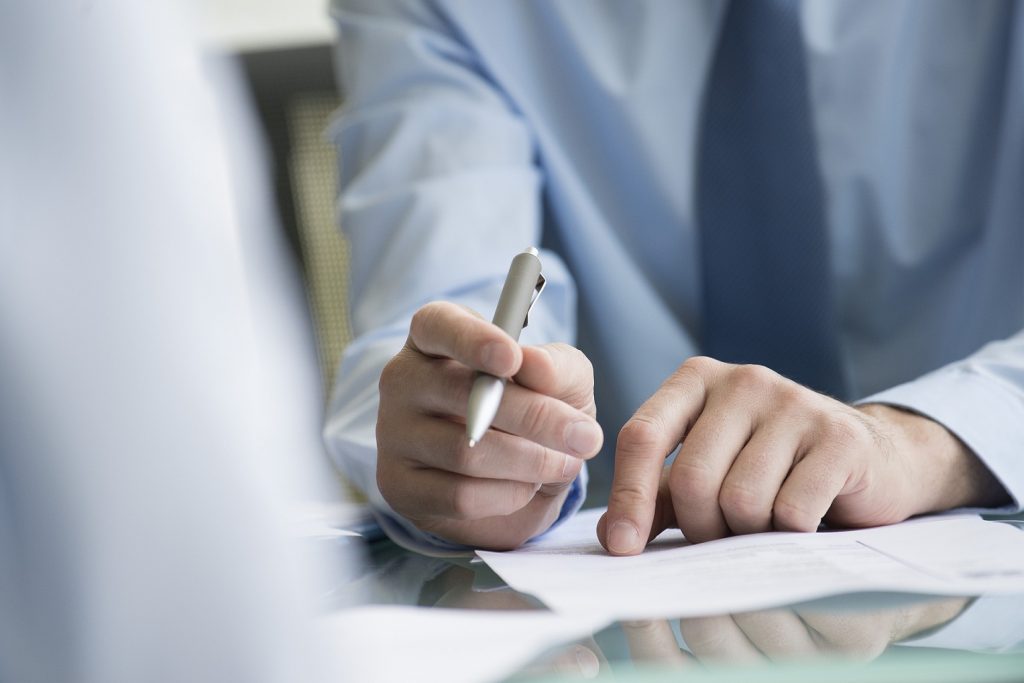
Introduction of prudential backstops for non-performing loans
Non-performing loans (NPLs) remain at the forefront of the European regulatory agenda, with two major consultations run by the European Central Bank (ECB) and the European Commission (EC) over the recent weeks, both in relation to the introduction of minimum prudential backstops. NPLs, a longstanding hot topic in Europe In the aftermath of the great […]
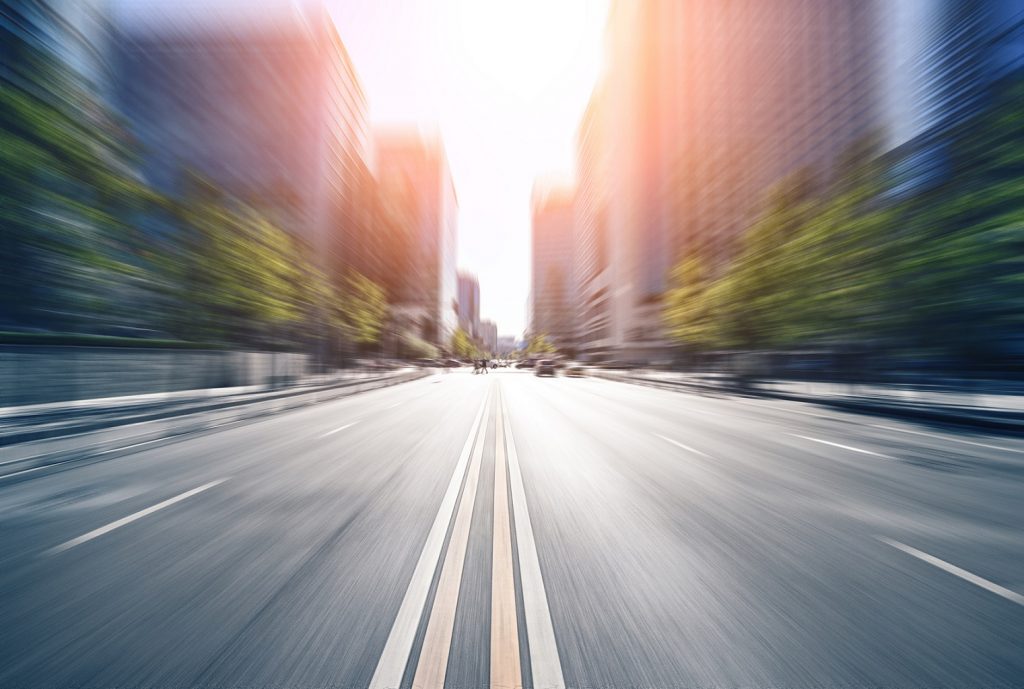
PSD2 supersedes PSD1 on 13 January 2018: comply with the minimum requirements or embrace the future?
PSD2 will supersede PSD1 on 13 January 2018, retaining key benefits from PSD1 such as increased competition with facilitated market entrance for regulated non-bank players, improved economies of scale, enhanced transparency, but also incorporating technological innovation, enhancements and protection into law. This will ensure that a competitive playing field continues to develop, without exposing individuals, […]
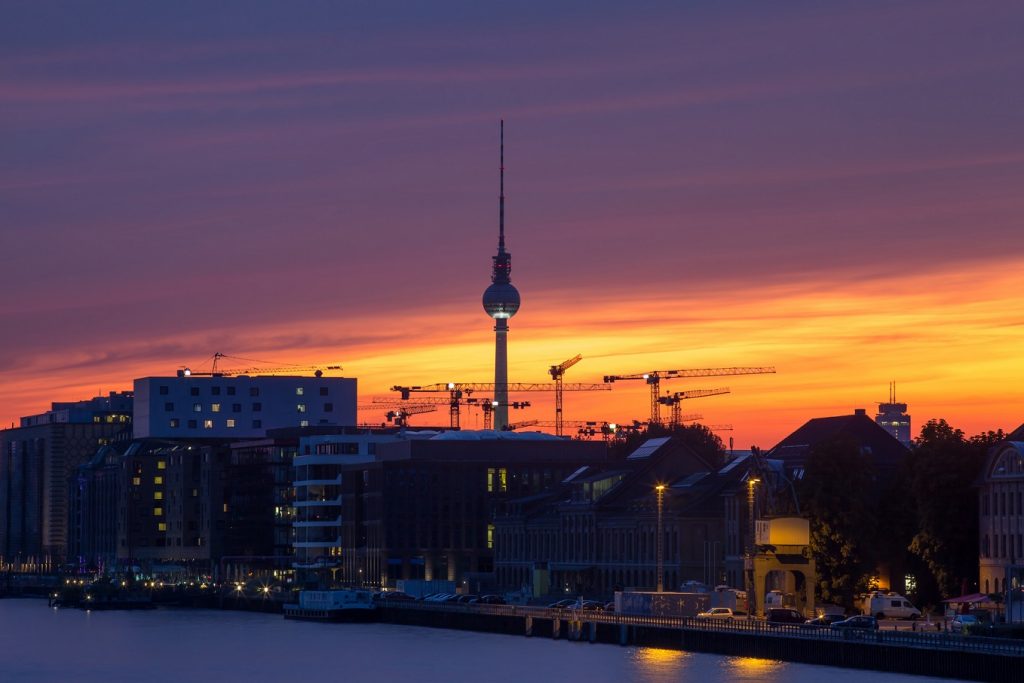
Action needed by foreign investment funds affected by new German Investment Tax Act
The new Investment Tax Act in Germany introduces new rules on investment taxation in the country. Coming into force on 1st Jan 2018, a widespread reform will apply to domestic and foreign investment funds with the transfer of transparent to non-transparent taxation status at fund level at a rate of 15% plus solidarity surcharge. Importantly, […]
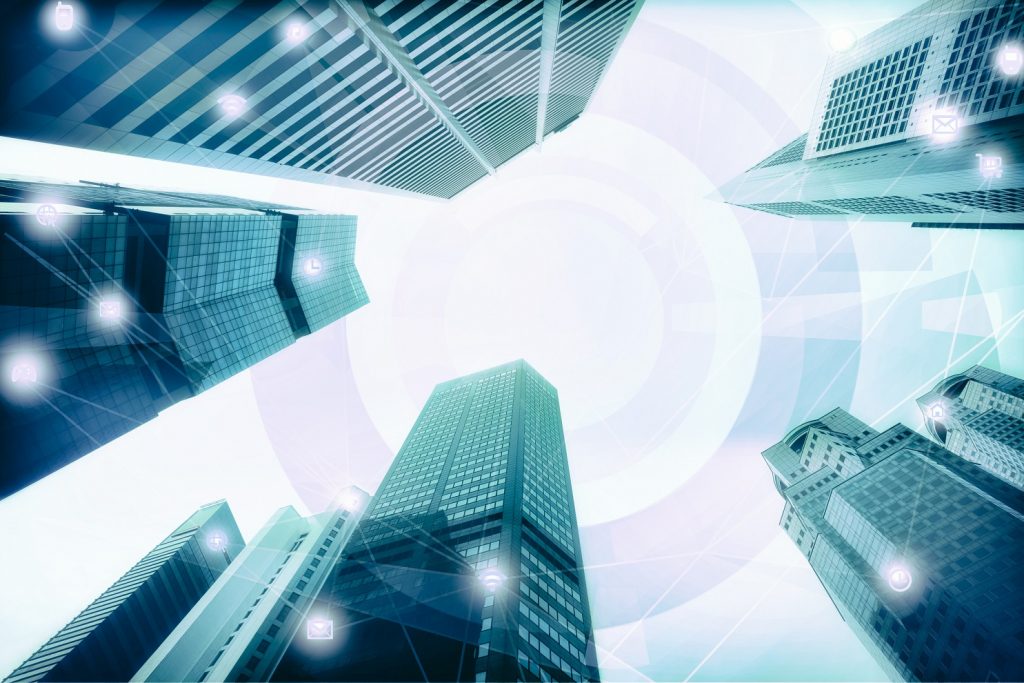
The banks’ business model – between function and transformation
The vast array of legal and supervisory requirements for determining a business model draws attention away from the inherent and indispensable need that a successful entrepreneur has for such a model. In the past, was an entrepreneur who had decided to run a bank ever asked about their business model? What is a business model? […]
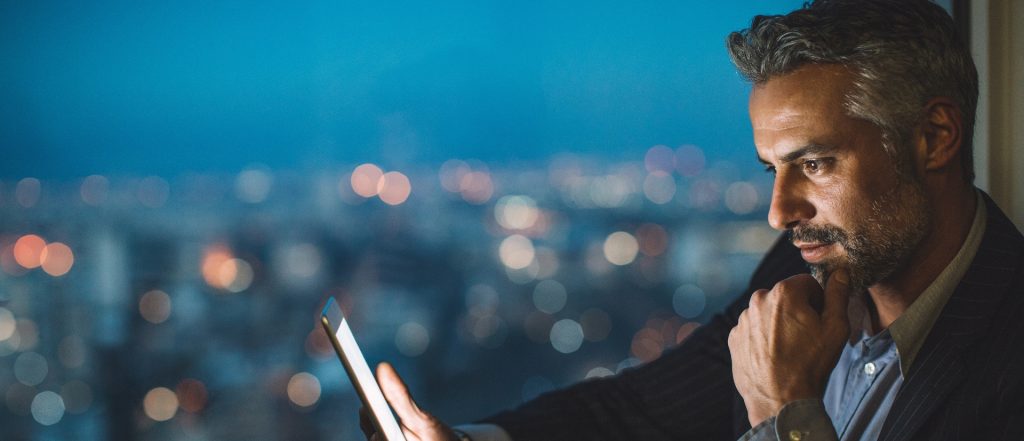
Embracing a pattern of change: business model innovation across banking, insurance and asset management
In this third article of our thought leadership programme around the transformation of Financial Services, we are focusing on how new business models are emerging. “THIS IS A WORLD OF SIX-MONTH PRODUCT DEVELOPMENT CYCLES AND CONSTANT UPDATES, PRIMARILY OF SOFTWARE, WITH A HUGE PREMIUM ON SIMPLE USER INTERFACES AND TRUSTED SECURITY.” Christine Lagarde, Managing Director […]
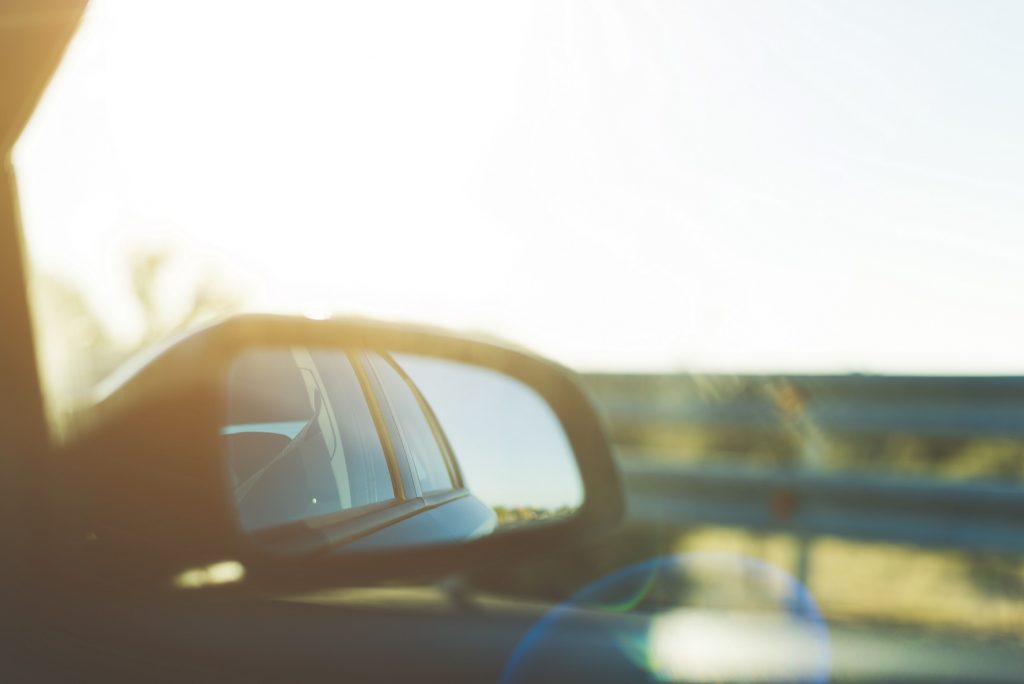
From Surrey to Sofia – making insurance a key driver in the race for better road safety
I was recently invited to speak at the 25th birthday conference of the Bulgarian Insurance Association in Sofia. The request came from my colleague, Thanos Petropoulos, who runs Mazars Bulgaria. As an integrated, international partnership, opportunities to work with our colleagues across borders is one of the most enjoyable parts of working at Mazars. This […]
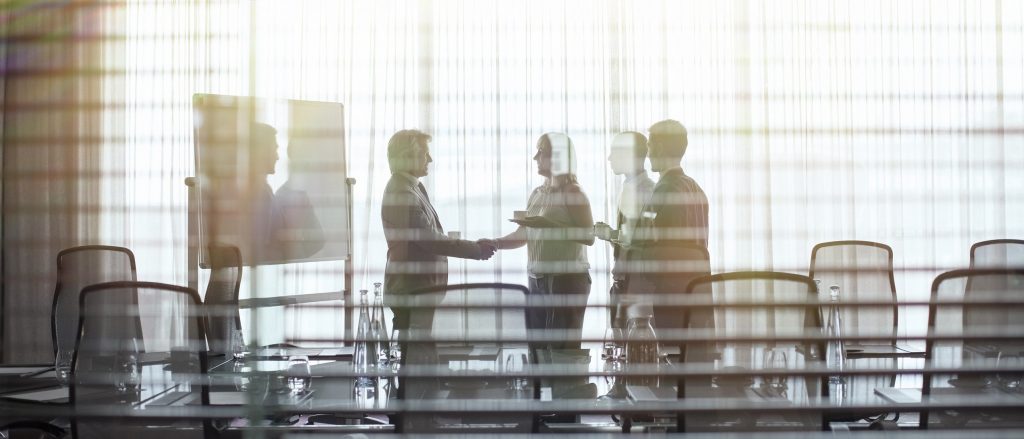
The Six Key Themes of Internal Governance Harmonisation
As recent as 26 September 2017, the European Banking Authority (EBA) under the Directive 2013/36/EU published its revised guidelines on harmonising the internal governance arrangements of banks across the European Union. The EBA Guidelines will apply as of 30 June 2018 to competent authorities across the EU, as well as to institutions on an individual […]
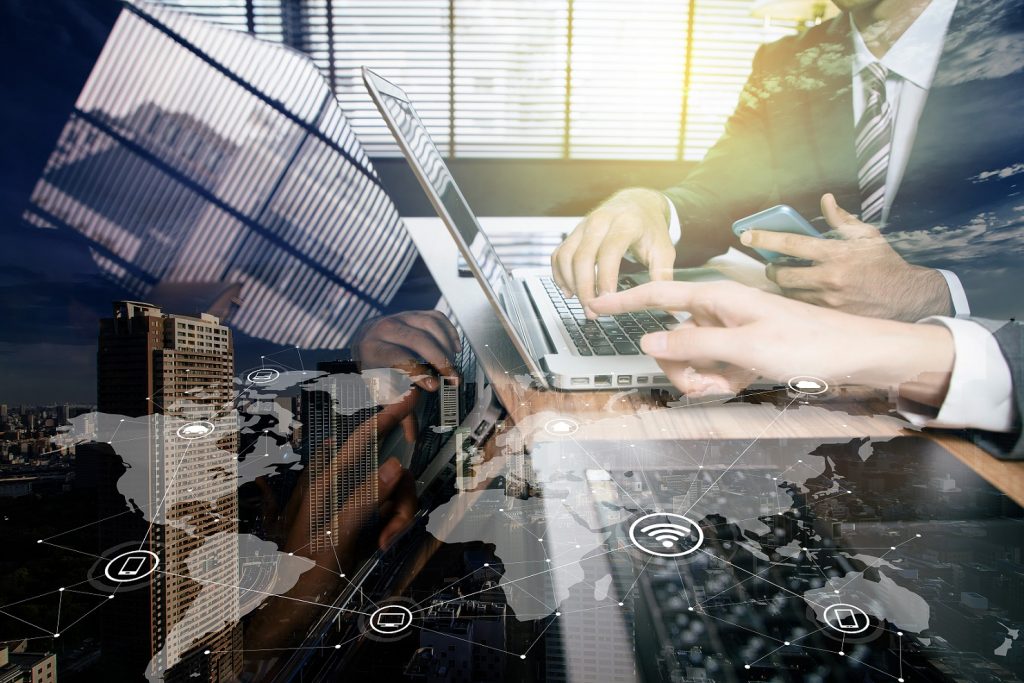
Could regulation play a role in transforming the FinTech business model?
One of the main differentiators FinTechs have is their current positioning within the financial services value chain ecosystem: they focus on customer experience while their operations execution and control activities are often outsourced to banking institutions. However, is this likely to remain given the increasing interest regulators are paying to these new industry players? While […]
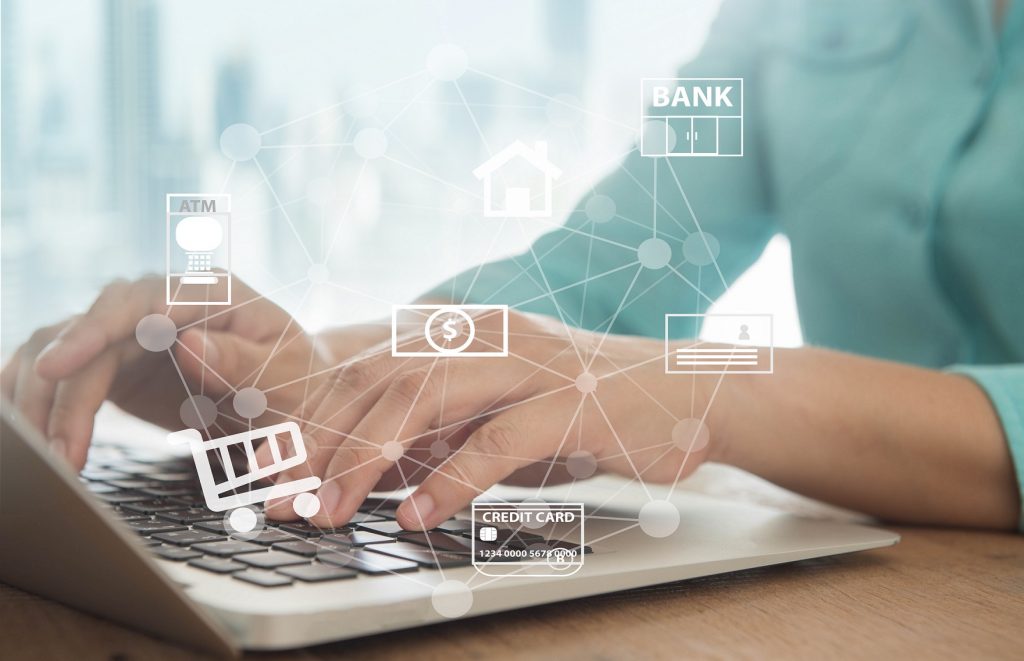
Rethinking Compliance for the Protection of Personal Data
A holiday photo on Instagram, a “like” on Facebook, a PayPal transfer to pay for your Airbnb, or just a bank card payment or an ATM withdrawal: every day, we feed data into greedy and enthusiastic algorithms that are gathering our personal information. A new European regulation adopted in response to this irrational frenzy, the […]
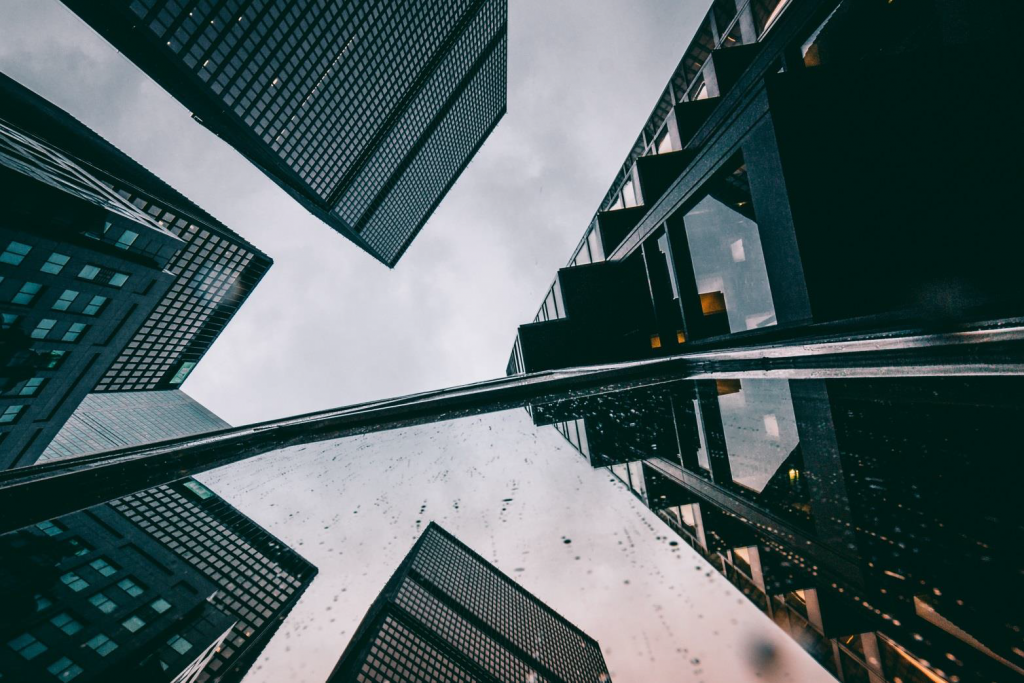
Let’s make the regulatory capital rule simple
On September 27, 2017, the Federal Reserve Board, the Federal Deposit Insurance Corporation and the Office of the Comptroller of the Currency (the “Banking Agencies”) issued a proposed joint rule to simplify several requirements in the Banking Agencies’ regulatory capital rule for banks. The proposed rule would apply only to banking organizations that are not […]
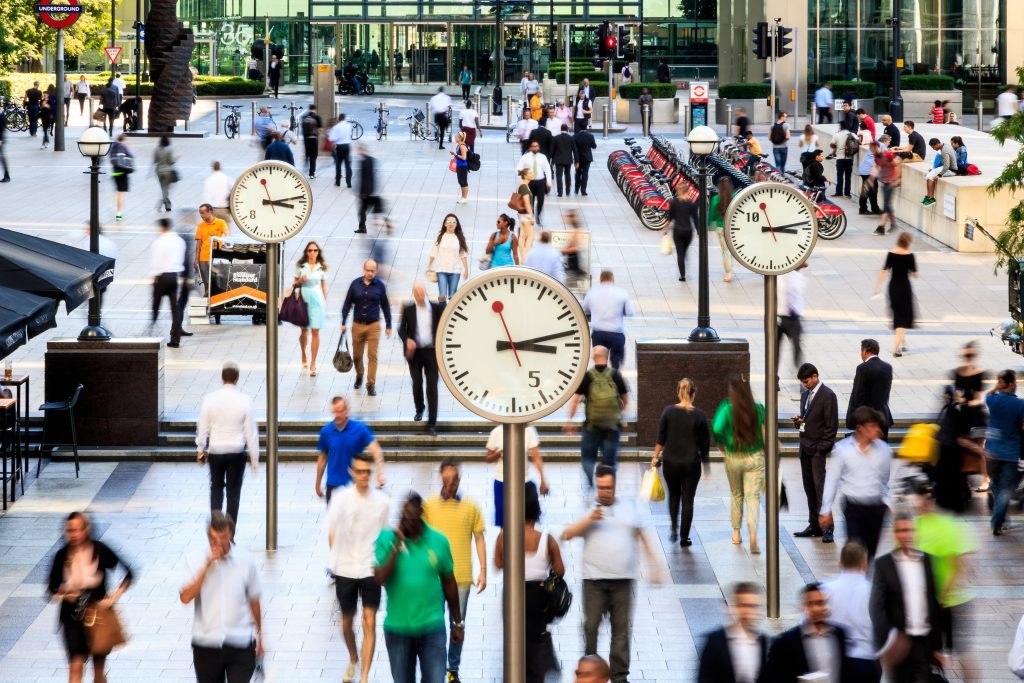
The clock is ticking
In August 2017, S&P Global Market Intelligence published an analysis of where banks will set up their European hubs. Germany, namely Frankfurt and Berlin, came first with 13 financial institutions. Dublin came a close second attracting 12 banks, insurers or asset managers. Luxembourg, France, the Netherlands and Belgium will also take a share. A bit […]
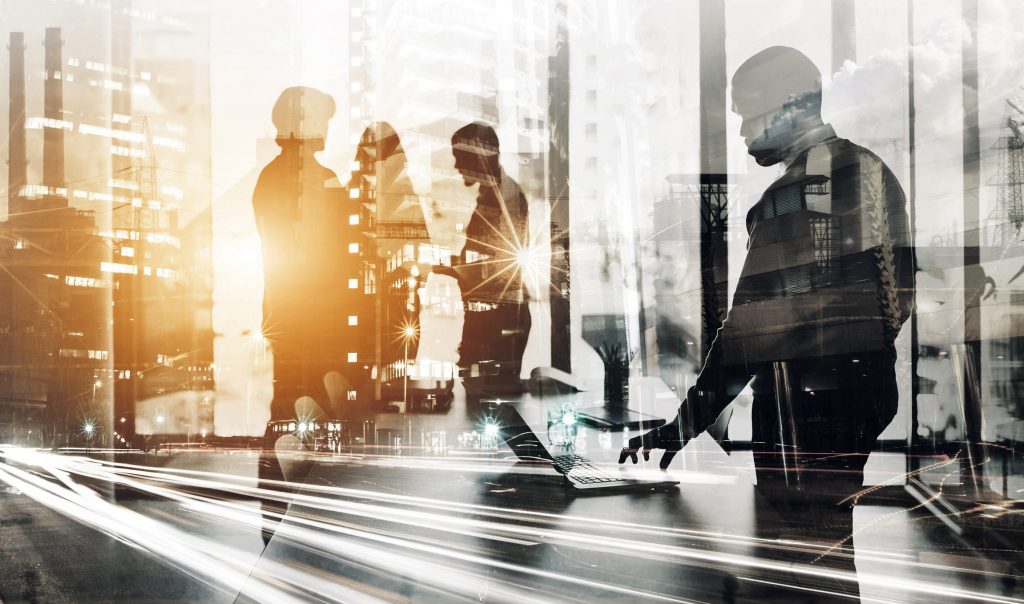
Action Plan for Banks in the West African Monetary Union
A new prudential framework applicable to banks and credit institutions in the West African Economic and Monetary Union (WAEMU) is set to come into force on 1 January 2018. However, a significant delay has been observed in implanting reforms in the majority of banks, where the scale and implications of the necessary work are widely […]
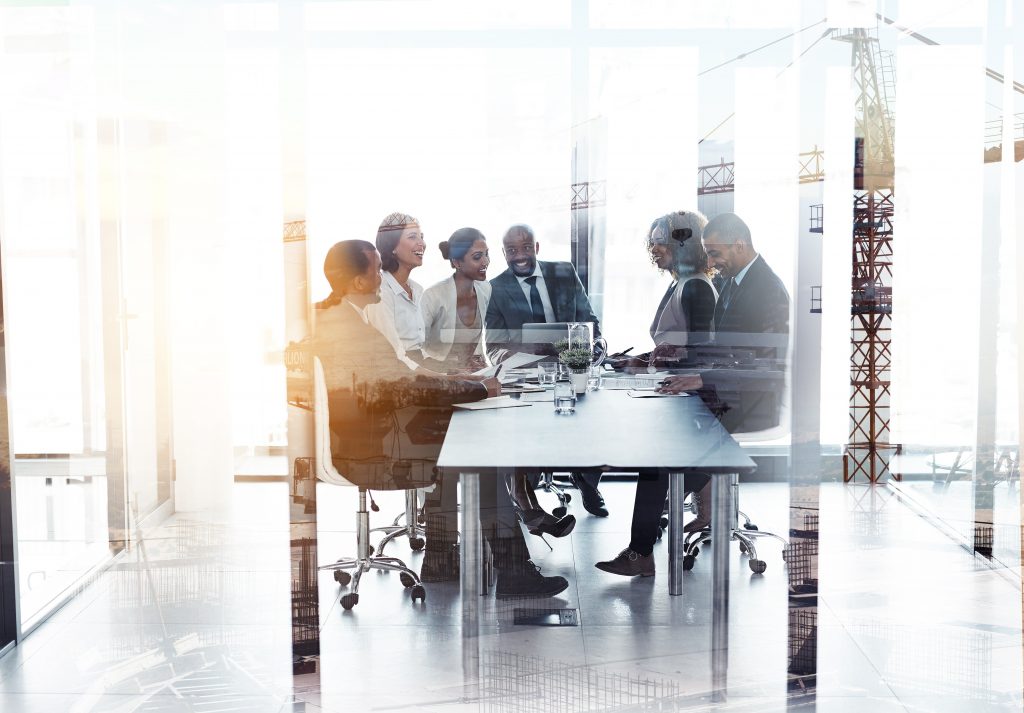
Implications of the Revised Banking Chart of Accounts for the West African Financial System
Reforms to an accounting system that has been in force for nearly 20 years will help to increase the security and robustness of the West African financial system, while improving the quality of financial reporting. The Revised Banking Chart of Accounts has been adopted by the monetary authorities in the course of convergence towards Basel […]
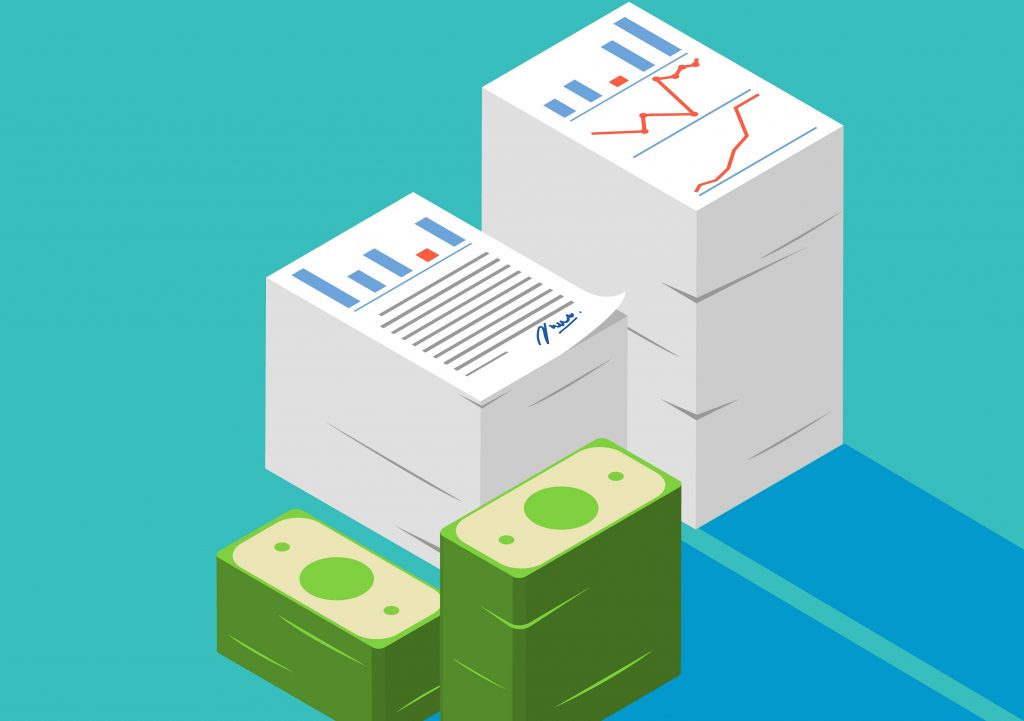
Regulatory reform in uncertain times: no rest for the weary
Despite “reform fatigue” and squeezed profit margins, financial services firms are dealing with a continued flood of regulation. Some are turning to new technologies for compliance help. In this article, written by the Economist Intelligence Unit and sponsored by Mazars, we explore regulatory change in the financial services sector and the impact that it has […]
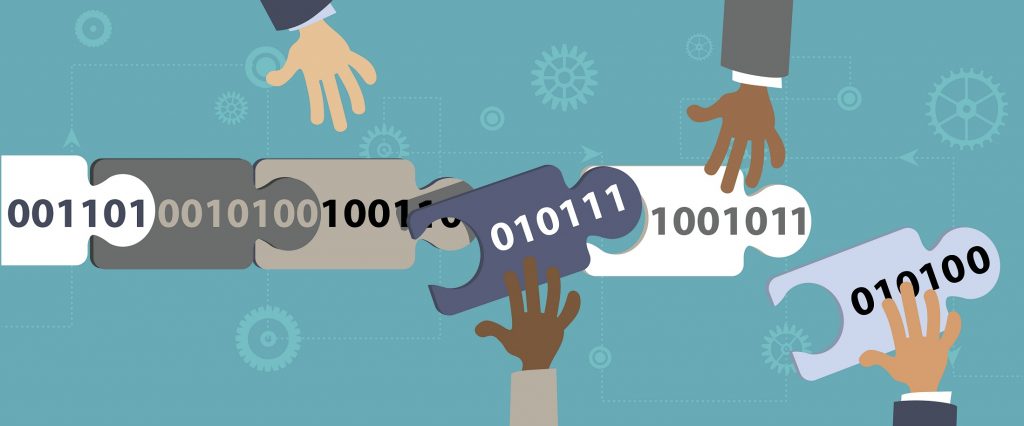
Are ICOs the New Future of Start-ups?
The term “ICO” is a common buzzword in investment circles these days. While these “Initial Coin Offerings” are distant cousins of IPOs (in the sense that both share the objective of helping firms raise capital from various sources), their likely timing and means of achieving funds are quite different. An ICO is a form of […]
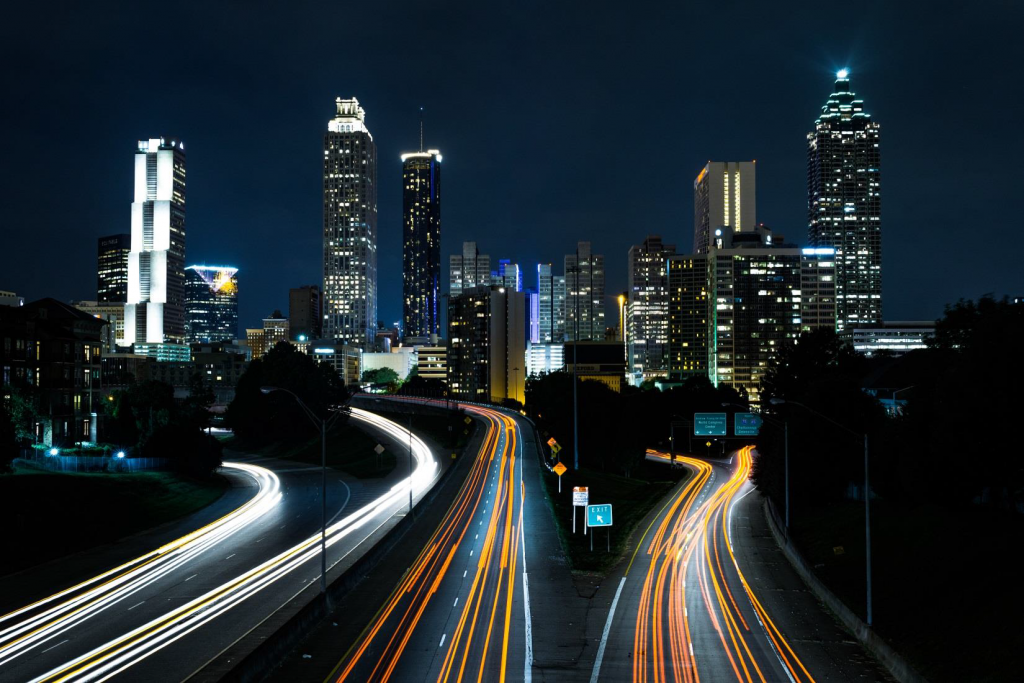
The Evolution of Banking
Over a decade ago looking at the state banking webpage for my home state of New Jersey, there were approximately 250 banks doing business in the state then. A similar check today would yield a list of less than 150 or so; a more than 40% decline. With banking regulation at an all-time high, the […]
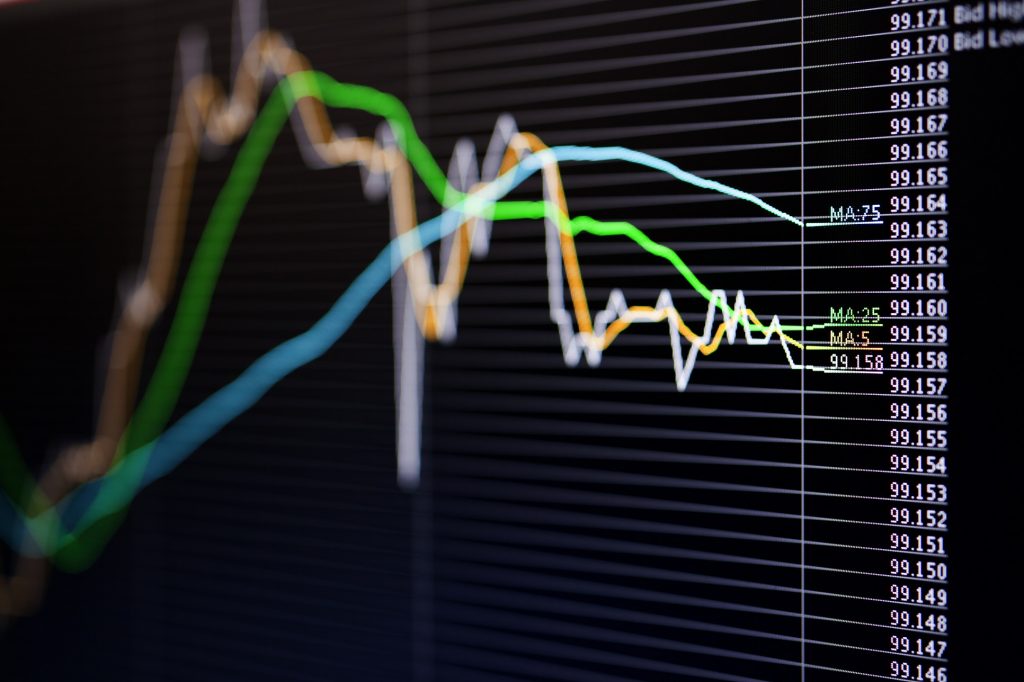
The Emergence and Significance of ‘FX GLOBAL CODE-2017’
The FX Global Code (Code) was finally published by the Foreign Exchange Working Group (FXWG)[1] on 25th May 2017, clearly laying down the guidelines for good practices in the FX Wholesale marketplace. With numerous scandals causing turbulence in the FX marketplace in the past, it was deemed necessary for global bodies to roll out a […]
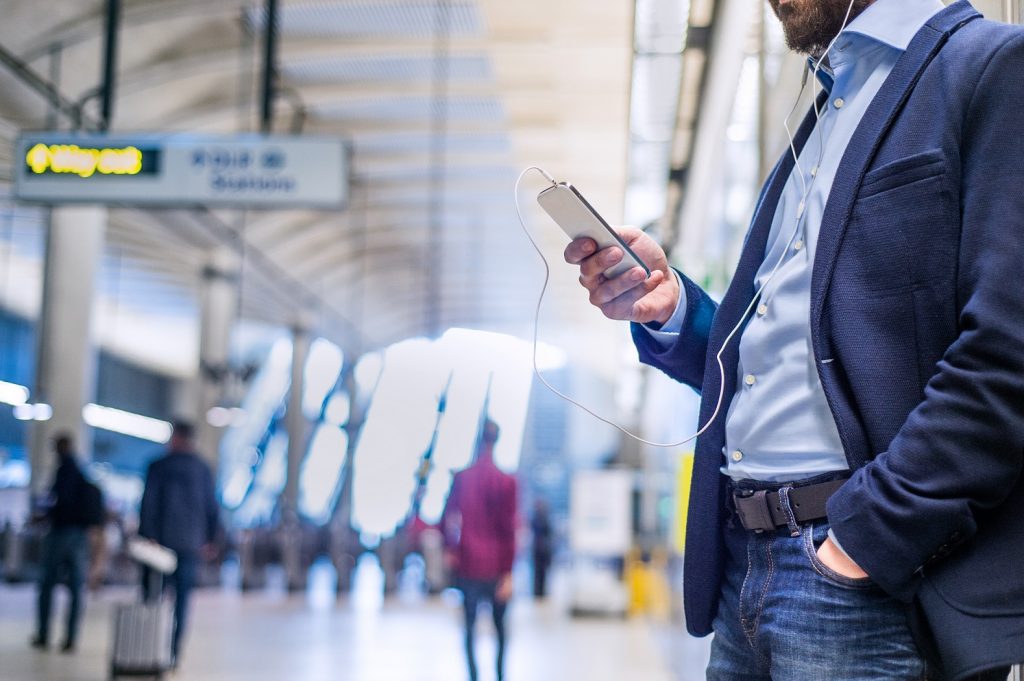
Podcast: Banking Regulatory Outlook
Along with Mazars banking regulatory advisory specialists Pauline Pelissier and Audrey Cauchet, Greg Simpson provides an overview of the banking regulatory outlook in the UK and Europe over the next 12 to 18 months. They also discuss IFRS9 in the context of regulatory treatments, credit risk management, non-performing loans, CRD5 and CRR2. Podcast player
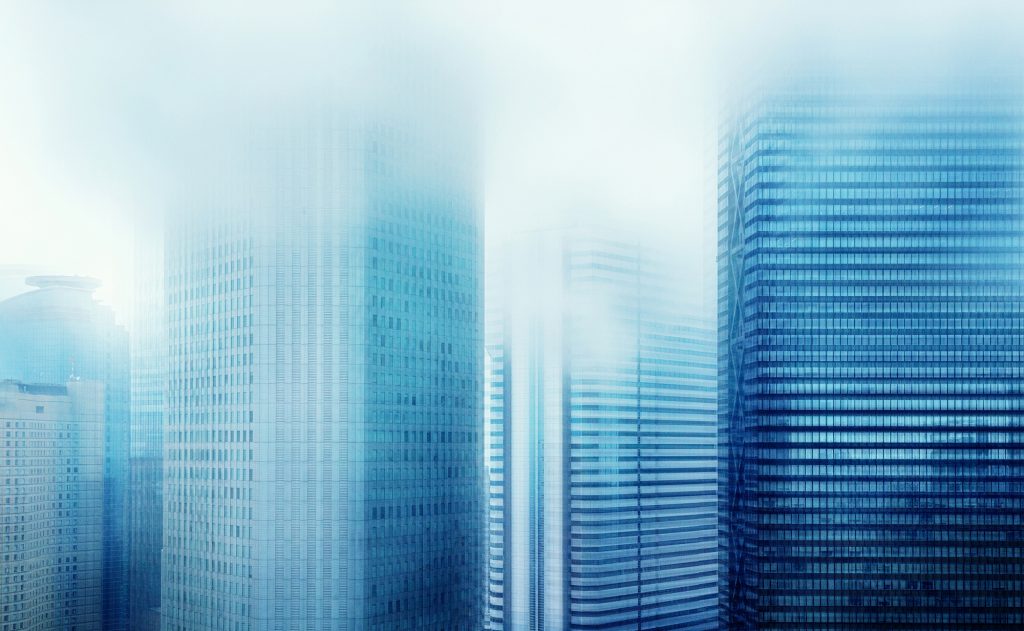
Asset Management sector under scrutiny from UK regulator
The Asset Management industry is positioned to be the main focus of the Financial Conduct Authority (“FCA”). On 28 June 2017, the FCA published the final report of its Asset Management Market Study. This follows on from the interim report published in November 2016. In the publication, the FCA has highlighted a number of pertinent […]
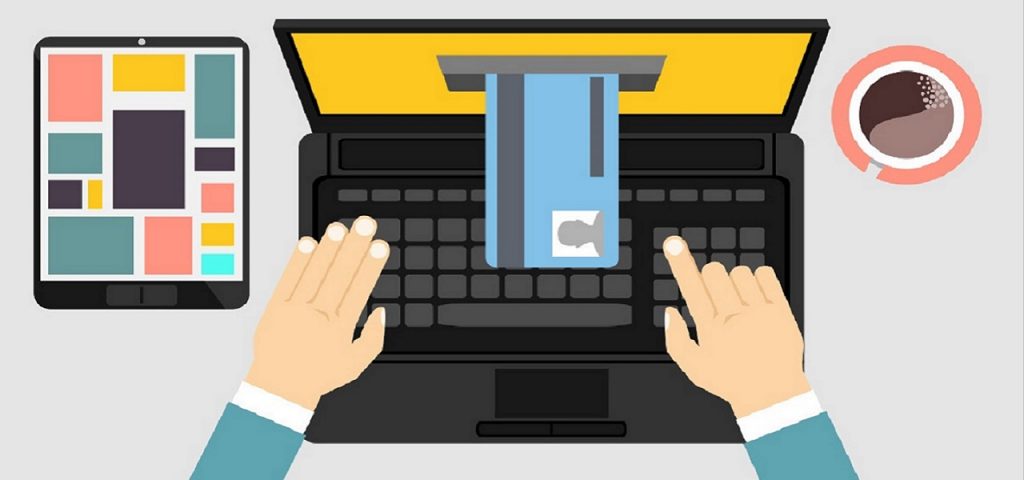
The marriage of high tech and high finance
The lines between financial services and high technology are becoming increasingly blurred. Four major technological changes will disrupt financial services in the years ahead. In this article, written by the Economist Intelligence Unit and sponsored by Mazars, we are discussing how technological disruptors are impacting the global financial services industry. We review how C-suite financial services executives, […]
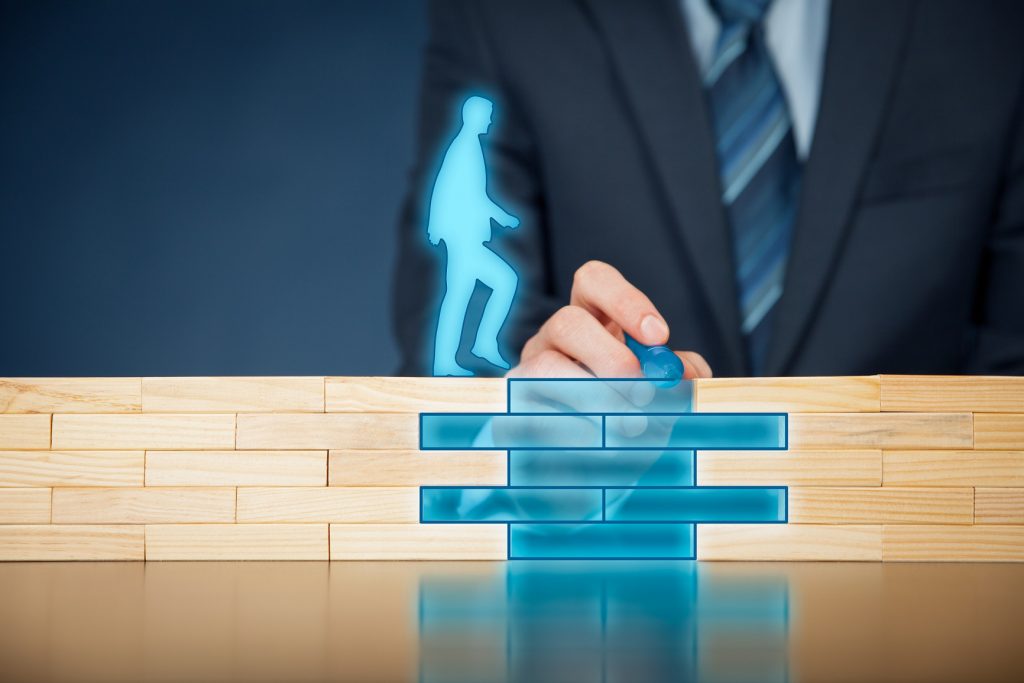
How insurers can make sense of risk
With Solvency II fully in force, the insurance industry has entered a new phase of transformational development. For many insurance companies, Solvency II has provided the opportunity to make better sense of risk and yet, insurance companies continue to operate using risk management programs that have not evolved and may not protect them from the […]
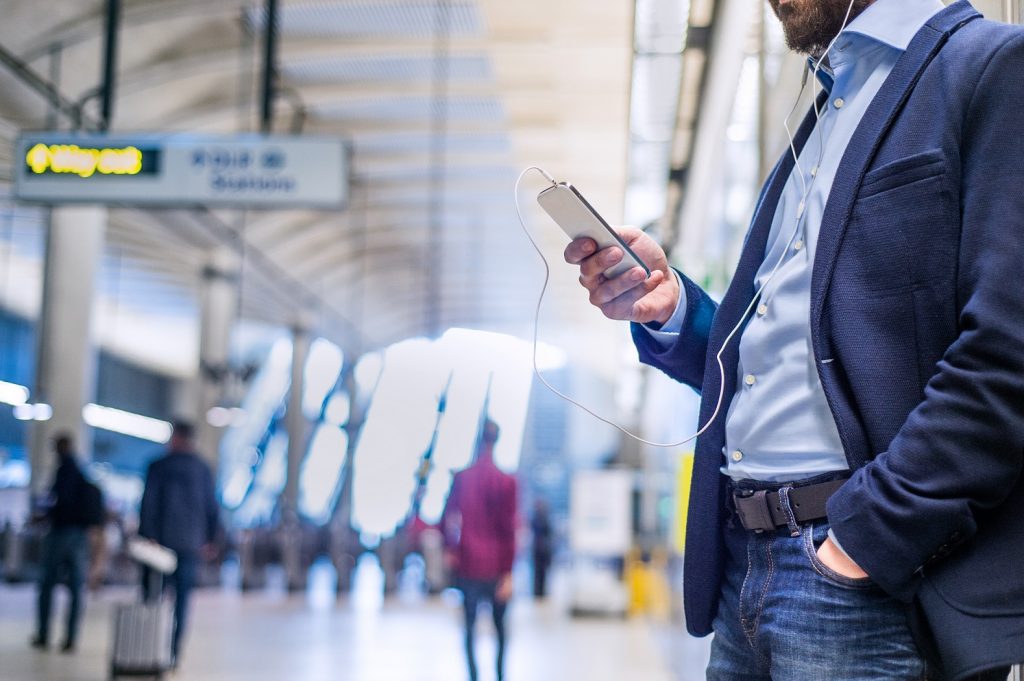
Podcast: Why banks need to address cyber security
In this podcast Greg Simpson discusses cyber security with our expert Francisco Sanches. They discuss major threats such as emerging risks, FCA guidance on cloud data storage and the cyber security skill gaps to name a few. Podcast player
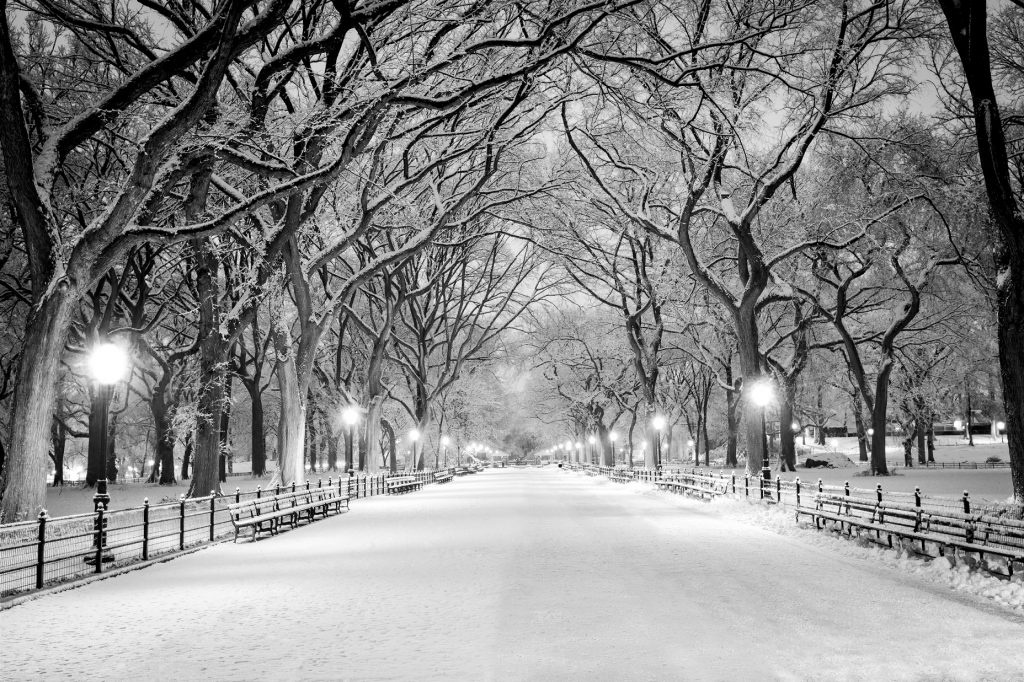
TRIM: Is Winter Coming for Internal Models?
Measuring banking risks is a difficult exercise, but striking a balance between simplistic and overly complex measurement techniques is the key to accurate risk measurement. This was the substance of the European Central Bank’s (ECB) Chair of the Supervisory Board, Danièle Nouy, in a speech at the Austrian Chamber of Commerce in Vienna, on 2nd […]
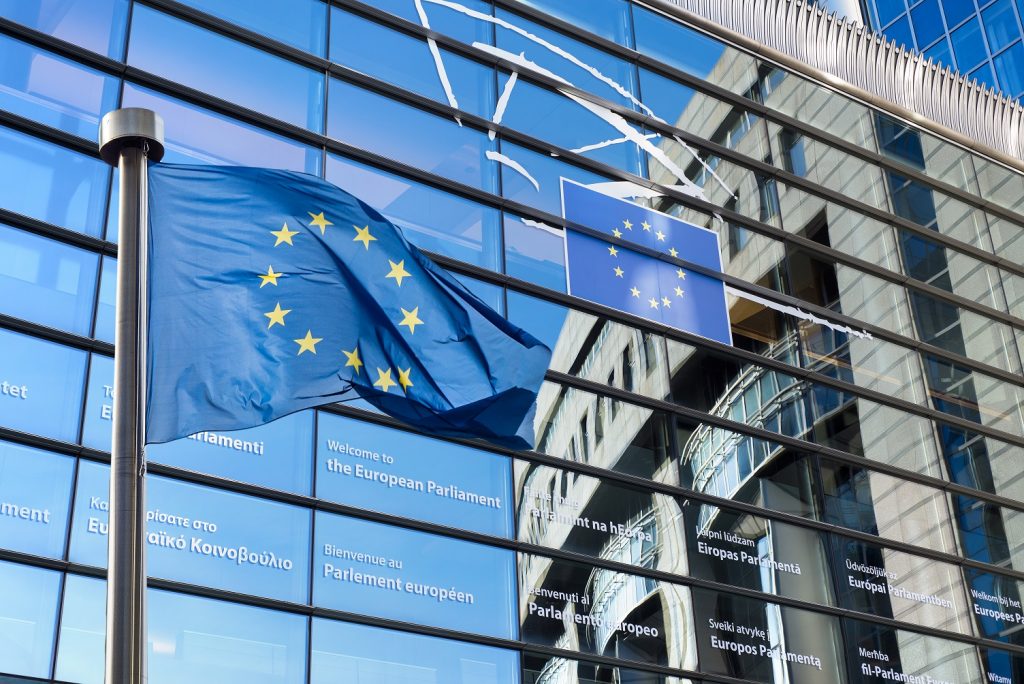
Four routes to better mobilisation of capital in Europe
Efficient supervision of capital markets is a priority of the Capital Markets Union (CMU) given its role in facilitating market integration and European cross-border transactions. But there are currently a number of supervisory barriers halting progress of European mobilisation of capital that now need to be addressed urgently. Following discussions at the CMU’s mid-term conference […]
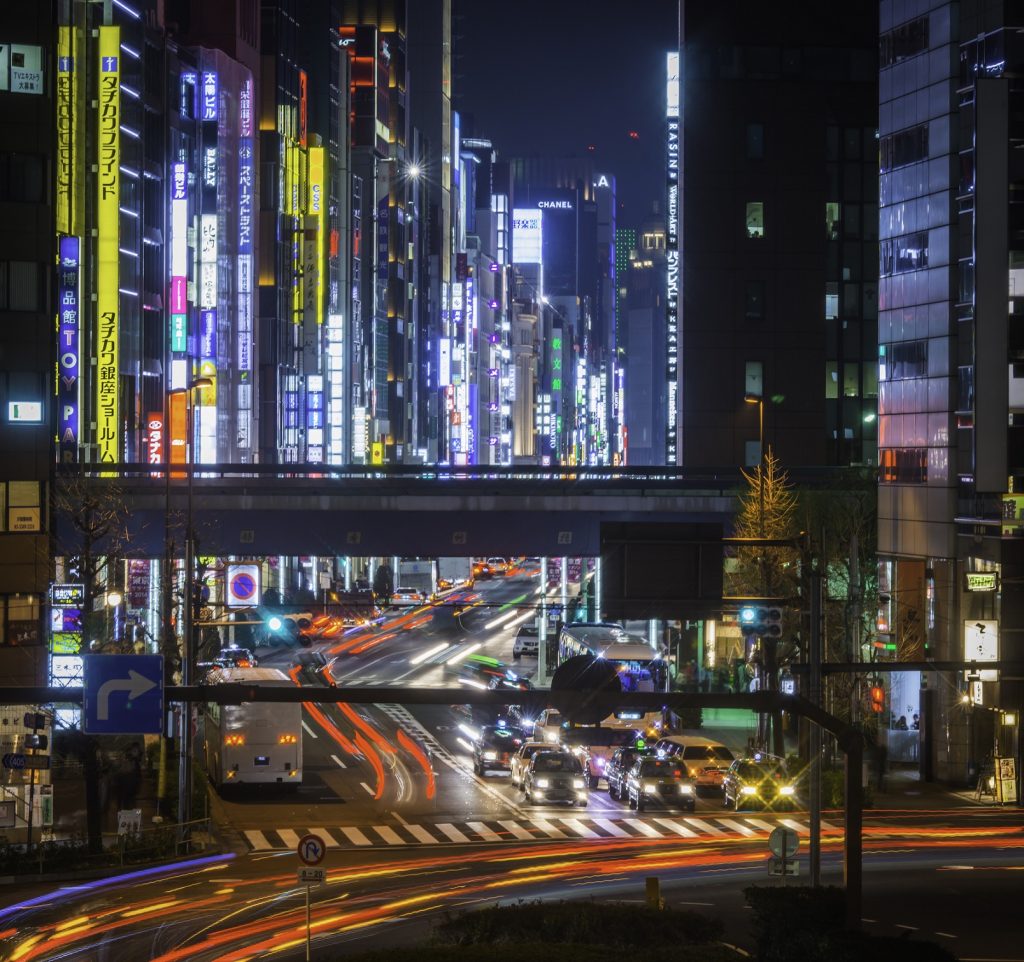
FinTech in Japan: a regulatory framework on the move to support a strong development
The field of money remittance transactions in Japan is expanding and is attracting many overseas companies looking to operate fund transfer and virtual currency exchange businesses. A regulatory framework for FinTech firms in Japan now presents major opportunities and challenges, not just in Japan, but for regulators around the world. To support progress and development […]
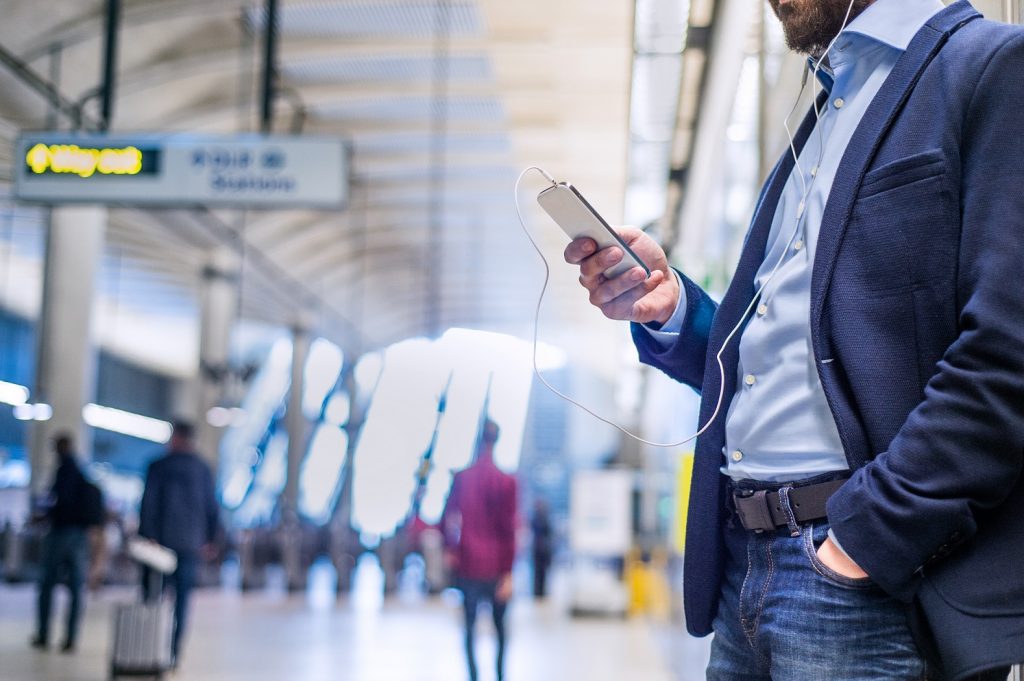
Podcast: Implications of Banks Implementing IFRS 9
Greg Simpson, Head of Banking UK and banking experts Paul Hodgett and Pierre Latrobe discuss the implications of IFRS9, more specifically they share some of their experience on helping banks implement IFRS 9. As the standard starts on 1 January 2018, they will also comment on some of the areas that banks need to consider […]
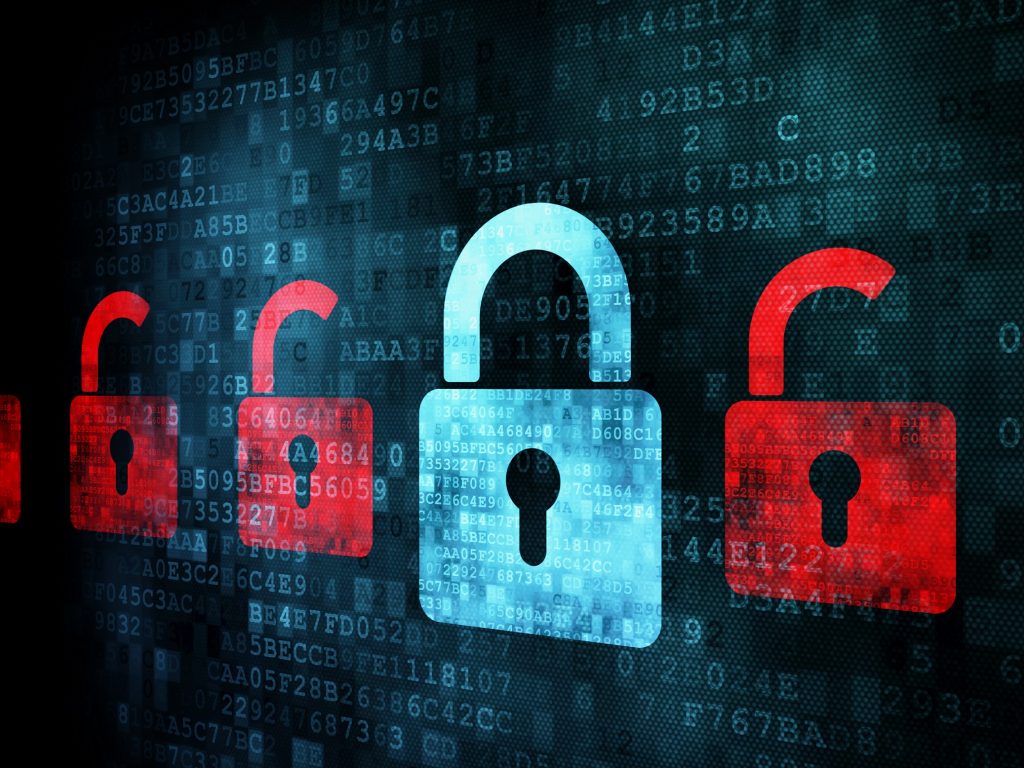
Data privacy – too strategic for boards to ignore
Personal data security is increasingly important, but many companies may not be ready to comply with the EU’s tough new data protection laws, which must be implemented by May 2018. All EU businesses that handle data will have to comply with the General Data Protection Regulation (GDPR), which will require investment in systems and training […]
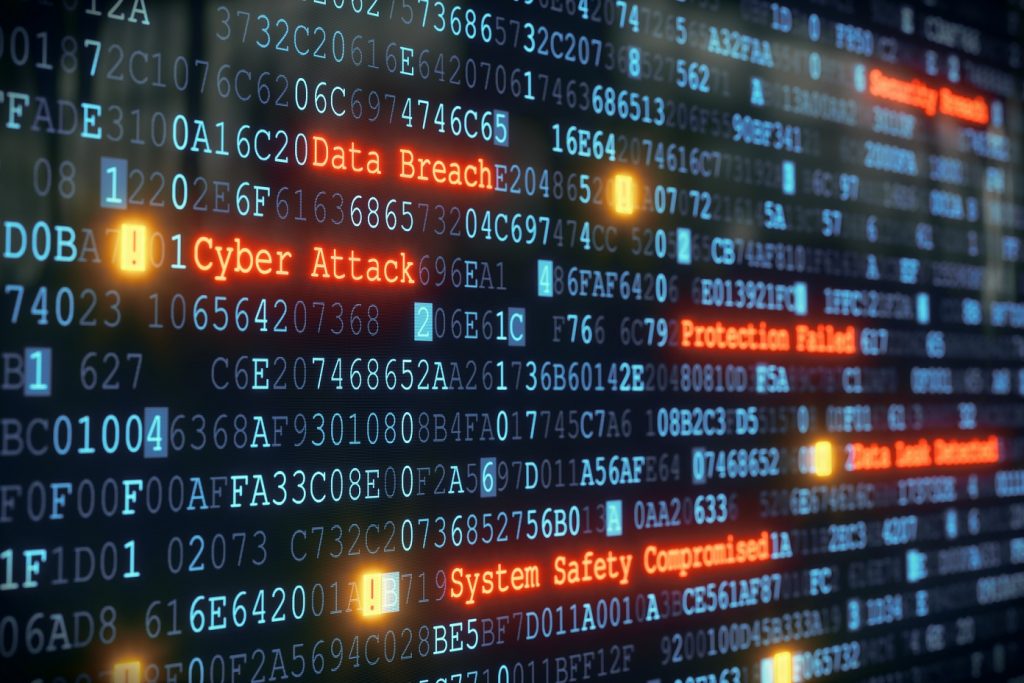
Evolving role of AI with cyber risk
Cyber as an organsational risk In 93% of data breaches, the targeted systems were compromised within minutes. 83% of the time, those breaches were not discovered for weeks, leaving the attackers with plenty of time to do their damage and exfiltrate data[1]. The average consolidated total cost of a U.S. data breach in 2016 was […]
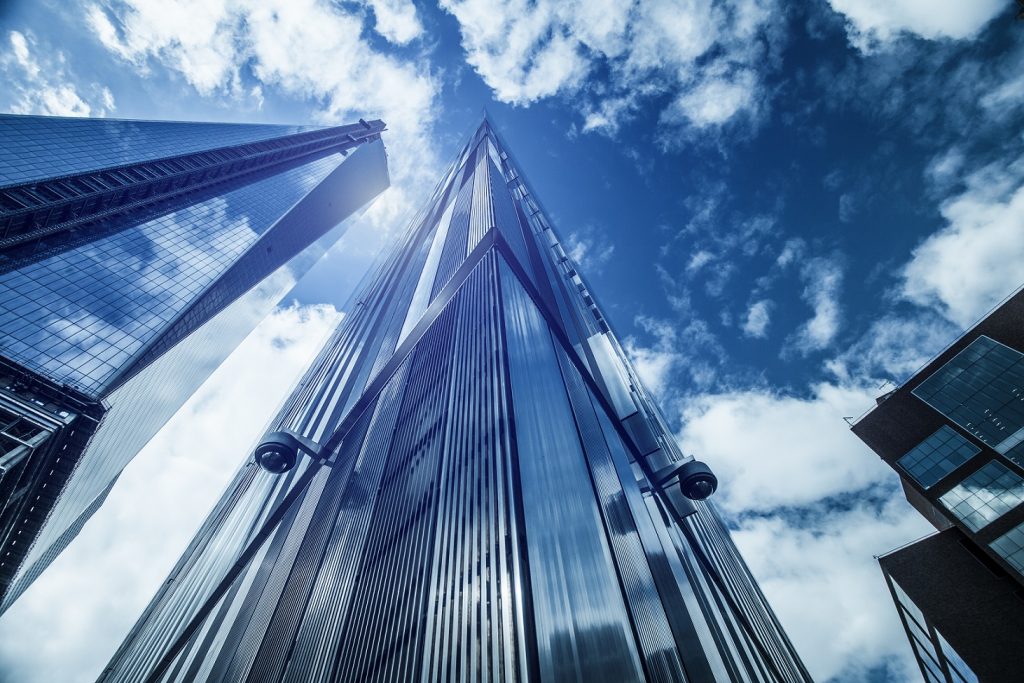
Unilateral deregulation by the USA would lead banks to seek new trade-offs
Solvency, liquidity, transparency and oversight requirements: as the Basel Committee meeting in Santiago, Chile in November 2016 demonstrated, there is still much to be discussed on the finalisation of the Basel III agreements. However, the inauguration of the new President of the USA could change the condition of the international dialog. Some might decide to […]
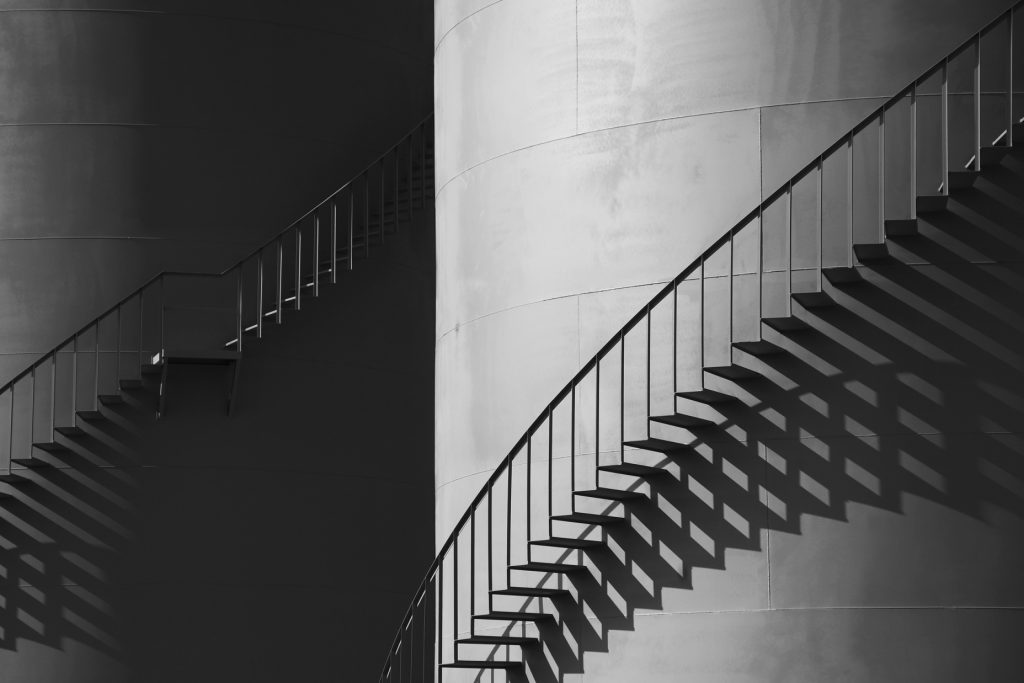
The Reduction of Regulatory Compliance Examinations for Financial Institutions
Examination Cycle for Certain Small Insured Depository Institutions and U.S. Branches and Agencies of Foreign Banks Under $1 Billion in Total Assets. The Federal Deposit Insurance Corporation (“FDIC”), the Federal Reserve Board (“FRB”), and the Office of the Comptroller of the Currency (“OCC”) issued joint final rules that increased the number of small banks and […]
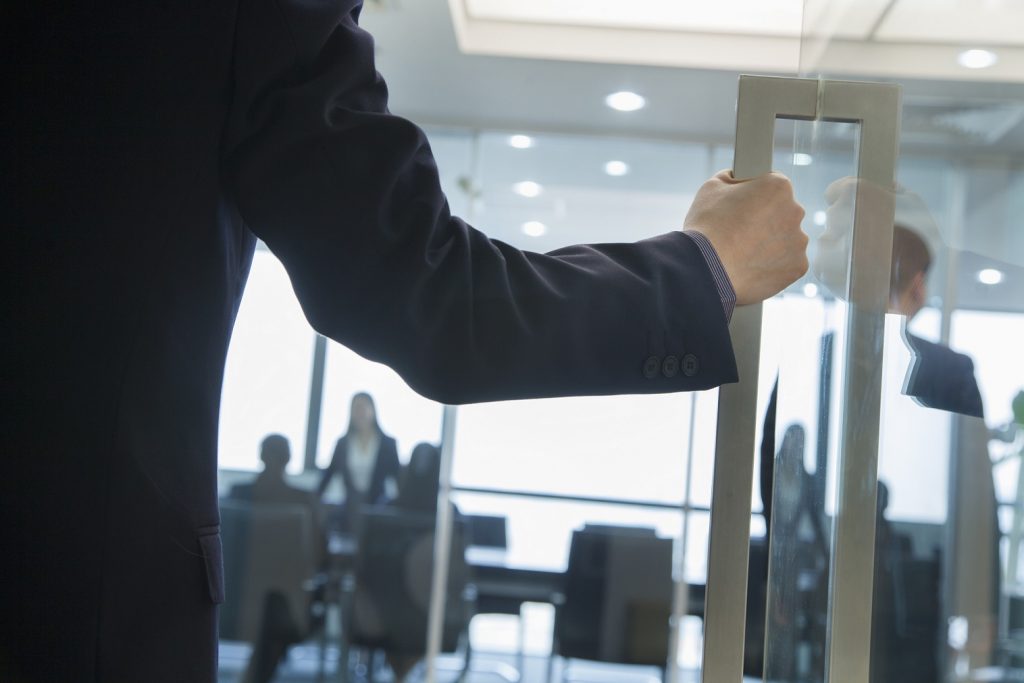
IFRS 9 new provisioning and the phase-in period of regulatory capital: a discretionary approach
In about nine months, IFRS 9 will replace IAS 39 and the new accounting environment won’t be the only element to be impacted. In recent months, the Basel Committee on Banking Supervision (BCBS), the EU Commission and the European Banking Authority (EBA) have also been tackling the impact of the new IFRS 9 provisioning on […]
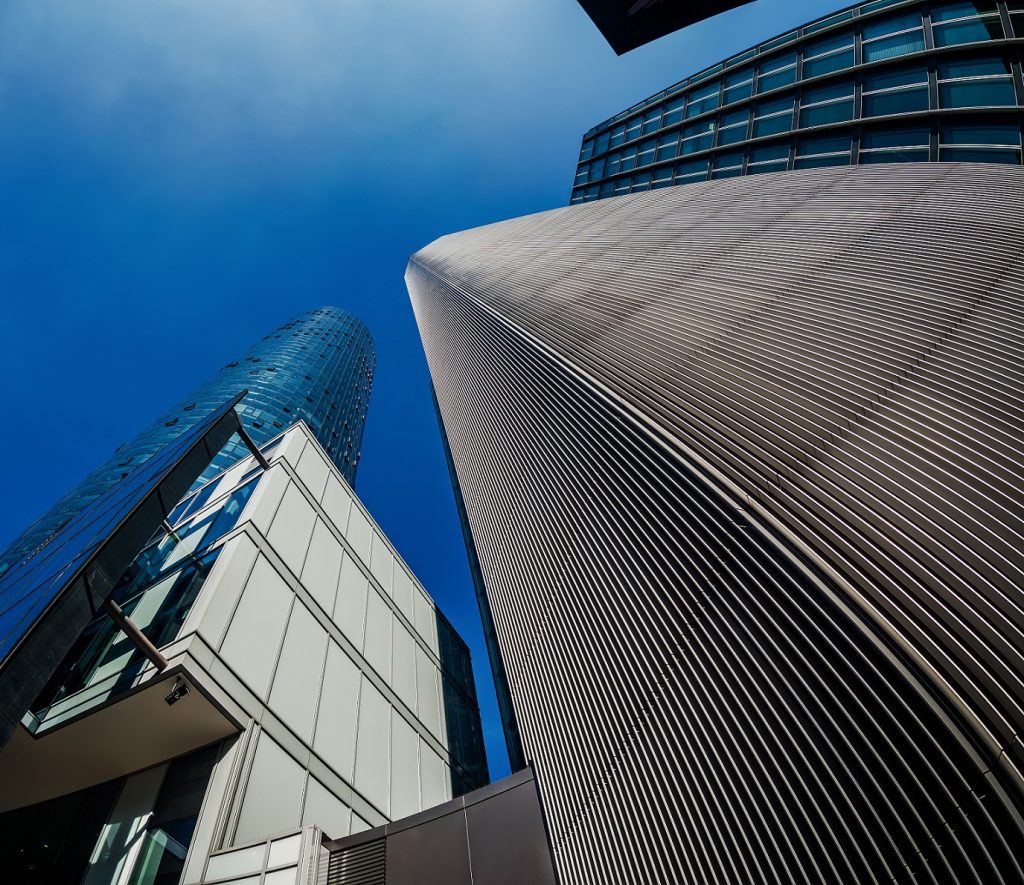
Is the German market the new Eldorado for Real Estate in Europe?
The figures speak for themselves: real estate investments in Germany rose to almost €66 bn in 2016, reaching almost €20 bn in the final quarter excluding residential, according to BNP Paribas Real Estate. Germany is now challenging the UK as the main European market, and some see it as the safest investment area following Brexit. […]
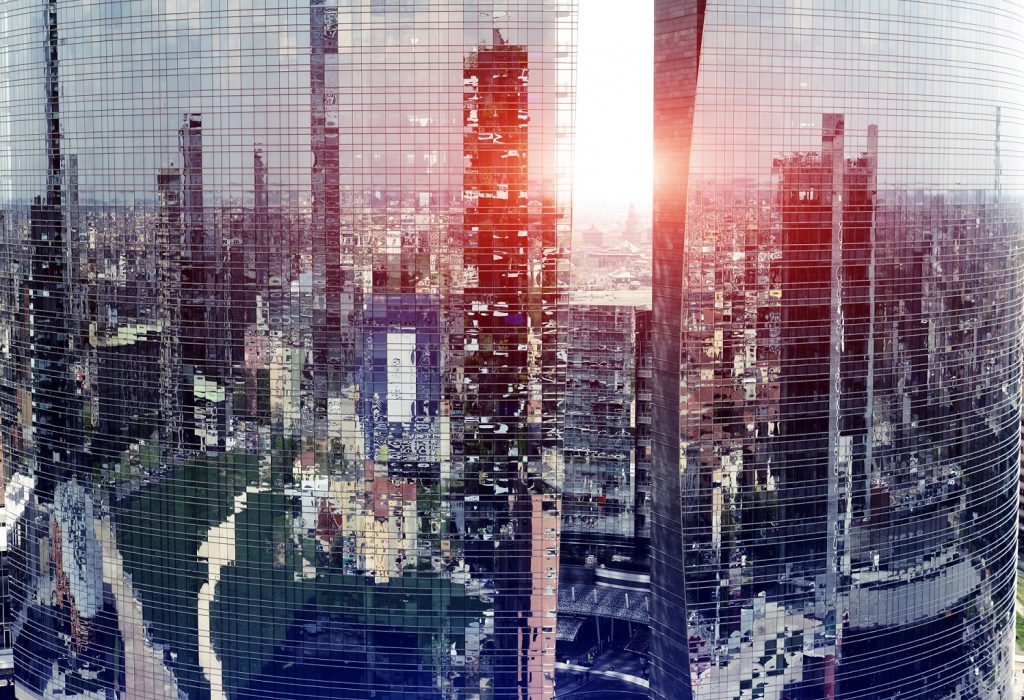
A closer look at the factors underlying the decision to sell NPLs in Italy
There is a clear pipeline of jumbo disposal Non-Performing Loans (NPLs) in Italy, thanks to the ECB push, however vendors will need to take into careful consideration a few factors in their decision, such as recent regulatory reforms of insolvency and foreclosure and a possible EU bad bank. 1. ECB Push Europe’s NPL assets are […]
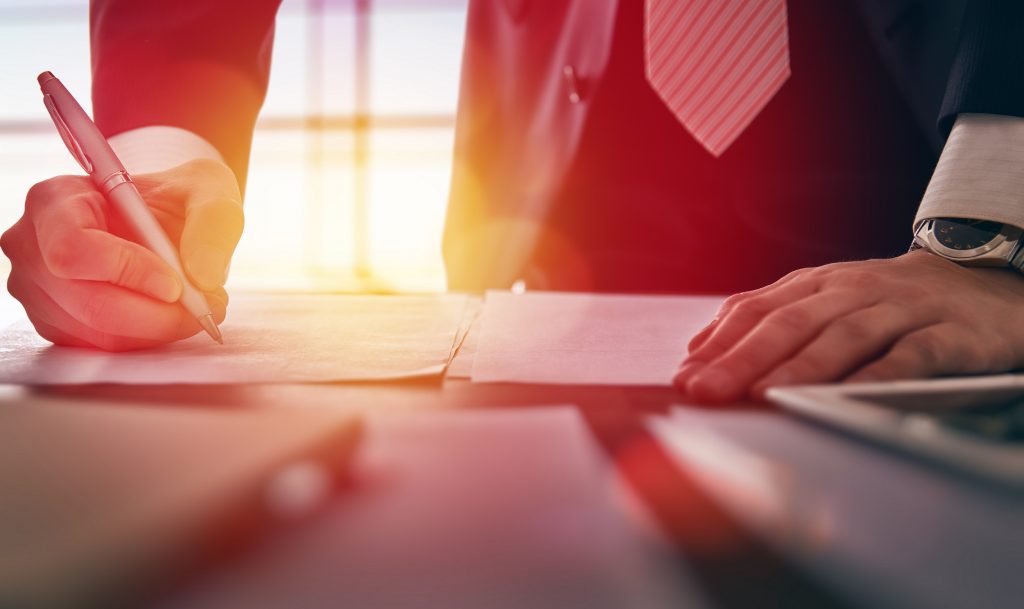
EBA opinion on IFRS 9 and the impact on regulatory capital
After the Basel Committee consultation in October and then the European Commission’s legislative proposal of the package CRR II / CRD V in November 2016, the EBA has now given its opinion on the management impact of IFRS 9 on regulatory capital, notwithstanding a second impact study has just been completed. This opinion, primarily addressed […]
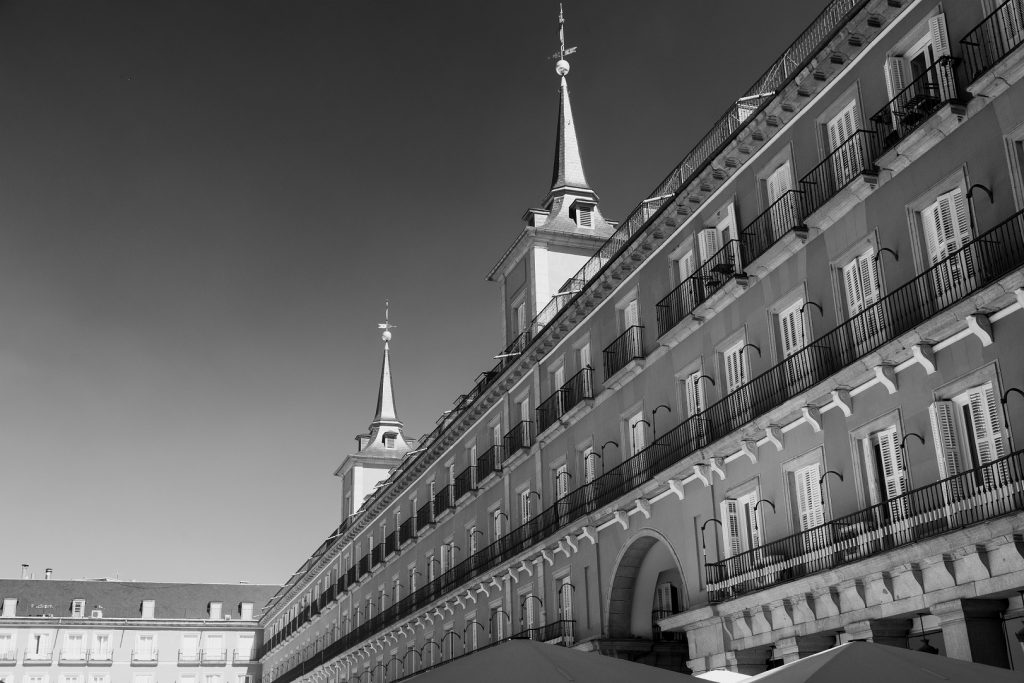
Tax Registration Issues for German Real Estate Funds in Spain
German Immobilien-Sondervermögen are domestic open-ended real estate funds without legal personality which are managed by an investment management company on behalf of its investors. It is the investment management company that buys, sells and manages property held within the fund according to the stated investment policy. The investment management company is the civil owner acting […]
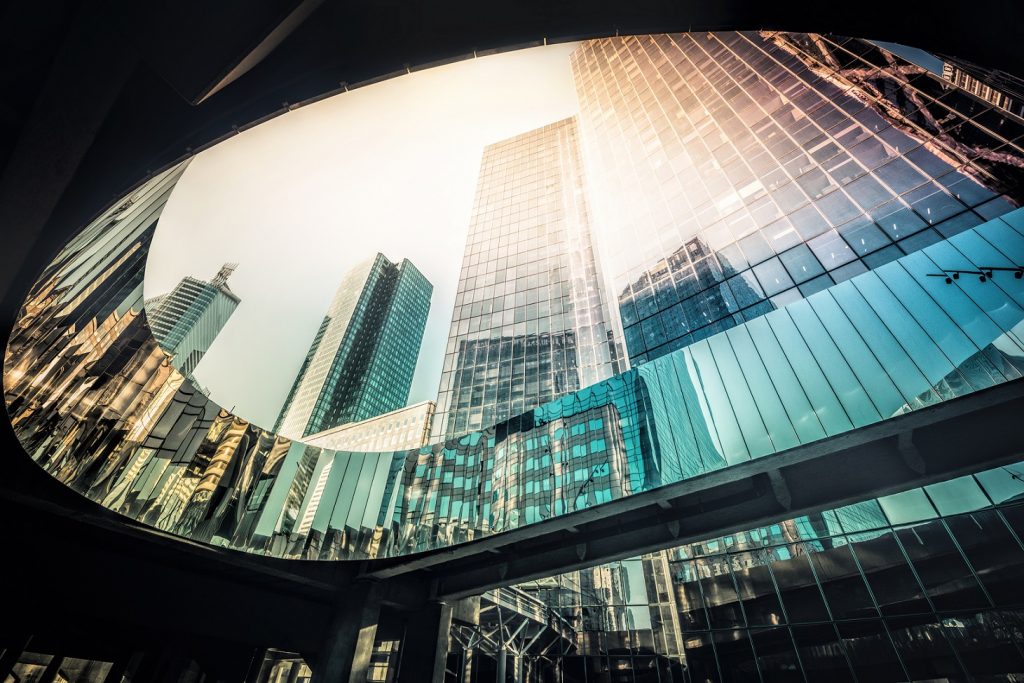
Infrastructure Investments: the Impact on Solvency II Balance Sheets for Insurers
Background and issues The Cambridge dictionary defines ‘infrastructure’ as ‘the basic systems and services that a country or organisation uses to work effectively’. This rather broad definition covers a wide range of assets at the heart of economic activity: they do not just provide a service to an individual or enterprise, but to economic agents […]
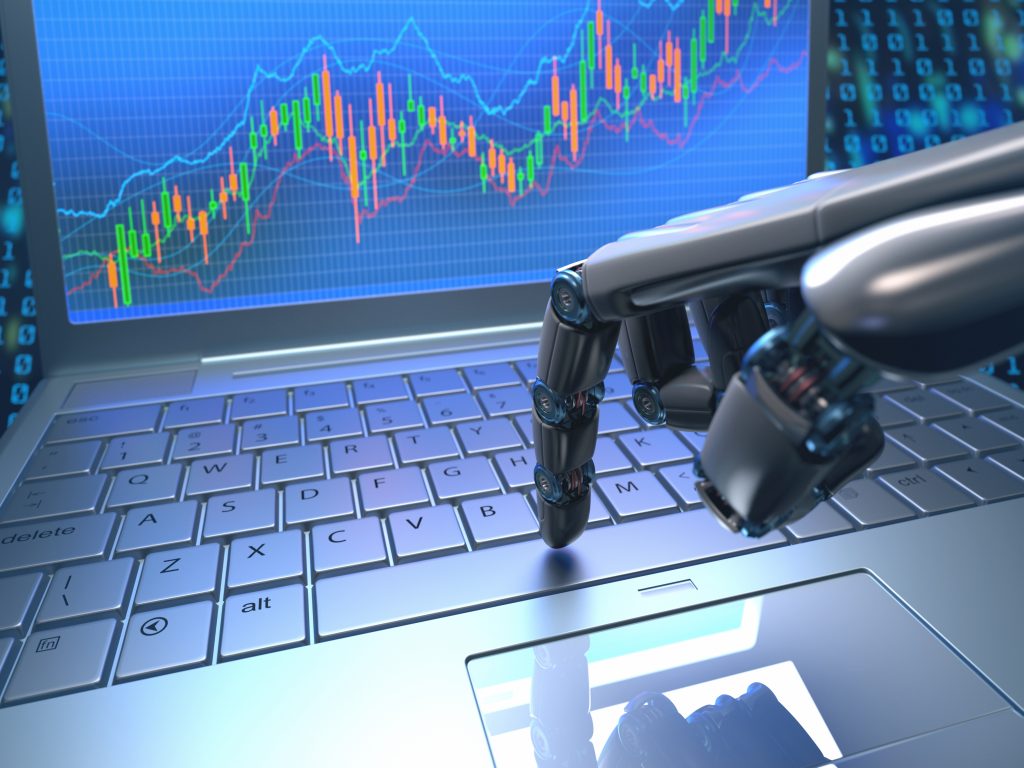
Do Androids Dream of Stock Prices?
We look at the rise of ‘robostocks’ and algorithmic trading, and consider the repercussions on financial markets. An old investment adage mockingly states that “a failed trade becomes a long term investment”. The idea behind it is that if a security is bought and underperforms, investors tend to keep it until it eventually becomes profitable, […]
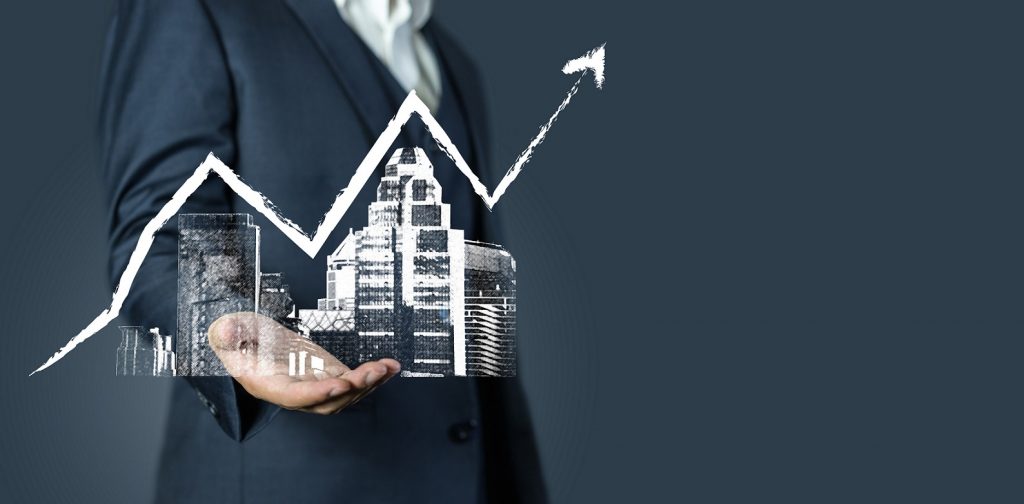
The Application of Tax Treaties on REITs
Real estate structures and tax treaty issues: questioning current thinking Real Estate Investment Trusts (“REITs”) give all investors the opportunity to invest in large-scale, diversified portfolios of income-producing real estate in the same way they typically invest in other asset classes – through the purchase and sale of liquid securities. Qualifying REITs are also tax […]
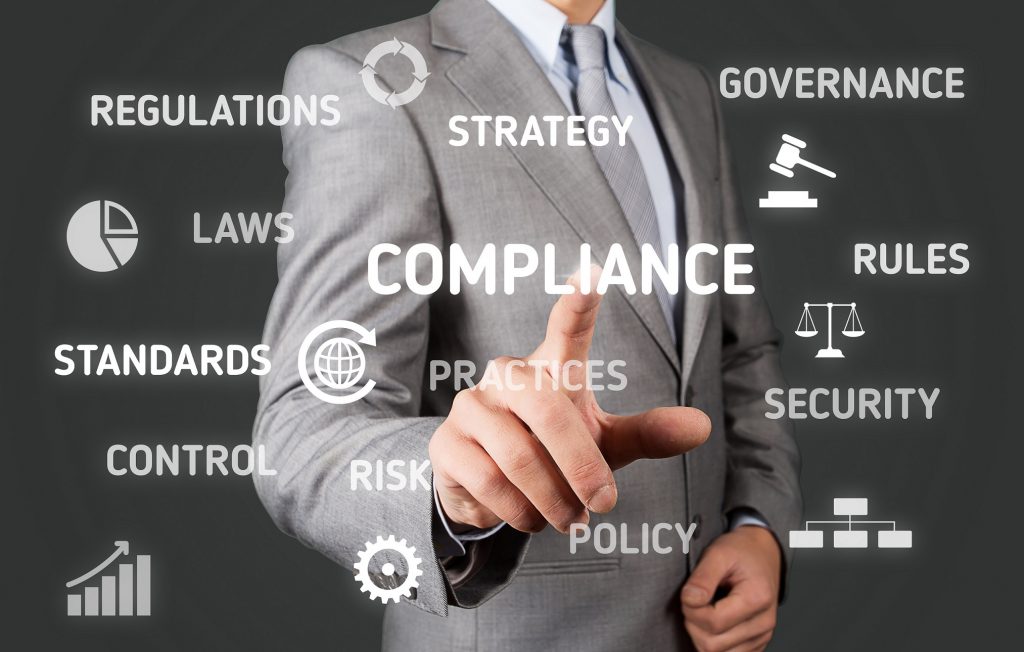
Consolidated Audit Trail Approved by the U.S. Securities and Exchange Commission
The Securities and Exchange Commission (“SEC”) approved a plan on November 15, 2016, to create the consolidated audit trail (“CAT”), a central data source to collect and accurately identify every order, cancellation, modification and trade execution for all exchange-listed equities and options across all U.S. markets. This plan is pursuant to SEC Rule 613, in […]
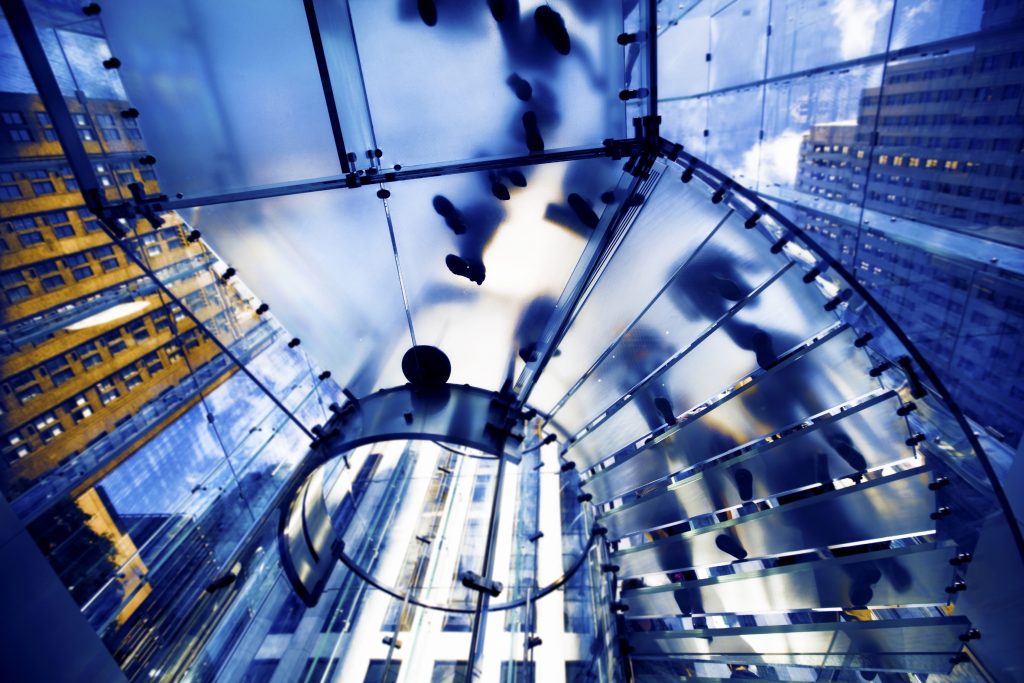
Germany reforms taxation of investment funds
The principle of tax transparency for investment funds will soon be replaced by taxation at fund level with partial exemption for investors. This will also apply to foreign funds with German income. The new rules have been integrated in the completely revised German Investment Tax Act (Investmentsteuergesetz). This will lead to a complete change in […]
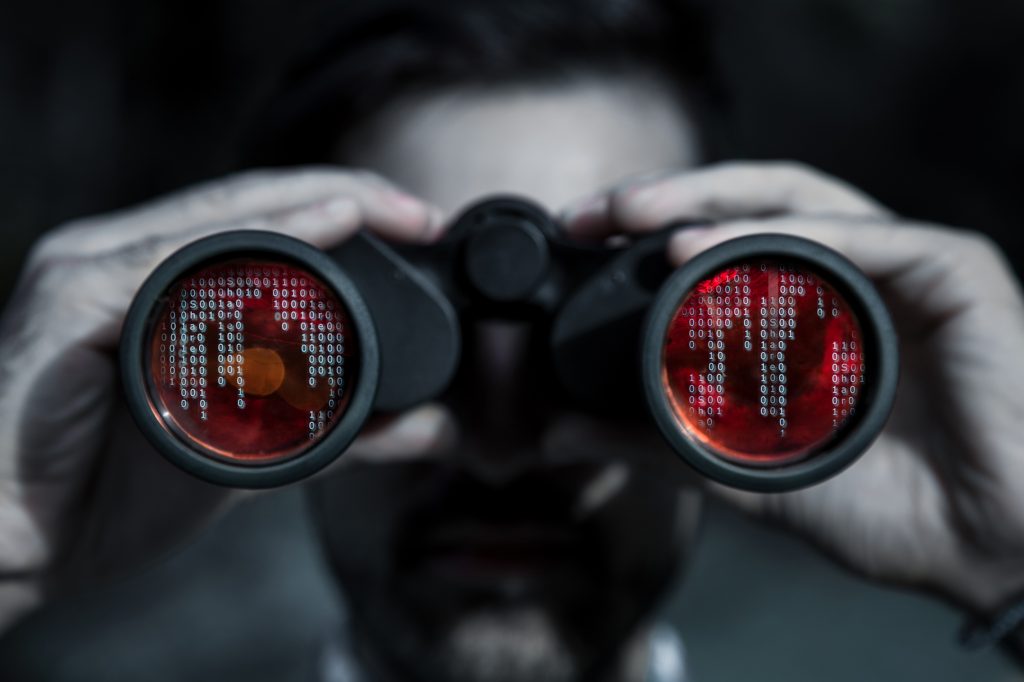
Empower your people to protect the bank
Cyberattacks aren’t just getting more frequent, they are also becoming significantly more vicious and sophisticated. The majority of today’s data breaches result from human error, making cybersecurity a “people problem” as well as a technology issue. The solution to this people problem can’t be solved by purchasing new hardware or software or implementing sophisticated network […]
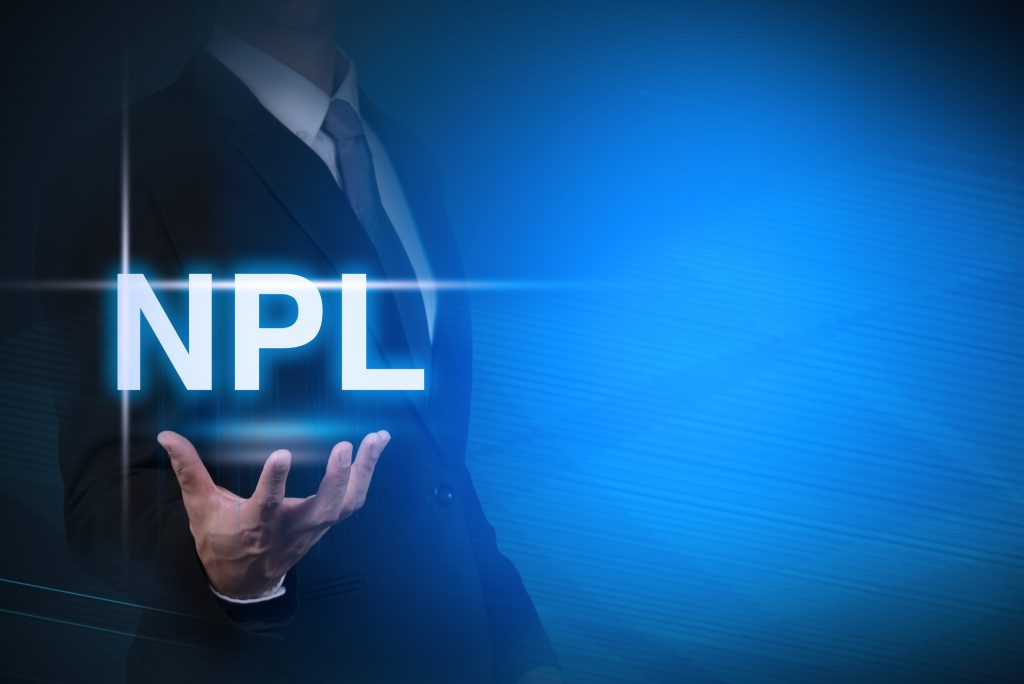
Creating a compliance pathway for dealing with NPLs
Non-Performing Loans (NPLs) are a key issue and will continue to be on the European agenda as a top priority for a long time. On the one hand, the ECB guidelines are already applicable, as they represent a best practice reference for the day-by-day work of the Joint Supervisory Teams. On the other hand, the […]
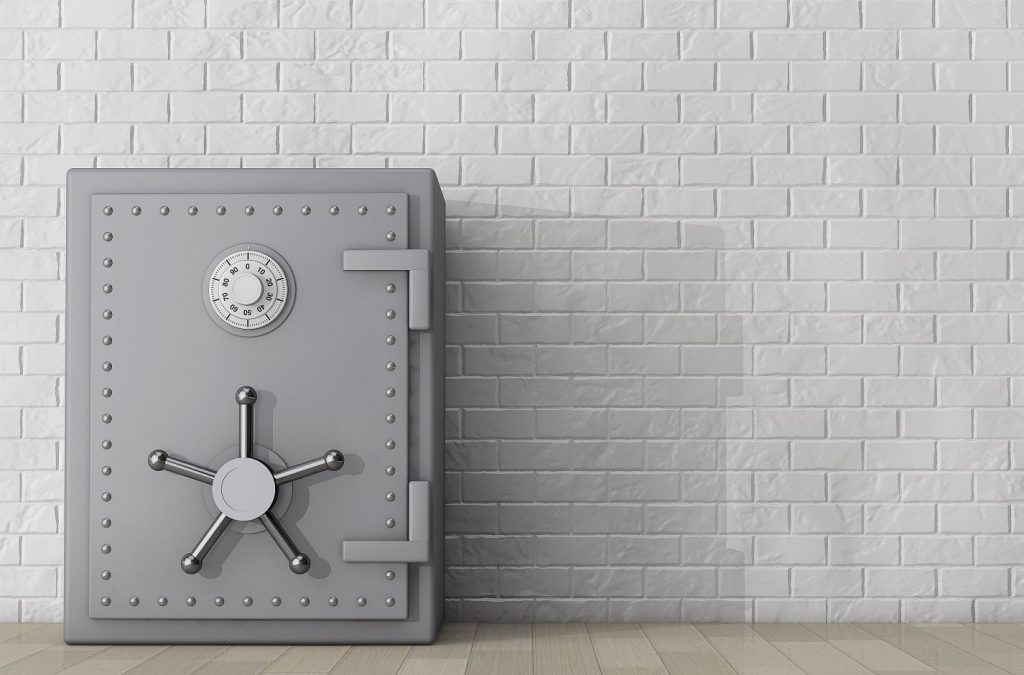
Revision of the CRR / CRD IV package
On Wednesday 23 November, the European Commission presented its long-awaited revision of the Capital Requirements Regulation (CRR) and Capital Requirements Directive (CRD IV), including the regulatory changes that mark the finalisation of the Basel III agreements. This revision follows five years of consultations conducted by the Basel Committee. It aims to foster financial stability and to enhance […]
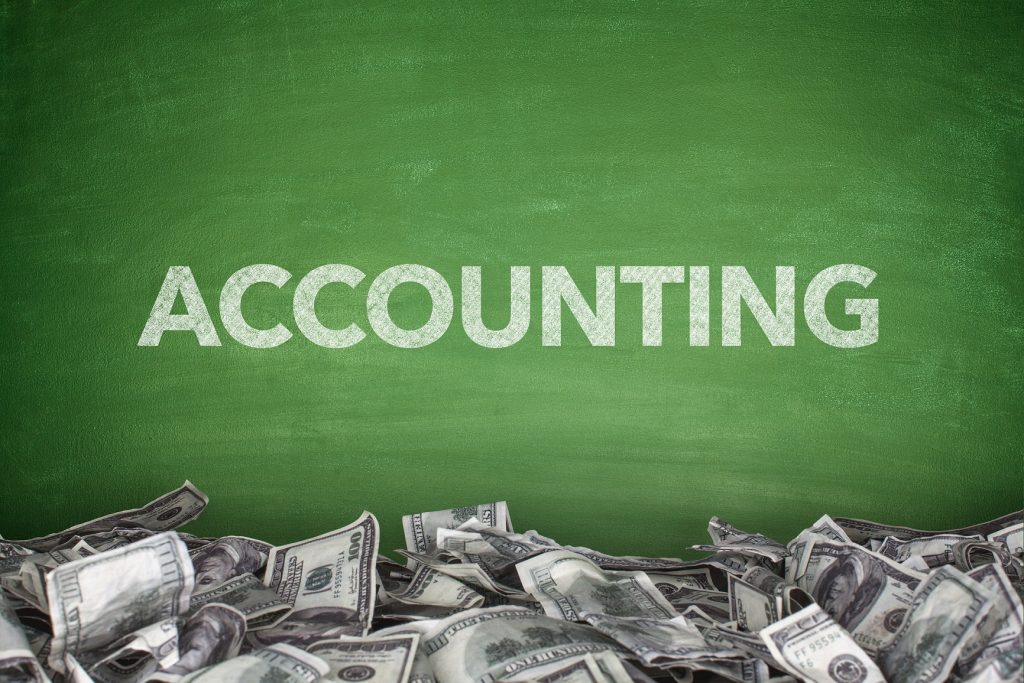
Impact of the New Revenue Recognition Standard on the Asset Management Industry
In May 2014, the Financial Accounting Standards Board (FASB) issued Accounting Standards Update (“ASU”) 2014-09, Revenue from Contracts with Customers. Topic 606, Revenue from Contracts with Customers, presents the new revenue recognition guidance. FASB also issued ASU 2015-14, Revenue from Contracts with Customers (Topic 606): Deferral of the Effective Date, in August 2015. As a […]
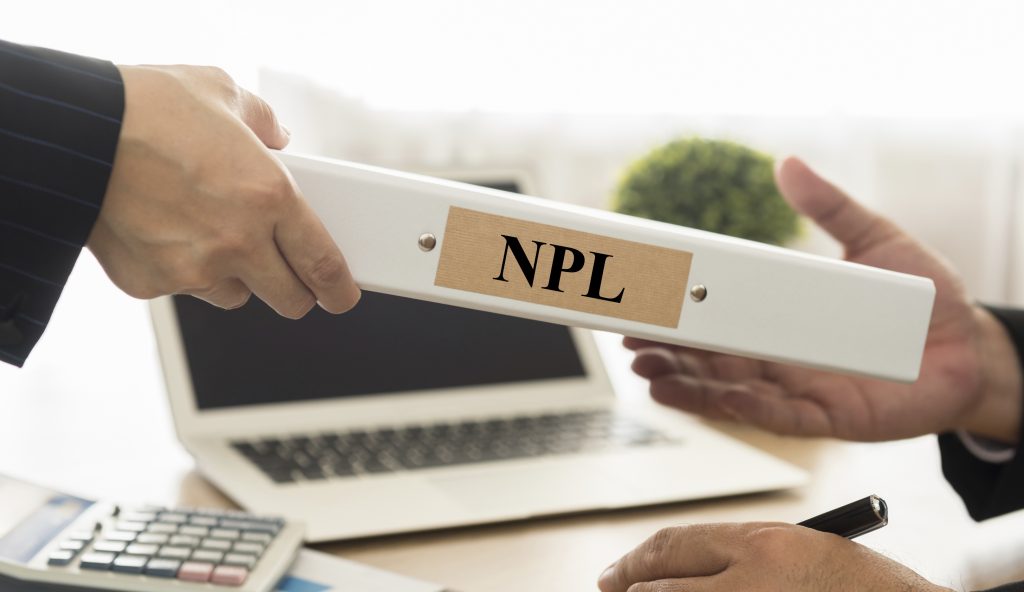
What’s at stake with the ECB draft guidance to banks on non-performing loans?
The European Central Bank (“ECB”) recently launched public consultation on guidance to banks on non-performing loans (“NPL”). Consultation periods runs until 15 of November 2016. As NPL harms the profitability, funding and capital of banks and more globally the real economy, the ECB issued this guidance, aiming to achieve common practices for how to handle […]
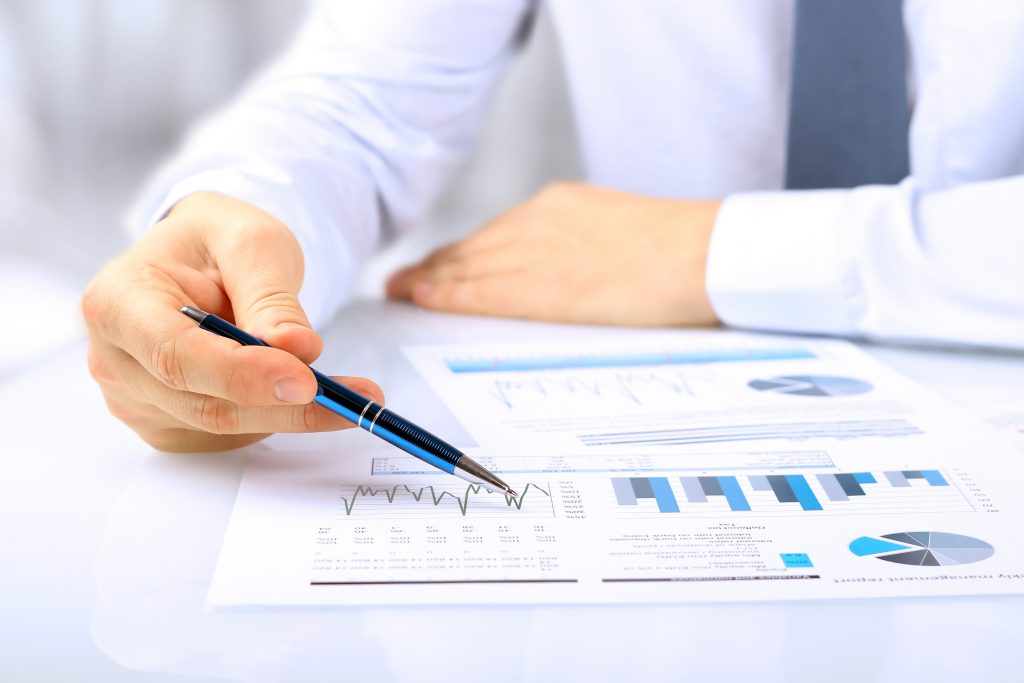
The management of regulatory capital following the transition to IFRS 9
On 1 January 2018, banks applying IFRSs move into a new era with the implementation of IFRS 9 on financial instruments, which will supersede the existing IAS 39. Although adopting this new standard will lead to many changes, it was phase 2 of the standard, on provisions, that interested the Basel Committee on 11 October, […]
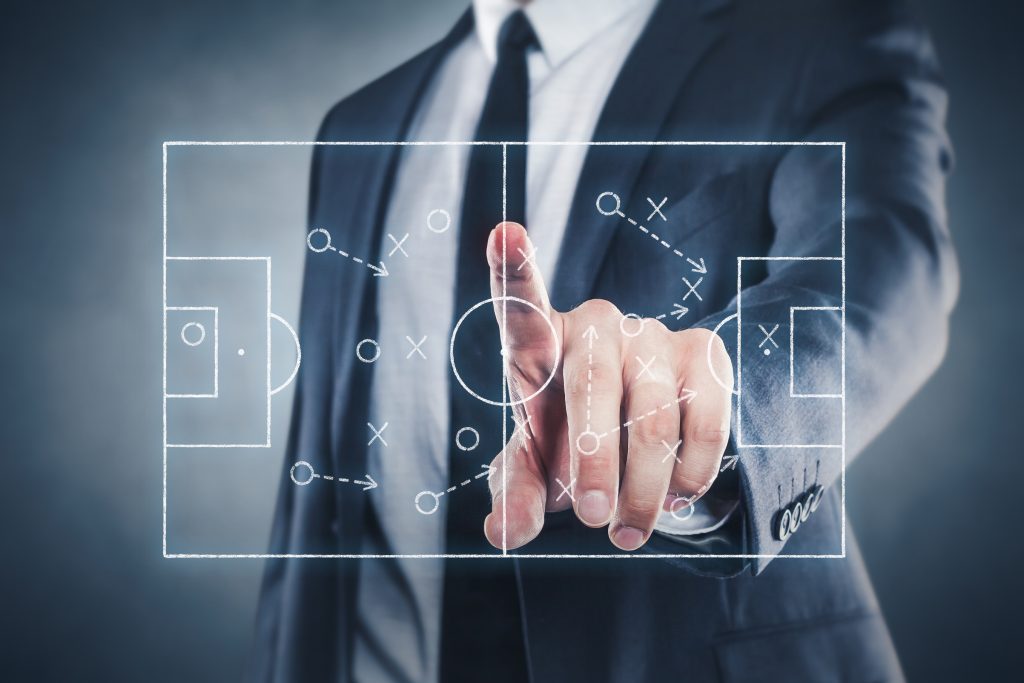
Brexit – playing for a draw?
On 20th October, I was delighted to chair an event in collaboration with OMFIF on the implications of Brexit for the financial services industry. We had an excellent panel of experts representing banks and asset managers as well as the City of London in general. The event was badged as a 100 days post referendum […]
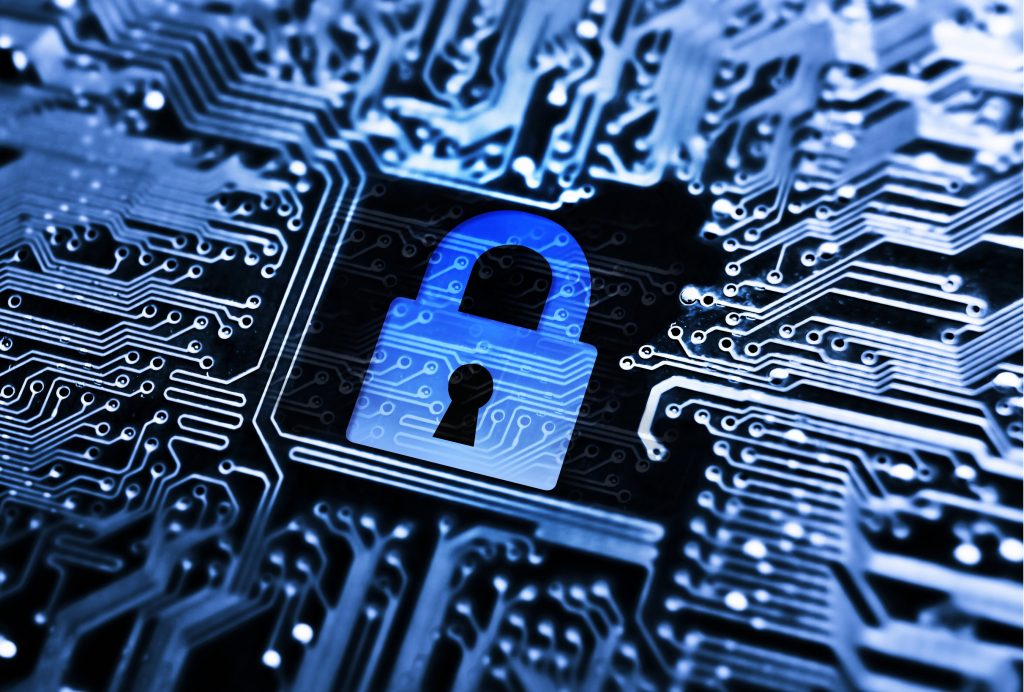
New York Proposes Cybersecurity Regulations for Financial Services Companies
New York Department of Financial Services (DFS) has significantly raised the bar for cybersecurity programs, releasing regulations on September 13, 2016 slated to go into effect on January 1, 2017. The regulation will affect all entities with a DFS “license, registration, charter, certificate, permit, accreditation or similar authorization under the banking law, the insurance law […]
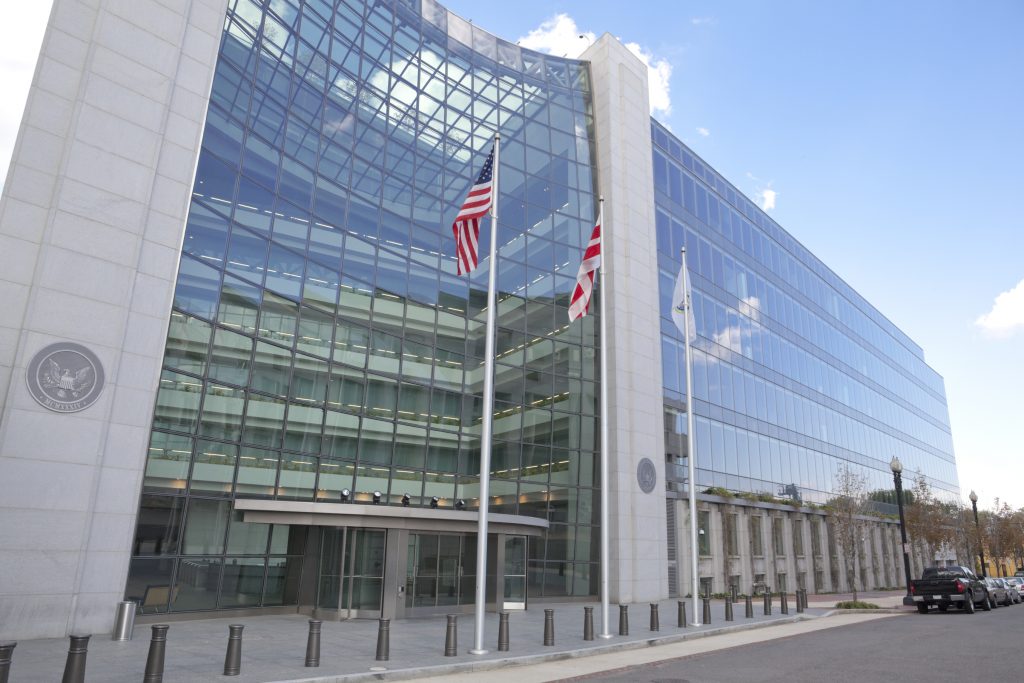
SEC Adopts Amendments to Investment Adviser Act Rules
On August 25, 2016, the Securities and Exchange Commission (the “SEC” or the “Commission”) adopted amendments to various rules under the Investment Advisers Act of 1940 (the “Act”). The amendments will be effective 60 days after the date of publication in the Federal Register, but investment advisers are expected to comply with the amendments after […]
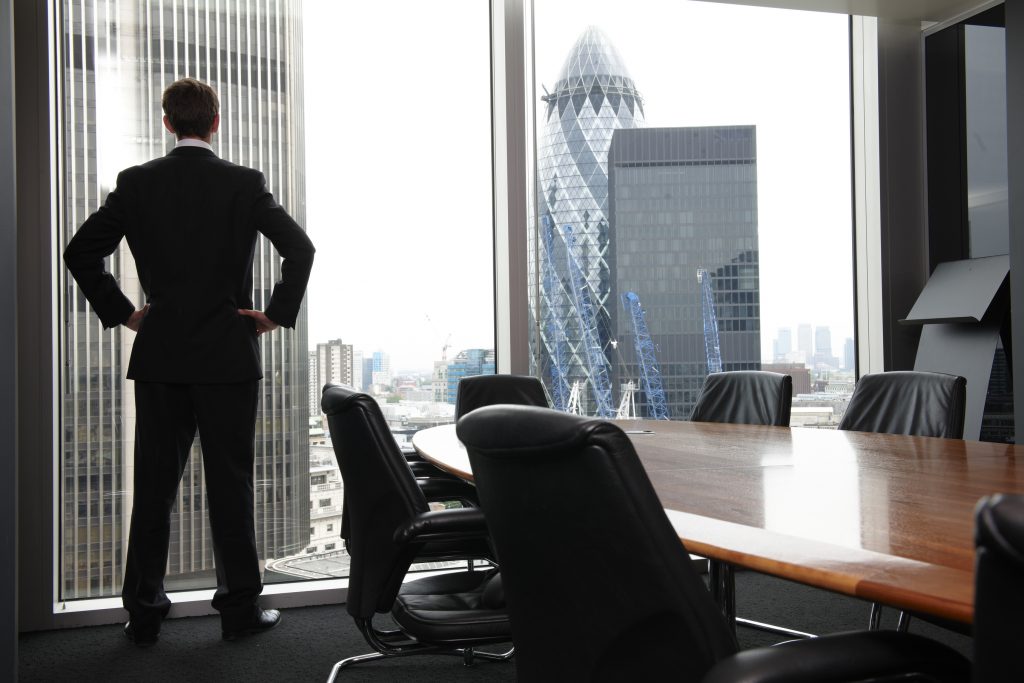
100 Days Post Brexit Referendum – How much smarter are we?
Saturday 1 October marked the 100th day since the result of the UK referendum to leave the European Union became known. And it seems neither the British nor their European neighbours have fully come to terms with the idea of a European Union without the UK. Yes, all continental Europeans love British eccentricity: driving “on […]
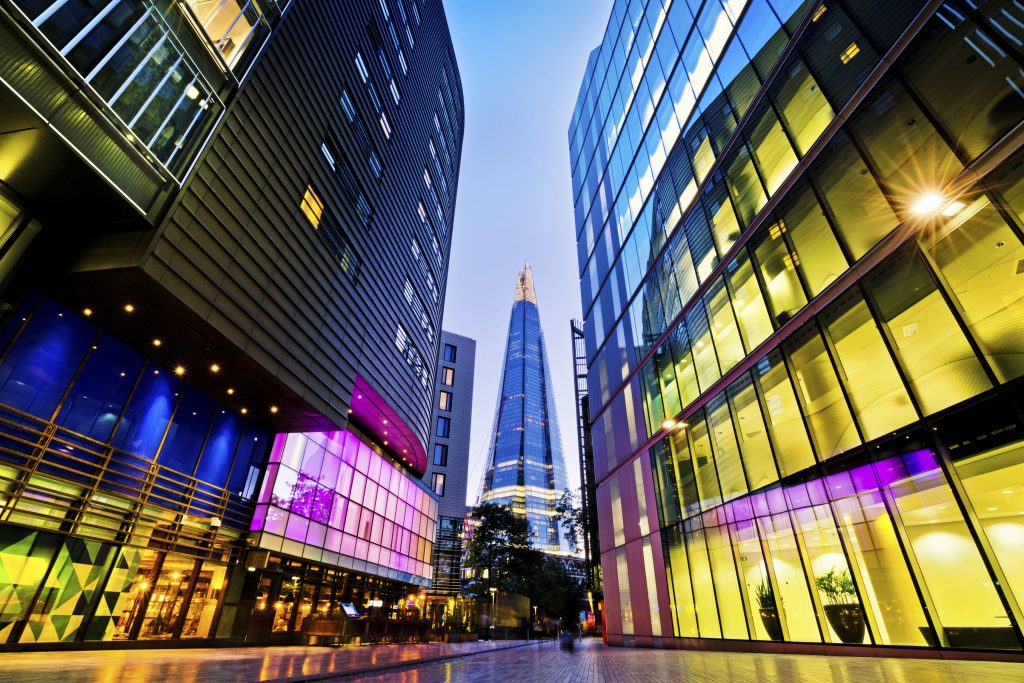
Brexit : Insights for the Real Estate Sector in the UK
Following the UK’s landmark referendum decision to leave the European Union, real estate investors have been dealing with the push and pull of post-Brexit market sentiment. Initial panic surrounding the outcome of the vote saw a raft of UK-based fund managers suspend redemptions from property funds worth £18bn[1] as investors looked to exit the asset […]
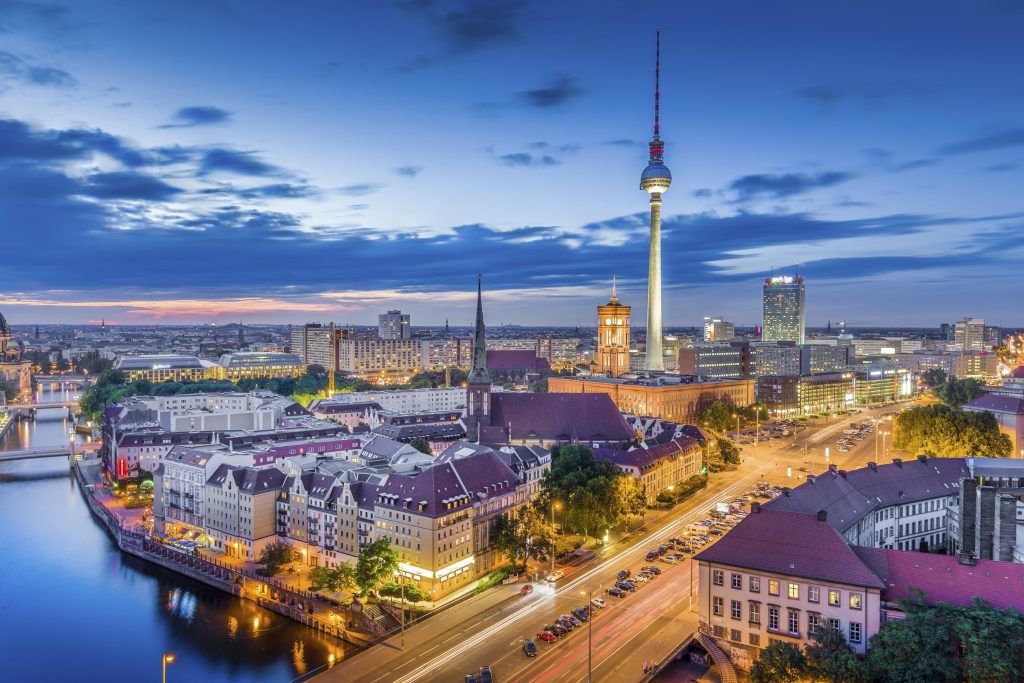
Brexit : Insights for the Real Estate Sector in Germany
In the second of our blogs looking at how the UK’s decision to exit the EU is impacting key real estate locations, our experts shine a light on some of the challenges facing German real estate investors, as well as the potential for greater breadth and depth of real estate opportunities going forward. Berlin: Strong […]
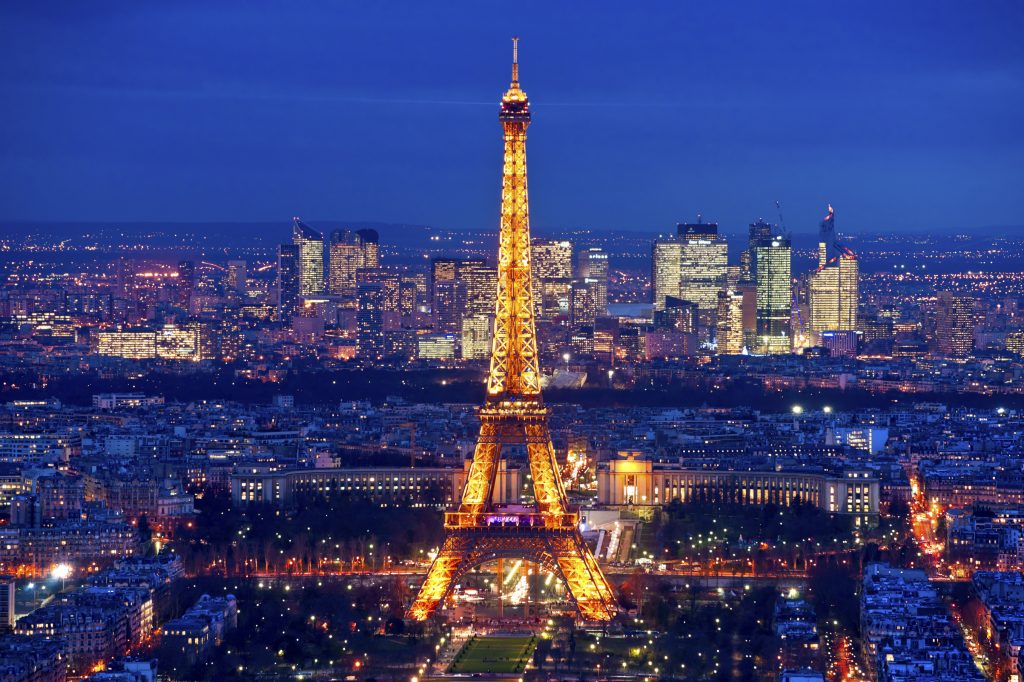
Brexit : Insights for the Real Estate Sector in France
In the third of our blogs looking at how the UK’s decision to exit the EU is impacting key real estate locations, our experts outline some important post-Brexit political and practical considerations for French real estate investors. Need for visibility as political issues take centre stage It’s looking increasingly unlikely that real estate investors will […]
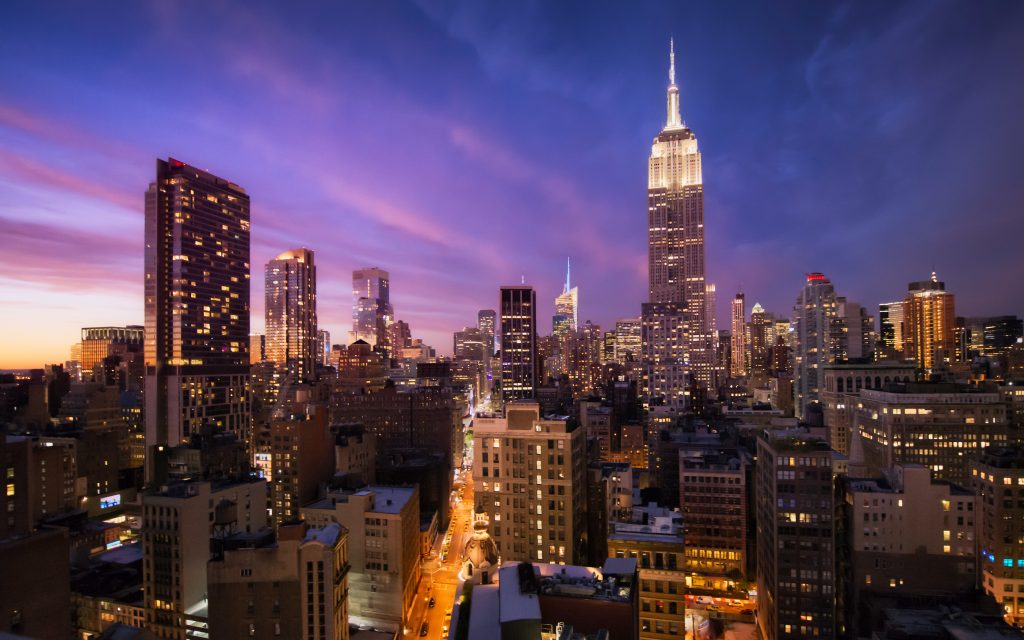
Brexit : Insights for the Real Estate Sector in the USA
In the fourth of our blogs looking at how the UK’s decision to exit the EU is impacting key real estate locations, our experts in the US outline how the current lack of clarity opens the door of opportunity for investors who currently have capital to deploy. Uncertainty also brings opportunity for real estate investors […]
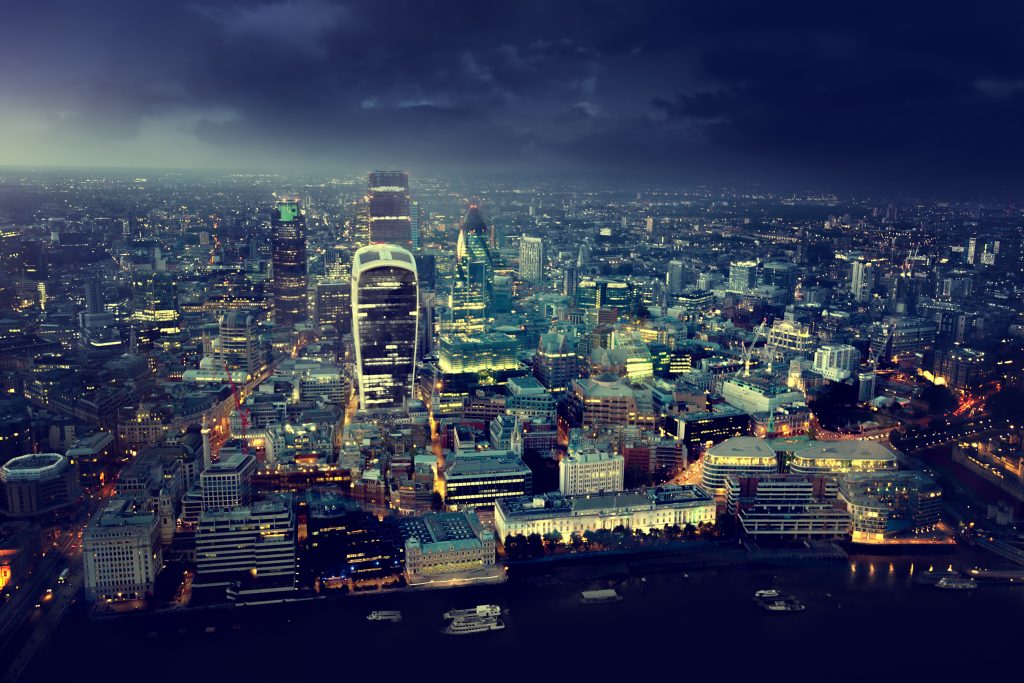
Brexit : Five Key Insights for the global Real Estate sector
Following the UK’s landmark referendum decision to leave the European Union, real estate investors have been dealing with the push and pull of post-Brexit market sentiment. Initial panic surrounding the outcome of the vote saw a raft of UK-based fund managers suspend redemptions from property funds worth £18bn[1] as investors looked to exit the asset […]
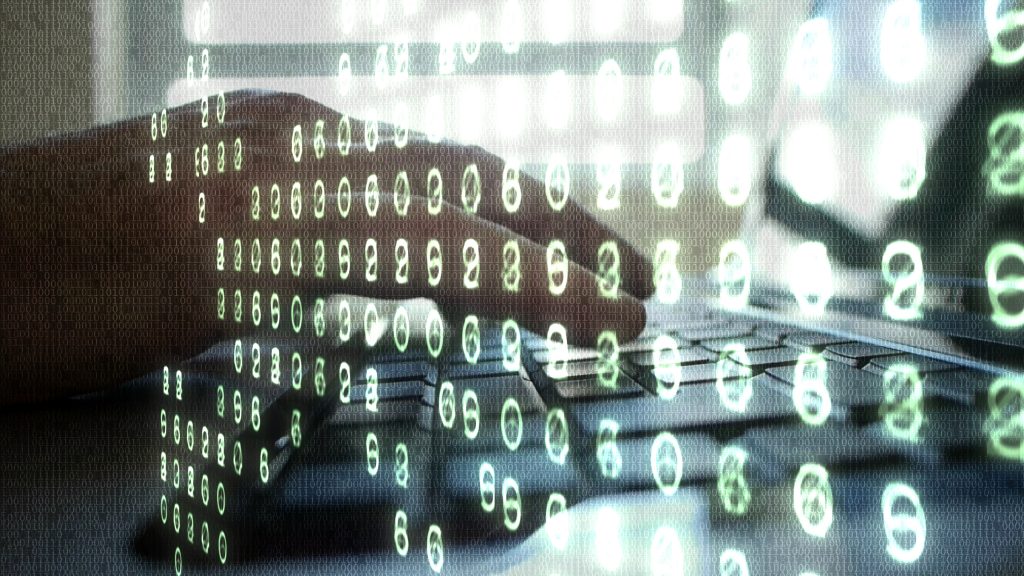
Digital finance: governance & blockchain
“Blockchain is a bit like gluten. Everyone is talking about it, but no one knows what it is,” said Tim Swanson, head of research at R3, the financial technology innovation firm that is leading a consortium partnership working on blockchain to develop industry-wide common practices and standards, architecture models and specific transaction systems for use […]
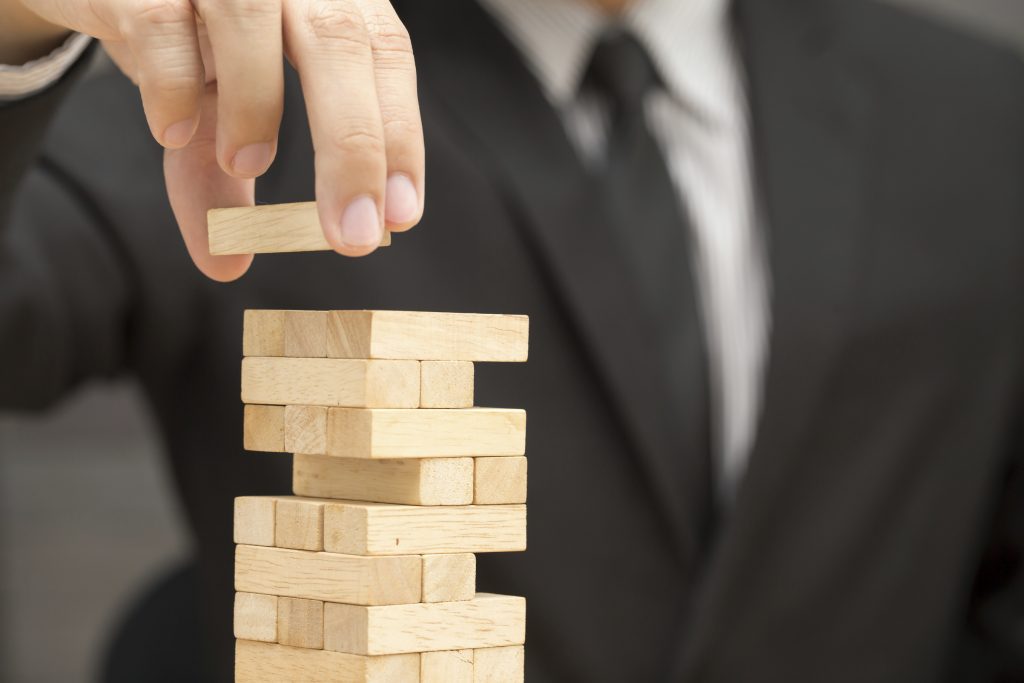
Sustainable banks must manage their risks
At a time when the European Banking Authority’s stress tests have provided valuable insights into the solvency levels of European banks, these banks are continuing their efforts to formalise the conceptual and operational framework of risk management. While changes in capital requirements (the Basel Pillar 1 quantitative requirements) still command the attention of bankers and […]
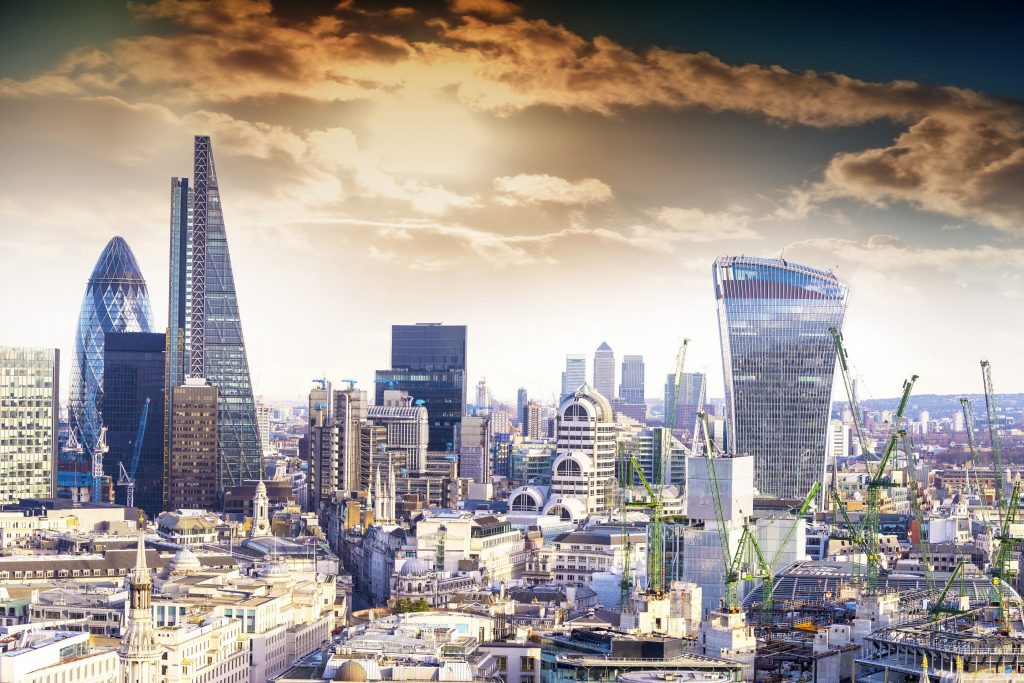
FCA data reveals 5,500 UK Companies make use of passporting rules
Brexit continues to be omnipresent. From British Airways’ Business Life (September 2016 p. 11-12, “Post Brexit, Brand Britain must show its mettle… and its sense of humour”) to the Spectator, articles are a mixed cocktail of seeing Britain great again to worries about what’s next. It really depends on the lens through which you are […]
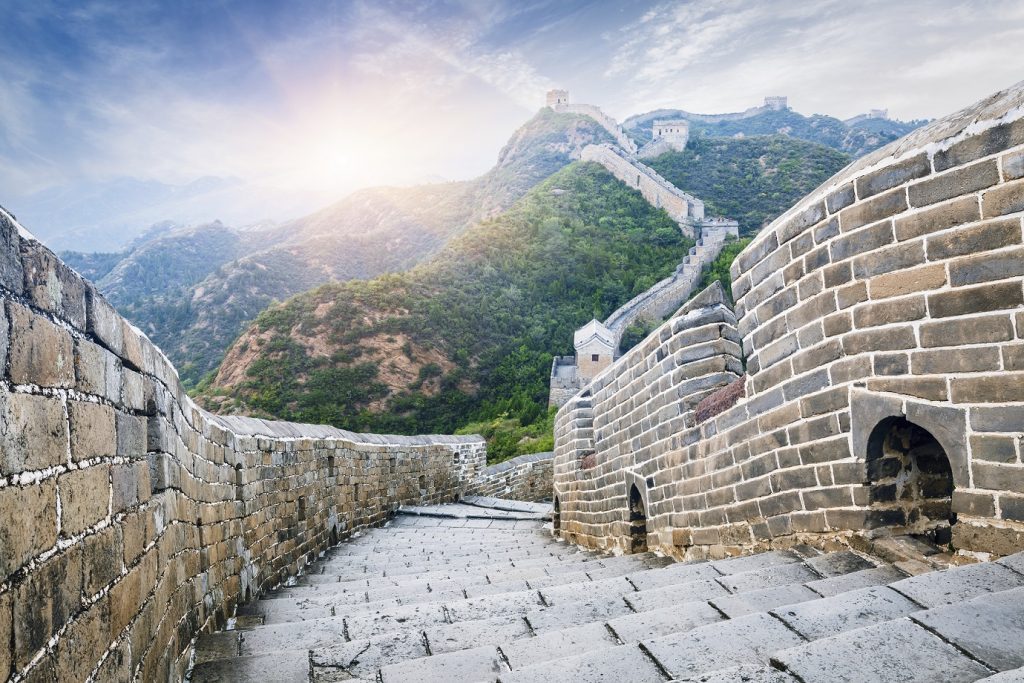
Brexit and China: a mountain to climb?
Over a beer with David Cameron in October last year, Chinese leader Xi Jinping expressed a very clear opinion on which way he wanted Britain’s vote on Brexit. He wanted a “prosperous Europe and a united EU” with the UK firmly in the EU. Britain certainly reached out to China during the last period of […]
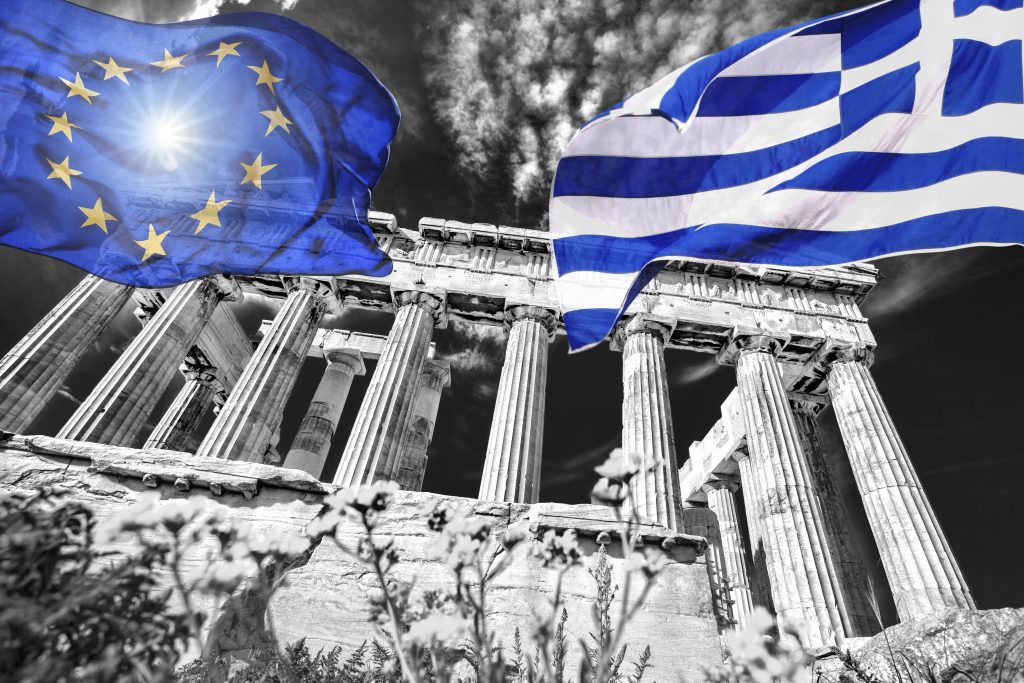
BREXIT! – Not GREXIT?
Greece’s financial systems have been tightly monitored by the institutions – once called the Troika – of ECB, IMF and EU Commission in recent years. The systemically relevant Greek banks are under close control of the Joint Supervisory Team (JST), consisting of staff from the European Central Bank and members of the Bank of Greece, […]
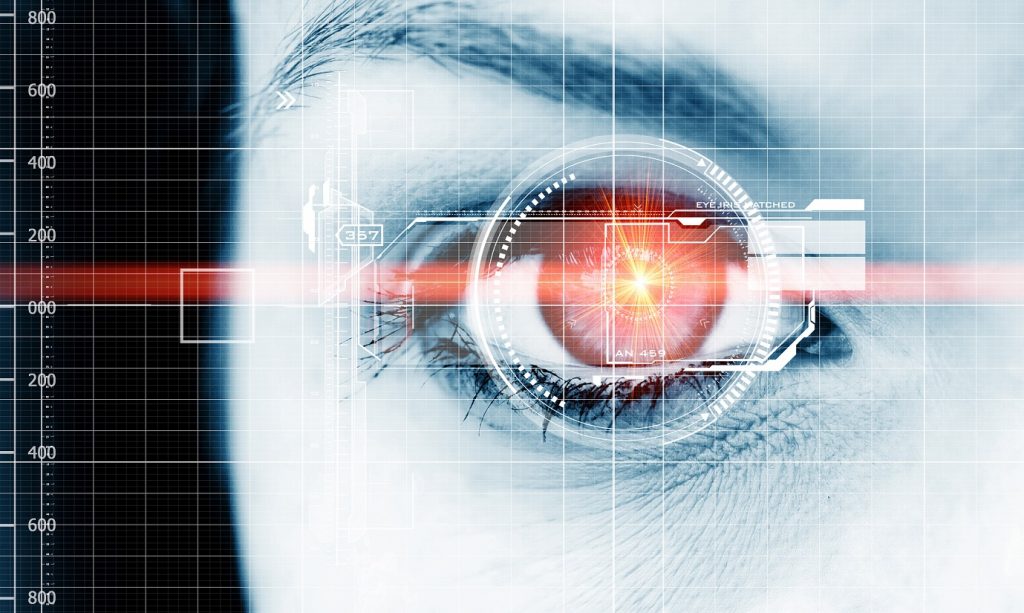
Three Key Elements for Building an Effective Human Firewall
Cyber attacks aren’t just getting more frequent, they are also becoming significantly more vicious and sophisticated. According to the latest figures from the Ponemon Institute, the average cost of a data breach has reached nearly $6.5 million in the US, alone. Yet today’s cyber attacks have far-reaching negative impacts that continue to ripple outward long […]
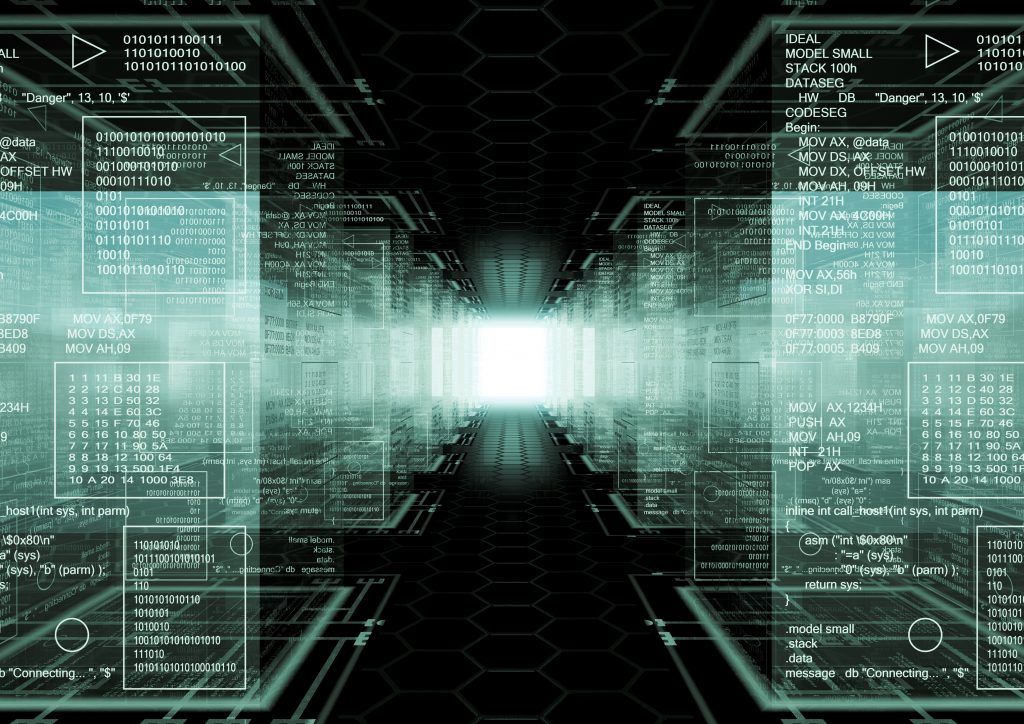
Optimizing claims: a Big opportunity for Big Data Advanced Analytics
Thanks to Advanced Analytics, insurance providers can bring claims management into a new era by combining automatic processing of large volumes of data with human expertise. Analyzing data in order to optimize the claims processing chain is not a new phenomenon for insurance providers, notably in the field of health care. The health care sector […]
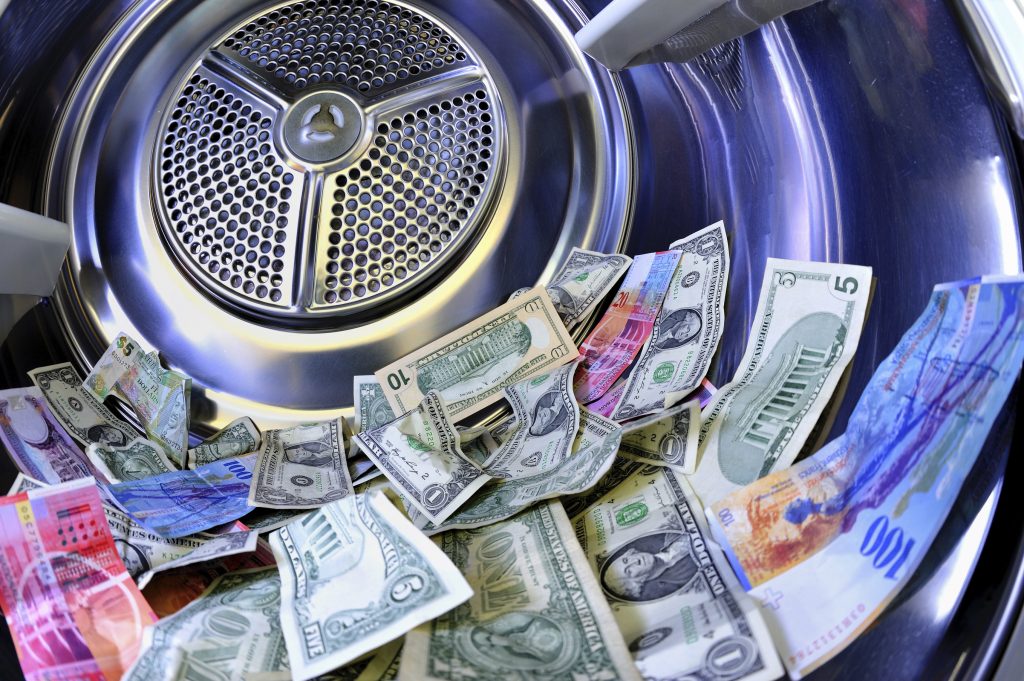
Are you prepared? New York State issues new BSA/AML/OFAC transaction monitoring and filtering program regulation
Recently released guidelines require institutions to adopt risk-based programs to monitor and filter transactions for potentially suspicious activity. Beginning January 1, 2017, financial institutions registered under the New York banking law are responsible for complying with anti-terrorism transaction monitoring and filtering program regulations, established by the New York Department of Financial Services (NYDFS). Last month, […]
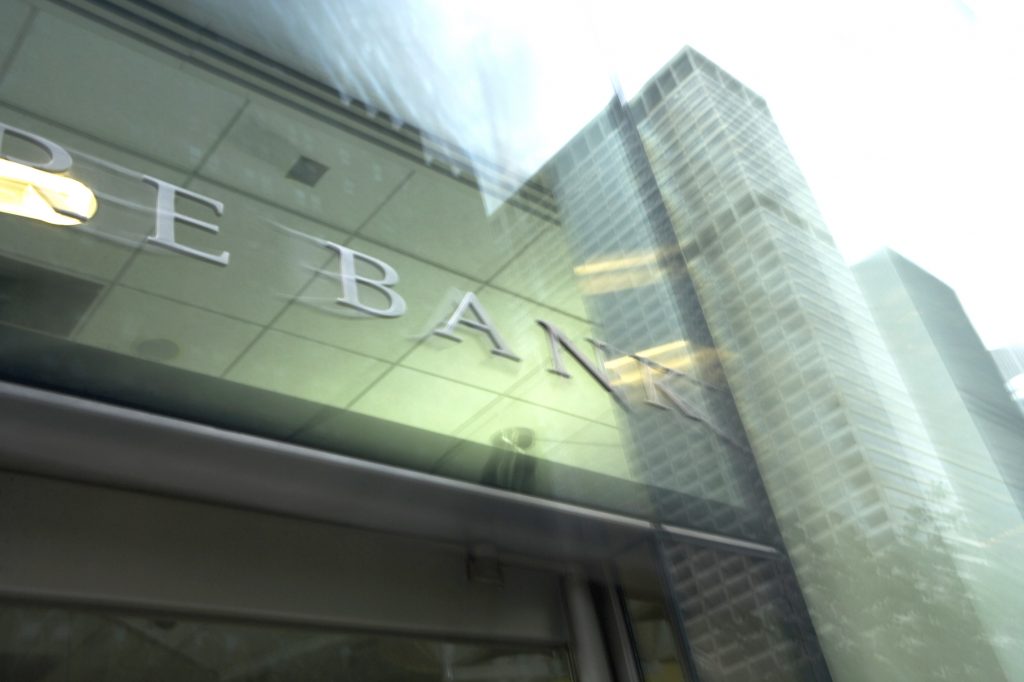
Brexit: opportunities for smaller overseas banks
The impact of Brexit on foreign banks, and especially the larger ones, has already been the subject of extensive media coverage and speculation. Discussions on the advantages and disadvantages of Brexit for larger overseas banks have been wide-ranging. Will the uncertainty over business models be solved in the short or mid-term? Would relocation bring cost […]
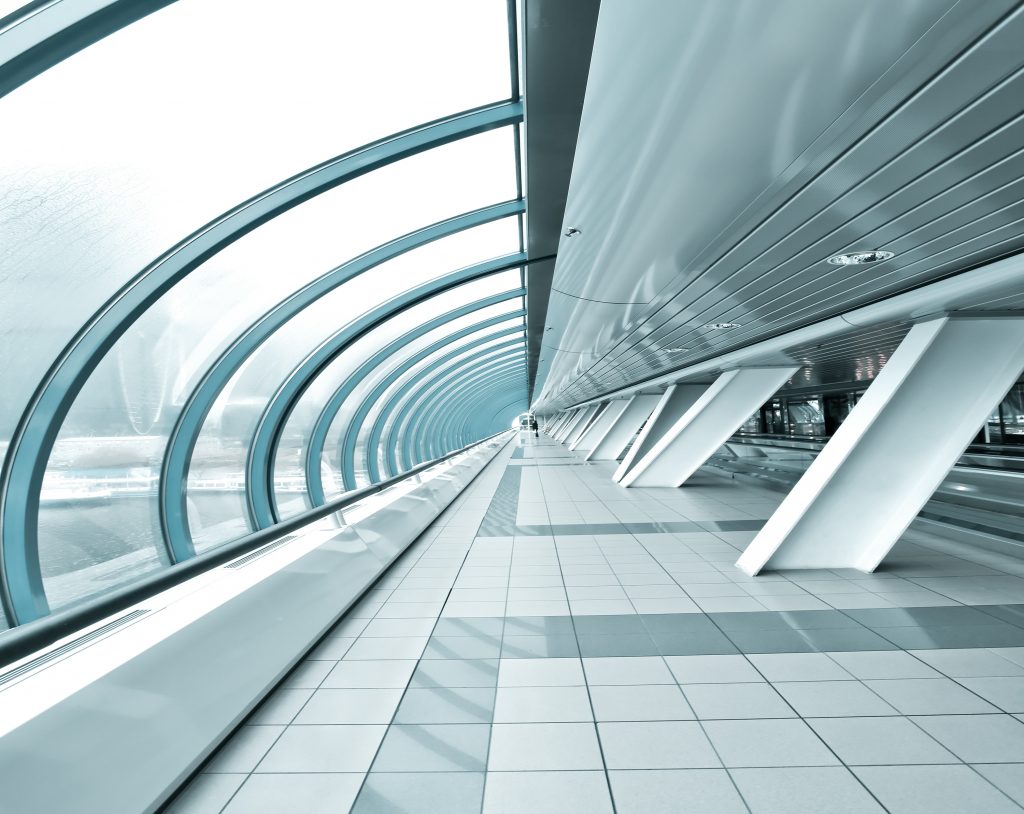
Brexit: the resilience of the infrastructure sector
In previous weeks, I have commented mostly on early signs and emerging risks in the banking sector, including some early warning shots in the property space in particular. But banks and funds invest in many other types of assets and one sector – infrastructure and energy – may even be feeling some benefits from Brexit. […]
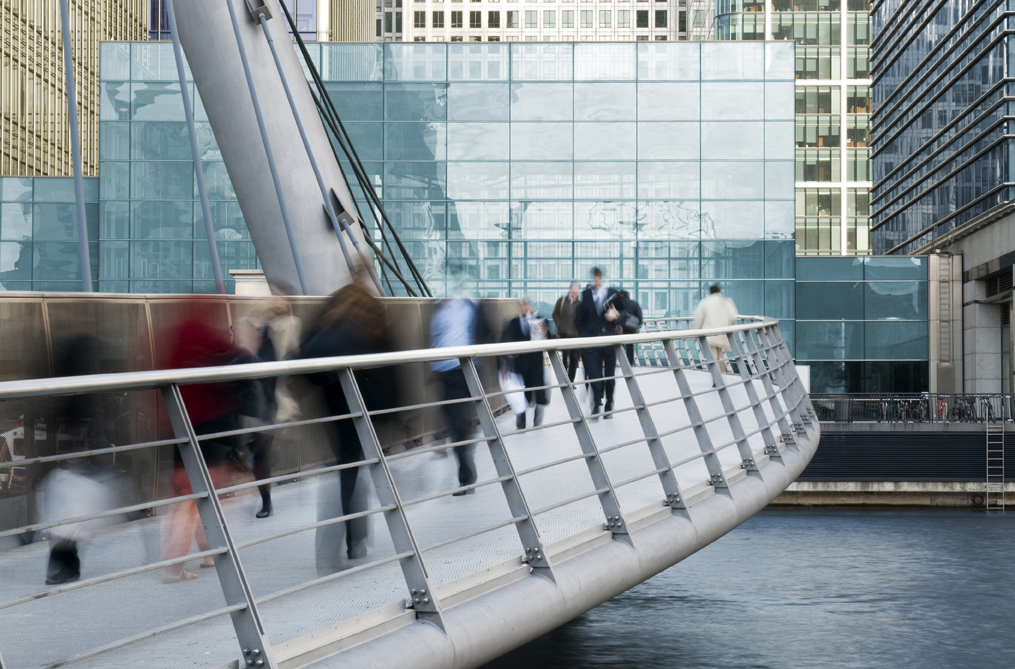
Ifs and Buts
The Brexit debate last week was overshadowed by Theresa May becoming the UK’s new Prime Minister and some of her more radical appointments to her Cabinet. On top came the horrific terror attack in Nice and the failed coup d’état in Turkey. Understandably, the core Brexit debate did not take centre stage but instead provided […]
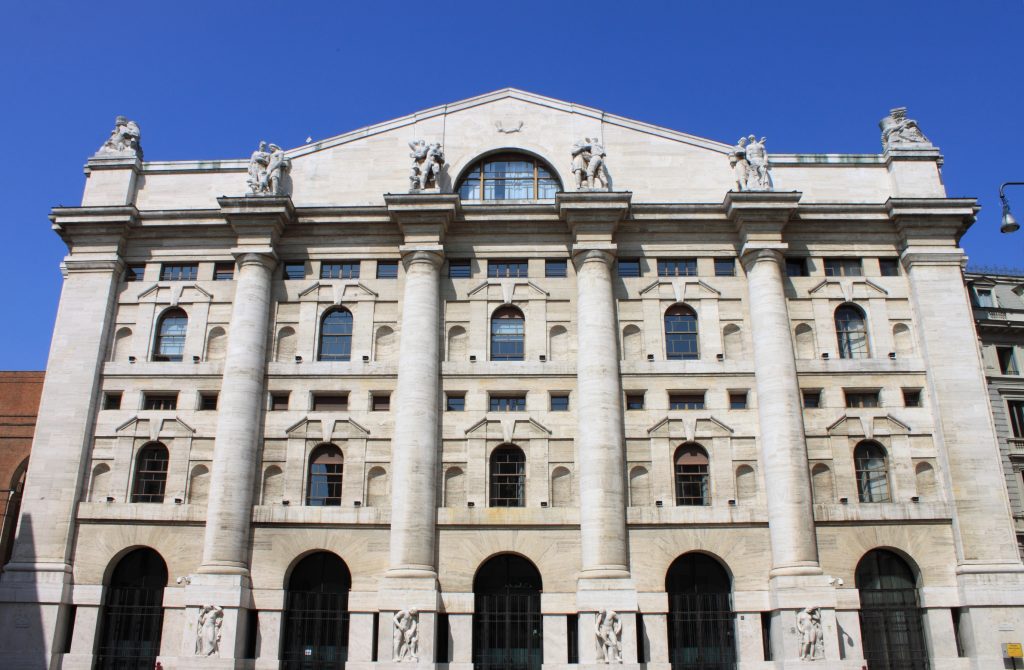
Italian banking – a Brexit-fueled calamity
Brexit continues to dominate the political and financial world across Europe and beyond as people wrestle with its impact. But there’s another massive storm on the horizon for the EU that has been brewing for some time and Brexit may well have brought forward its impact: The Italian Banking Crisis. As reported in the Wall […]
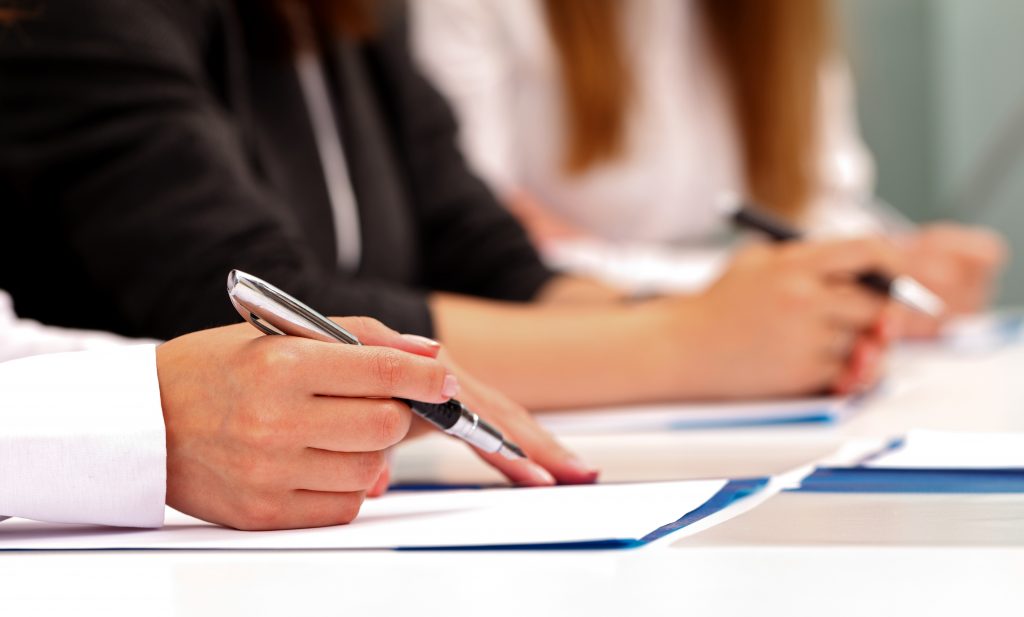
BCBS’ amendments on Simple, Transparent and Comparable (STC) securitisations
As implied in the BCBS consultative document on November 2015, the BCBS published new standards on 11 July 2016 to include amendments on STC securitisations and thus make its final decision for their calibration. The securitisation framework was highlighted in the 2008 financial crisis because of the major role of subprimes in the collapse of […]
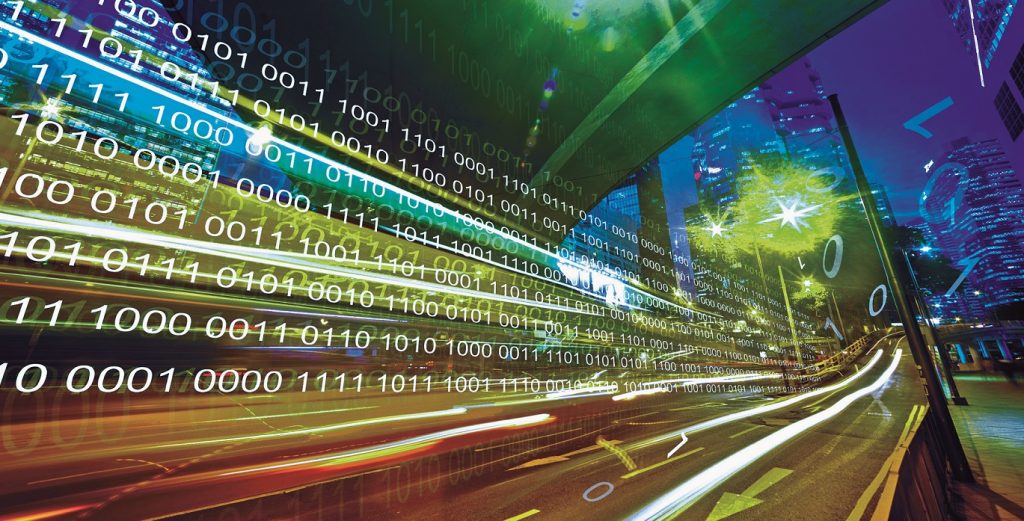
Data governance : the key to reconciling contradictory requirements
Confronted with legislation that is becoming increasingly constrictive, banks must optimize the management of their data. It’s a challenge that is compounded by the fact that data is often dispersed throughout information systems – a fact that aggregators are capitalising on to offer new multi-bank applications. Banks have a role to play in detecting abnormal […]
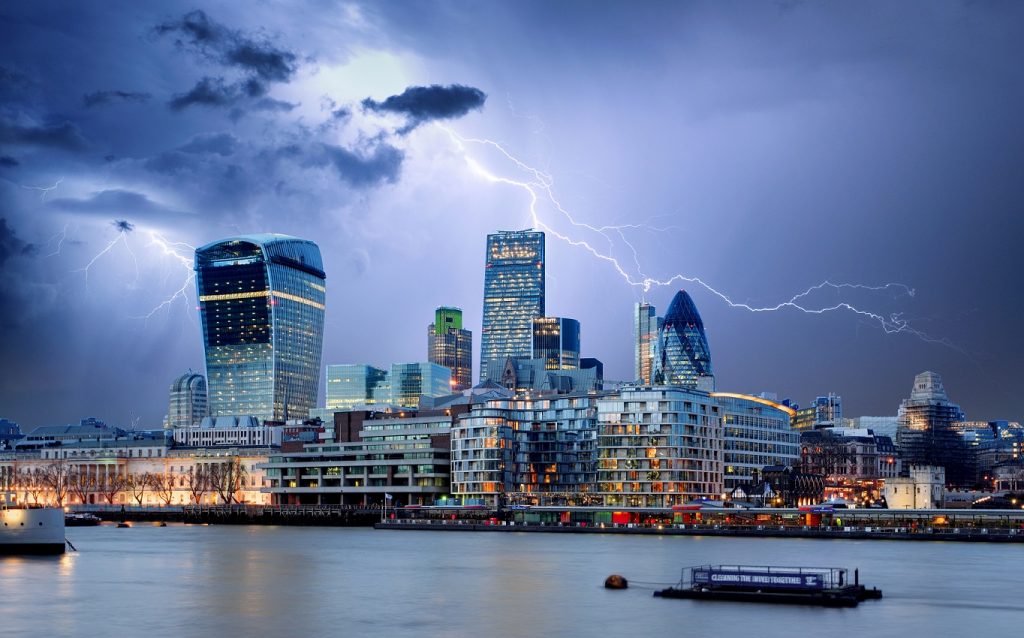
Feeling the effects of Brexit
It’s two weeks since the Brexit Referendum and it’s fair to say that its consequences are already being felt. At a broad economic level, sterling has hit a 31 year low and economists have revised down the economic outlook for the UK and the Eurozone. The UK has been downgraded by Standards & Poor to […]
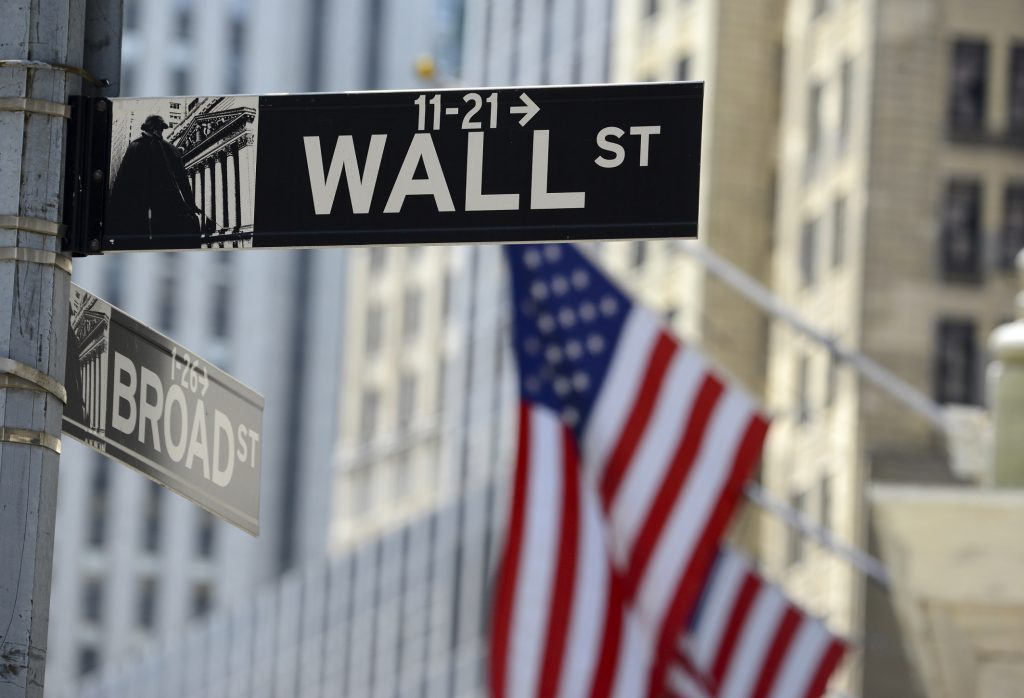
Overview of US stress tests and CCAR’s results 2016
The Federal Reserve disclosed the results of its annual supervisory stress tests and CCAR (Comprehensive Capital Analysis and Review) respectively on 23 and 29 June 2016. In a combined exercise which has been running since the financial crisis, 33 US Bank Holding Companies (BHCs) were tested, including US-based banks and the American units of big […]
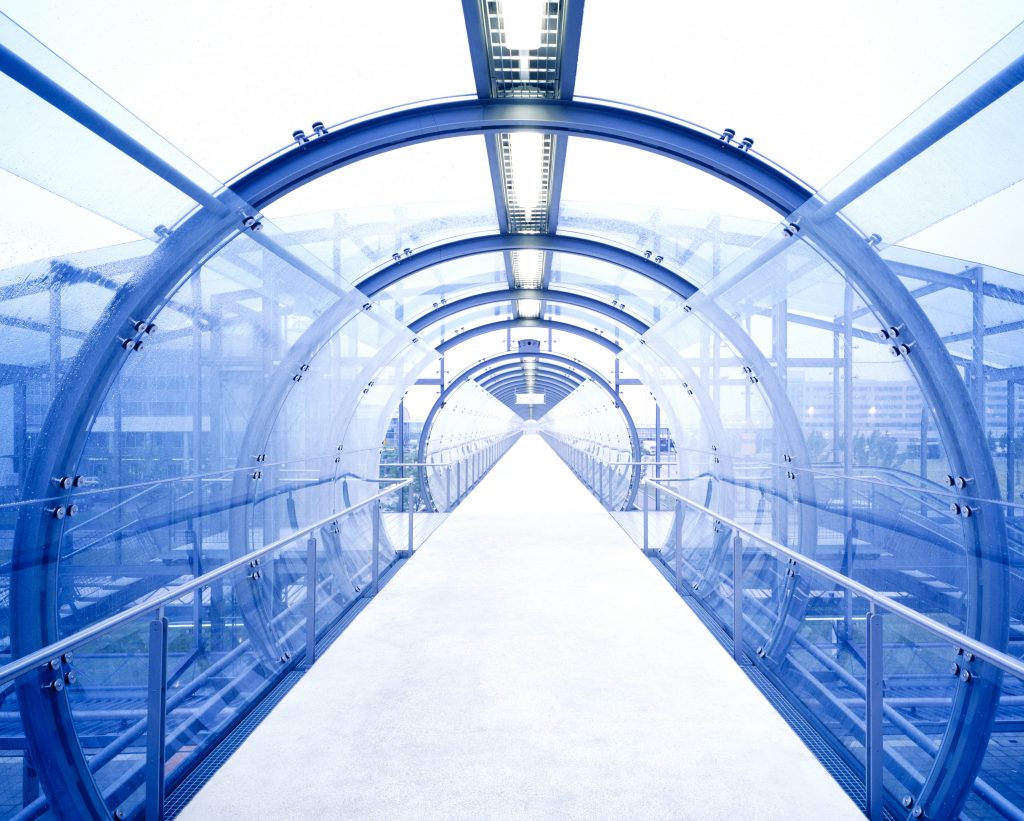
Harmonisation of internal model approaches, a new era for banks?
Basel 4 and Single Supervisory Mechanism act to reduce the excessive variability in the results of internal model approaches to credit risk. For more than ten years, Basel reform has encouraged the development and use of internal models designed to better place risk management at the heart of banks’ control arrangements. Basel II saw massive banking […]
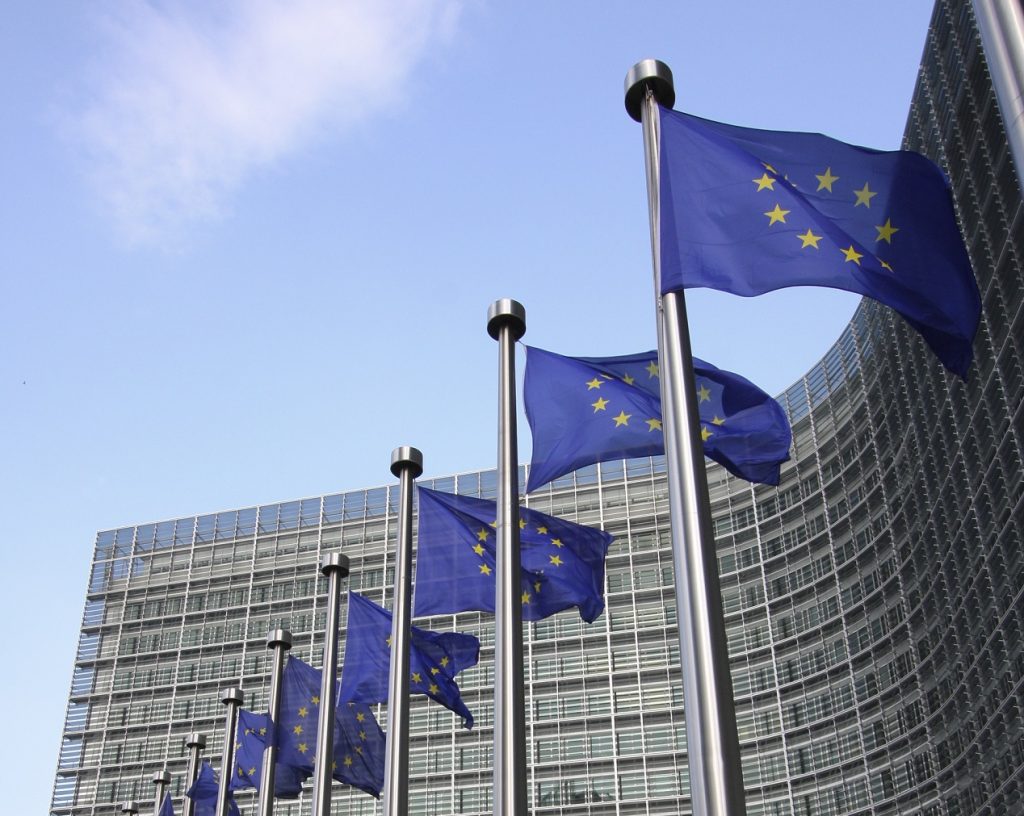
Capital Markets Union: The Impact on Banks
Business funding diversification, helping to increase options for savers and making the economy more resilient are some of the main objectives set out in the European Commission’s Action Plan on Building a Capital Markets Union (CMU) published on 30 September 2015. While a better spread of financing sources over the capital markets, insurers, households and […]
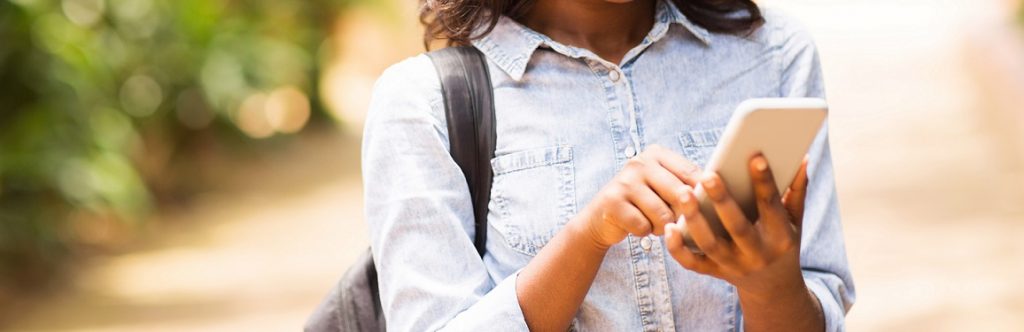
Mobile money in Africa: promise and perils
As part of the content program on digital finance that Mazars has launched in partnership with the Economist Intelligence Unit, we are exploring in a new article on how mobile money is driving Africa’s cashless future. Over the last few years, Africa has become a global leader in mobile money with the rate of smartphone […]
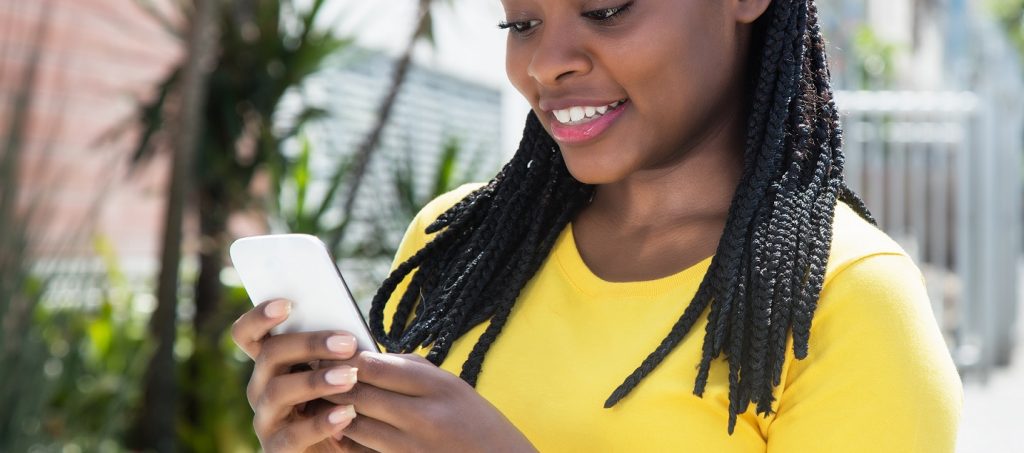
What next for Africa’s mobile money sector?
Over the last decade, Africa has become a global leader in mobile money. The statistics for the use of smartphones as a payment mechanism are compelling with M-Pesa, in particular, by far the continent’s biggest mobile money triumph. Backed by UK-based Vodafone and launched in 2007 in Kenya by mobile network operator Safaricom, by 2015 […]
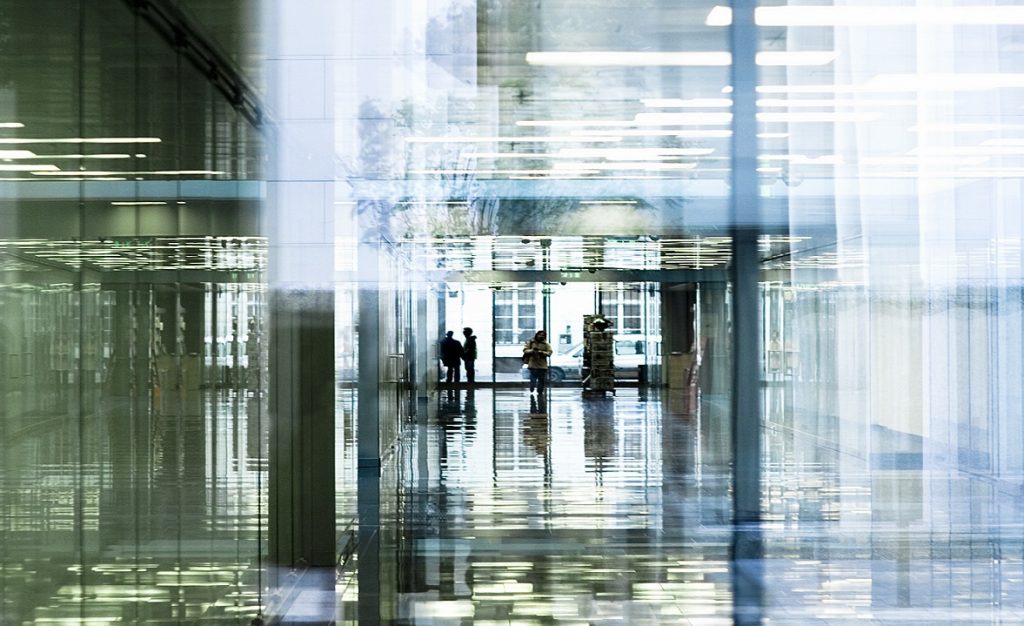
Latest consultation puts systemically important banks (G-SIBs) under the microscope
On 6 April 2016, the Basel Committee published a new consultative document outlining revision plans for the calculation of the leverage ratio and submit proposals for additional requirements applicable to systemically important banks (G-SIBs). This new consultation comes as no surprise. Since 2014 the leverage ratio, calculated by dividing Tier 1 capital by the bank’s […]
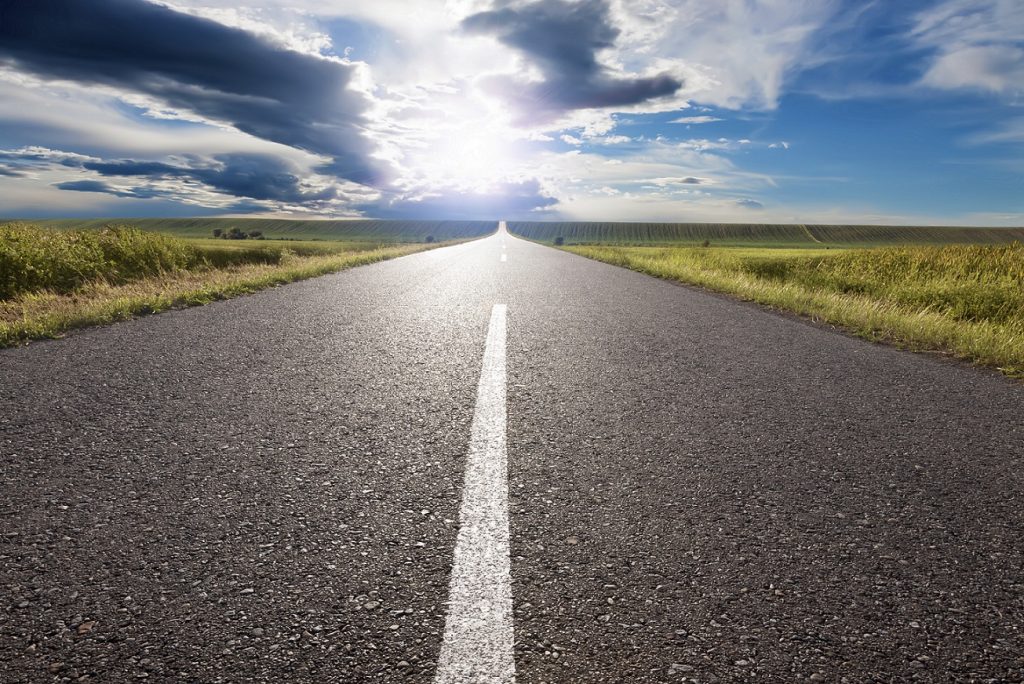
ICAAP / ILAAP: what will change in 2016?
Banks prepare for the reinforcement of prudential supervision via the Single Supervisory Mechanism (SSM). After successive waves of new regulatory requirements in recent years, the outlook for the calculation of risks and Pillar 1 capital requirements is becoming clearer. At the same time, whereas the implementation of these new requirements has and continues to mobilise […]
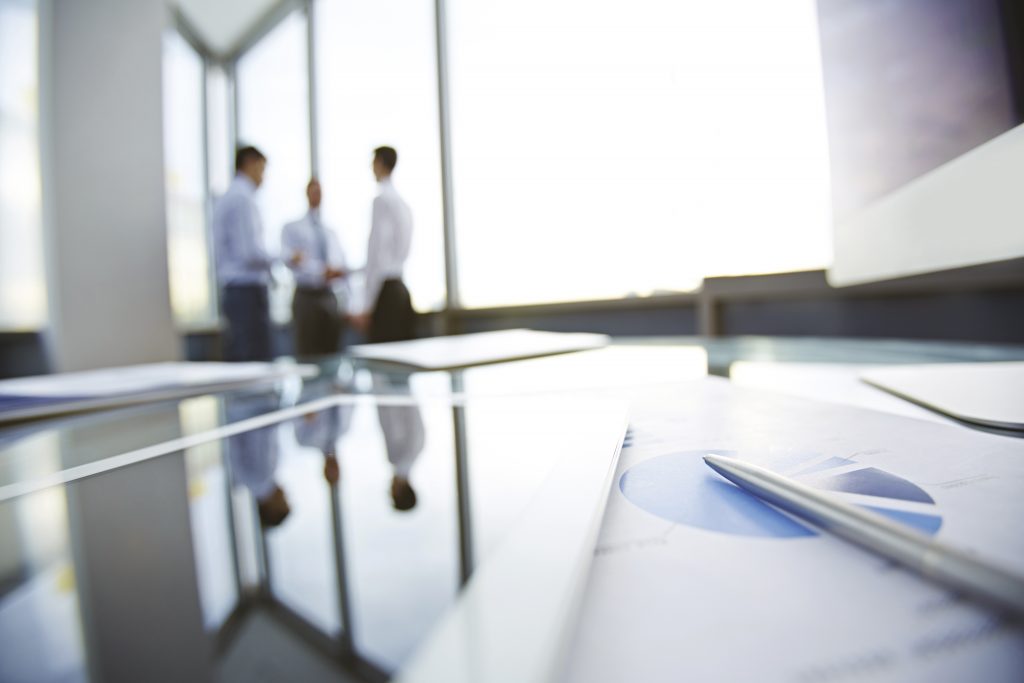
New constraints threaten the future of internal model approaches
At the same time as regulators as a whole express their support for the harmonisation, transparency and comparability of banking models at the European level, a new consultative document published by the Basel Committee on 24 March 2016 partly calls into question the use of internal model approaches when evaluating credit risk. The release of […]
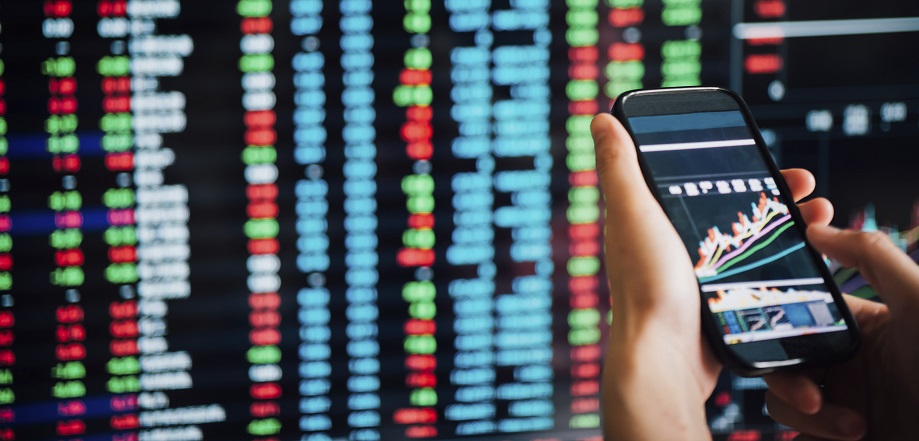
Banks: Five Tactics to Survive Fintech Disruption
The revised Payment Services Directive (PSD2) that comes into force in January 2018 will essentially remove many of the barriers to new players looking to enter the payments market by providing access to customer data and accounts through an EEA wide common legislative platform. Already under pressure from regulatory change and increased competition PSD2 is […]
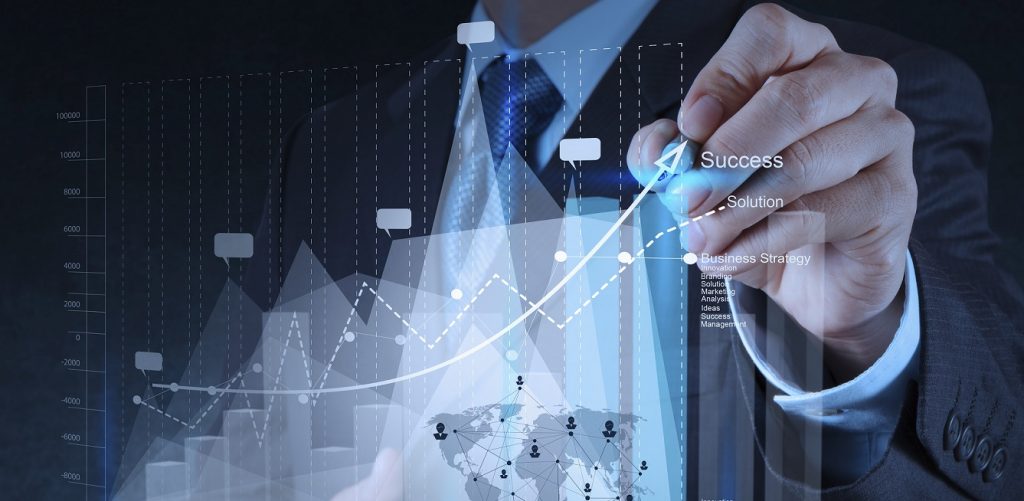
Digital Finance : Meeting Ethics and Compliance Challenges in Financial Services
We recognise digitisation as an important topic for the financial services industry; for this reason, we have developed a content programme with the Economist Intelligence Unit that focuses on how Financial Services companies are adapting their risk and reporting procedures to the new digital environment. This unique programme of thought leadership examines the new challenges in […]
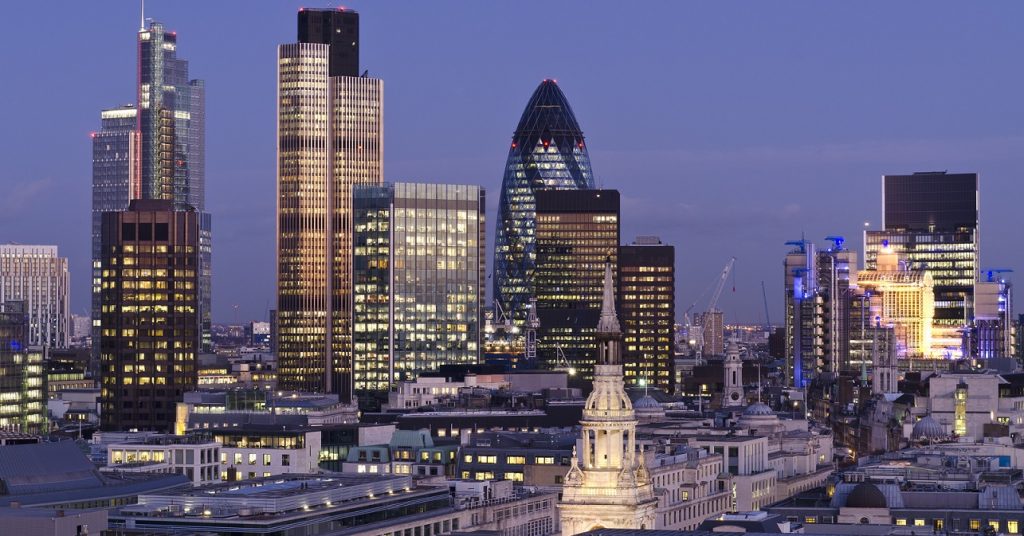
PSD2, FinTechs and Brexit: The Wider Implications
UK Banks are already gearing up for the introduction of the revised Payment Services Directive (PSD2) that comes into force in January 2018. By providing clear guidelines and regulations, the Directive essentially removes the barriers to new players and opens the doors to FinTechs bringing new products and services to the electronic payments market. While […]
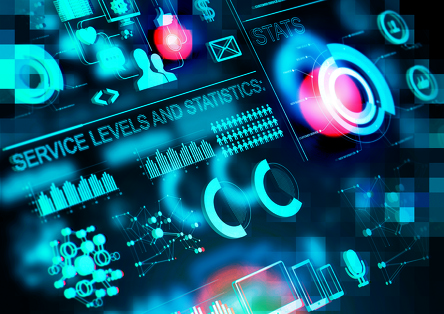
The use of Big Data tools to improve the effectiveness for AML/CFT and KYC policy
A series of initiatives designed to help combat terrorism financing have put electronic payment cards in the spotlight due to the fact they guarantee anonymity in the use of small sums. Announced on 23 November 2015, these initiatives supplement the action plan for combatting the financing of the terrorism presented by the Minister on 18 […]
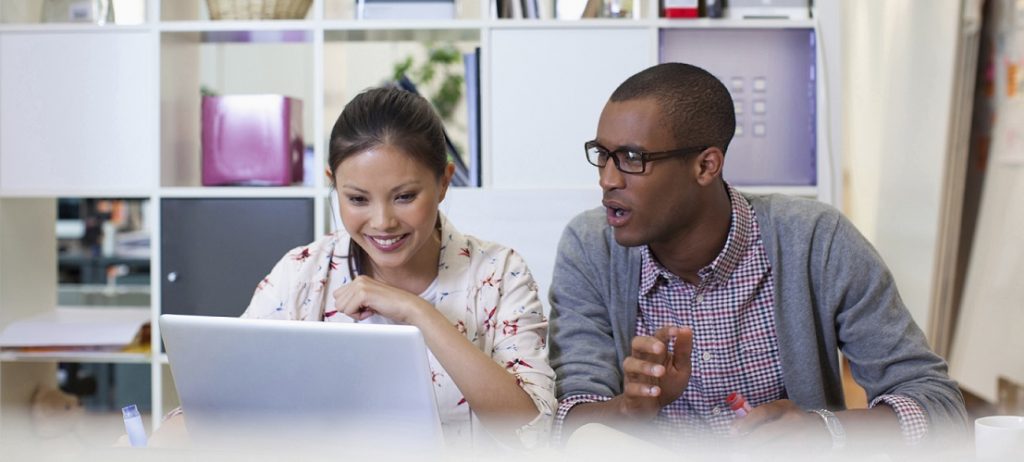
Digital Banking: Lessons from Millennials
By 2025, The Wall Street Journal ([1]) estimated that Generation Y, also known as Millennials, would represent nearly half of the total active population in the world. The challenge for banks is to adapt their strategy to match Generation Y consumer habits and behaviours. Unlike previous generations, Millennials have the increased ability to choose between […]
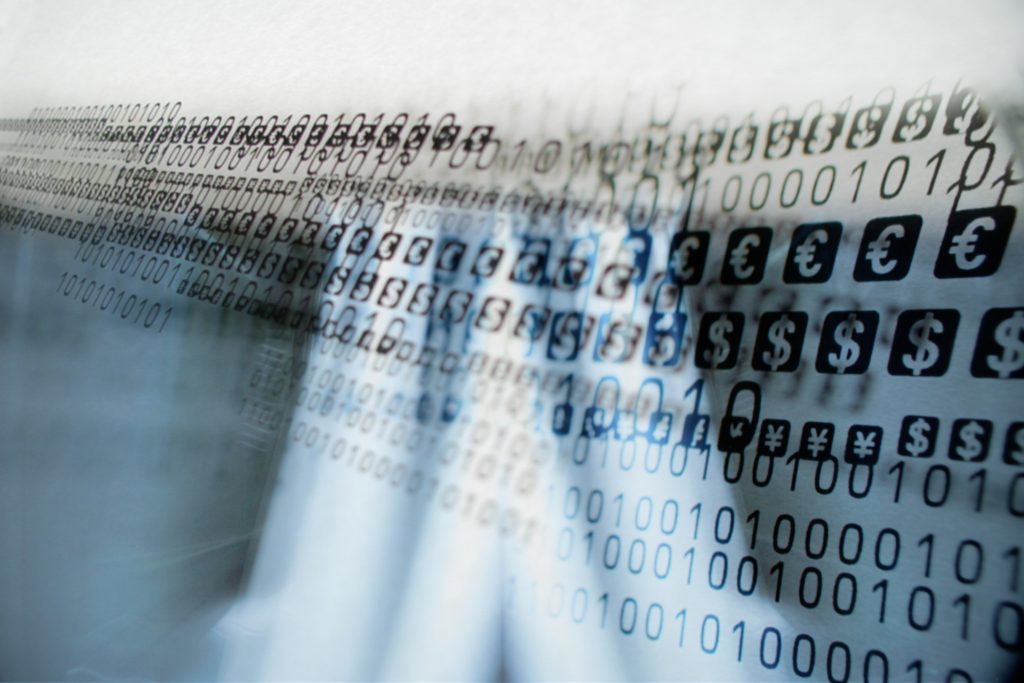
Change in French regulatory landscape for electronic money issuers
The number of electronic money players in the European market has increased in recent years, from 4 in 2010 up to 48 in 2014*. Add to this the fact that numerous players beyond the banking world have also created their own electronic money institutions, including Leetchi, Google and Amazon and the sector is now seen […]
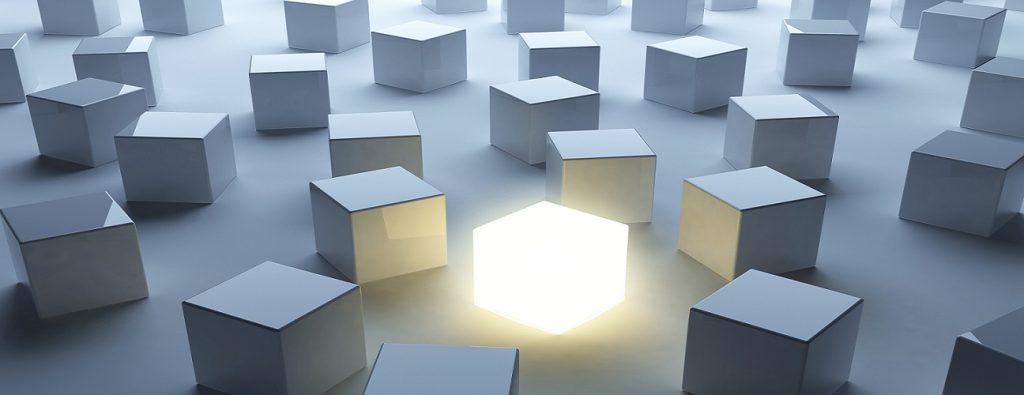
Risk culture and supervision: beyond the box-ticking exercise, striving for fair balance
Since the 2008 crisis, the financial sector has been under scrutiny. Identified as one of the crisis root causes, the importance of risk management framework and risk culture and its interconnectedness to ensure the long run financial stability of each organisation has been revealed. Accordingly, institutions are expected to develop an effective risk management framework […]
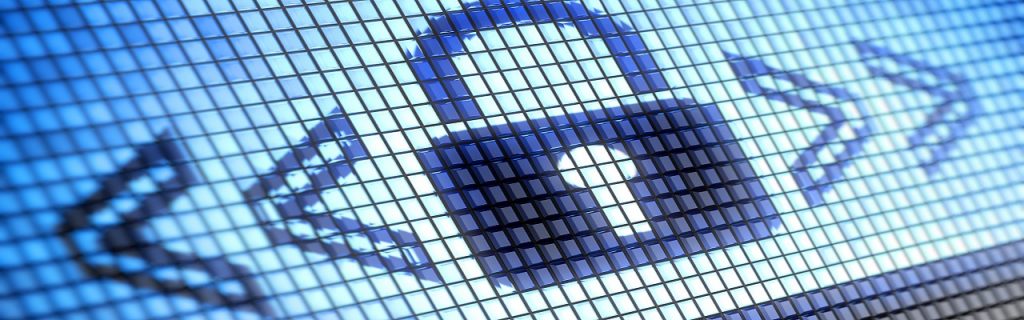
How Innovation is revolutionising the payments landscape
Financial Technology (“Fintech”) companies have kick-started a revolution in the payments landscape. Using state-of-the art technology, fintech companies are transforming how payments are transacted and processed. Banks and traditional payment providers are having to entirely rethink their approach to how they interact with innovations in order to stay on top of their game. Technological change […]
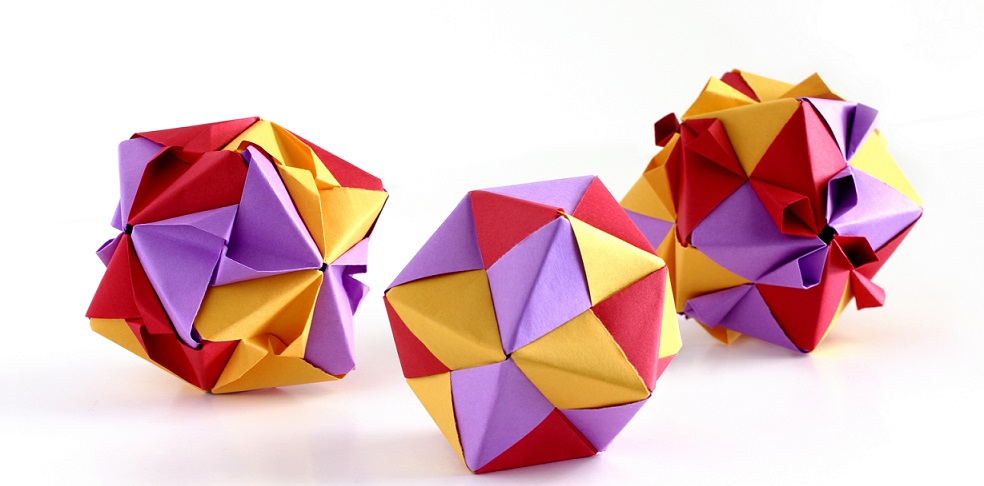
Simplicity is a complex issue
Lorem ipsum dolor sit amet, consectetur adipiscing elit. Sed vehicula sem rhoncus quam suscipit convallis. Quisque eu nulla blandit nibh rutrum convallis. Nullam rhoncus dapibus ex.
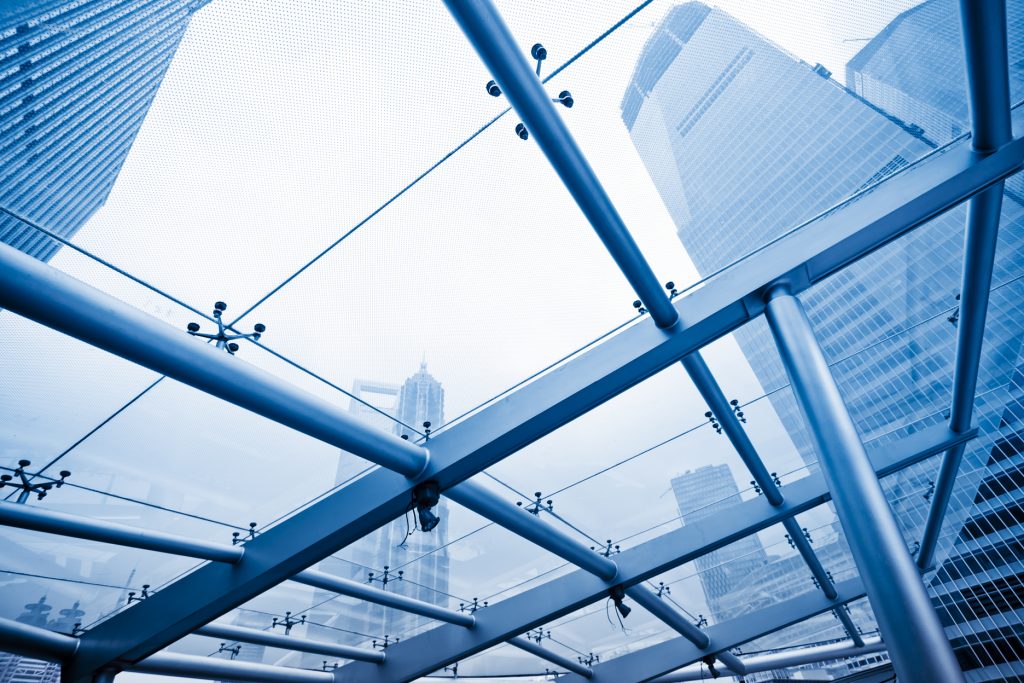
A good bank requires good supervision
To be a ‘good bank’, a bank must be efficient, innovative and trustworthy. Given its central role at the heart of the economy and financial system and the risks associated with fulfilling its role, banks have to operate within an environment subject to laws, regulations and directives. Outcomes can often be subject to the constraints […]
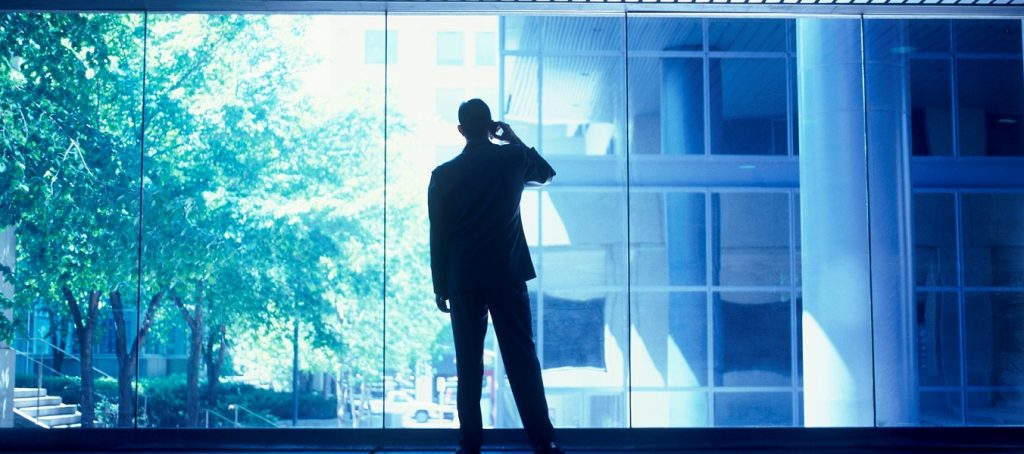
Looking ahead – ECB and NCA focus 2016, and what does it mean for the market participants?
The last five years have been a time of much challenge and change for the Central Banking Fraternity in Europe. Crisis, both economic and political, has been followed by much adjustment and change, including both practical economic and policy interventions, structural change in the form of Banking Union, much new regulation and most recently the […]